RAG Rewrite Instructions (Outlier)
Summary
TLDRВ этом видео представлен обзор обновленных инструкций и руководства по внедрению переписей в проект Goldfish Rag. Основная идея - улучшение обучения модели, добавляя переписи, которые исправляют несовершенства в ответах. Переписи помогают модели учиться идеалам ответов, учитывая критерии, такие как точность, полезность и приятность. Видео объясняет, когда и как следует выполнять переписи, используя диаграмму для определения случаев, требующих корректировки. Также рассматривается редактор для внесения изменений, а также процесс оформления и оправдания изменений в рамках обучения модели.
Takeaways
- 😀 В видео представлен обзор обновленных инструкций и руководства по внедрению переписей в проект Goldfish Rag.
- 🔄 Перепись означает, что модель будет обучена на основе двух предложенных вариантов ответа и их оценке по пяти критериям.
- ✅ Оценка каждого ответа будет производиться по шкале от 1 до 5 или 1 до 3, в зависимости от критерия.
- 🔍 Если предпочтительный ответ не совершенен, будет предпринята попытка переписать его, чтобы исправить возможные недостатки.
- 📝 Причины для переписывания включают проблемы с достоверностью, основанностью, полезностью или включением вежливых фраз.
- 📊 Для определения необходимости переписывания используется диаграмма-расписание, которое помогает определить, требуется ли перепись.
- ✍️ Перепись включает удаление или исправление ошибок, добавление полезной информации и устранение избыточных элементов.
- 📋 Важно сохранять соответствие между изменениями, оправданием их и оценками ответов.
- 🔗 Переписанный ответ становится контекстом для следующего взаимодействия с моделью.
- 💬 Автор видео призывает к обсуждению вопросов и предоставлению отзывов в канале Discourse для улучшения процесса обучения модели.
Q & A
Что означает термин 'перезаписи' в контексте проекта Goldfish rag?
-Перезаписи в проекте Goldfish rag означают процесс изменения и улучшения ответов модели, чтобы сделать их идеальными в соответствии с определенными критериями.
Какие основные компоненты используются для обучения модели в проекте Goldfish rag?
-Для обучения модели используются промпты, два варианта ответов модели, оценка по пяти критериям, оценка Liker от 1 до 5 и обоснование выбора.
Что делает оценка Liker в процессе обучения модели?
-Оценка Liker помогает определить, какой из двух ответов модели является лучшем, предоставляя моделью чуть более высокий балл от 1 до 5.
Почему иногда выбранный ответ требует перезаписи?
-Выбранный ответ может потребовать перезаписи, если он имеет проблемы с правдивостью, основанностью, полезностью или включает ненужные приветственные фразы.
Как определяется, требуется ли перезапись ответа?
-Требуется ли перезапись определяется на основе диаграммы процесса, которая учитывает наличие проблем с правдивостью, основанностью, полезностью, наличием приветственных фраз или других проблем.
Что такое приятности, и почему их необходимо удалить?
-Приятности - это ненужные или незначительные фразы, которые не добавляют ценности к содержанию ответа и могут быть удалены для улучшения его.
Какие изменения в процессе обучения модели были внедрены с помощью перезаписей?
-С помощью перезаписей внедрены изменения, которые включают в себя исправление ошибок, добавление полезной информации, удаление ненужных приветственных фраз и обеспечение полного соответствия запросу.
Что означает полное соответствие запросу?
-Полное соответствие запросу означает, что модельный ответ должен учитывать все ограничения и требования промпта, чтобы полностью удовлетворить его.
Какие действия предпринимаются для исправления ошибок в ответе?
-Для исправления ошибок в ответе может быть произведено удаление ложных или необоснованных заявлений, исправление основанных, добавление полезной информации из текста ссылки и улучшение стиля написания.
Чем отличается оправдание перезаписи от оправдания оценок и Liker?
-Оправдание перезаписи должно точно соответствовать тому, что было сделано в редакторе, в то время как оправдание оценок и Liker должно соответствовать выбранному ответу и его оценке.
Outlines
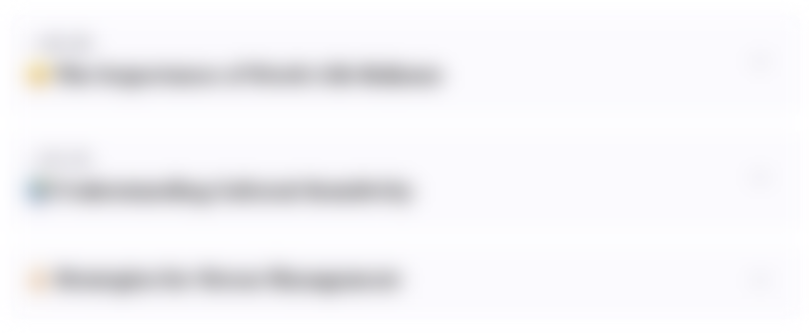
This section is available to paid users only. Please upgrade to access this part.
Upgrade NowMindmap
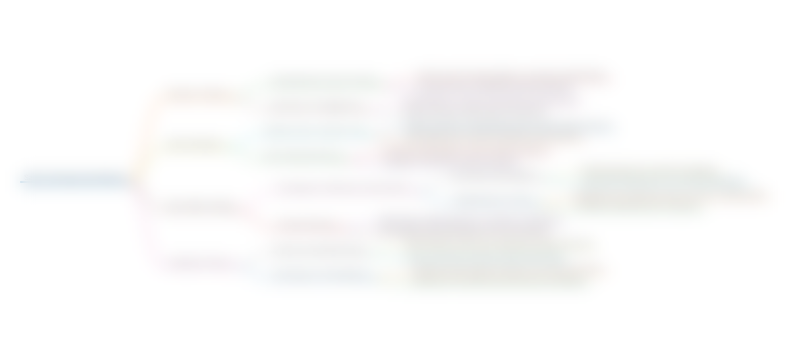
This section is available to paid users only. Please upgrade to access this part.
Upgrade NowKeywords
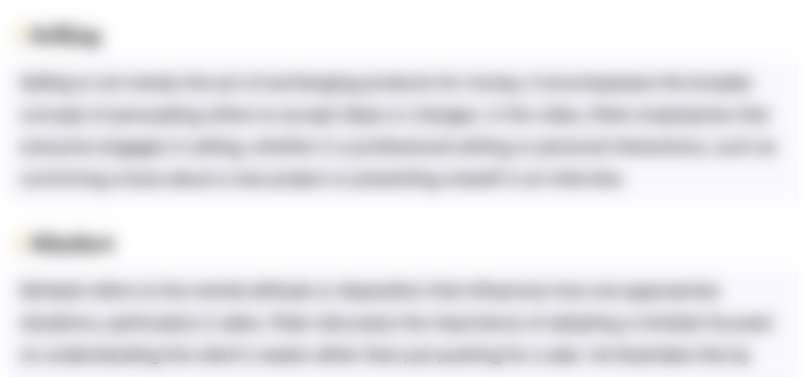
This section is available to paid users only. Please upgrade to access this part.
Upgrade NowHighlights
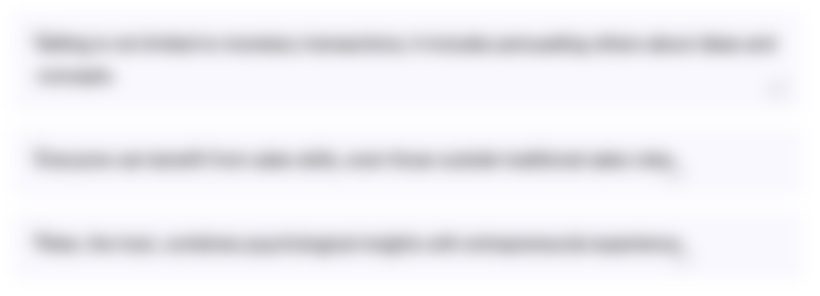
This section is available to paid users only. Please upgrade to access this part.
Upgrade NowTranscripts
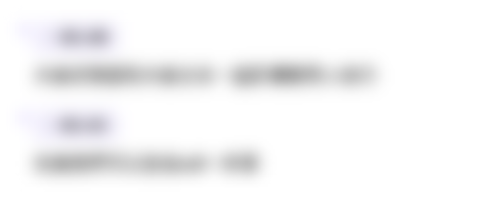
This section is available to paid users only. Please upgrade to access this part.
Upgrade NowBrowse More Related Video
5.0 / 5 (0 votes)