Few-Shot Prompting Explained
Summary
TLDRفي هذا الفيديو، نستعرض مفهوم الـ 'Few-shot prompting' وكيف يمكن استخدامه لتحسين أداء وجودة المخرجات من النماذج اللغوية الكبيرة. تم تغطية مفهوم 'zero-shot prompting' سابقًا، والذي يعتمد على إعطاء النموذج تعليمات بدون أمثلة. ومع ذلك، يتيح 'few-shot prompting' تقديم أمثلة للنموذج لتوضيح المهمة المطلوبة، مما يزيد من دقة وموثوقية النتائج. يُظهر الفيديو كيفية استخدام هذه التقنية لتحسين النماذج، مع تقديم أمثلة توضيحية من ورقة بحثية سابقة. في النهاية، يتم التطرق لإمكانية استخدام هذه الطريقة في تطبيقات مختلفة.
Takeaways
- 🤖 مفهوم 'few-shot prompting' يشير إلى تحسين أداء النماذج اللغوية الكبيرة عن طريق إعطائها أمثلة على كيفية تنفيذ المهام.
- 📜 تم التطرق إلى 'zero-shot prompting' حيث يتم إعطاء النموذج تعليمات مباشرة دون أمثلة مسبقة.
- 📝 عند استخدام 'zero-shot prompting' يمكن للنموذج تصنيف النصوص بناءً على تعليمات بسيطة مثل تصنيف النصوص إلى إيجابية أو سلبية.
- ⚙️ 'few-shot prompting' يكون مفيداً عند مواجهة مهام معقدة أو غير مألوفة للنموذج، حيث يمكن للأمثلة أن تساعد النموذج على فهم المهمة بشكل أفضل.
- 🎓 يتم استخدام أمثلة توضيحية في 'few-shot prompting' لتحديد المدخلات والمخرجات المتوقعة من النموذج.
- 🧠 يتم استخدام أمثلة من ورقة بحثية لتوضيح فعالية 'few-shot prompting' في تحسين دقة النموذج.
- 💡 'few-shot prompting' يمكن أن يكون مفيدًا في ضبط نغمة رسائل البريد الإلكتروني أو توليد عناوين لمقالات معينة.
- ⚠️ يتم التأكيد على أهمية تصميم التعليمات بعناية واختيار الأمثلة المناسبة لتحقيق أفضل نتائج من النماذج اللغوية.
- 🔄 يمكن تعديل هيكل التعليمات والأمثلة لتناسب المهمة المحددة وتوجيه النموذج بشكل أكثر دقة.
- 📚 سيتم تناول تقنية 'Chain of Thought prompting' في الفيديو التالي كمثال آخر على تحسين استجابة النماذج اللغوية.
Q & A
ما هو مفهوم 'F Shot Prompting' الذي يتم التحدث عنه في الفيديو؟
-مفهوم 'F Shot Prompting' يشير إلى استخدام أمثلة أو تقديم نماذج للمهام التي تريد من النموذج اللغوي تنفيذها، مما يساعد النموذج على فهم المهمة بشكل أفضل وتقديم إجابات أكثر دقة وجودة.
ما الفرق بين 'Zero Shot Prompting' و'F Shot Prompting'؟
-'Zero Shot Prompting' يعني إعطاء النموذج تعليمات دون تقديم أي أمثلة، بينما في 'F Shot Prompting' يتم تزويد النموذج بأمثلة توضيحية للمهمة مما يساعده على تحسين الأداء وفهم المهمة بشكل أعمق.
لماذا يمكن أن يكون 'F Shot Prompting' مفيدًا في بعض الحالات؟
-'F Shot Prompting' يكون مفيدًا في الحالات التي لا يمتلك فيها النموذج بيانات كافية أو فهمًا كافيًا للمهمة المعقدة، حيث تساعد الأمثلة المقدمة النموذج على تحسين دقة الإجابات.
كيف يساعد تقديم الأمثلة في 'F Shot Prompting' النموذج اللغوي؟
-تقديم الأمثلة في 'F Shot Prompting' يساعد النموذج على فهم التوقعات المطلوبة من المهمة وتحديد السياق المناسب للإجابة بشكل صحيح، مما يؤدي إلى تحسين جودة المخرجات.
ما هي خطوات إعداد نموذج لغوي باستخدام 'F Shot Prompting'؟
-الخطوات تشمل تقديم رسالة نظام توضح المهمة، متبوعة بتقديم أمثلة تحتوي على المدخلات والمخرجات المتوقعة، ثم يتم تشغيل النموذج لتقديم الإجابات بناءً على الأمثلة المقدمة.
هل يجب دائمًا تقديم أمثلة المدخلات والمخرجات في 'F Shot Prompting'؟
-ليس دائمًا، بعض المهام يمكن أن تكتفي بتقديم المخرجات فقط دون الحاجة إلى تقديم المدخلات، حسب طبيعة المهمة والهدف المطلوب.
ما هو التحدي المحتمل عند استخدام 'F Shot Prompting' مع النماذج اللغوية؟
-أحد التحديات هو أن النموذج قد يكون له انحياز نحو نوع معين من المخرجات (مثل تفضيل الإجابات الإيجابية) إذا لم يتم تزويده بأمثلة كافية ومتوازنة.
كيف يمكن تحسين نموذج لغوي إذا لم يقدم أداءً جيدًا في 'F Shot Prompting'؟
-يمكن تحسين أداء النموذج بتزويده بمزيد من الأمثلة المناسبة التي تغطي الحالات التي يواجه فيها صعوبة، مما يساعده على التعلم وتقديم إجابات أكثر دقة.
ما هي الفائدة من استخدام نموذج لغوي لتوليد كلمات جديدة وتكوين جمل باستخدام هذه الكلمات؟
-الفائدة تكمن في قدرة النموذج على توليد جمل منطقية باستخدام كلمات لم تكن موجودة مسبقًا، مما يظهر مرونته وفهمه للسياق اللغوي بطريقة مبتكرة.
ما هو الفيديو القادم الذي سيتم تقديمه بعد هذا الفيديو وفقًا للمحتوى؟
-الفيديو القادم سيغطي موضوع 'Chain of Thought Prompting'، وهو طريقة قوية أخرى لتحفيز النماذج اللغوية.
Outlines
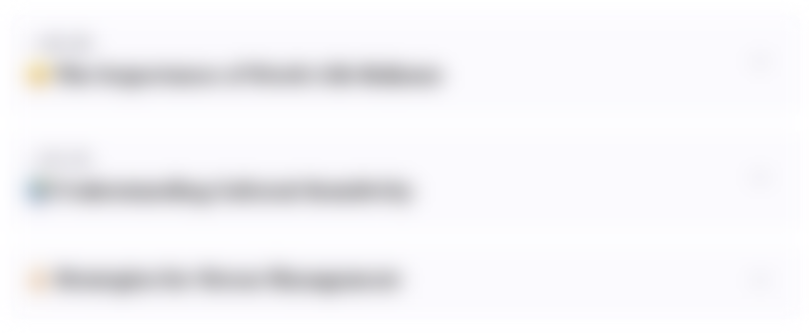
هذا القسم متوفر فقط للمشتركين. يرجى الترقية للوصول إلى هذه الميزة.
قم بالترقية الآنMindmap
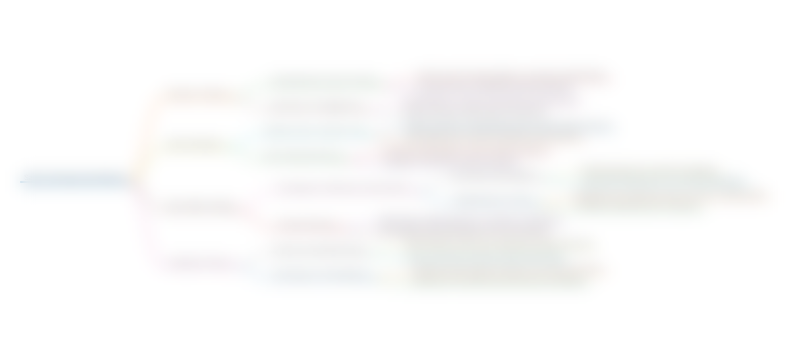
هذا القسم متوفر فقط للمشتركين. يرجى الترقية للوصول إلى هذه الميزة.
قم بالترقية الآنKeywords
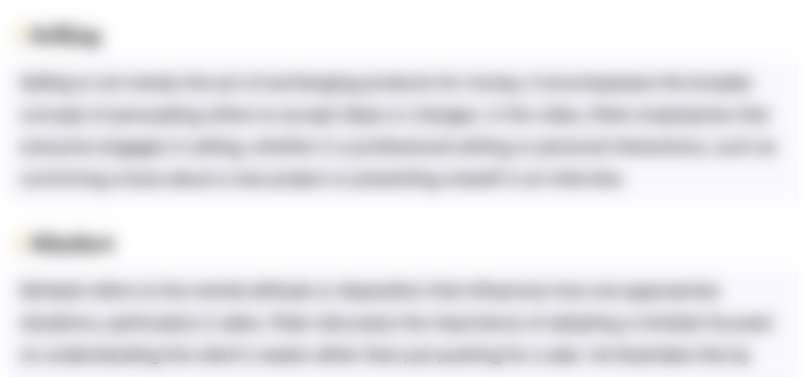
هذا القسم متوفر فقط للمشتركين. يرجى الترقية للوصول إلى هذه الميزة.
قم بالترقية الآنHighlights
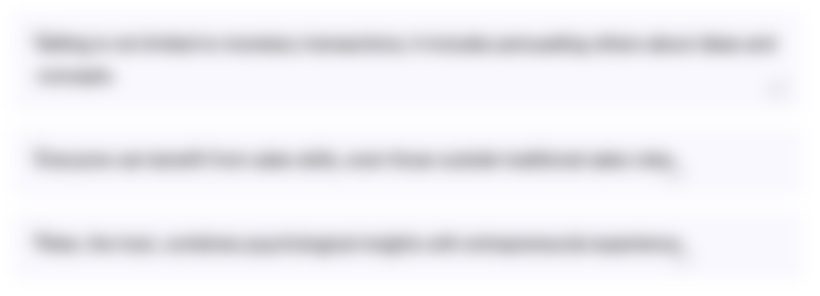
هذا القسم متوفر فقط للمشتركين. يرجى الترقية للوصول إلى هذه الميزة.
قم بالترقية الآنTranscripts
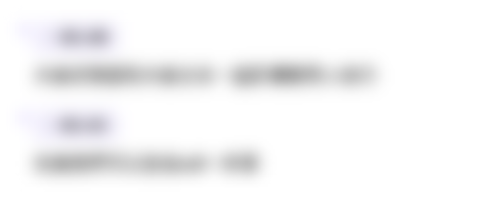
هذا القسم متوفر فقط للمشتركين. يرجى الترقية للوصول إلى هذه الميزة.
قم بالترقية الآنتصفح المزيد من مقاطع الفيديو ذات الصلة
5.0 / 5 (0 votes)