Illustrated Guide to Transformers Neural Network: A step by step explanation
Summary
TLDR该视频详细介绍了transformer模型的工作原理。它利用注意力机制,允许模型关注输入序列中每个词与其他词之间的关系。视频通过一个聊天机器人的示例,步步解读了transformer模型的encoder和decoder部分。它展示了注意力机制如何帮助模型生成更好的预测。总的来说,该视频帮助观众深入理解这种基于注意力的模型的威力。
Takeaways
- 😀 转换器通过注意力机制实现了在NLP任务上的突破
- 😊 转换器可以参考很长的上下文信息,不像RNN有短期记忆问题
- 🤔 多头注意力允许模型学习输入中不同单词之间的关联
- 😯 位置编码给输入注入了顺序信息,因为转换器没有循环结构
- 😀 将查询,键和值的注意力机制应用于自注意力中
- 🤔 编码器通过自注意力和前馈网络编码输入序列
- 😊 解码器通过遮挡未来记号只关注过去
- 🧐 前馈网络和残差连接帮助网络更好地训练
- 😀 堆叠编码器和解码器层可提升模型预测能力
- 🥳 转换器架构让NLP取得了前所未有的成果
Q & A
变压器的核心机制是什么?
-变压器最核心的机制是“attention机制”,这使得模型可以学习关注输入序列的,不同部分,从而做出更好的预测。
多头注意力机制是什么?
-多头注意力机制产生多个attention层或“头”,每个头会独立地学习不同的attention表示,然后把它们合并以产生更丰富的表示。
为什么要使用位置编码?
-因为变压器编码器没有RNN所具有的循环结构,所以需要通过位置编码向嵌入向量注入位置信息,让模型能够区分不同位置的词。
编码器的作用是什么?
-编码器的作用是将输入序列映射为一个连续的、带有注意力信息的表示,这可以帮助解码器在解码过程中正确地关注输入的相关部分。
解码器的关键特点是什么?
-解码器是自回归的,它接收先前输出作为当前输入,同时也接收来自编码器的输出。解码器通过Masked Attention 防止模型看到未来词。
变压器为什么常超越RNN?
-因为注意力机制的关系,变压器在理论上具有无限的工作记忆,可以更好地处理长序列。而RNN则受限于短期记忆问题。
变压器主要应用于哪些领域?
-变压器被广泛应用于机器翻译、开放域问答、语音识别等多种自然语言处理任务中,取得了显著成效。
BPE是什么?
-BPE(Byte Pair Encoding)是一种词元化算法,通过迭代地将频繁共现的字符对合并,可以有效地产生词元,提高模型对词汇的覆盖率。
变压器为何需要大量训练数据?
-变压器的参数较多,需要大量标注数据进行有效训练,以防止模型过拟合。数据量不足时,变压器的性能会明显下降。
变压器的主要缺点是什么?
-变压器计算复杂,需要大量计算资源,同时也需要大量标注数据进行有效训练。这限制了其应用范围。
Outlines
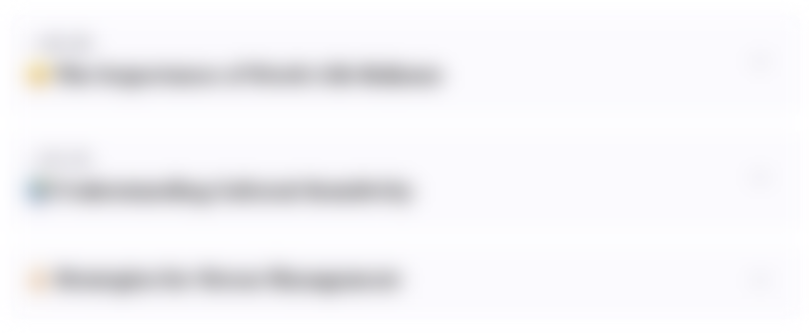
此内容仅限付费用户访问。 请升级后访问。
立即升级Mindmap
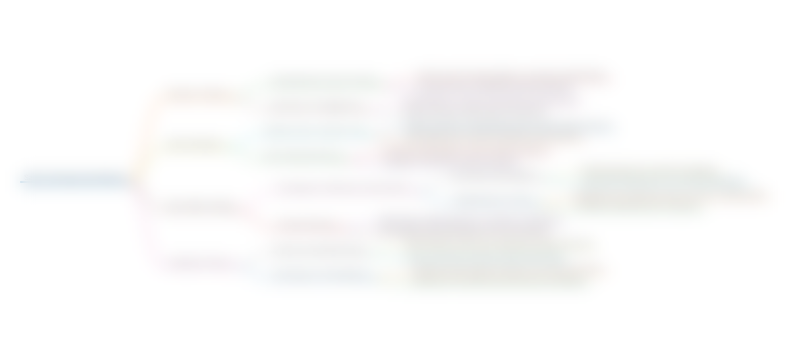
此内容仅限付费用户访问。 请升级后访问。
立即升级Keywords
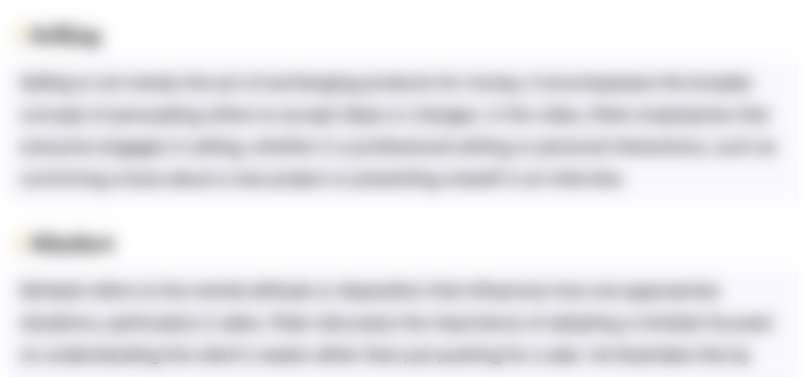
此内容仅限付费用户访问。 请升级后访问。
立即升级Highlights
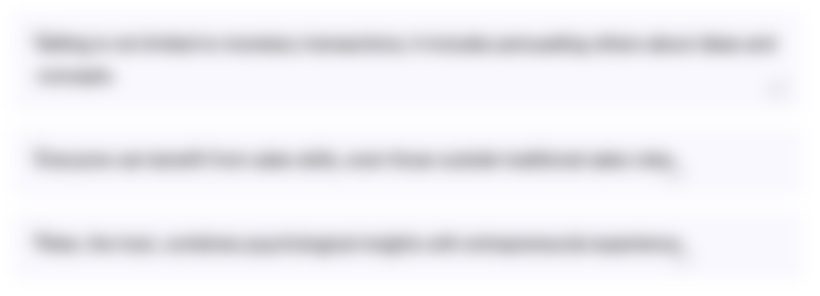
此内容仅限付费用户访问。 请升级后访问。
立即升级Transcripts
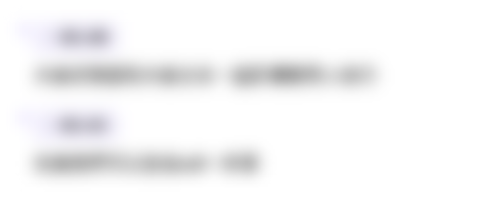
此内容仅限付费用户访问。 请升级后访问。
立即升级浏览更多相关视频
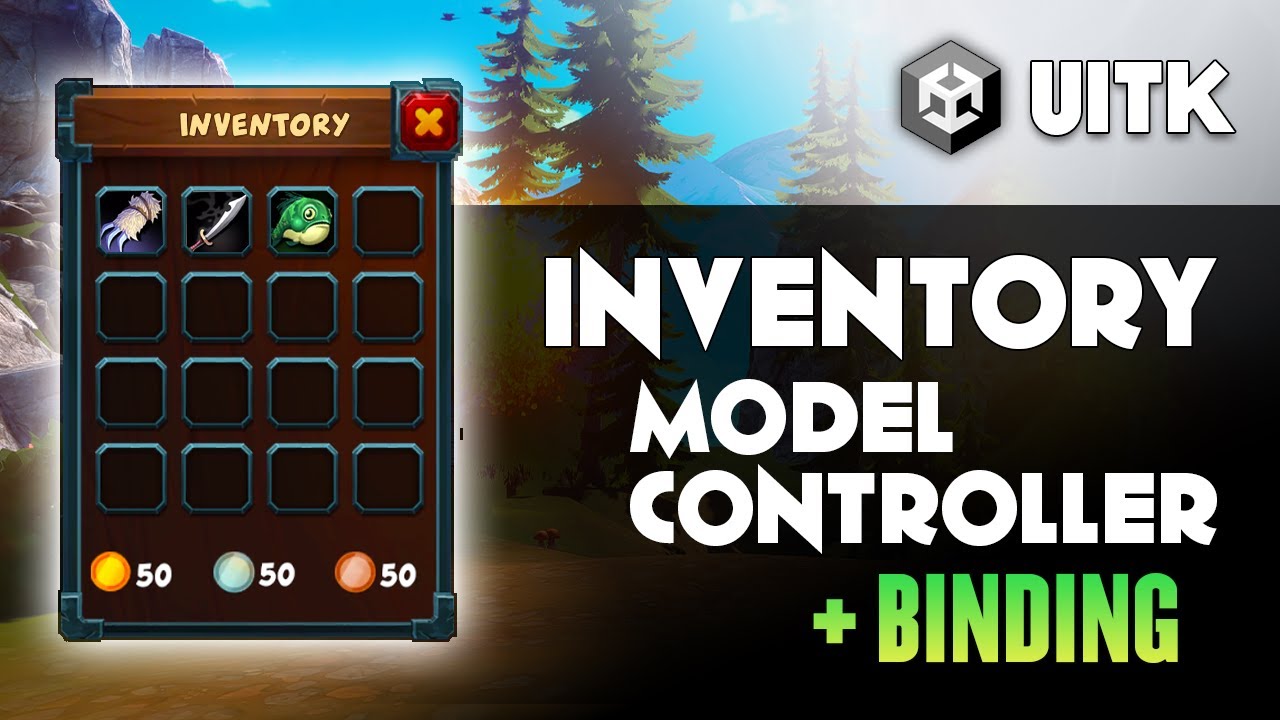
UI Toolkit Runtime Data Binding and Logic
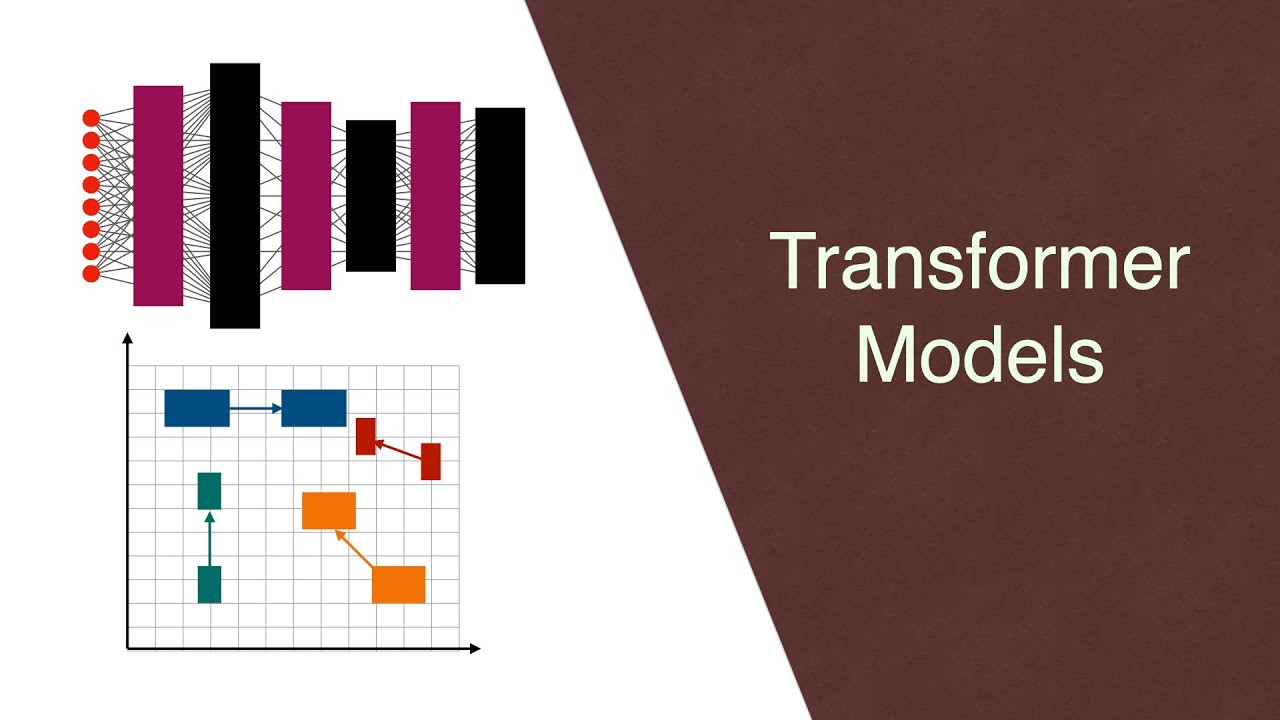
What are Transformer Models and how do they work?
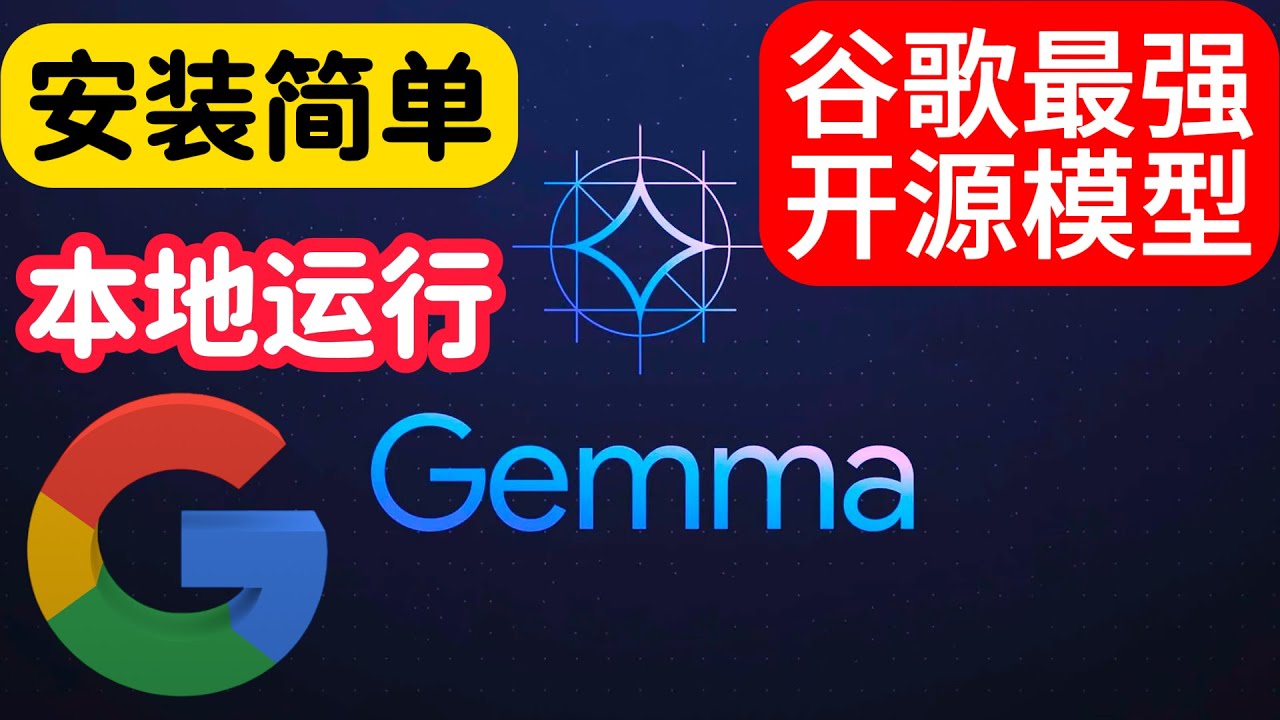
【2024 最新】谷歌最新最强开源Gemma 大模型,人工智能对话,小白也能轻松部署网页端对话 (附详细步骤),媲美GPT,本地笔记本或台式电脑运行,GPU 加速 | 零比特
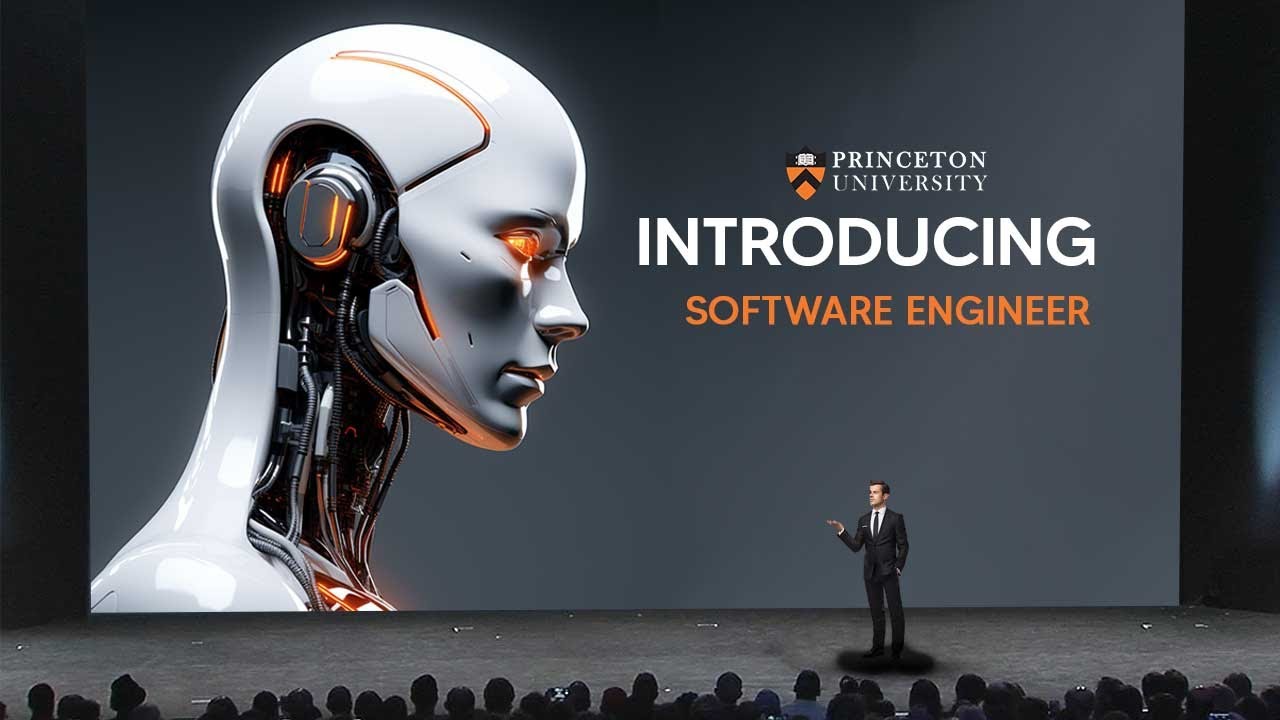
New OPEN SOURCE Software ENGINEER Agent Outperforms ALL! (Open Source DEVIN!)

Lecture 1.3 — Some simple models of neurons — [ Deep Learning | Geoffrey Hinton | UofT ]
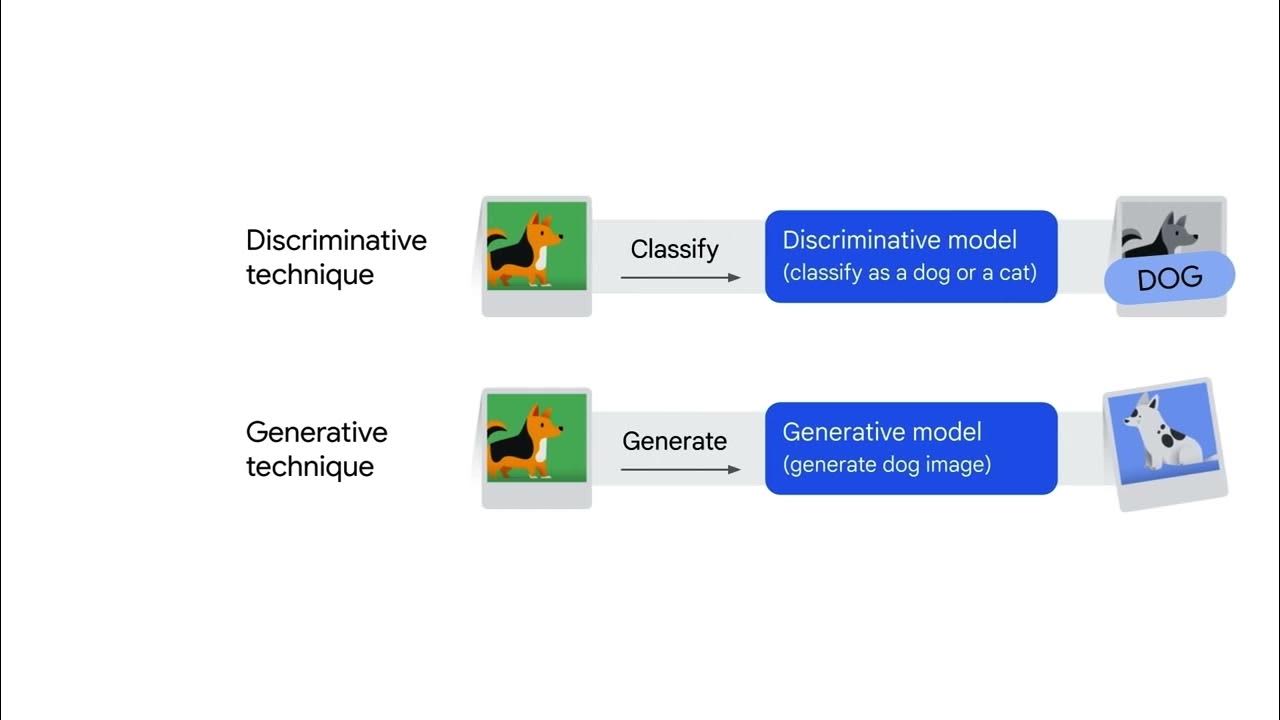
Introduction to Generative AI
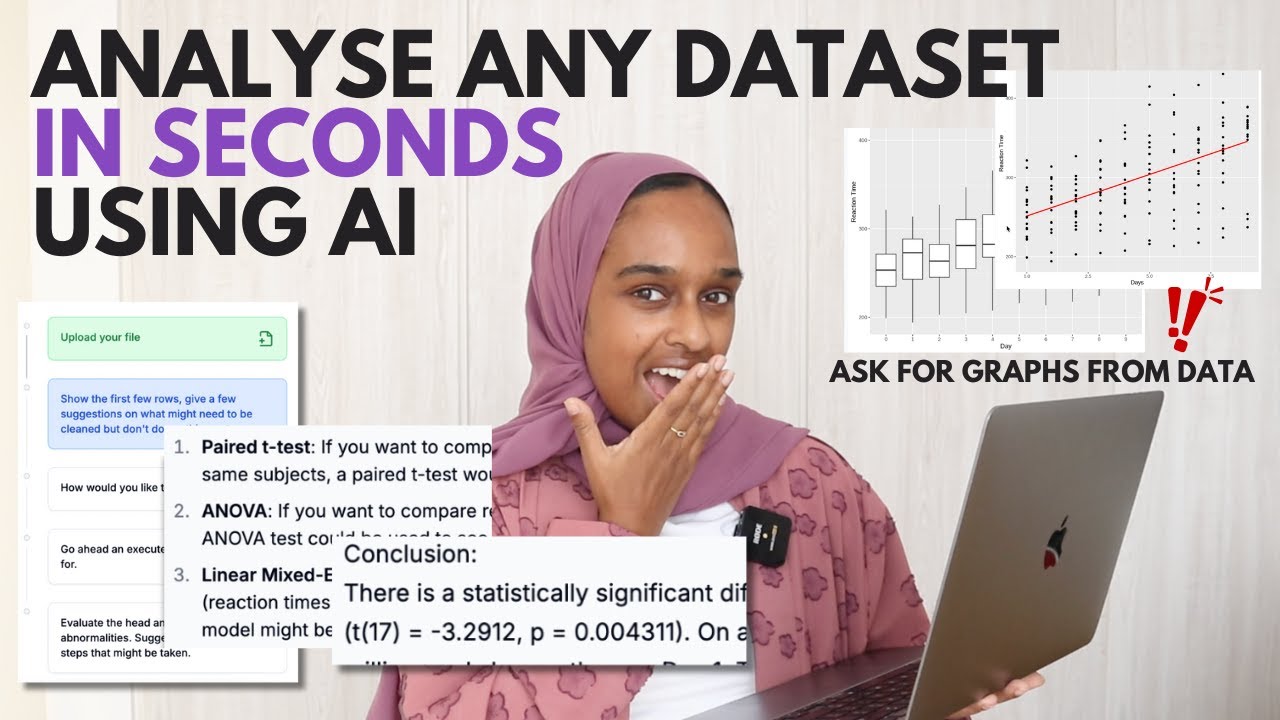
How To Analyse YOUR DATA With Julius AI (No Need For CODE) | Analyse Data Quickly
5.0 / 5 (0 votes)