Data & Infographics: Crash Course Navigating Digital Information #8
Summary
TLDRIn this Crash Course episode, John Green explores the nuances of data and statistics, emphasizing the importance of context and source reliability. He discusses how data can be powerful yet deceptive, and urges viewers to critically evaluate its relevance and the credibility of its sources. Green also highlights the potential for manipulation in data visualization, illustrating how charts can be designed to mislead. The episode concludes with a call for vigilance in interpreting data and infographics to ensure quality decision-making.
Takeaways
- 😀 Data is powerful evidence that can be quickly absorbed but is not inherently neutral due to human involvement in its collection, interpretation, and presentation.
- 🔍 The context and source of data are crucial; even seemingly positive statistics can be misleading if not properly contextualized.
- 📊 Data visualizations like charts and infographics can be both informative and misleading, depending on how they are designed and what data they represent.
- 🤔 It's important to critically evaluate data by asking if it supports the claim and if the source is reliable.
- 🏓 The example of Serena Williams and tennis penalties illustrates how raw data can be misinterpreted without considering the rate of occurrence.
- 🔎 Lateral reading is essential to understand who commissioned and conducted research to assess the reliability of data sources.
- 👀 The 'seeing is believing' trap can lead to uncritical acceptance of data, which is why understanding how data is presented is vital.
- 🌐 Data can be made to seem more or less significant by adjusting the scale and context in which it is presented, as shown by climate change and graduation rate charts.
- 📉 Misleading data visualizations can be created by using an inappropriate scale or by focusing on a narrow aspect of the data.
- 🧐 Always check the accuracy, relevance, source reliability, and honesty of data presentation when encountering data visualizations.
Q & A
What is the key message in the introduction of the Crash Course episode?
-The key message is that data, like statistics, needs to be placed in context to understand its true meaning. Simply seeing numbers without understanding their source and context can be misleading.
Why does John Green mention the survey he conducted with 10 Crash Course employees?
-John Green uses this example to show how data can be manipulated. Although 90% of people surveyed said they loved Crash Course, it’s misleading because the survey was conducted with only 10 employees, making it unreliable.
What does Mark Twain’s quote, 'There are three kinds of lies: Lies, damned lies, and statistics,' imply?
-The quote highlights that statistics, despite appearing factual and neutral, can be used to mislead or manipulate perceptions depending on how they are presented or interpreted.
How does the 2015 Stanford History Education Group study relate to people's perception of data?
-The study found that many middle schoolers believed data in a comment was credible without checking its source, showing how easily people can be swayed by the mere presence of statistics, even when there is no reason to trust the data.
What’s the problem with Glenn Greenwald's tweet about male tennis players being punished more often?
-Glenn Greenwald’s tweet is misleading because it only shows the total number of punishments, not the rate of punishment relative to misbehavior. To determine whether men are punished more frequently, we need to know how often both men and women misbehave.
What is 'lateral reading,' and how does it help in evaluating data?
-Lateral reading involves opening new tabs to research the credibility and authority of the data's source. This helps verify whether the source is reliable, why the data was collected, and whether the source has a vested interest in the results.
Why is the '500 million straws per day' statistic often criticized?
-This statistic, which is frequently cited, originated from a 9-year-old who conducted informal research by calling straw manufacturers. The figure lacks scientific rigor, making it unreliable, though widely circulated.
What should we look for when analyzing data visualizations like charts or infographics?
-We need to ensure the data is accurate, comes from a reliable source, and is presented fairly without manipulation. Visualizations should not sacrifice accuracy for aesthetics or mislead through techniques like inappropriate scaling.
How can manipulating the y-axis of a graph mislead viewers, as seen in the climate change and graduation rate examples?
-Manipulating the y-axis can either exaggerate or downplay trends. In the climate change example, zooming out made the temperature change appear minor, while zooming in on the graduation rate chart made a modest increase look dramatic.
What is the 'proportional ink principle' in data visualization?
-The proportional ink principle states that the size of inked areas in a chart should be proportional to the data values they represent. This ensures that visual representations are accurate and not exaggerated or minimized.
Outlines
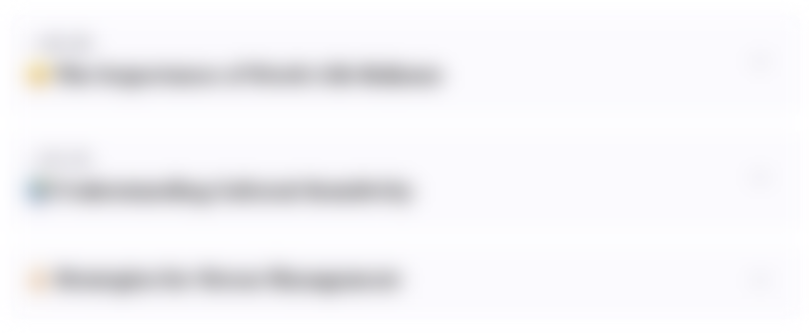
Esta sección está disponible solo para usuarios con suscripción. Por favor, mejora tu plan para acceder a esta parte.
Mejorar ahoraMindmap
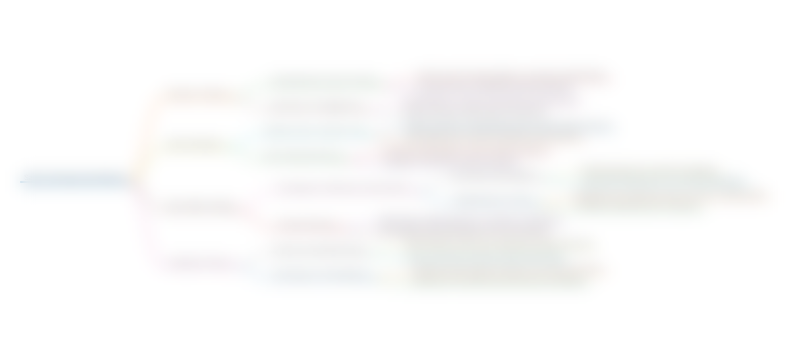
Esta sección está disponible solo para usuarios con suscripción. Por favor, mejora tu plan para acceder a esta parte.
Mejorar ahoraKeywords
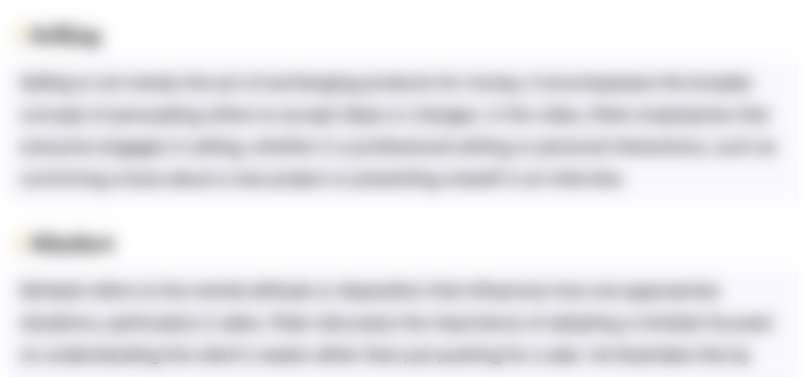
Esta sección está disponible solo para usuarios con suscripción. Por favor, mejora tu plan para acceder a esta parte.
Mejorar ahoraHighlights
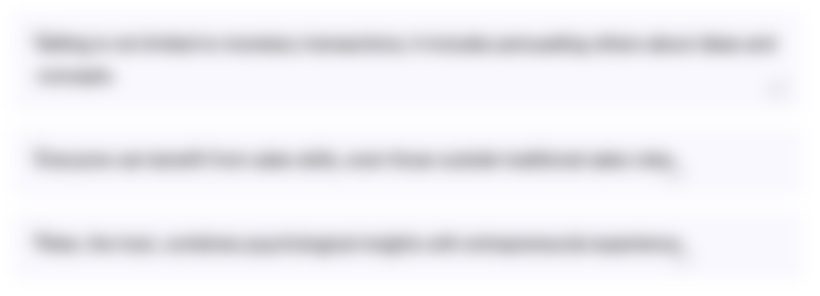
Esta sección está disponible solo para usuarios con suscripción. Por favor, mejora tu plan para acceder a esta parte.
Mejorar ahoraTranscripts
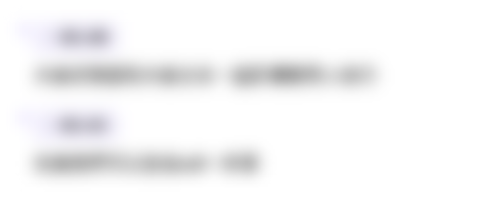
Esta sección está disponible solo para usuarios con suscripción. Por favor, mejora tu plan para acceder a esta parte.
Mejorar ahoraVer Más Videos Relacionados
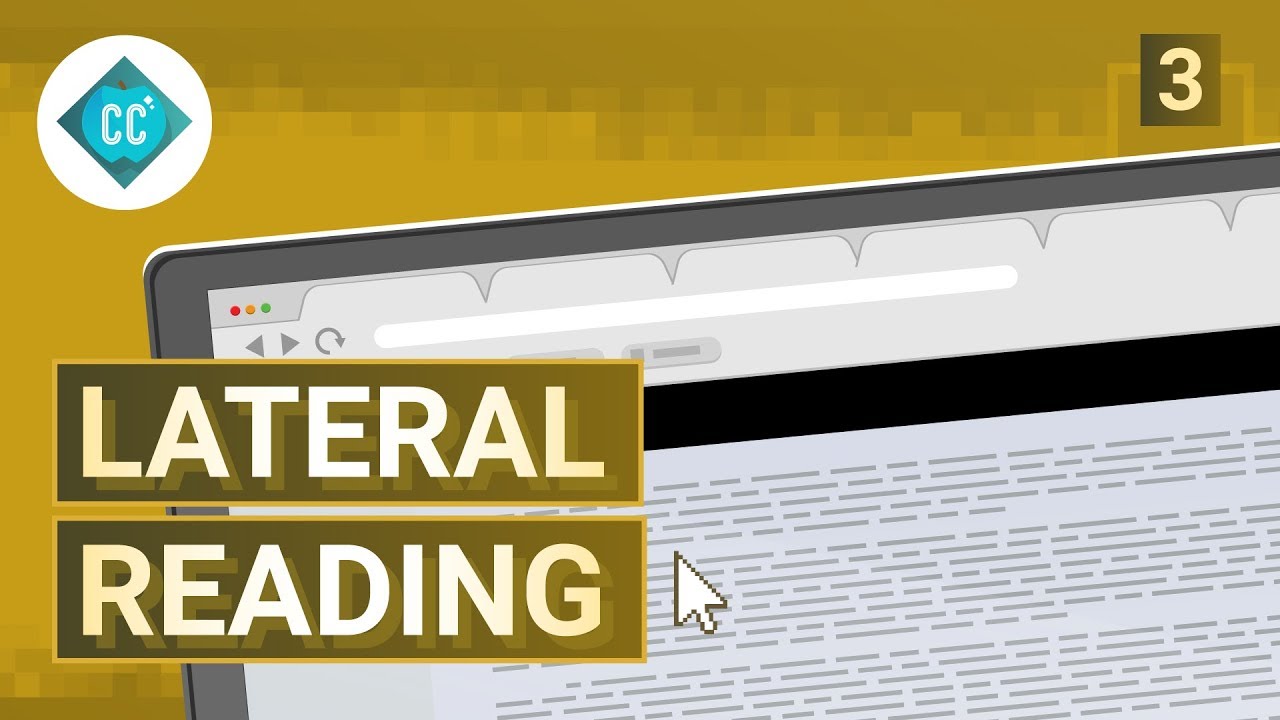
Check Yourself with Lateral Reading: Crash Course Navigating Digital Information #3
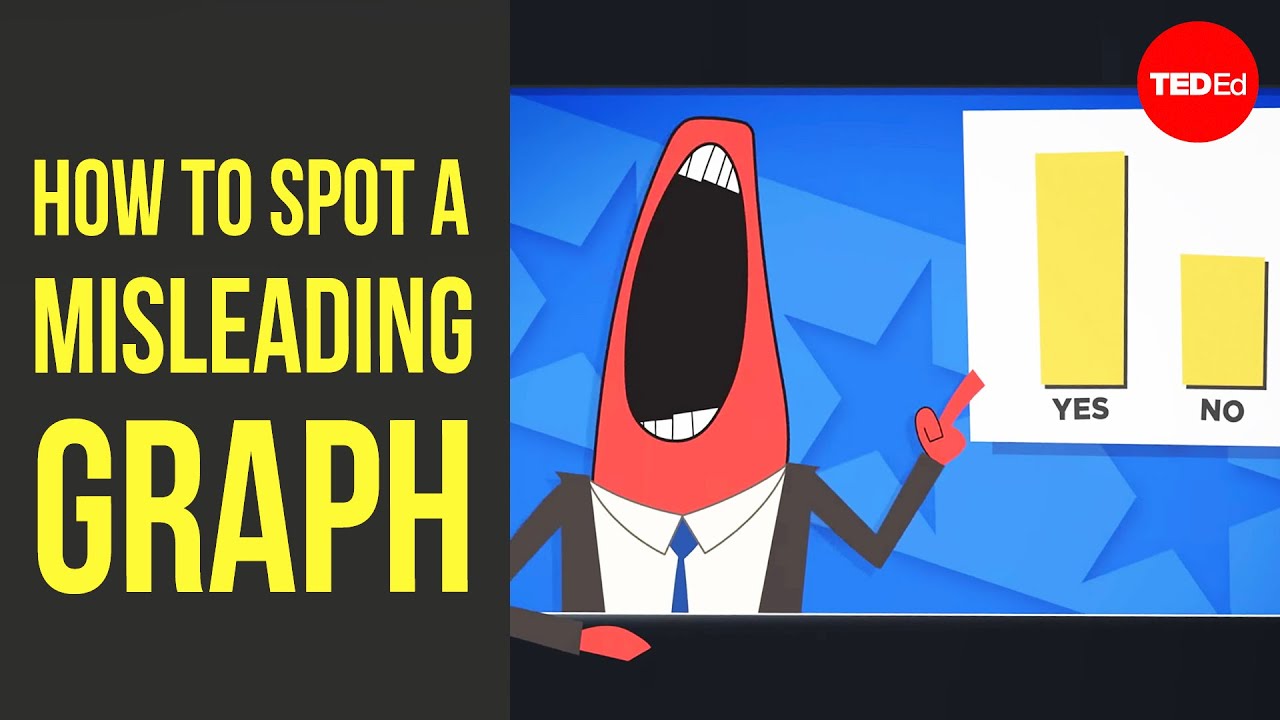
How to spot a misleading graph - Lea Gaslowitz
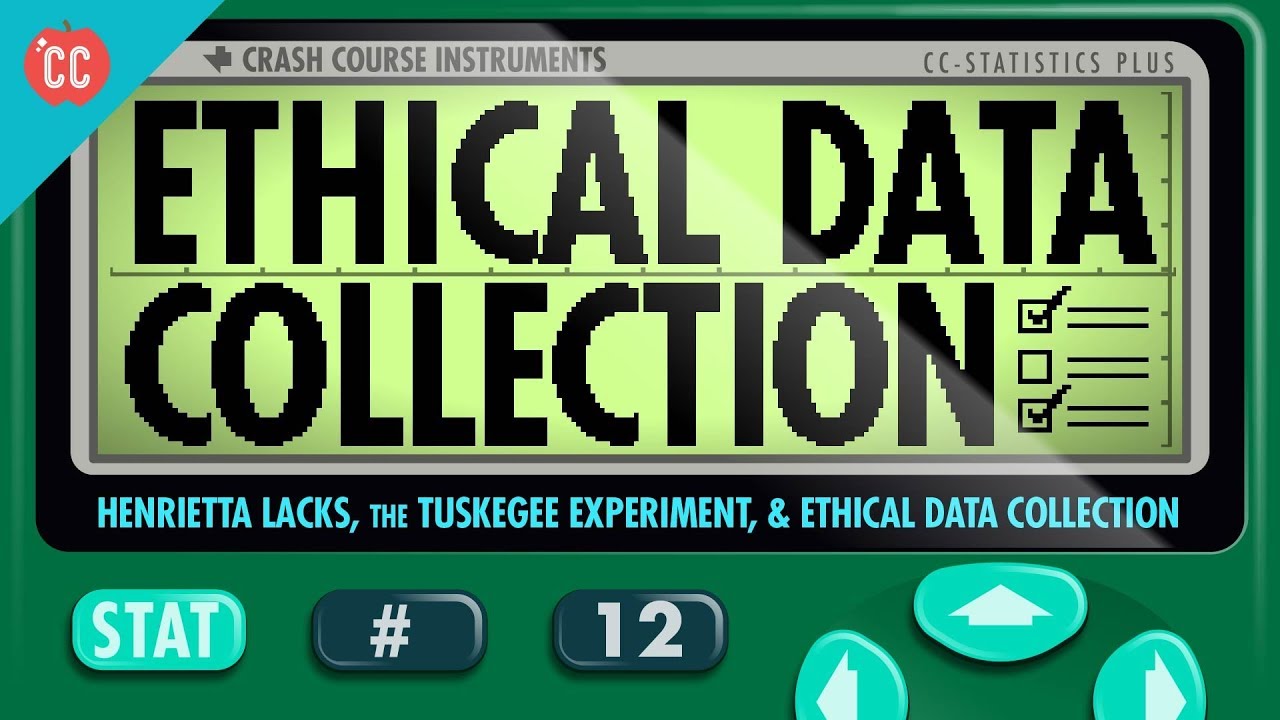
Henrietta Lacks, the Tuskegee Experiment, and Ethical Data Collection: Crash Course Statistics #12
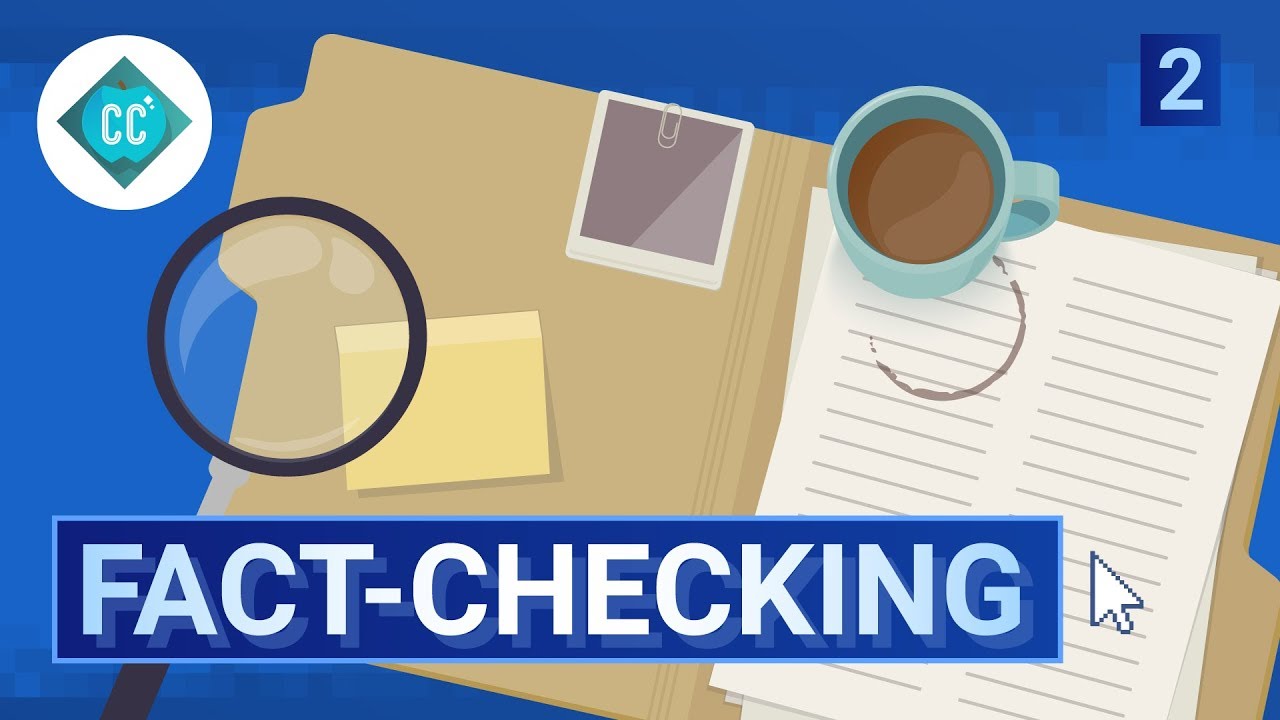
The Facts about Fact Checking: Crash Course Navigating Digital Information #2

Measures of Spread: Crash Course Statistics #4
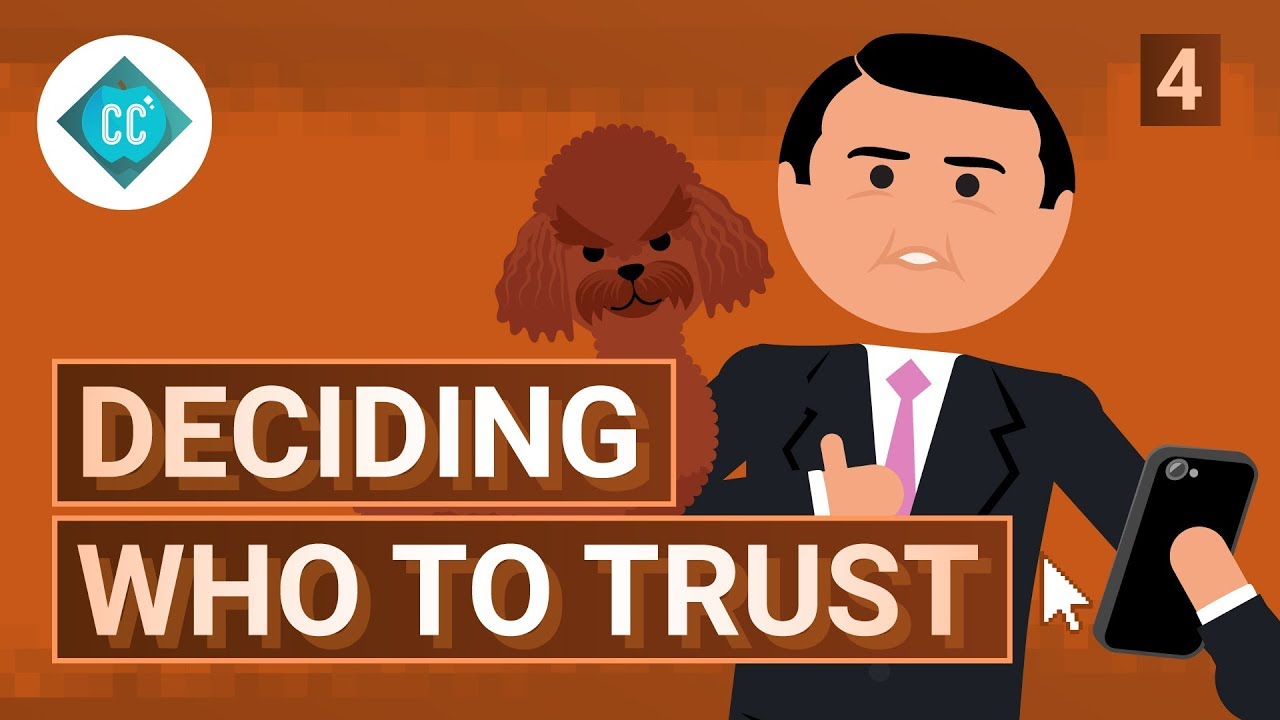
Who Can You Trust? Crash Course Navigating Digital Information #4
5.0 / 5 (0 votes)