What Are The Types Of Sampling Techniques In Statistics - Random, Stratified, Cluster, Systematic
Summary
TLDRThe video explains the importance of sampling in research to gather data about populations. It highlights four main sampling methods: random, stratified, cluster, and systematic sampling. Random sampling gives all members an equal chance of being selected, while stratified sampling ensures each subgroup is represented. Cluster sampling targets specific sections of a population, and systematic sampling selects members at regular intervals. It also mentions convenience sampling, which is prone to bias. The video concludes by noting that even with the best techniques, sampling errors can occur.
Takeaways
- 🔍 A sample is a part of a population used by researchers to collect data about variables.
- 🎯 There are four main sampling techniques: random sampling, stratified sampling, cluster sampling, and systematic sampling.
- 🎲 Random sampling gives every member of the population an equal chance of being selected.
- 📊 Stratified sampling involves dividing the population into subgroups and taking a random sample from each subgroup.
- 🏙️ Cluster sampling involves dividing the population into clusters, then selecting one or more clusters and using all members of the selected clusters as the sample.
- ⚠️ Cluster sampling can be cost-effective but may not always represent the population well.
- 🔢 Systematic sampling assigns a number to each population member and selects members at regular intervals starting from a random number.
- 📝 A sampling error can occur, which is the difference between the sample results and the population results.
- 🚶 Convenience sampling involves selecting members of the population that are easy to reach, but it often leads to biased results.
- 📈 Even with the best sampling methods, researchers should be aware of potential errors or biases in their samples.
Q & A
What is the purpose of using a sample in research?
-A sample is used to collect data and information about a variable or variables from a larger population. It helps researchers analyze a subset of the population instead of the entire group, which is often impractical.
What are the four main sampling techniques mentioned in the script?
-The four main sampling techniques are random sampling, stratified sampling, cluster sampling, and systematic sampling.
How does random sampling ensure unbiased data collection?
-Random sampling ensures unbiased data collection by giving every member of the population an equal chance of being selected for the sample.
What is a stratified sample, and when is it used?
-A stratified sample involves dividing the population into subgroups based on shared characteristics, and then selecting a random sample from each subgroup. It is used when researchers want to ensure representation from different segments of the population.
What is the main difference between stratified sampling and cluster sampling?
-In stratified sampling, the subgroups have similar characteristics, while in cluster sampling, the clusters are intended to vary in characteristics.
How does cluster sampling work, and when is it useful?
-Cluster sampling involves dividing the population into sections or clusters, randomly selecting one or more clusters, and using all members of the selected clusters as the sample. It is useful when the population is large or geographically dispersed, making it cost-effective and efficient.
What is an example of systematic sampling?
-An example of systematic sampling is selecting a random starting number, such as 234, and then choosing every 20th member from that starting point to create a sample. This method is used when the population can be easily numbered.
What is a sampling error, and why can it occur?
-A sampling error is the difference between the results of a sample and the actual population. It can occur even when using the best sampling methods, due to the inherent variability between samples and populations.
What is convenience sampling, and why can it lead to biased results?
-Convenience sampling involves selecting a sample from members of the population that are easy to access or convenient. It often leads to biased results because the sample may not be representative of the entire population.
What are some methods researchers can use to create a random sample?
-Researchers can create a random sample by numbering each member of the population, drawing numbered cards, using a calculator or computer to generate random numbers, or using a random number table.
Outlines
📊 Understanding Sampling Methods
This paragraph introduces the concept of sampling in research, where a sample is a subset of a population used to gather data about a variable or variables. To ensure unbiased results, researchers employ four main sampling methods: random sampling, stratified sampling, cluster sampling, and systematic sampling. Each method is designed to accurately reflect the population while overcoming practical challenges of data collection.
🎲 Random Sampling Explained
A random sample gives each member of the population an equal chance of selection. Researchers can achieve this by numbering individuals and using methods such as drawing numbers from a hat, using a calculator or random number generator, or using a random number table. This method ensures fairness and eliminates selection bias.
🔀 Stratified Sampling Overview
Stratified sampling involves dividing the population into subgroups, or strata, based on shared characteristics. Researchers then take a random sample from each subgroup to ensure that all segments of the population are represented. For instance, if a study seeks to explore annual savings habits across age groups, researchers would collect random samples from people in their 20s, 30s, 40s, and 50s, ensuring a balanced representation across ages.
📦 Cluster Sampling Simplified
Cluster sampling divides a large population into clusters, often based on geographical regions or sections. Researchers randomly select one or more clusters and include all members of those clusters in the sample. This technique is particularly useful for studying large or geographically dispersed populations. However, it may lead to biases if the selected clusters don't fully represent the population.
🔑 Key Differences Between Cluster and Stratified Sampling
While both cluster and stratified sampling involve dividing the population, the subgroups in stratified sampling have similar characteristics, whereas clusters in cluster sampling are intended to vary in characteristics. This distinction helps ensure that stratified samples represent different characteristics equally, while cluster samples aim for geographic or sectional convenience.
⏱️ Systematic Sampling Explained
Systematic sampling involves selecting individuals at regular intervals after a random starting point is chosen. For instance, in a study of Netflix viewing habits in an apartment complex, the researcher could randomly select a starting unit number, then choose every 20th unit thereafter until the desired sample size is reached. This method is efficient if the population can be easily numbered.
⚠️ Sampling Errors and Convenience Sampling
Sampling errors, which represent the difference between the results of a sample and the entire population, can still occur even with the best methods. Convenience sampling, which involves selecting participants that are easy to access, often leads to biased results. Despite its ease, this method is less reliable due to the potential for significant bias in representation.
Mindmap
Keywords
💡Sample
💡Population
💡Random Sampling
💡Stratified Sampling
💡Cluster Sampling
💡Systematic Sampling
💡Sampling Error
💡Convenience Sampling
💡Unbiased
💡Subgroup
Highlights
A sample is part of a population, and researchers use samples to collect data and information about variables from the larger population.
To obtain unbiased samples, there are four main sampling techniques: random sampling, stratified sampling, cluster sampling, and systematic sampling.
A random sample gives every member of the population an equal chance of being selected.
One way to conduct random sampling is to number each member of the population and select random numbers, either manually or using a computer.
A stratified sample divides the population into subgroups based on similar characteristics, and a random sample is taken from each subgroup.
Cluster sampling divides the population into clusters, and a random selection of one or more clusters is used to sample all members from those clusters.
Cluster sampling is useful for large populations or wide geographic areas but may sometimes not represent the entire population.
The key difference between stratified and cluster sampling is that stratified sampling involves subgroups with similar characteristics, while cluster sampling involves subgroups with varied characteristics.
Systematic sampling assigns a counting number to the population and selects members for the sample at regular intervals.
An example of systematic sampling is using a random starting number and a consistent interval to select participants.
Systematic sampling is simple and works well if the population can be easily numbered.
Sampling error is the difference between sample results and actual population results, which can still occur even with the best methods.
Convenience sampling involves selecting members of the population that are easy to access but often leads to biased results.
Each sampling method has its strengths and weaknesses, and the choice depends on the population and research context.
Even the best sampling techniques are not foolproof, and researchers must be aware of potential biases and errors.
Transcripts
A sample is part of a population, and researchers use samples to collect data and information about
a variable or variables from the larger population. To obtain samples that are unbiased there are
mainly four different sampling techniques or methods, random sampling, stratified sampling,
cluster sampling, and systematic sampling. A random sample is a sample where every
member of the population has an equal chance of being selected. There are a few different ways
to do this, the researcher could number each member of the population, to keep it simple
say a population of 90 members, he or she could then place numbered cards 1 through 90 in
a hat or bowl or mixer, and select as many cards as needed to complete the sample. Or they can
use a calculator or computer to generate random numbers, or they could use a random number table
like this one. A stratified sample is where a researcher will divide the population into
subgroups to have members from each segment of the population, and a random sample is derived
from each subgroup. For example let's say you wanted to know how much money people saved on
a yearly basis, you could have subgroups of people in their 20s, in their 30s, in their 40s, and in
their 50s. You would then take a random sample for each of these groups. A cluster sample is
obtained by dividing the population into sections or clusters, then randomly selecting one or more
of the clusters and using all of its members, as members of the sample. This is often used when the
population is large or there's a large geographic area. For instance let's say you wanted to survey
small business owners in a very populated city, it would be costly and time-consuming to survey every
single small business owner. So you could create a cluster sample, using zip codes and maybe survey
two or three of the thirteen different possible zip codes. Cluster samples can be efficient and
cost effective, however there are times when the cluster does not represent the population. A little
note, the main difference between cluster sampling and stratified sampling is that subgroups in the
stratified sample have similar characteristics and the subgroups or clusters in the cluster
sample are intended to vary in characteristics. A systematic sample is where a researcher will
assign a counting number to have of the population, then select a random number, then select members
for the sample at regular intervals, from the starting random number that was selected. For
example let's say you wanted to know how much time people living in a singles only apartment complex
spent watching Netflix on a weekly basis. You would want to get, say, a sample of 50 members. If there
were a thousand units in the complex you could number the units one to a thousand and generate
a random starting number, say 234, since you want a sample of 50, you could divide 1,000, the number of
the population, by 50, to get 20, which would be your interval number. So, 234 would be selected
for the sample, then 254, which is 234 plus 20, then 274, which is 254 for plus 20, and so on until you
had your 50 members selected for your sample. This sampling method is easy to use if the population
can be easily numbered. Another note, even when using the best sampling methods a sampling error,
which is the difference between the results of a sample and a population can occur, and there
is one other sampling method called a convenience sample, where a researcher develops a sample from
members of the population that are easy to get or convenient. Many times these samples lead to biased
results. Alright my friends, I have more videos right there for you, till next time, I am outta here.
تصفح المزيد من مقاطع الفيديو ذات الصلة
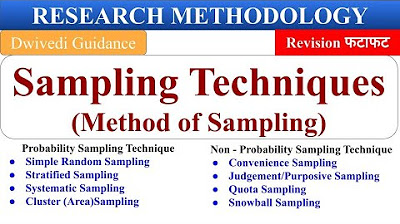
sampling techniques, types of sampling, probability & non probability sampling, Research methodology
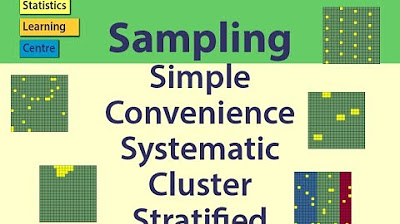
Sampling: Simple Random, Convenience, systematic, cluster, stratified - Statistics Help
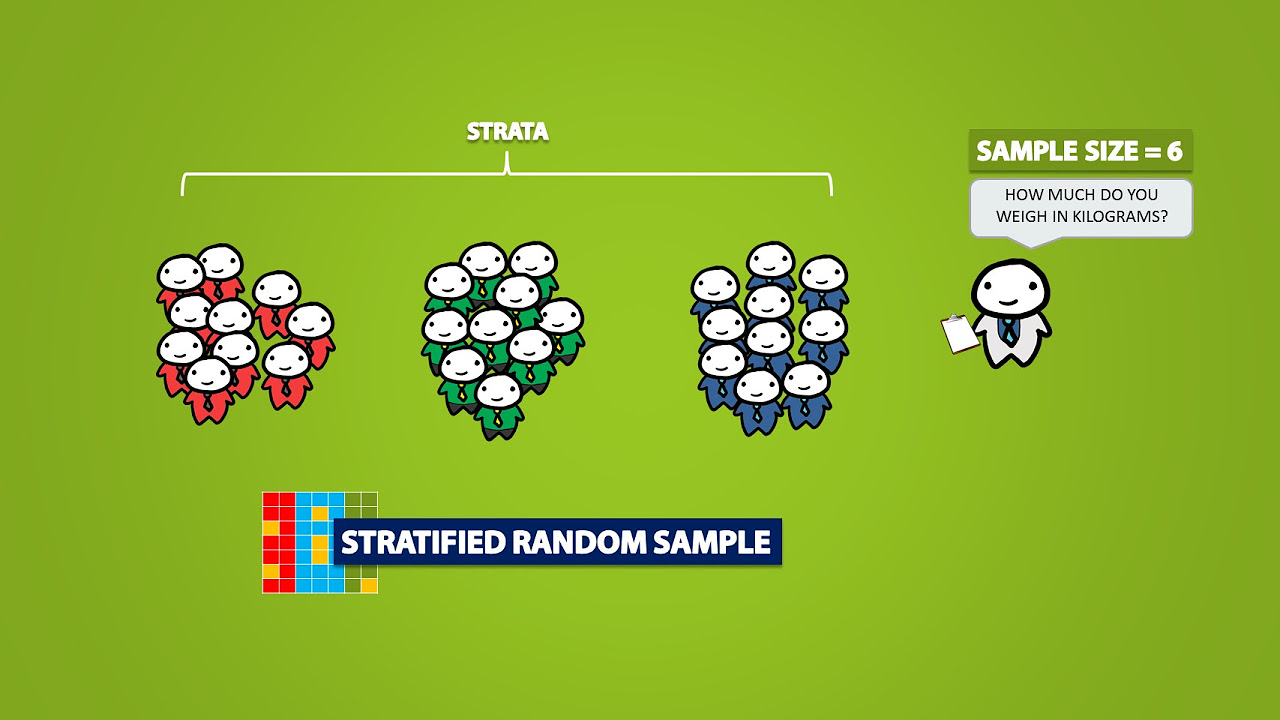
Types of Sampling Methods (4.1)
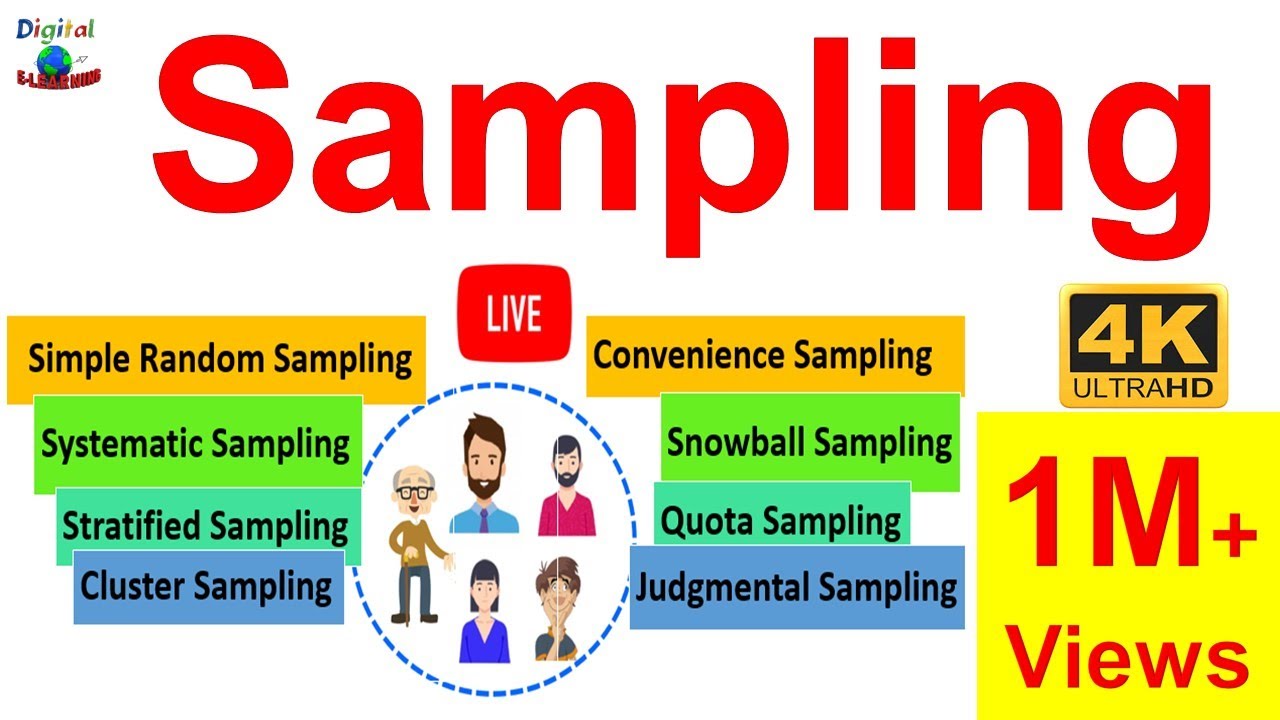
Sampling: Sampling & its Types | Simple Random, Convenience, Systematic, Cluster, Stratified
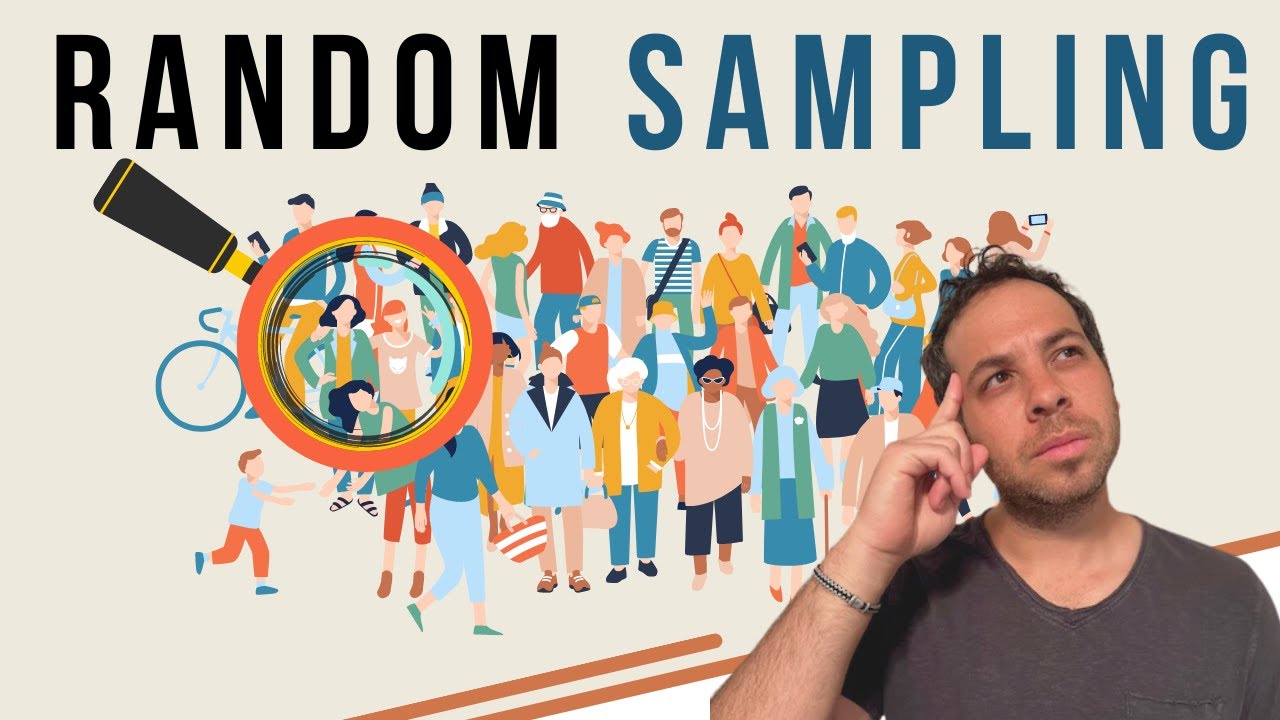
Sampling: Population vs. Sample, Random Sampling, Stratified Sampling
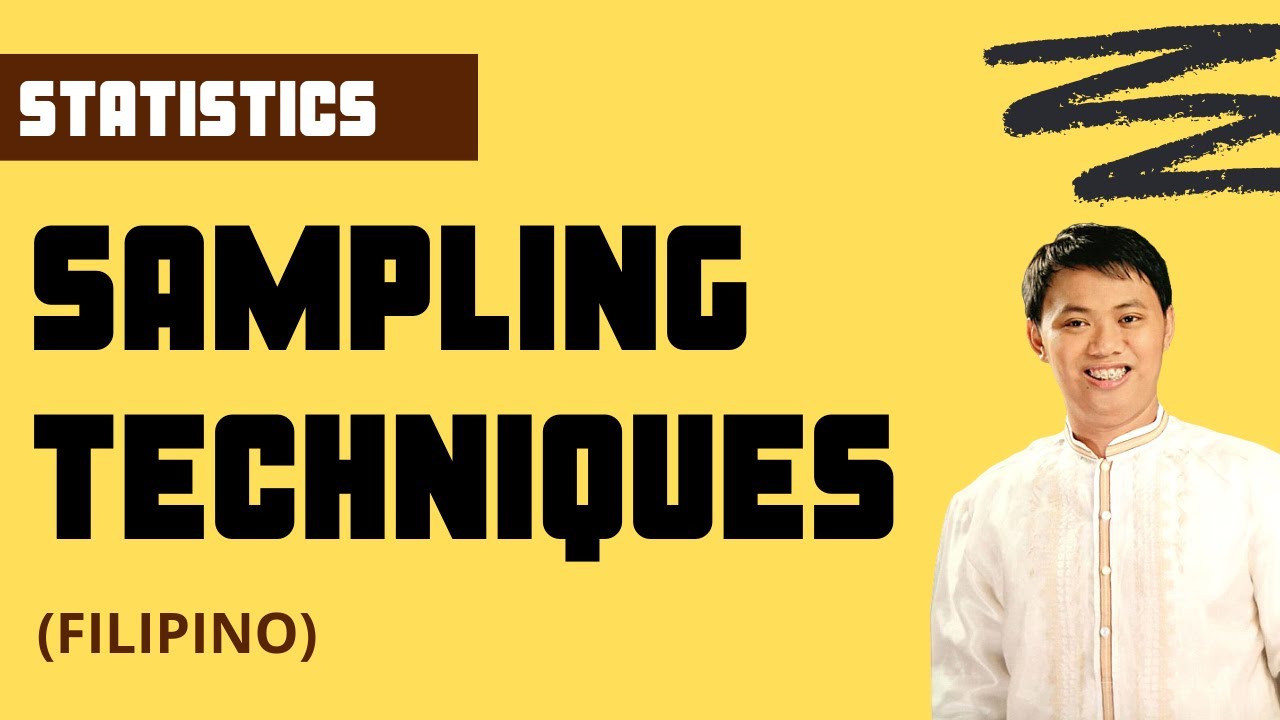
Probability & Non-Probability Sampling Techniques - Statistics
5.0 / 5 (0 votes)