Goldfish Bowl RAG Intro
Summary
TLDRВ этом видео представлен обзор проекта RAG (Retrieval Augmented Generation), который использует модель для обучения с помощью дополнительных контекстов в виде ссылочных текстов. Цель - научить модель эффективно комбинировать информацию из разных источников для ответа на вопросы. Проект включает в себя создание уникальных запросов, основанных на ссылочных текстах, и оценку двух генерируемых ответов по шкале от 1 до 3 по пяти критериям. Основные требования к запросам - использование ссылочных текстов и избегание общих тем. Подробно рассматриваются примеры хороших и плохих запросов, а также методика оценки ответов.
Takeaways
- 🐟 RAG (Retrieval Augmented Generation) - это метод, при котором модель обучается использовать дополнительный контекст из ссылочных текстов для ответа на запросы.
- 🔍 В рамках проекта Goldfish Bowl, участникам предлагается создавать запросы, используя ссылочные тексты, чтобы обучить модель эффективно комбинировать различную информацию.
- 📝 Создание запросов должно быть основано на ссылочных текстах, и они должны быть достаточно конкретными, чтобы избежать общих или неправильных запросов.
- 🚫 Запрещено использовать чат-ботов или другие LLM (Large Language Models) для создания запросов, что может привести к блокировке участника.
- ✅ Хорошие примеры запросов должны быть основаны на ссылочных текстах и включать в себя основное требование и дополнительное ограничение.
- 📑 Для каждого запроса необходимо добавить от двух до десяти ссылочных текстов, каждый из которых должен содержать не менее 150 слов.
- ✅ Ответы модели оцениваются по шкале от 1 до 3 в пяти категориях: основанность на ссылочных текстах, достоверность, полезность, следование инструкциям и стиль написания.
- 🔍 Оценка основанности на ссылочных текстах и достоверности является наиболее важным критерием при выборе предпочитаемого ответа.
- 📝 Для многоступенчатых задач предпочтение отдается ответу, который лучше всего удовлетворяет запросы и использует ссылочные тексты для продолжения диалога.
- 👍 Важно тщательно проверять и оценивать каждый ответ, чтобы обучить модель правильно использовать ссылочные тексты и давать точные ответы.
Q & A
Что означает аббревиатура RAG?
-RAG означает 'retrieval augmented generation', что подразумевает использование модели в определенном контексте для обучения ее предоставлять ответы на основе дополнительных контекстов, предоставленных через ссылки на тексты.
Каковы ключевые требования к написанию запроса (prompt) для проекта RAG?
-Запрос должен быть основан на ссылочных текстах, иметь как минимум 10 слов, не содержать вежливых фраз, быть достаточно сложным с основным запросом и дополнительными ограничениями, избегать словосложием и не использовать ограничения по количеству слов или предложений.
Что такое ссылочный текст и какова его роль в проекте RAG?
-Ссылочный текст - это дополнительная информация, предоставленная для модели, чтобы помочь ей ответить на запрос. Модель использует этот текст специфического контекста для генерации ответа.
Какие критерии используются для оценки ответов модели в рамках проекта RAG?
-Ответы оцениваются по пяти критериям: основанность на ссылочном тексте, достоверность, полезность, следование инструкциям и стиль написания.
Как определяется 'основанность на ссылочном тексте' в контексте оценки?
-Ответ считается основанным на ссылочном тексте, если все утверждения напрямую основаны на информации из ссылочных текстов и не содержат информации из других источников.
Что подразумевается под категорией 'достоверность' при оценке ответов?
-Категория 'достоверность' оценивается на основе того, являются ли утверждения в ответе правильными или нет, основаны ли они на достоверных и проверенных фактах.
Какова цель использования системы оценки по шкале от 1 до 3 для каждого из пяти критериев?
-Целью использования шкалы оценки является детальное измерение качества ответа модели по каждому из критериев, что позволяет сделать более обоснованное и точечное сравнение между двумя ответами.
Почему важно использовать Liker score при выборе предпочитаемого ответа?
-Liker score используется для указания предпочтения одного из двух ответов и дает возможность оценить, насколько один ответ лучше другого по шкале от 'много лучше' до 'равноценно'.
Что означает 'следование инструкциям' в оценке и как это влияет на результат?
-Следование инструкциям оценивается на основе того, как хорошо модель понимает и выполняет требования запроса пользователя, включая ограничения и основные запросы.
Какой является последовательность действий для создания и оценки запроса в рамках проекта RAG?
-Последовательность действий включает в себя написание запроса, основанного на ссылочных текстах, предоставление двух ответов модели, оценку каждого ответа по пяти критериям, выбор предпочитаемого ответа с использованием Liker score и обоснование выбора.
Outlines
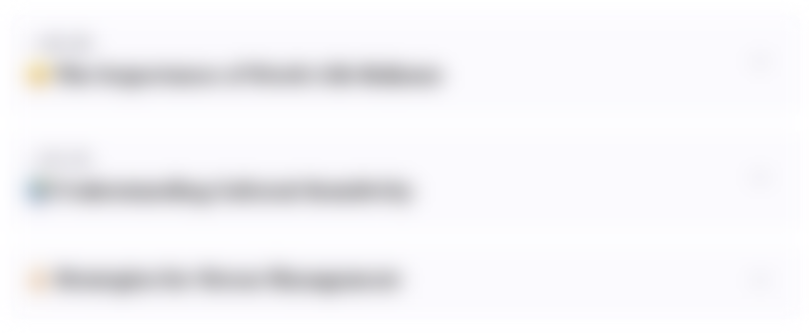
Esta sección está disponible solo para usuarios con suscripción. Por favor, mejora tu plan para acceder a esta parte.
Mejorar ahoraMindmap
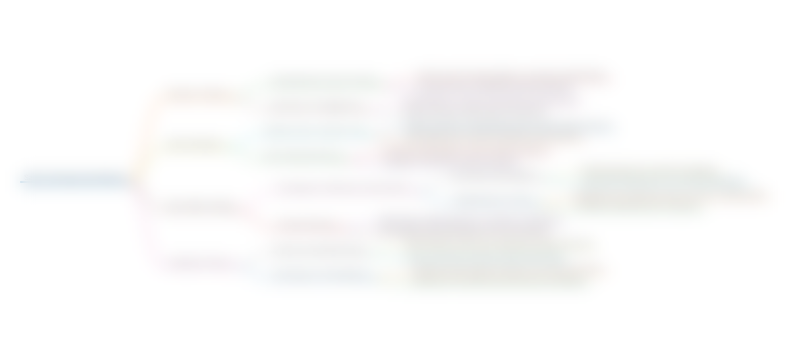
Esta sección está disponible solo para usuarios con suscripción. Por favor, mejora tu plan para acceder a esta parte.
Mejorar ahoraKeywords
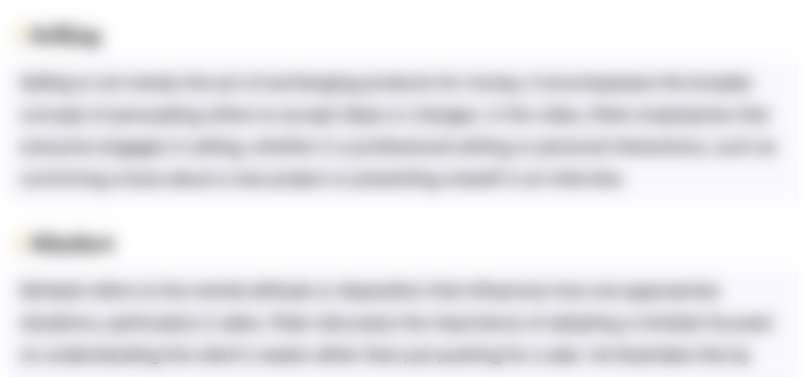
Esta sección está disponible solo para usuarios con suscripción. Por favor, mejora tu plan para acceder a esta parte.
Mejorar ahoraHighlights
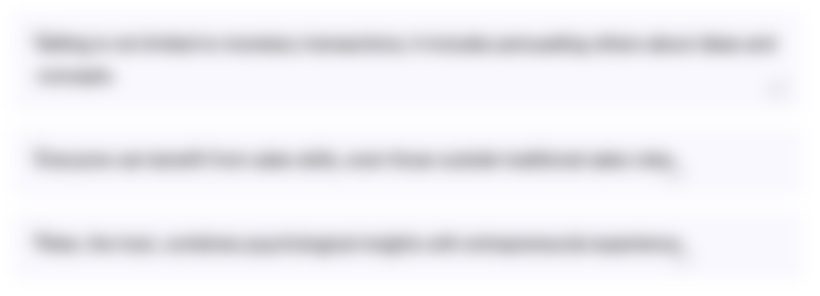
Esta sección está disponible solo para usuarios con suscripción. Por favor, mejora tu plan para acceder a esta parte.
Mejorar ahoraTranscripts
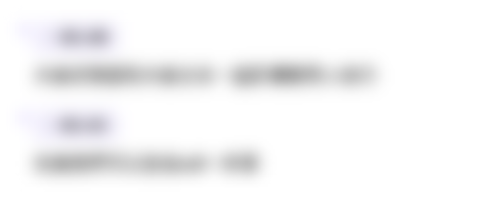
Esta sección está disponible solo para usuarios con suscripción. Por favor, mejora tu plan para acceder a esta parte.
Mejorar ahoraVer Más Videos Relacionados
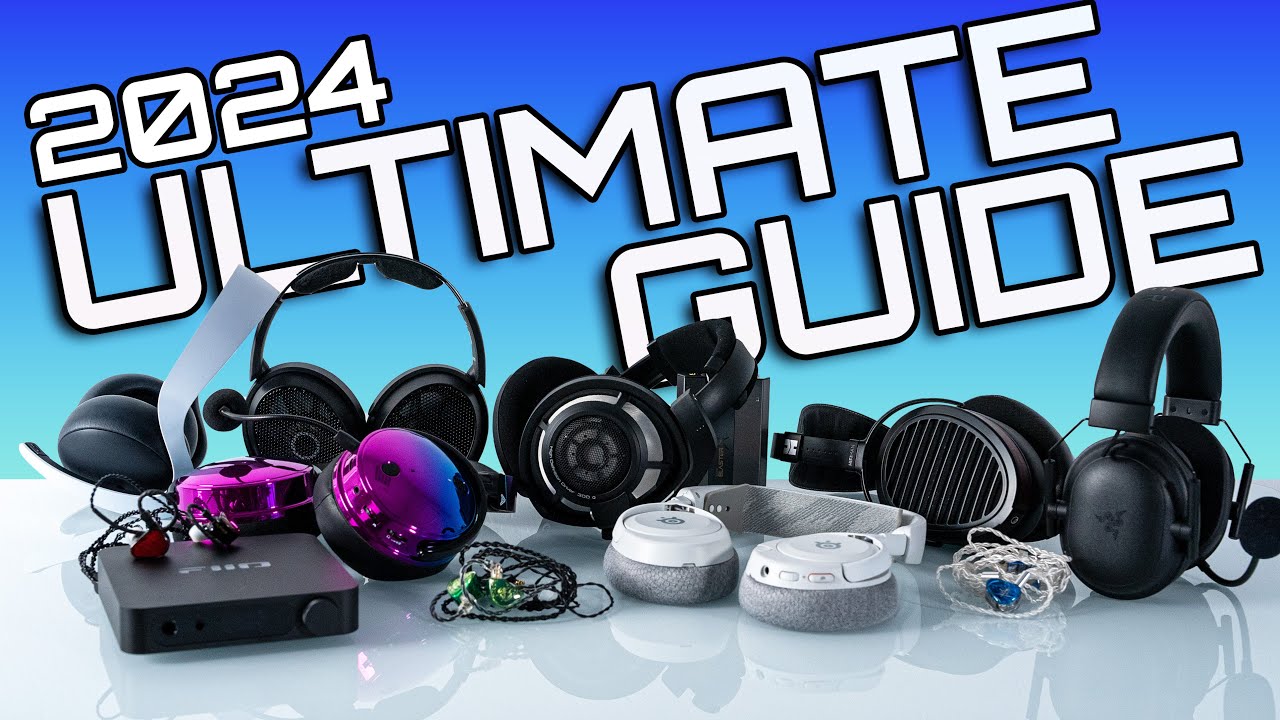
🎮 GAMING AUDIO GUIDE - Top Gaming Audio Picks at ANY Price 2024 - IEMs, Headphones, and Headsets!
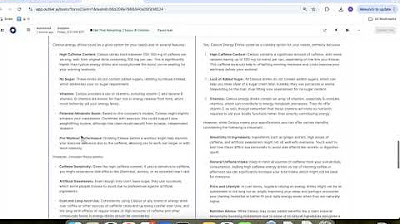
RAG Rewrite Instructions (Outlier)
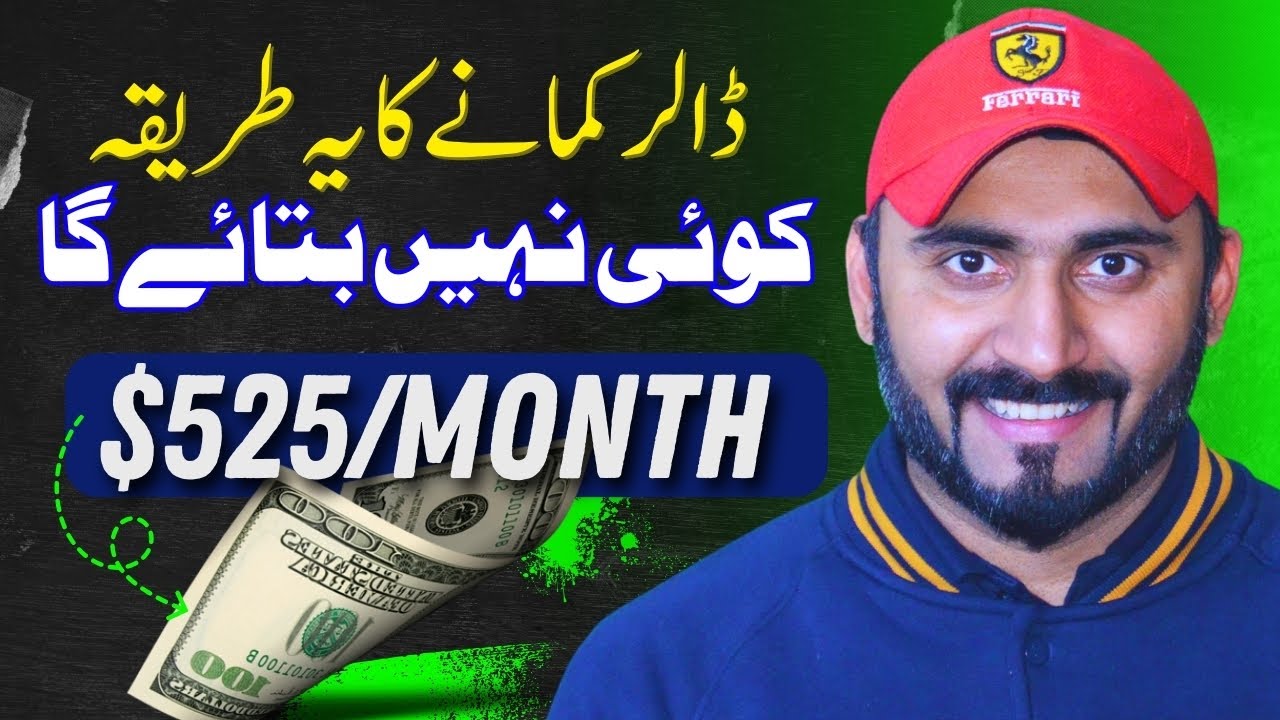
Make Money Blogging in 2024 Google AdSense ( My Secret Online Earning Method)
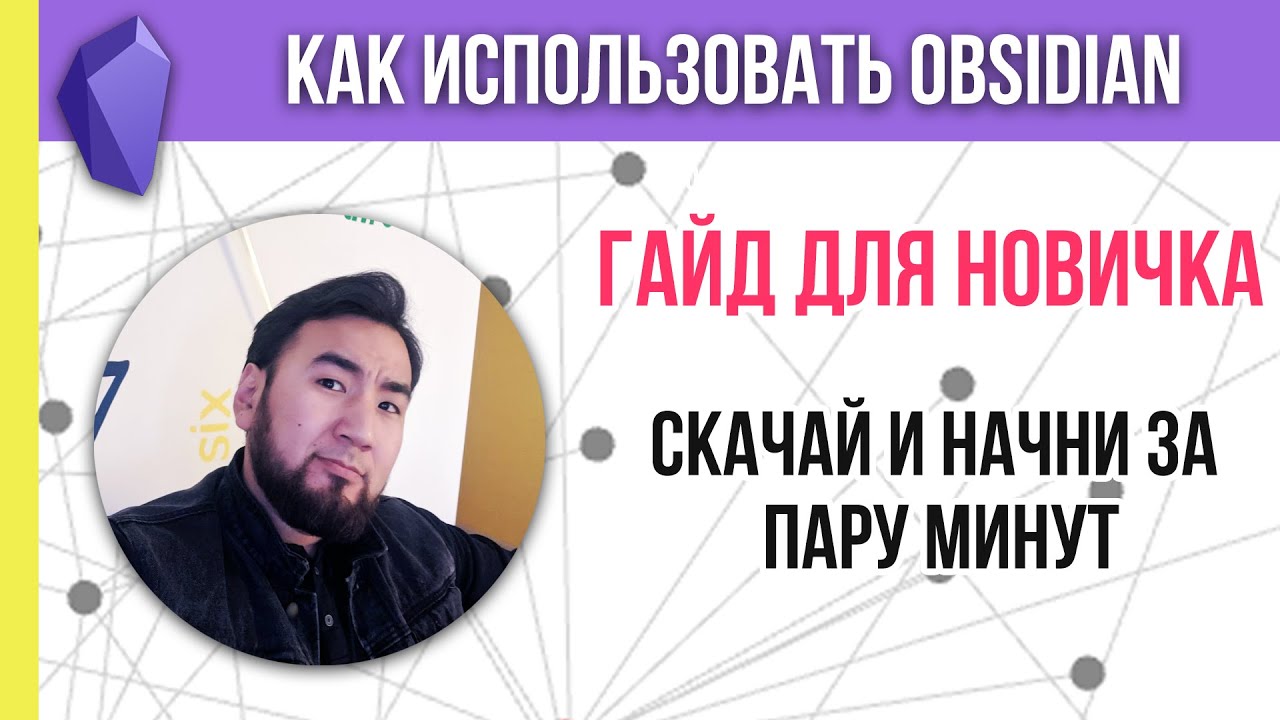
Обсидиан для начинающих. Как использовать Obsidian
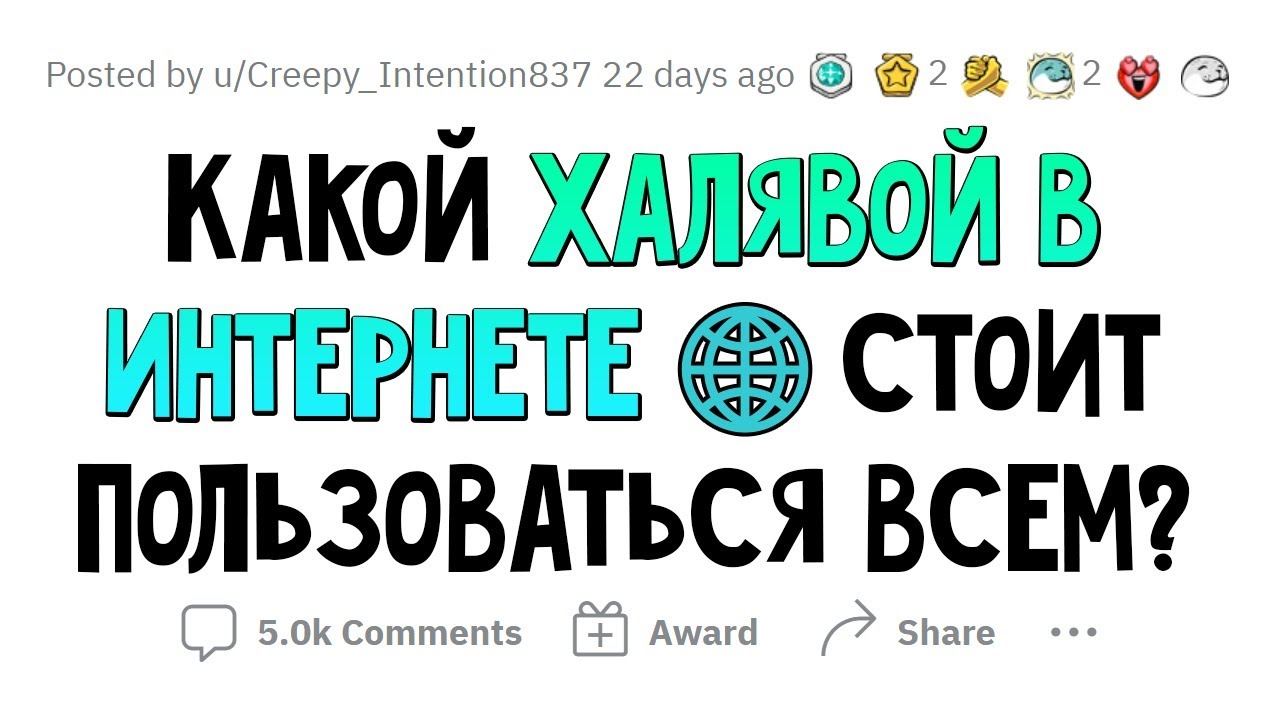
ХАЛЯВА ИЗ ИНТЕРНЕТА ДЛЯ КАЖДОГО
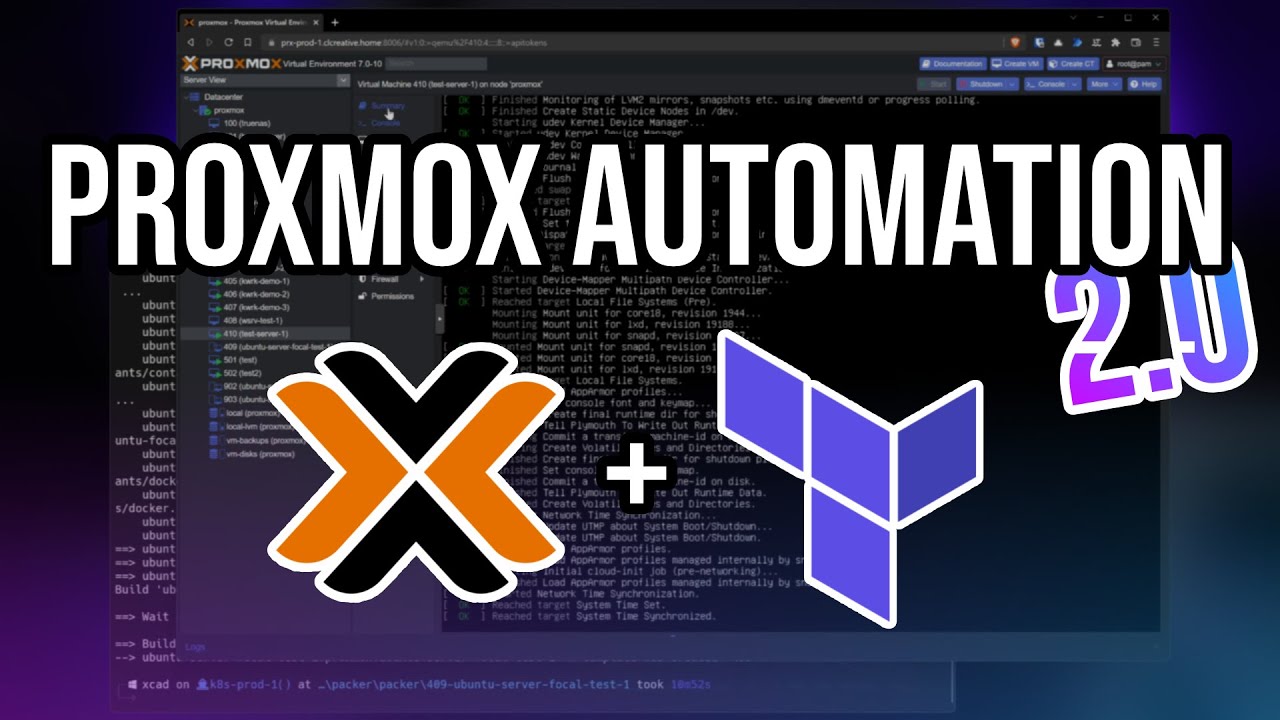
Proxmox virtual machine *automation* in Terraform
5.0 / 5 (0 votes)