What does AI mean for the future of IT? | Cloud Chat Ep. 35
Summary
TLDRIn this episode of Cloud Chat, Stephan and Matt delve into the implications of artificial intelligence, particularly large language models like Chat GPT, on business and IT. They discuss the challenges of intellectual property exposure, the impact on education and research, and the ethical considerations of AI's decision-making capabilities. The conversation highlights the importance of data management and the role of NetApp in facilitating AI's potential through optimized data storage and access across various platforms.
Takeaways
- π§ AI and large language models are top of mind for many, with Chat GPT being a significant driver in capturing attention and making AI more approachable.
- π Intellectual property concerns are paramount when exposing AI to information, as IT organizations must balance leveraging AI with protecting proprietary data.
- π¨βπ« Educational institutions are grappling with AI's impact on academic integrity, as students can now use AI to generate term papers, prompting a reevaluation of how research is verified.
- π The integration of AI in everyday life is becoming more prevalent, with implications in politics, personal data, and even in how we perceive reality and truth.
- π€ The distinction between AI and machine learning is important; AI is about machines making decisions based on data, whereas machine learning is about teaching systems to behave in certain ways.
- π IT shops are compelled to embrace AI while figuring out how to manage and scale it without compromising intellectual property or sensitive information.
- π The inadvertent exposure of code to public large language models raises concerns about the loss of control over intellectual property and the potential misuse of such information.
- π‘ AI can assist in overcoming initial inertia in projects by providing first drafts or ideas, which can then be refined by human input.
- π§ Practical applications of AI include improving efficiency in tasks such as writing, coding, and even in the development of robotics.
- π« The potential for AI to cause harm, such as providing harmful code for a robotic arm, underscores the importance of oversight and the consideration of liability.
- π As AI continues to evolve, the challenge lies in how to classify and protect data at a more abstract level, ensuring that the combination of disparate data sets does not inadvertently create sensitive information.
Q & A
What is the main concern regarding the use of AI in business as discussed in the script?
-The main concern is about what information is exposed to artificial intelligence, particularly in relation to protecting intellectual property and avoiding unintentional exposure of sensitive data.
How has the advent of large language models like Chat GPT made AI more approachable to people?
-Large language models have made AI more approachable by allowing people to interact with it directly, making it feel more real and accessible beyond specialized fields like self-driving car development.
What impact is AI having on educational institutions as mentioned in the script?
-AI is impacting educational institutions by raising concerns about students using online systems to write term papers, prompting teachers and universities to refine their programs to ensure original research.
How is AI affecting the political season in terms of information dissemination as discussed?
-AI is affecting the political season by influencing the material and facts that people receive, necessitating a higher level of awareness and intelligence about the information being consumed.
What is the significance of AI 'hallucinations' in the context of IT organizations?
-AI 'hallucinations' refer to the generation of incorrect or misleading outputs by AI systems. IT organizations need to navigate this by ensuring the accuracy of AI outputs and managing the implications of such errors.
How does NetApp IT utilize AI in managing data from systems?
-NetApp IT uses AI to analyze data coming in from systems, understand patterns of behavior from IoT data, and make decisions based on that data.
What are some practical uses of AI that Matt Brown has experienced?
-Matt Brown has used AI for ideation, overcoming initial inertia in projects, and for writing Python code, which helped him get to the first draft faster and refine from there.
What ethical considerations does AI bring to the table in terms of liability?
-AI brings up questions about liability when AI-generated outputs lead to negative consequences, such as physical damage or incorrect advice, and who is responsible for those outcomes.
Why did Chat GPT refuse to participate in gambling-related queries in the script?
-Chat GPT refused to participate in gambling-related queries due to its programming to avoid engaging in activities that could be considered unethical or inappropriate.
How does the script illustrate the importance of data classification in the context of AI?
-The script illustrates the importance of data classification by discussing how the combination of disparate data sets can create new, sensitive information that requires careful handling and classification to prevent misuse.
What role does NetApp play in the AI space according to the script?
-NetApp plays a crucial role in the AI space by providing the underlying infrastructure that supports AI, enabling the quick and efficient movement and processing of data, which is essential for AI models to learn and operate effectively.
Outlines
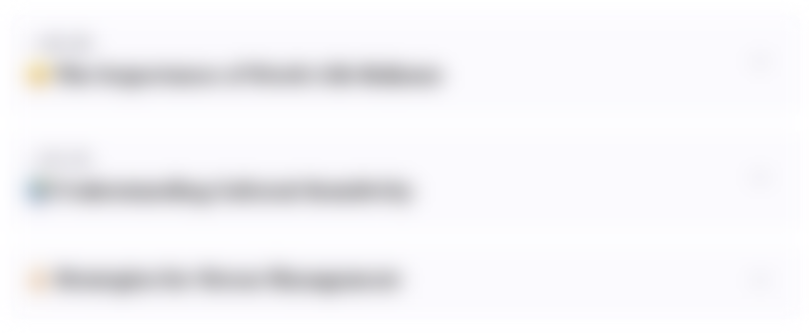
This section is available to paid users only. Please upgrade to access this part.
Upgrade NowMindmap
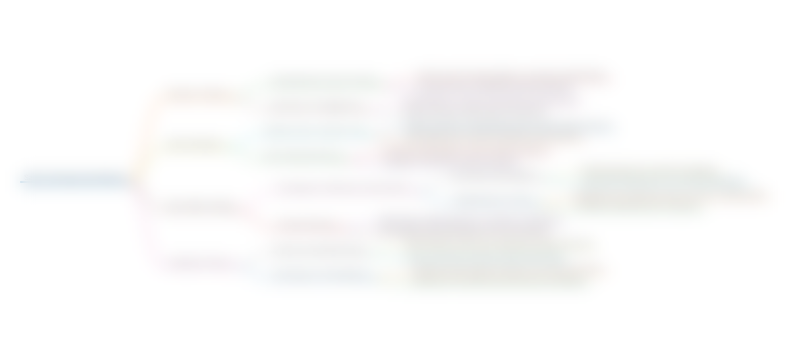
This section is available to paid users only. Please upgrade to access this part.
Upgrade NowKeywords
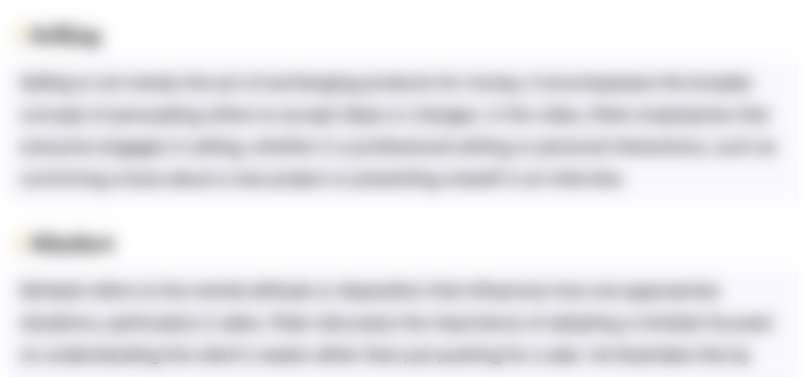
This section is available to paid users only. Please upgrade to access this part.
Upgrade NowHighlights
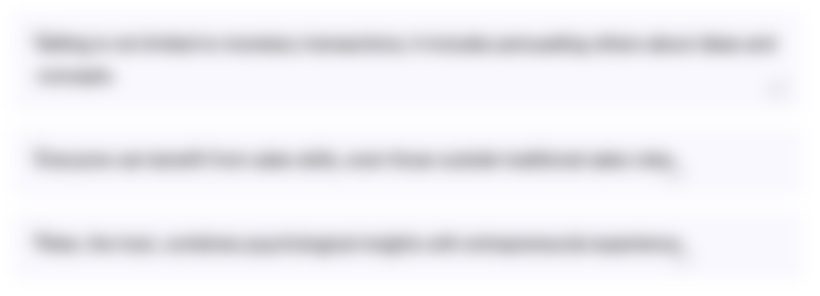
This section is available to paid users only. Please upgrade to access this part.
Upgrade NowTranscripts
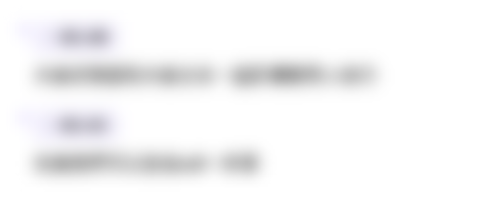
This section is available to paid users only. Please upgrade to access this part.
Upgrade NowBrowse More Related Video
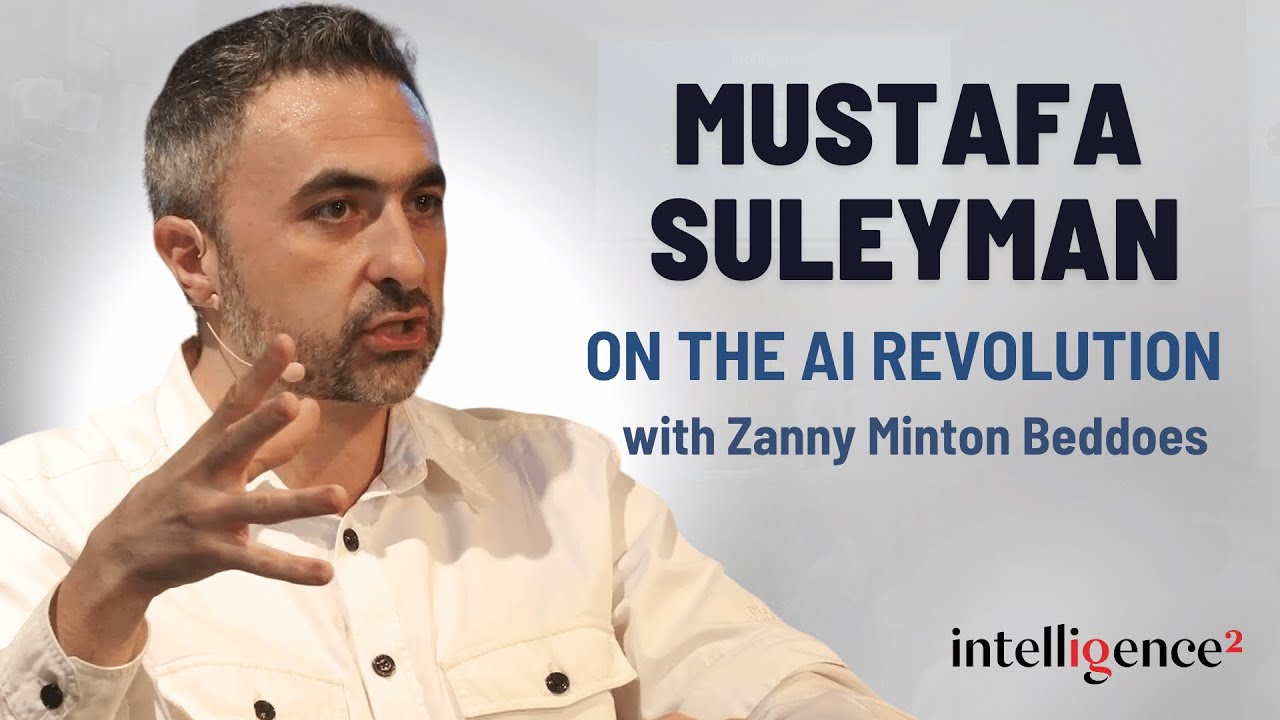
Mustafa Suleyman on The Coming Wave of AI, with Zanny Minton Beddoes

EP7 reduzido
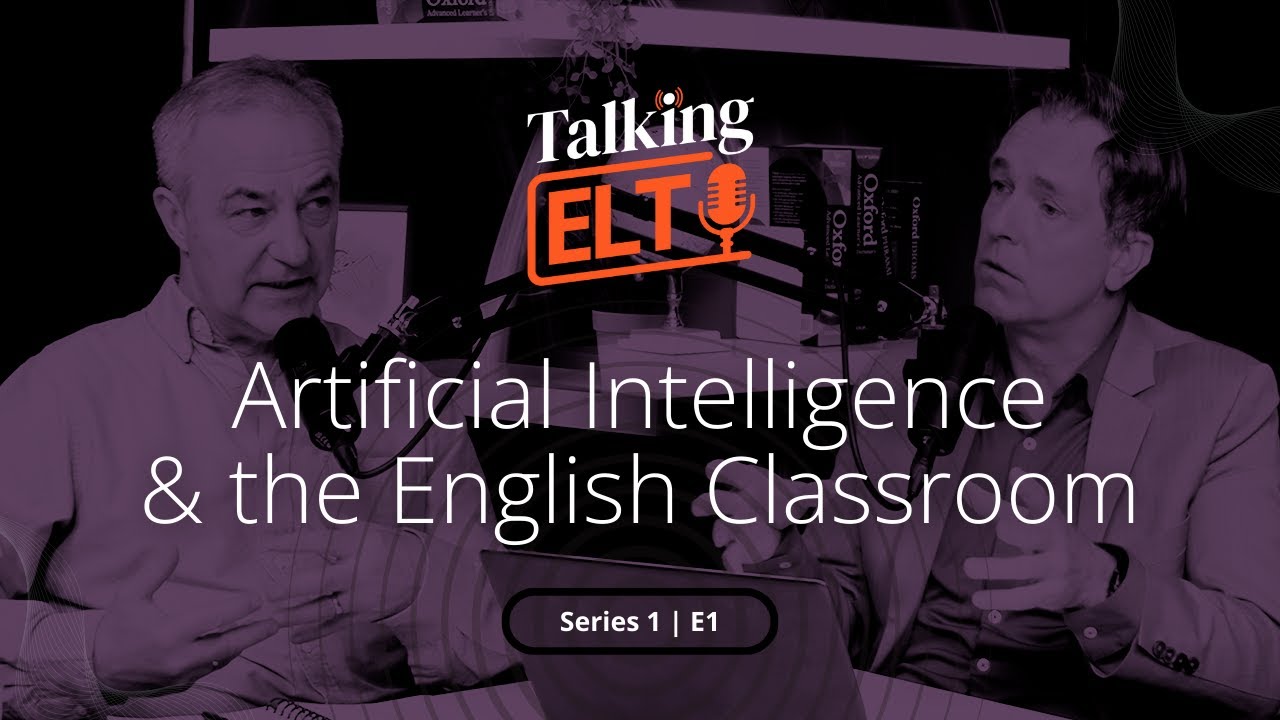
Artificial Intelligence: The Impact on Language Teaching (Talking ELT Episode 1)
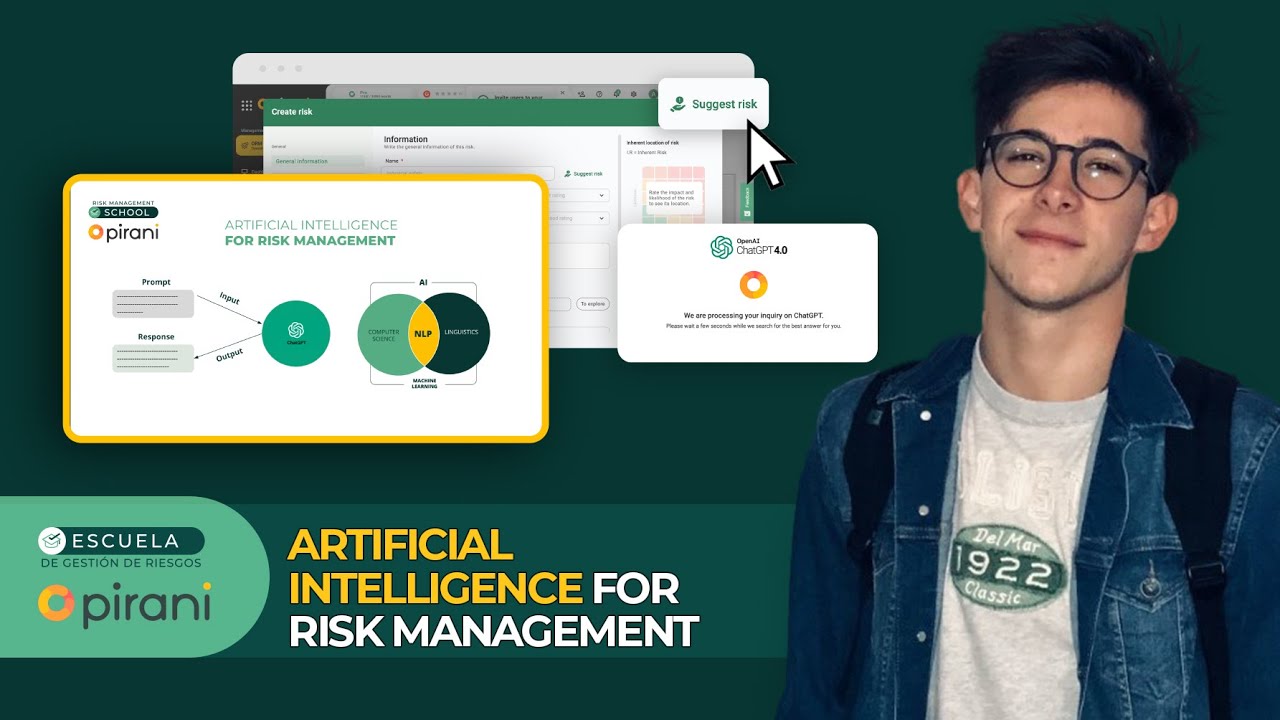
How works Artificial Intelligence for risk management
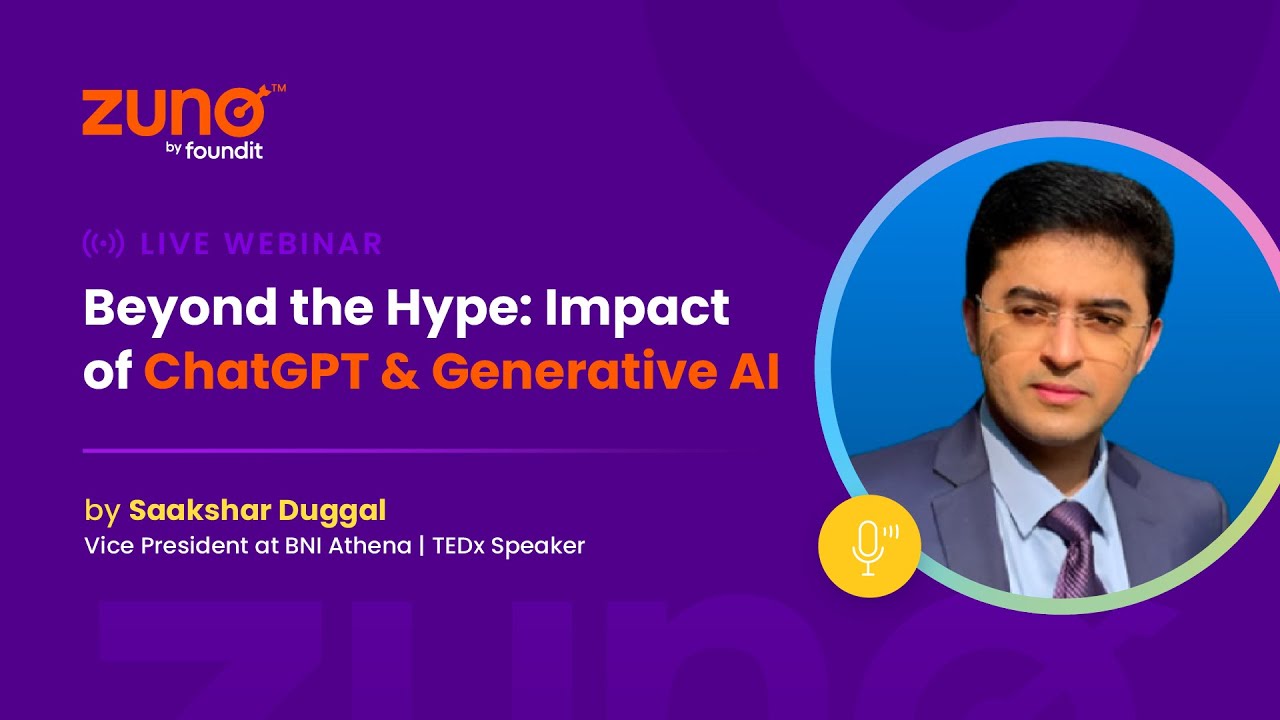
Beyond the Hype Impact of ChatGPT & Generative AI 2 | Zuno Weekly Events
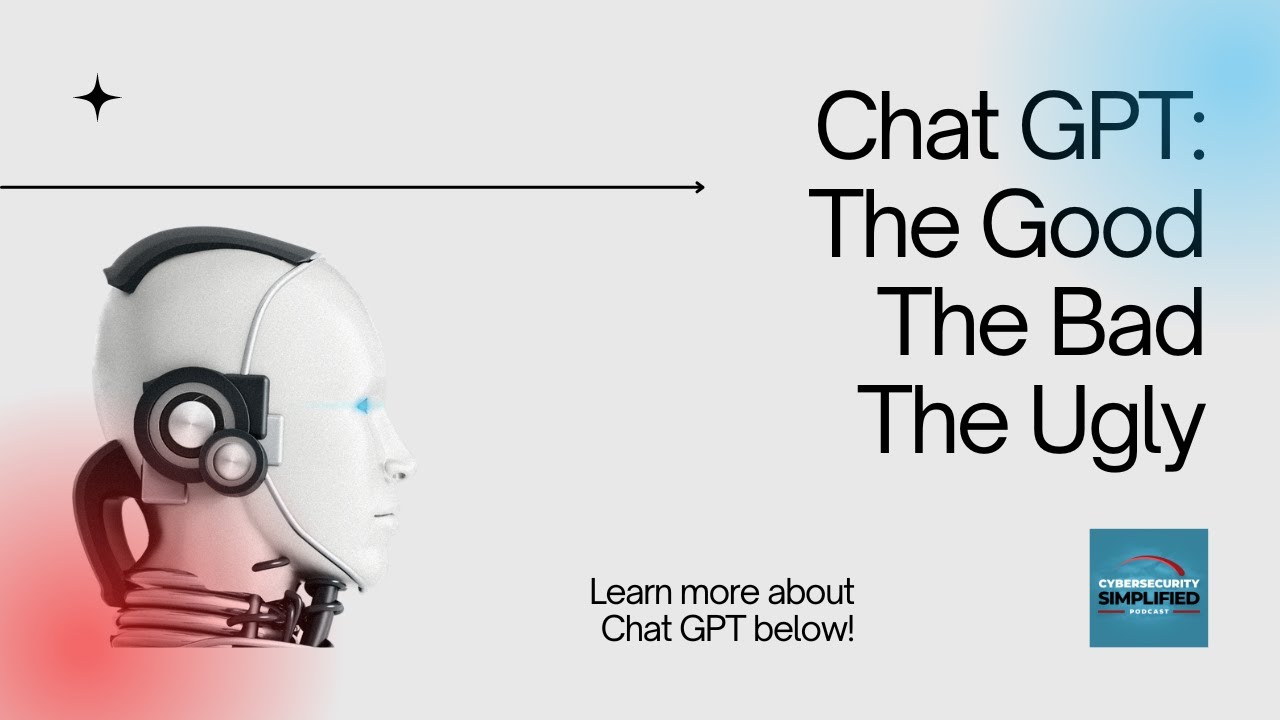
Cybersecurity Simplified β Episode 42: ChatGPT and Cybersecurity β The Good, Bad & Ugly
5.0 / 5 (0 votes)