MINI LESSON 7: P-Values and P-Value Hacking: a simplified lecture.
Summary
TLDRIn this lecture, the speaker expresses skepticism about the p-value, a statistical concept often used in hypothesis testing. They argue that p-values lack a solid probabilistic basis and are frequently misunderstood. The speaker emphasizes that metrics are often stochastic and can be influenced by survivorship bias, leading to skewed results. They explain that p-values are stochastic and may not accurately represent the probability of a true effect. The lecture warns against the misuse of p-values and suggests considering alternative statistical methods, particularly in fields like psychology where sample sizes are often small.
Takeaways
- π The speaker expresses skepticism about the p-value, suggesting it lacks a solid probabilistic basis despite its widespread use.
- π The lecture emphasizes that metrics are often stochastic variables, meaning they can vary with each sample, especially when the sample size is small.
- π It's highlighted that stochastic variables can lead to survivorship bias, where only the most successful outcomes are observed, skewing the understanding of the variable's distribution.
- π The p-value is explained as the probability of observing a statistic as extreme as, or more extreme than, the one observed, under the null hypothesis.
- π€ The speaker points out that the p-value is itself stochastic and can be skewed, with most values falling below the true mean.
- π§ The p-value's problem is exacerbated by the fact that it does not account for the sample size 'n', which is crucial for understanding its significance.
- π¬ The speaker warns against 'gaming the metric' by running multiple experiments and taking the maximum p-value, which can lead to misleading results.
- π The distribution of the maximum p-value is lower than the true p-value, suggesting that repeated experiments can artificially lower the p-value and misrepresent significance.
- π Reference is made to the speaker's book 'Statistical Consequences of Fat Tails', where further discussion on this topic can be found.
- π« The speaker advises caution when using p-values and suggests considering alternative methods for statistical analysis.
- π€ The preference for p-values among psychologists is critiqued, implying that larger sample sizes in other fields might offer more robust results.
Q & A
What is the speaker's opinion on p-values?
-The speaker is not in favor of p-values, considering them to be a concept without a strong probabilistic basis and not very solid, despite their widespread use.
What are the two central points the speaker wants to recap from the session on correlation and metrification?
-The first point is that metrics are often stochastic variables that converge to something by the law of large numbers but can vary in sample, especially with small sample sizes. The second point is the presence of survivorship bias in stochastic variables, where one may only see the upper bound of outcomes.
What does the speaker mean by 'hacking of variables'?
-The speaker refers to the manipulation of stochastic variables to achieve the upper bound of results, which can be misleading as the distribution of the maximum is different from the distribution of the variable itself.
What is the p-value and how is it calculated in the context of the speaker's explanation?
-The p-value is the probability of observing a statistic as extreme as, or more extreme than, the observed value, assuming the null hypothesis is true. It is calculated as the probability that the z-score (mean minus a hypothesized value divided by the standard deviation) is higher than a certain threshold.
Why does the speaker believe p-values are problematic?
-The speaker argues that p-values are problematic because they are stochastic and do not inherently account for the sample size (n), which can lead to misunderstandings about the significance of the results.
What is the issue with p-values being stochastic according to the speaker?
-The issue is that p-values themselves can vary and are skewed, with most values falling below their true mean, which can lead to incorrect conclusions about the significance of the results.
What is the 'survival function' mentioned by the speaker?
-The survival function is the probability that a random variable is greater than a certain value. In the context of p-values, it refers to the probability of exceeding a certain threshold under the assumption of a null hypothesis.
Why does the speaker suggest that the p-value should be considerably smaller than 1?
-The speaker suggests this because if the true p-value is significantly higher (e.g., 0.11), then achieving a p-value of 0.01 through multiple experiments is misleading and not representative of the actual probability.
What does the speaker imply about the use of p-values in psychology?
-The speaker implies that psychologists may prefer p-values because they allow for smaller sample sizes, which might be easier to manage in a college campus setting but can lead to flawed quantitative analysis.
What advice does the speaker give regarding the use of p-values?
-The speaker advises to be cautious with p-values and to consider alternative methods of analysis to avoid the pitfalls associated with their stochastic nature and potential for misinterpretation.
Outlines
π Critique of P-Value Reliability
The speaker expresses dissatisfaction with the concept of p-values, arguing that they lack a solid probabilistic foundation despite widespread use. They emphasize that metrics are often stochastic variables, meaning they converge to a value by the law of large numbers but can vary with different samples, especially when the sample size is small. The speaker also discusses the issue of survivorship bias, where only the most successful outcomes are observed, leading to a skewed perception of events. They highlight that p-values are stochastic and that the distribution of the maximum values is different from the variable itself, which is often misunderstood. The p-value is explained as the probability of observing a statistic as extreme as, or more extreme than, the one observed, given that the null hypothesis is true. The speaker points out that the scaling by the square root of n in p-value calculations does not eliminate the problem of stochasticity.
π‘ The Problem with P-Values and Experimentation
This paragraph delves into the problems associated with p-values, noting that they are inherently stochastic and often skewed, with most values falling below their true mean. The speaker uses an example to illustrate that if the true p-value is 0.11, there's a significant chance of obtaining a p-value less than 0.05 through multiple experiments due to the distribution of maximum values. They argue that this discrepancy makes p-values a poor metric for determining statistical significance. The speaker also touches on the tendency to 'game' metrics by running multiple experiments to achieve a desirable p-value, which can mislead about the true significance of the results. They conclude by cautioning against the reliance on p-values and suggesting that alternative methods should be considered, particularly pointing out that psychologists seem to favor p-values, possibly due to smaller sample sizes in their studies.
Mindmap
Keywords
π‘p-value
π‘stochastic variables
π‘law of large numbers
π‘survivorship bias
π‘correlation
π‘statistical significance
π‘z-score
π‘hacking of variables
π‘maximum distribution
π‘fat tails
π‘experimentation
Highlights
The speaker expresses dissatisfaction with the concept of p-value, considering it not solid and lacking a strong probabilistic basis despite its widespread use.
P-values are discussed as stochastic variables, which may not converge to a single value due to the law of large numbers, especially with small sample sizes.
The concept of survivorship bias is introduced, where only the successful outcomes are observed, leading to skewed perceptions.
The speaker warns of the potential for 'hacking' stochastic variables by repeatedly trying to achieve a desired outcome, which can distort results.
The distribution of the maximum value in a stochastic process is different from the distribution of the variable itself.
A detailed explanation of what p-value is, including its calculation and the assumptions behind it.
The p-value is revealed to be stochastic, with a distribution that often has most values below its true mean.
The probability of a p-value being less than certain thresholds (e.g., 0.01 or 0.05) is discussed, highlighting the skewness of the distribution.
The speaker criticizes the use of p-values in experiments, suggesting that they can be easily manipulated to achieve desired results.
The distribution of the maximum p-value in multiple experiments is discussed, and how it can lead to misleading conclusions.
A recommendation against relying solely on p-values, suggesting that alternative statistical methods should be considered.
The speaker notes that p-values are particularly favored in psychology due to the ease of use with smaller sample sizes.
A critique of the quantitative approach in psychology, suggesting that the reliance on p-values is flawed.
The speaker emphasizes the need for caution when using p-values and the importance of understanding their stochastic nature.
The transcript concludes with a general warning against the misuse of p-values and a call for more rigorous statistical practices.
The speaker provides a chapter reference for further reading on the topic, indicating a comprehensive discussion in their book.
Transcripts
friends hello again
today i finally talked about p-value
and to be honest i'm not crazy about
uh this lecture so if you don't know
what p-value means
forget about it don't just don't don't
follow
because it's a concept that is not very
solid
it doesn't have any strong probabilistic
basis
and yet is used uh abundantly
let's recap from uh the session on
correlation
hacking uh and and
and the metrification of things let's
recap
and focus on the two central points
metrics are often stochastic variables
in other words the random variables
that by the law of large numbers
converge to something
sometimes they don't
but they're not deterministic
so in sample you may get a different
result
each time you try if particularly if
your
n observation is not very large that's
the first point
the second point is whenever something
is stochastic
you have what i
call in most of the books uh this
sanitary assignment evidence in other
words there are a lot of things you
don't see
the survivorship bias if you will
we saw it and fooled by randomness when
you have a collection of traders
you only see the upper bound people
making money
likewise as we saw in the black swan
how you tend to see events that were
recorded
and have a bias and again this is the
upper bound
so the second point to remember is that
you have hacking of these variables
because someone could keep trying
when something is stochastic to get the
upper bound
and the upper bound on average
the distribution of the maximum is
different from the distribution of the
variable
itself so people do not know
that p-value is stochastic or at least
from what i've seen since i had to write
a paper on it
the paper is buried in here chapter 19
statistical consequences of fat tales
and you can find it on archive because
everything i write
must be available for free for those who
like you know
electronic versions so
what is the problem when we use
correlation
say i have x and y
two vectors and then an observation and
n is small you know you're gonna get a
stochastic number
when n becomes large compresses you
reveal your end so people can know what
you know whether your
correlation is statistically significant
or not
so when you use correlation okay you
provide people with an end
the problem with the p-value is that it
is not
provided with an n because supposedly
the
end comes out of it hence the problem
we're going to see now
let me start from the beginning what is
p value
i have observation x 1 x n
and let's say i want to see if the mean
the mean x bar if the mean
is uh different
from say zero i'm gonna simplify
okay you can use something different
than zero let me simplify
so the statistic is going to be x bar
minus zero over sigma the
standard deviation of the elevation
square root of n
okay so
that metric let's call it the z okay
the z is going to be the variable under
consideration
and the p value is probability
okay that
z higher than z
in other words probability of exceeding
c
now you have the illusion that square
root of n
is a scaling here that takes out the
subtleties of the problem but in fact it
does not
i'm going to see what the result is
going to be like
in fact what we're considering is the
distribution
assuming the mean of zero okay
of z higher than that number
and that is the p-value okay
so to re-simplify
i'm assuming that that i'm taking
the exceedance probability or so-called
survival
uh survivor survival function
the probability exceeding a certain
number using the distribution as the
mean was zero
and that number can be 0.05 0.06
0.1 uh
0 0 1 etc now
what is the problem it turns out
that the probability p value
probability of exceeding z is itself
stochastic
so what do i mean by stochastic this is
this distribution
and it's going to look like this
this is this true mean
mean value and it's going to have
observations
below the mean in fact most observations
are gonna be below the mean
so and to give you actually the the
difficulties of this and you can get it
in the book it's page
339 chapter
chapter 19 okay in my book or download
it from archive
let's say the mean is about
now 11. the true p-value is eleven if
you do it a million times
on the same ensemble of things you get
point eleven
the probability the p-value probability
that a p-value
less than 0.01
is 25 percent and we've got a p-value
less than
0.05 53
so part one just discussed
p-value is stochastic it tends to be so
skewed that most value will be below the
true mean and if the true mean is 11
you're going to have more than half the
observation below 0.05
starting with that things get worse how
how do people game a metric
okay you run experiments
experiment one and you take the maximum
the maximum okay
so the distribution of the maximum is
gonna be lower
you see so odds are that if you do four
or five times
you're going to get a p-value of 0.01
with something
with a true p-value point 11.
now you may tell me point 11 is better
than random i say yes
but your 0.01 is not 0.01
which is why for it to not be gained
the p-value should be considerably
smaller
than 1 so we're off by more than other
magnitude
so let me repeat
p-value is a metric that metric
is not thought as being stochastic but
it is
stochastic and the fact that the end
number of observation comes out
of the equation is uh
you know what makes it a bad bad metric
in fact not only it is stochastic but
it's very stochastic
as you can see most of the elevation
fall below its true mean
so beware when you do p-values try to do
something else
and it looks like the only people you
know who like
p-value are psychologists because
they avoid having to do homework by
having a larger uh
uh n in in in medicine ends tend to be
large
uh and psychologists tend to be small
usually college people on campus first
of all i think
psychology has a bigger problem p-value
is pretty much the quantitative approach
to it is severely flaws flawed so
thank you for listening to me and have a
nice whatever it's
so i think it's the weekend here it's a
great great day thanks
have a good day thanks bye
Browse More Related Video
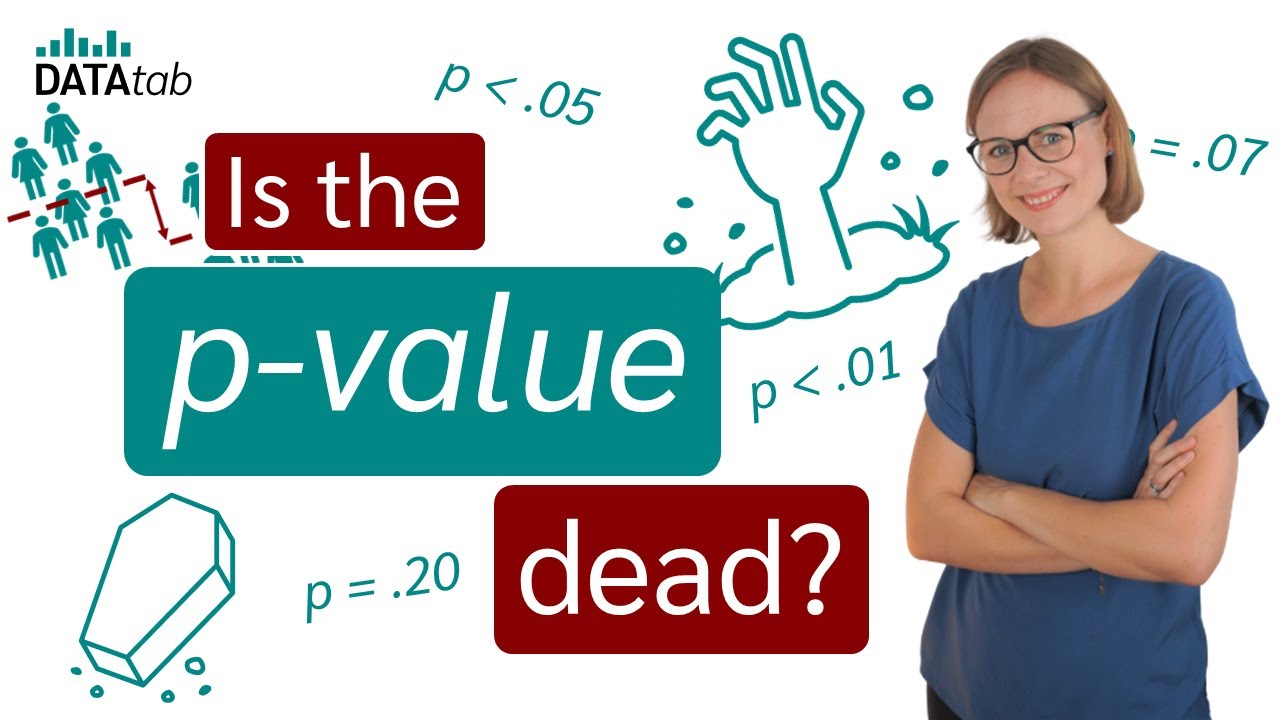
Why the p-Value fell from Grace: A Deep Dive into Statistical Significance

p-values: What they are and how to interpret them
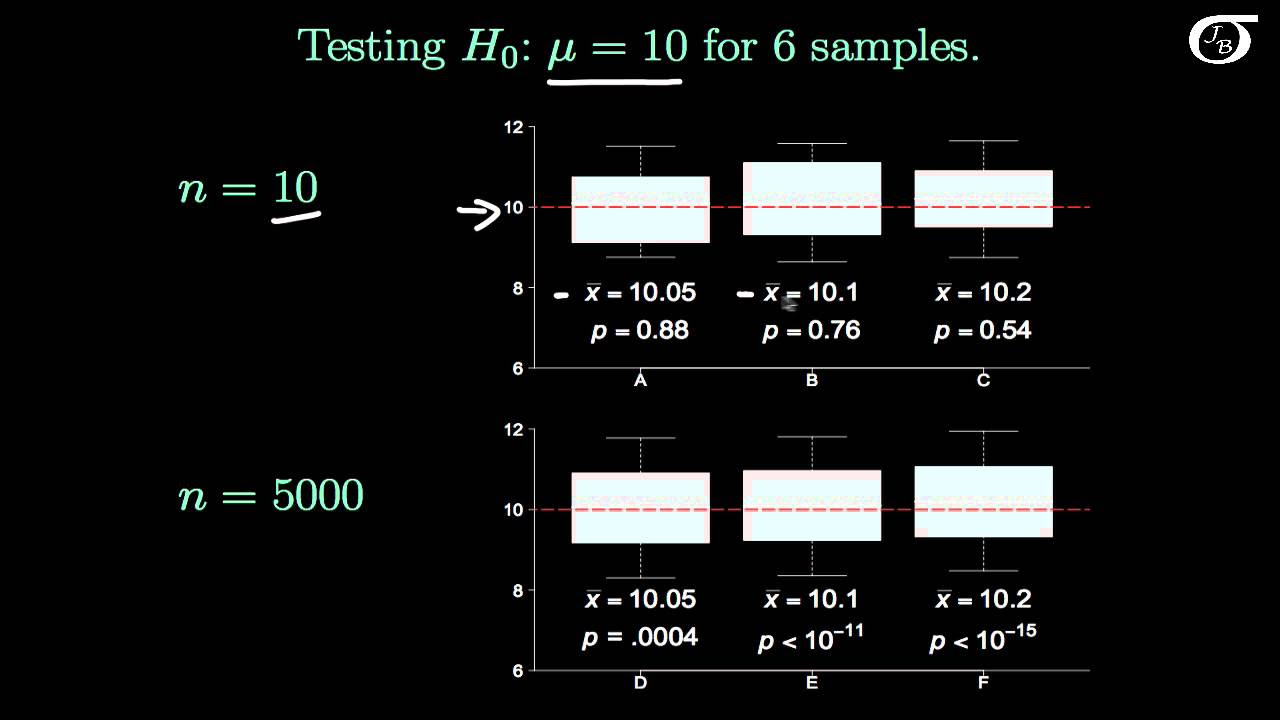
Statistical Significance versus Practical Significance
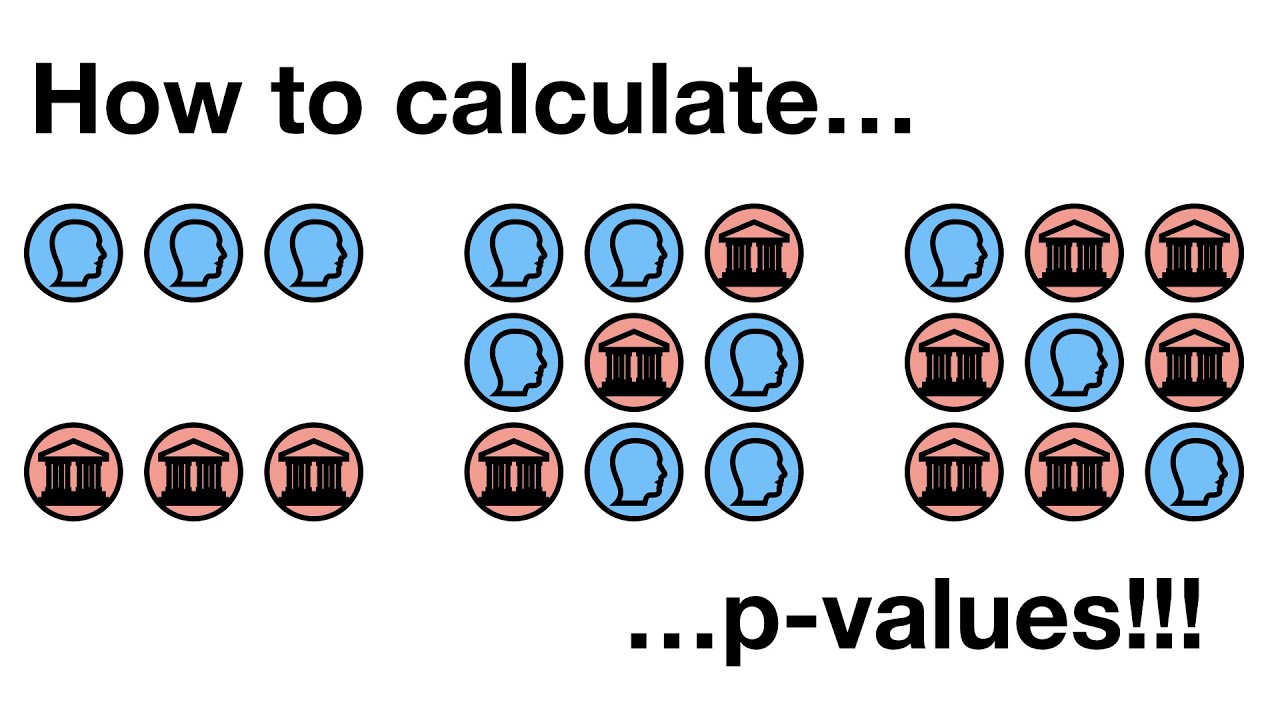
How to calculate p-values

Confidence Intervals, Clearly Explained!!!
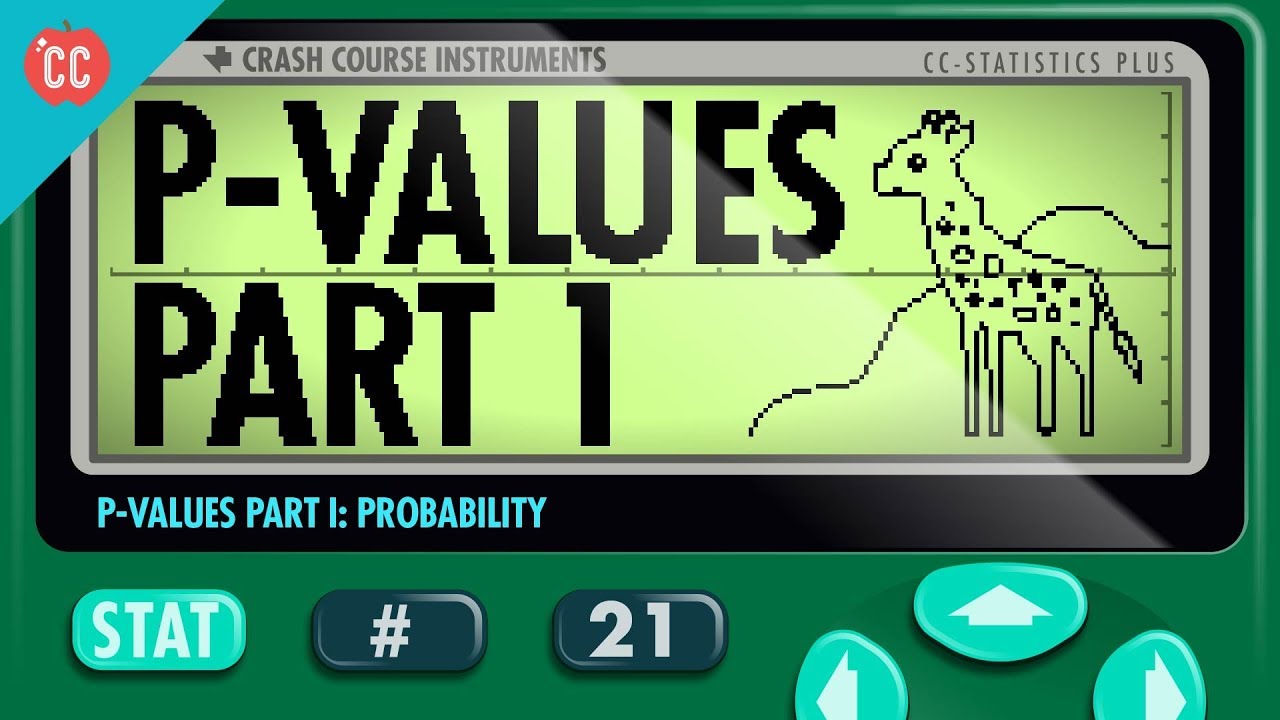
How P-Values Help Us Test Hypotheses: Crash Course Statistics #21
5.0 / 5 (0 votes)