The Ethics of Deep Learning
Summary
TLDRThe speaker discusses the ethical implications of deep learning, urging caution in its application. They highlight the unintended consequences that can arise from errors, such as false positives and false negatives, which can lead to harmful outcomes. The talk emphasizes the importance of addressing biases in training data and ensuring that AI systems are responsibly deployed. The speaker also warns about the misuse of technology and encourages those in the field to prioritize its benefits for humanity, while remaining vigilant about potential harm and moral dilemmas.
Takeaways
- 🤖 Deep learning presents ethical concerns, and developers need to ensure it's used for good, not evil.
- ⚠️ Unintended consequences of deep learning systems are a major concern, such as false positives and false negatives in critical fields like healthcare and autonomous driving.
- 📊 High accuracy isn't enough; even a 99.9% accurate model can fail, and failures can have serious consequences.
- 👩⚕️ False positives (e.g., misdiagnosing cancer) and false negatives (e.g., missing a dangerous situation) both have potentially dangerous outcomes.
- 🧠 Bias in training data can lead to biased models, perpetuating societal inequalities like racism, ageism, or sexism.
- 🚗 Developers need to evaluate whether AI systems (e.g., self-driving cars) truly outperform humans, especially in high-stakes scenarios.
- 💼 Deep learning systems marketed to replace human jobs should be scrutinized for their actual performance and potential dangers.
- ⚙️ Technologies can be misused; developers should consider unintended malicious applications, as seen with recommendation algorithms fueling misinformation.
- 👨💻 Ethical responsibility falls on developers to steer powerful AI technologies in the right direction for societal good.
- 🔒 Technologists must question morally questionable projects (e.g., using AI for malicious purposes) and understand they have the freedom to choose ethical paths.
Q & A
What are the potential ethical concerns associated with deep learning?
-Deep learning has both positive and negative potential. Ethical concerns include unintended consequences, biases in data, misuse of technology, and failure to account for errors that can impact human lives.
What are type 1 and type 2 errors in deep learning, and why are they important?
-A type 1 error is a false positive, where something is wrongly identified as present. A type 2 error is a false negative, where something is missed that should have been identified. Both types of errors can have serious consequences, especially in fields like healthcare or autonomous driving.
How does high accuracy in a model not always guarantee its reliability?
-Even with a 99.9% accuracy rate, the remaining 0.1% can still lead to significant errors, especially in high-risk scenarios. It is crucial to consider the real-world impact of the mistakes the model may make.
Why is it important to understand the biases present in training data for deep learning models?
-Training data may reflect the biases of the humans who created it, which can lead to biased models. For example, if the training data reflects discriminatory hiring decisions, the resulting model will also be biased. Identifying and addressing these biases is essential for building fair systems.
What steps can be taken to mitigate unintended biases in a deep learning model?
-Avoid including features directly related to sensitive attributes like age, sex, or race. Additionally, carefully analyze the features for indirect biases, such as years of experience correlating with age, and ensure transparency about the model's limitations.
When should deep learning systems be used to replace human decision-making?
-Deep learning systems should only replace human decision-making if they are demonstrably better than humans in that context. For life-critical areas, such as medical diagnoses or driving, they should be used as supplementary tools rather than outright replacements to ensure safety.
Why should technologists think about the unintended applications of their work?
-Technologists need to consider how their work might be repurposed in harmful ways, as others may misuse it for malicious purposes. Being aware of potential negative uses can guide more ethical development and avoid causing harm.
What is an example of unintended consequences of deep learning technology being used for malicious purposes?
-One example is using recommendation algorithms, originally designed for suggesting products, to spread misinformation or manipulate public opinion through targeted content on social media.
What advice is given to deep learning practitioners who face ethical dilemmas in their jobs?
-Practitioners should remember that deep learning is a hot field, and if they are asked to do something morally questionable, they can find another job. They should prioritize their ethics and refuse to participate in harmful projects.
What should researchers consider before publishing research involving deep learning models?
-Researchers should think twice before publishing research that could have negative social consequences, such as cracking passwords or predicting sensitive personal information. They should consider whether the potential applications are ethical and beneficial to society.
Outlines
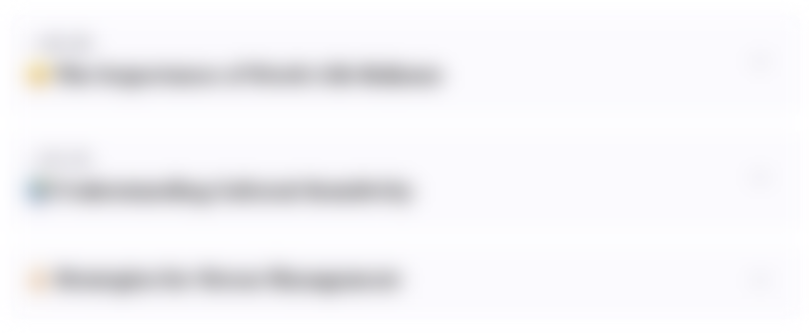
Esta sección está disponible solo para usuarios con suscripción. Por favor, mejora tu plan para acceder a esta parte.
Mejorar ahoraMindmap
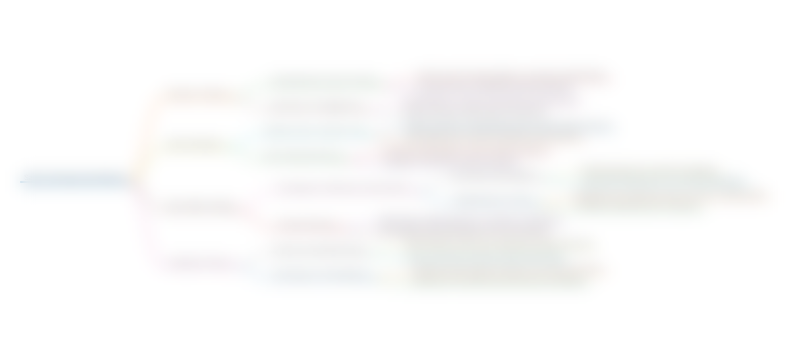
Esta sección está disponible solo para usuarios con suscripción. Por favor, mejora tu plan para acceder a esta parte.
Mejorar ahoraKeywords
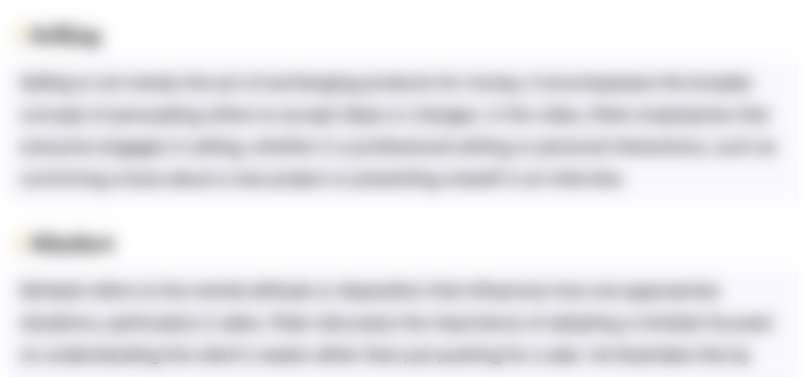
Esta sección está disponible solo para usuarios con suscripción. Por favor, mejora tu plan para acceder a esta parte.
Mejorar ahoraHighlights
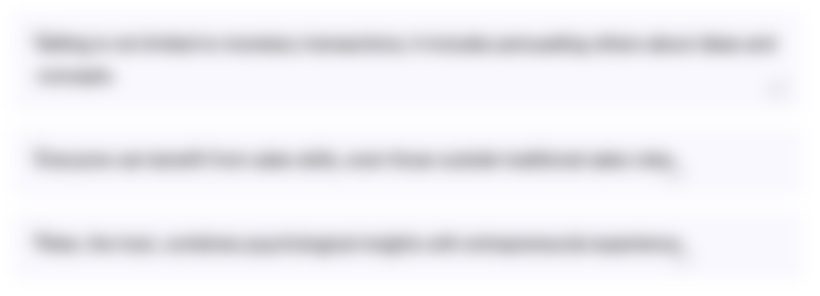
Esta sección está disponible solo para usuarios con suscripción. Por favor, mejora tu plan para acceder a esta parte.
Mejorar ahoraTranscripts
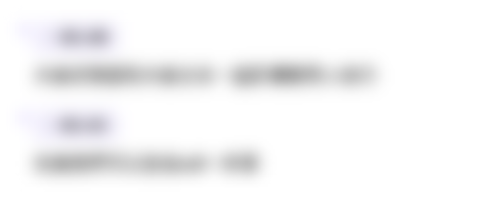
Esta sección está disponible solo para usuarios con suscripción. Por favor, mejora tu plan para acceder a esta parte.
Mejorar ahoraVer Más Videos Relacionados
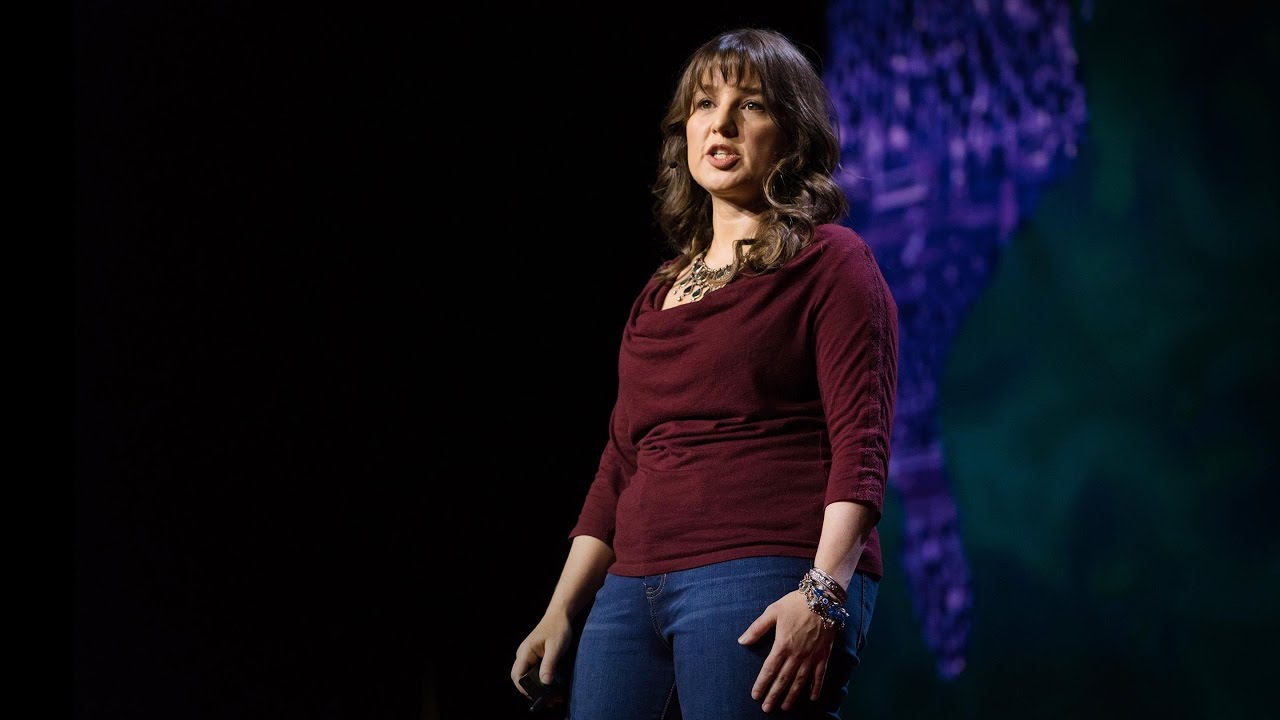
Machine intelligence makes human morals more important | Zeynep Tufekci

Responsible Data Management – Julia Stoyanovich

The Future of AI | Peter Graf | TEDxSonomaCounty
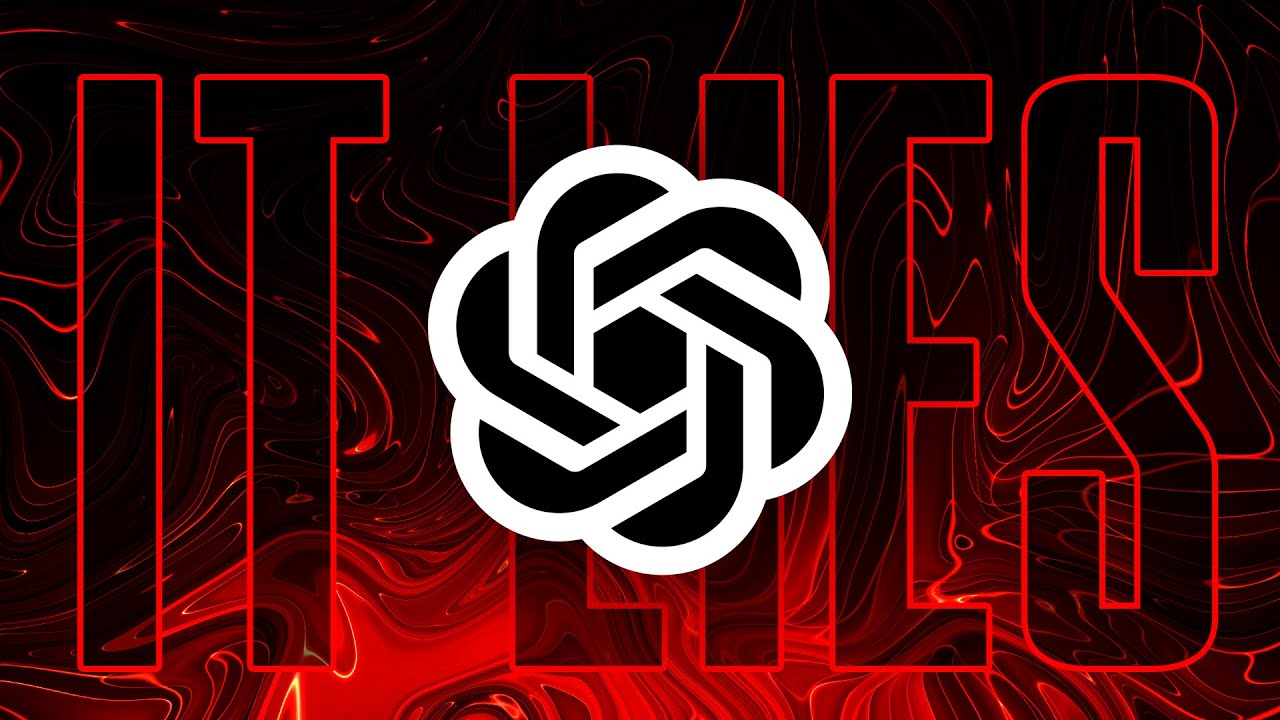
AI - We Need To Stop
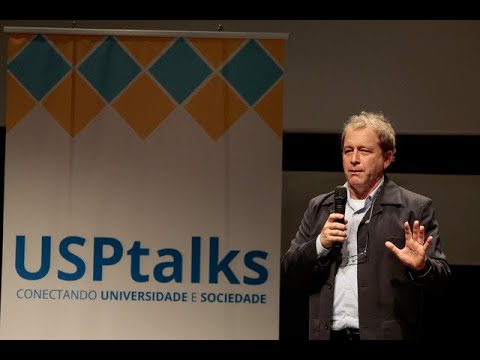
Implicações éticas e sociais da inteligência artificial - Marcelo Finger - USP Talks #48
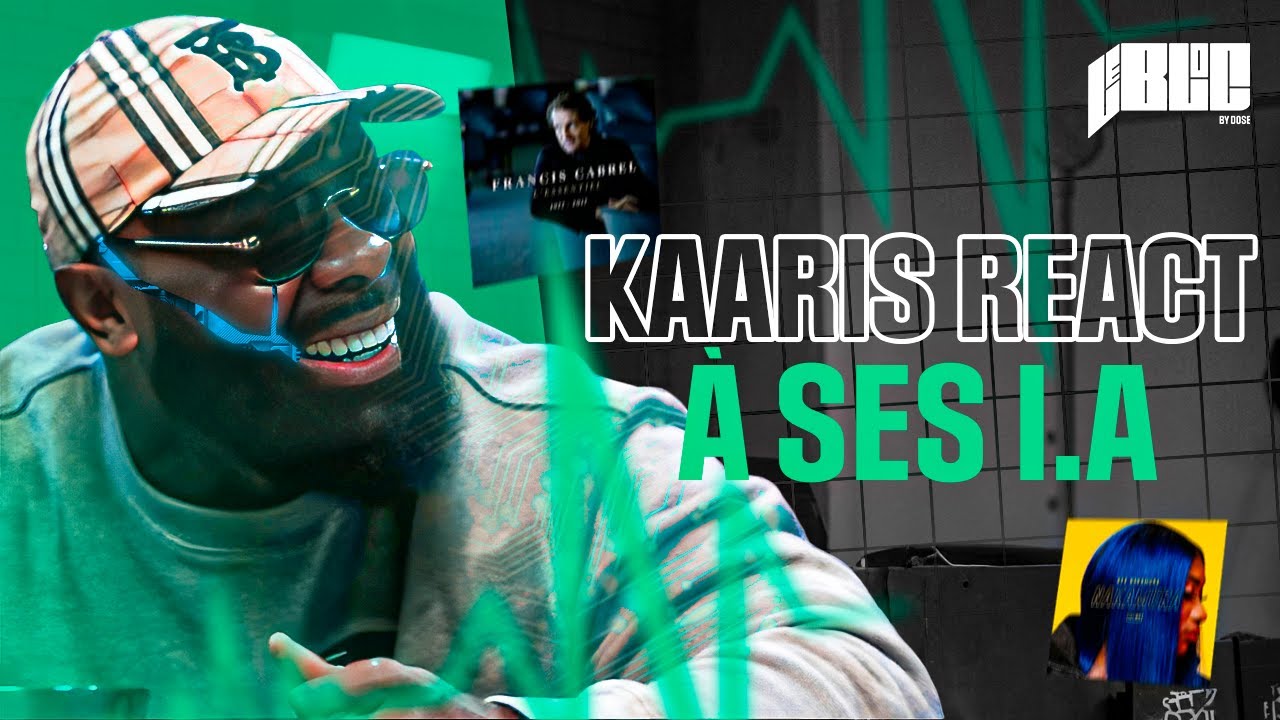
Kaaris réagit à ses I.A (Chou Daddy, Aya Nakamura, Francis Cabrel..) - LE BLOC #1
5.0 / 5 (0 votes)