Brain Criticality - Optimizing Neural Computations
Summary
TLDRThe video script delves into the critical brain hypothesis, which likens the brain's neural networks to a system at the brink of phase transition, akin to Maxwell's equations for electromagnetism. It explains phase transitions, critical points, and their relevance to neuroscience, suggesting that the brain operates near a critical point to optimize information processing. The script explores concepts like neuronal avalanches, power laws, and the balance between excitation and inhibition, highlighting the brain's computational efficiency at the critical state.
Takeaways
- 🧠 Understanding the brain's complexity is a major challenge in neuroscience, with a goal to develop a comprehensive theory similar to Maxwell's equations for electromagnetism.
- 🌟 The critical brain hypothesis has gained attention; it suggests that neural networks operate near a phase transition point, akin to the critical state of water molecules coexisting in liquid and gas phases.
- 🔍 The concept of phase transitions is introduced with the example of water boiling, illustrating how energy can lead to a change in the macroscopic properties of a system.
- 📊 Phase transitions are characterized by an order parameter and driven by a control parameter, with first-order transitions showing a discontinuity and second-order transitions being continuous.
- 🌡 The Ising model, used to explain magnet properties, is highlighted as an example of a system undergoing a second-order phase transition, leading to a critical point with unique properties.
- 🌐 At the critical point, systems exhibit long-range communication and scale-free behavior, with self-similar patterns observable at all scales, a characteristic of fractals.
- 📉 Neuronal avalanches, observed in neural cultures, are bursts of activity that spread across networks and are indicative of the brain operating near a critical point.
- 📈 The distribution of features of neuronal avalanches follows a power law, suggesting a lack of characteristic scale in brain activity and supporting the idea of criticality in neural systems.
- 🔄 The balance between excitation and inhibition in the brain is crucial and can be adjusted to demonstrate phase transitions, with compounds that block these processes causing shifts from critical dynamics.
- 💡 Operating near a critical point is beneficial for the brain as it optimizes information processing and computational power, allowing for efficient transmission and reduction of uncertainty about inputs.
- 📚 The study of criticality in neuroscience is an exciting and growing field, with potential applications in understanding brain function and developing treatments for neurological disorders.
Q & A
What is one of the Holy Grails of Neuroscience?
-One of the Holy Grails of Neuroscience is to build an elegant yet comprehensive theory that describes the general roles of the nervous system, similar to the impact of Maxwell's equations on electromagnetism.
What does the critical brain hypothesis propose about the operation of networks of neurons?
-The critical brain hypothesis proposes that networks of neurons operate near a point of phase transition, in a special critical state similar to when water molecules coexist in liquid and gaseous phases.
How does the phase transition concept relate to the boiling of water?
-The phase transition concept relates to the boiling of water as an example of a first-order transition, where water changes from a liquid to a gaseous state, absorbing latent heat and undergoing a qualitative change in properties.
What is an order parameter in the context of phase transitions?
-An order parameter is a macroscopic property that quantifies the organization of a system, such as entropy, volume, fluidity, or surface tension in the case of water.
How does the control parameter influence the phase transition?
-The control parameter, such as temperature or pressure, drives the phase transition by altering the system's state. It is an independent variable that can be freely varied in an experiment.
What is a continuous or second-order phase transition?
-A continuous or second-order phase transition is a type of phase transition where the change in the order parameter is continuous, allowing the system to smoothly transition from one phase to another.
What is the significance of the critical point in a phase transition?
-The critical point is a unique intermediate state at the interface of two different phases where the boundaries are blurred, and new properties emerge. It is a point of balance between order and disorder.
How does the Ising model relate to the concept of criticality in the brain?
-The Ising model, originally developed to explain the properties of magnets, demonstrates how a system can undergo a second-order phase transition, exhibiting properties of criticality such as long-range communication and scale-free behavior, which are relevant to understanding neural networks.
What is the role of the correlation length in the Ising model?
-The correlation length in the Ising model is the distance at which the dynamic correlation between spins drops to zero. It indicates the extent of long-range communication in the system, peaking at the critical point.
What is the significance of scale-free behavior in the context of the brain?
-Scale-free behavior signifies that the system, such as the brain, has no characteristic scale and resembles itself at any scale. This property is indicative of criticality and is associated with optimal information processing and computational power.
How does the branching ratio (Sigma) act as a control parameter in neural networks?
-The branching ratio (Sigma) is a control parameter that governs the transition from subcritical to supercritical dynamics in neural networks. When Sigma equals 1, the network is at a critical point where activity is neither decaying nor amplifying, and information transfer is optimized.
What experimental evidence supports the idea that the brain operates near a critical point?
-Experimental observations such as neuronal avalanches, which are power-law distributed patterns of activity in neural networks, support the idea that the brain operates near a critical point. These have been observed in various species and scales, from single neurons to EEG recordings.
Why is operating near a critical point considered beneficial for the brain?
-Operating near a critical point is considered beneficial for the brain because it maximizes capabilities such as information processing and computational power. At the critical point, the brain can efficiently transmit information and maintain a balance between activity and quiescence.
Outlines
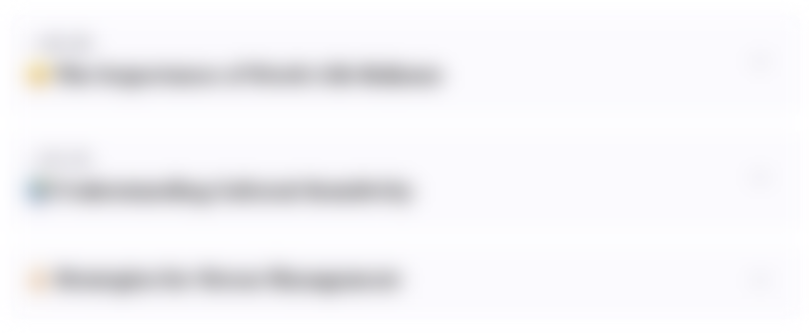
This section is available to paid users only. Please upgrade to access this part.
Upgrade NowMindmap
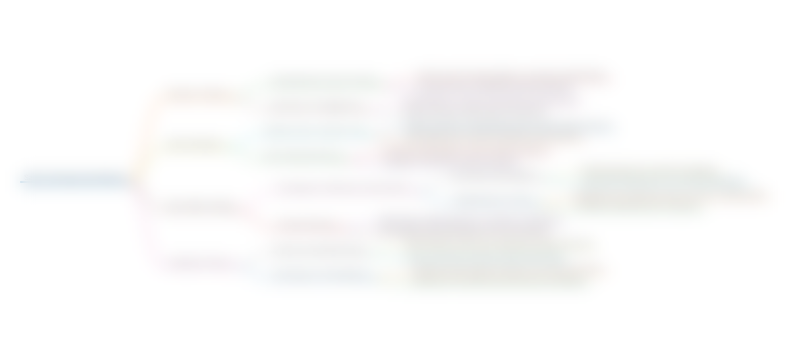
This section is available to paid users only. Please upgrade to access this part.
Upgrade NowKeywords
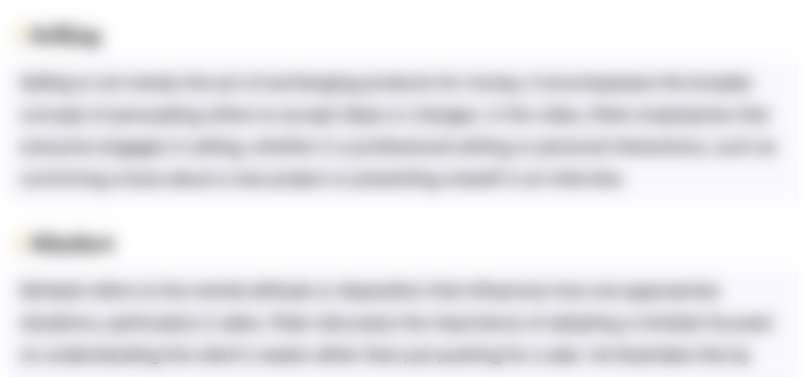
This section is available to paid users only. Please upgrade to access this part.
Upgrade NowHighlights
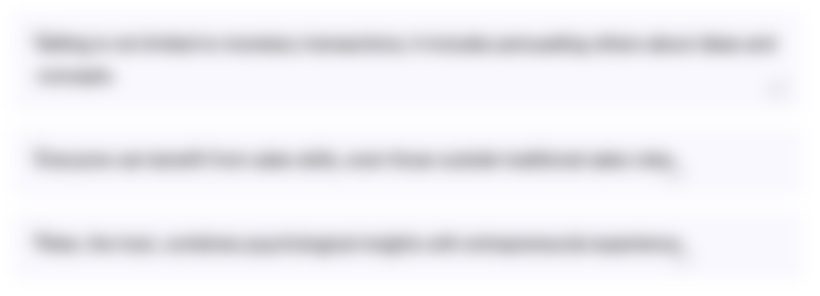
This section is available to paid users only. Please upgrade to access this part.
Upgrade NowTranscripts
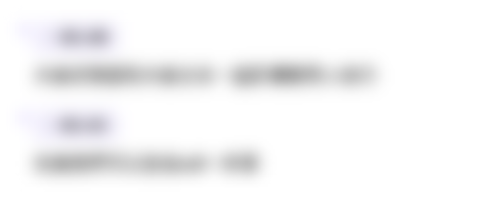
This section is available to paid users only. Please upgrade to access this part.
Upgrade NowBrowse More Related Video
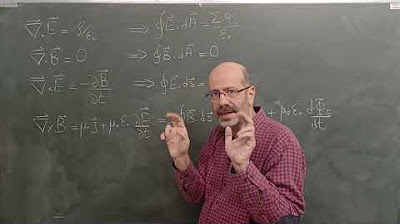
Maxwell Denklemleri Ne Anlama Gelir?
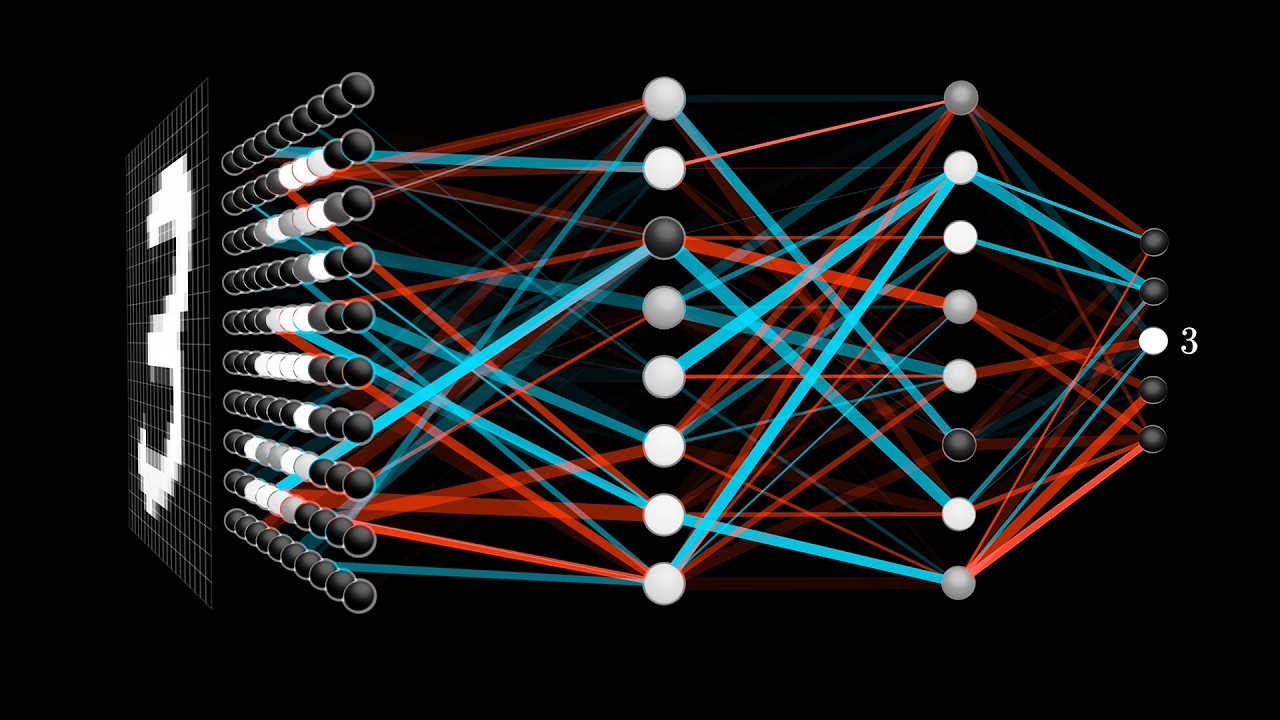
But what is a neural network? | Chapter 1, Deep learning
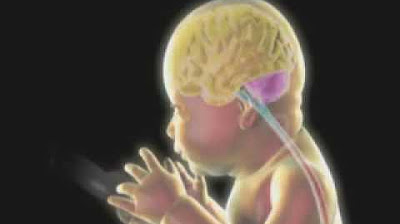
Prenatal Brain Development
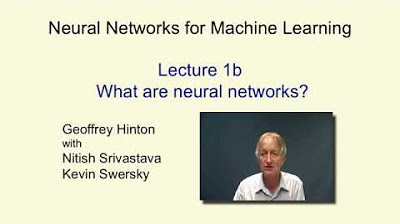
Lecture 1.2 — What are neural networks — [ Deep Learning | Geoffrey Hinton | UofT ]

A Lei de Ampère-Maxwell: Campos elétricos induzem campos magnéticos.
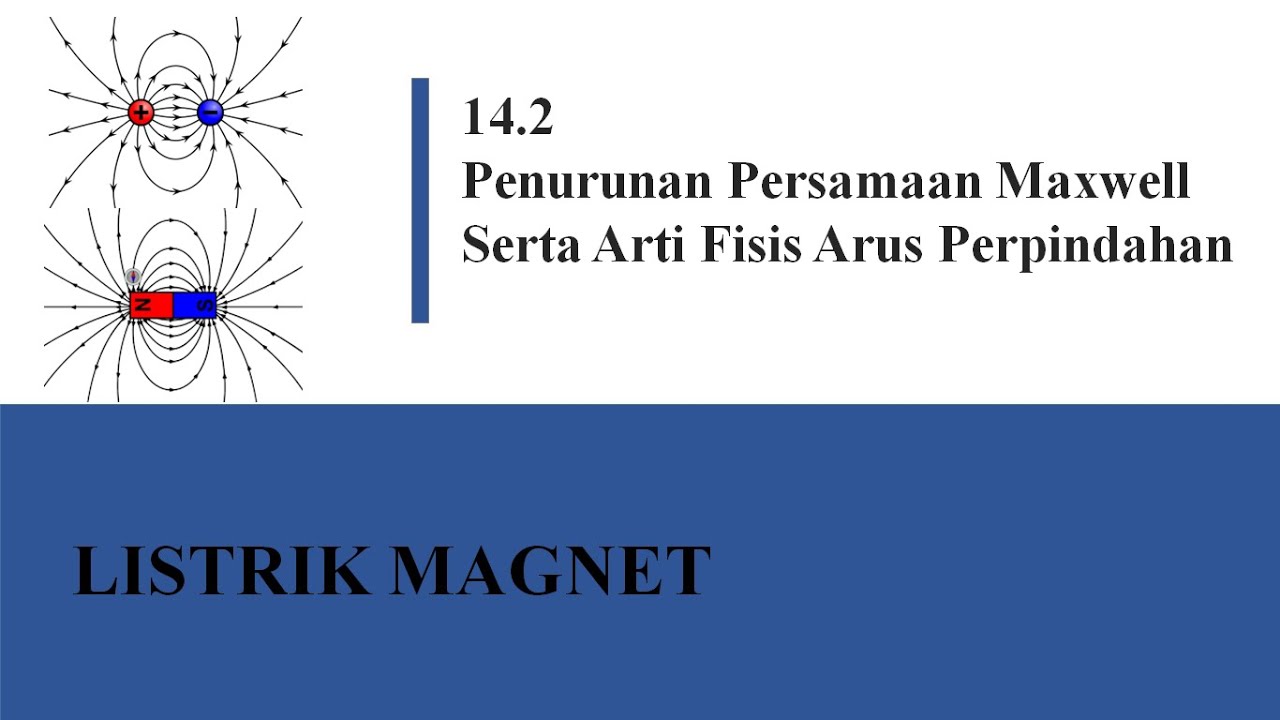
LISTRIK MAGNET 14 2 Penurunan persamaan Maxwell serta arti fisis Arus Perpindahan
5.0 / 5 (0 votes)