Which nVidia GPU is BEST for Local Generative AI and LLMs in 2024?
Summary
TLDRThe video discusses advancements in open-source AI, emphasizing the ease of running generative AI locally for images, video, and podcast transcription. It explores the cost-effectiveness of Nvidia GPUs for compute tasks, comparing them to Apple and AMD. The script delves into the latest Nvidia RTX 40 Super Series GPUs, their AI capabilities, and the potential of using older models for AI development tasks. It also highlights the significance of Nvidia's Tensor RT platform for deep learning inference and showcases the impressive performance of modified enterprise-grade GPUs in a DIY setup.
Takeaways
- 🚀 Open source AI has seen significant advancements, enabling local generation of AI content for images, video, and even podcast transcriptions at a rapid pace.
- 💡 Nvidia GPUs are currently leading in terms of cost of compute for AI tasks, with Apple and AMD being close competitors.
- 💻 The decision between renting or buying GPUs often leans towards buying for those who wish to experiment and develop with AI tools like merge kits.
- 🔍 Nvidia's messaging is confusing due to the variety of GPUs available, ranging from enterprise-specific to general consumer products.
- 📅 The release of Nvidia's RTX 40 Super Series in early January introduced GPUs with enhanced AI capabilities, starting at $600.
- 🔢 The new GPUs boast improved performance metrics such as Shader teraflops, RT teraflops, and AI tops, catering to gaming and AI-powered applications.
- 🎮 Nvidia's DLSS (Deep Learning Super Sampling) technology allows for AI-generated pixels to increase resolution in games, enhancing performance.
- 🤖 The AI tensor cores in the new GPUs are highlighted for their role in high-performance deep learning inference, beneficial for AI models and applications.
- 🔧 Techniques like model quantization have made it possible to run large AI models on smaller GPUs, opening up more affordable options for AI development.
- 🌐 Nvidia's Tensor RT platform is an SDK that optimizes deep learning inference, improving efficiency and performance for AI applications.
- 💡 The script also discusses the use of enterprise-grade GPUs in consumer settings, highlighting the potential for high-performance AI tasks outside of professional environments.
Q & A
What advancements in open source AI have been made in the last year according to the transcript?
-The transcript mentions that there have been massive advancements in open source AI, including the ease of running local large language models (LLMs) for generative AI like Stable Diffusion for images and video, and the capability to transcribe entire podcasts in minutes.
What is the current best option in terms of cost of compute for AI tasks?
-The transcript suggests that Nvidia GPUs are currently the best option in terms of cost of compute for AI tasks, with Apple and AMD being close competitors.
Should one rent or buy GPUs for AI tasks according to the transcript?
-The transcript recommends buying your own GPU instead of renting for those who want to experiment and mix and match with tools or for developers who want to do more in-depth work.
What is the latest series of GPUs released by Nvidia as of the transcript's recording?
-Nvidia has released the new RTX 40 Super Series, which is a performance improvement over the previous generation, aimed at gaming and creative applications with AI capabilities.
What is the starting price for the new RTX 40 Super Series GPUs mentioned in the transcript?
-The starting price for the new RTX 40 Super Series GPUs is $600, which is around the same price as used RTX 3090s or 3090 Ti.
What is the significance of the AI tensor cores in the new Nvidia GPUs?
-The AI tensor cores in the new Nvidia GPUs deliver high performance for deep learning inference, which is crucial for AI tasks and applications, including low latency and high throughput for inference applications.
How does Nvidia's DLSS technology work, and what does it offer?
-DLSS, or Deep Learning Super Sampling, is a technology that infers pixels to increase resolution without the need for more ray tracing. It can accelerate full rate racing by up to four times with better image quality.
What is the role of Nvidia's Tensor RT in AI and deep learning?
-Nvidia's Tensor RT is an SDK for high-performance deep learning inference, which includes optimizations for runtime that deliver low latency and high throughput for inference applications, improving efficiency and performance.
What is the potential of quantization in making large AI models run on smaller GPUs?
-Quantization allows for the adjustment of the representation of underlying datasets, enabling large AI models that would normally require multiple GPUs to run on smaller ones, like the 3090 or even a 4060, with reasonable accuracy.
What are some of the models that Nvidia has enhanced with Tensor RT as mentioned in the transcript?
-Nvidia has enhanced models like Code Llama 70b, Cosmos 2 from Microsoft Research, and a lesser-known model called Seamless M4T, which is a multimodal foundational model capable of translating speech and text.
What is the situation with the availability of Nvidia's A100 GPUs in the SXM4 format according to the transcript?
-The transcript mentions that due to the discovery of how to run A100 GPUs outside of Nvidia's own hardware, it has become nearly impossible to find reasonably priced A100 40 and 80 GB GPUs in the SXM4 format on eBay.
Outlines
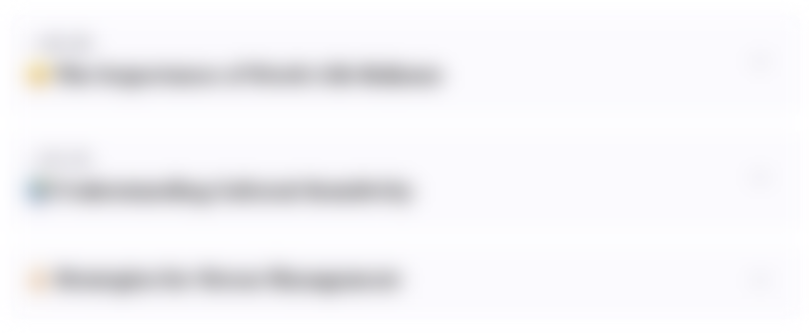
This section is available to paid users only. Please upgrade to access this part.
Upgrade NowMindmap
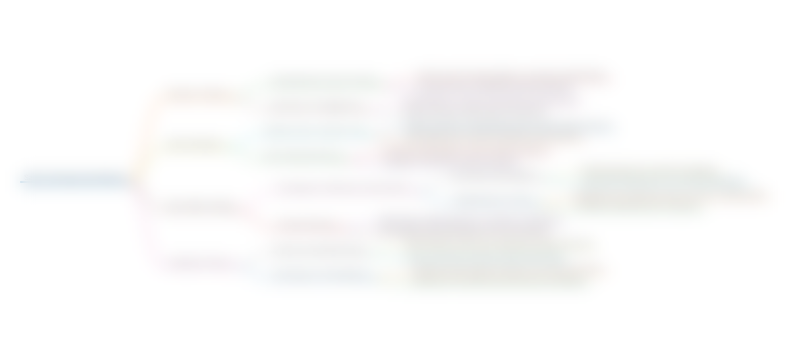
This section is available to paid users only. Please upgrade to access this part.
Upgrade NowKeywords
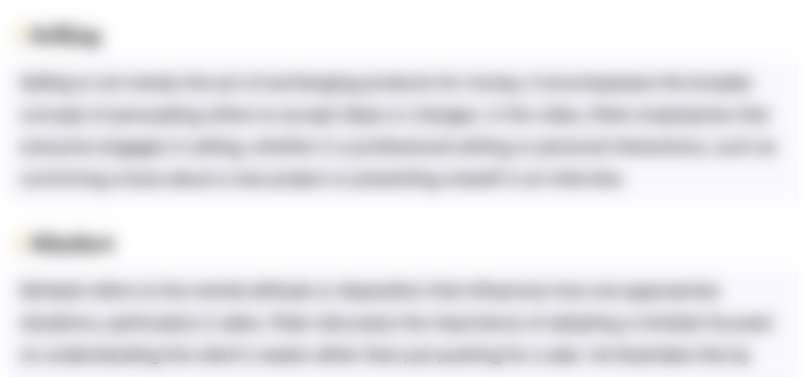
This section is available to paid users only. Please upgrade to access this part.
Upgrade NowHighlights
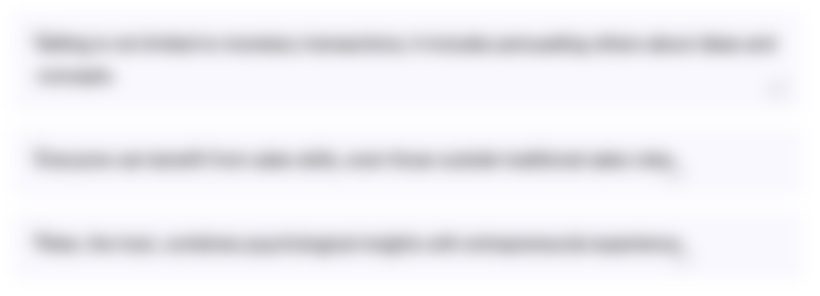
This section is available to paid users only. Please upgrade to access this part.
Upgrade NowTranscripts
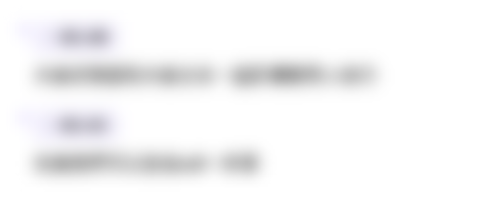
This section is available to paid users only. Please upgrade to access this part.
Upgrade NowBrowse More Related Video
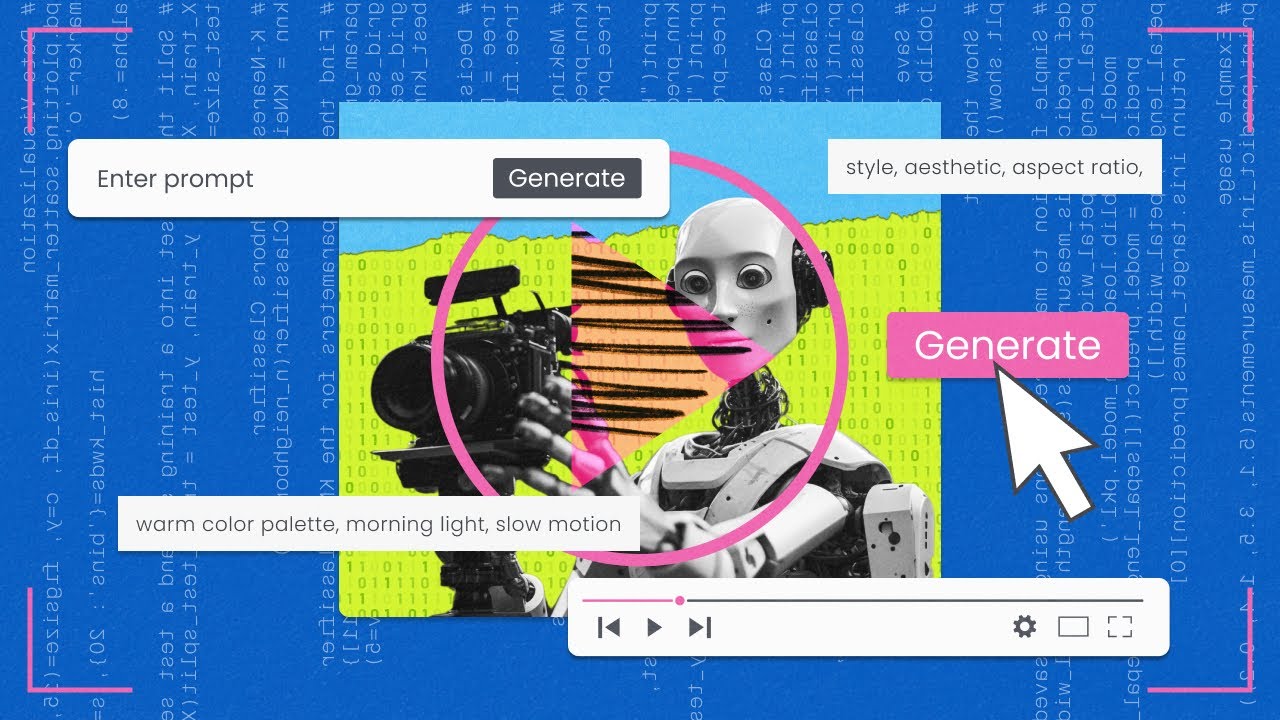
Text to Video: The Next Leap in AI Generation
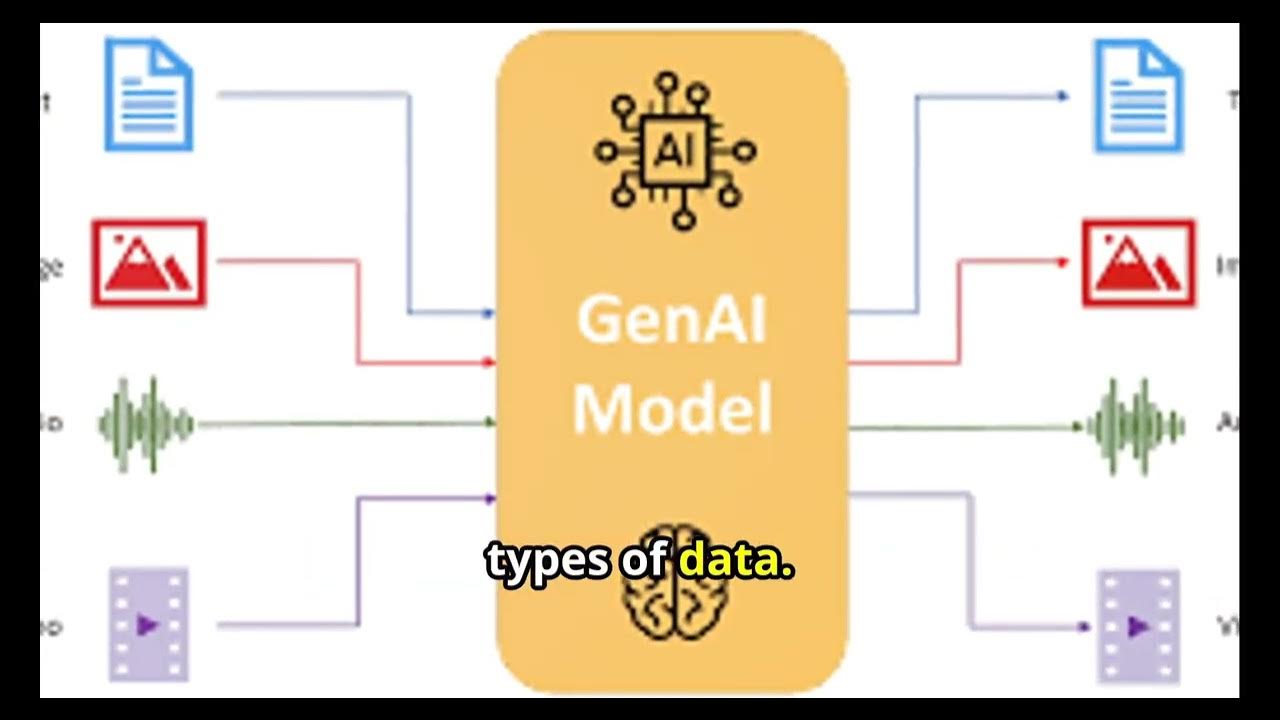
Generative AI for Absolute Beginners : Types of Generative AI

Big Wins for Open Source | TONs of New AI Projects! (All Open)
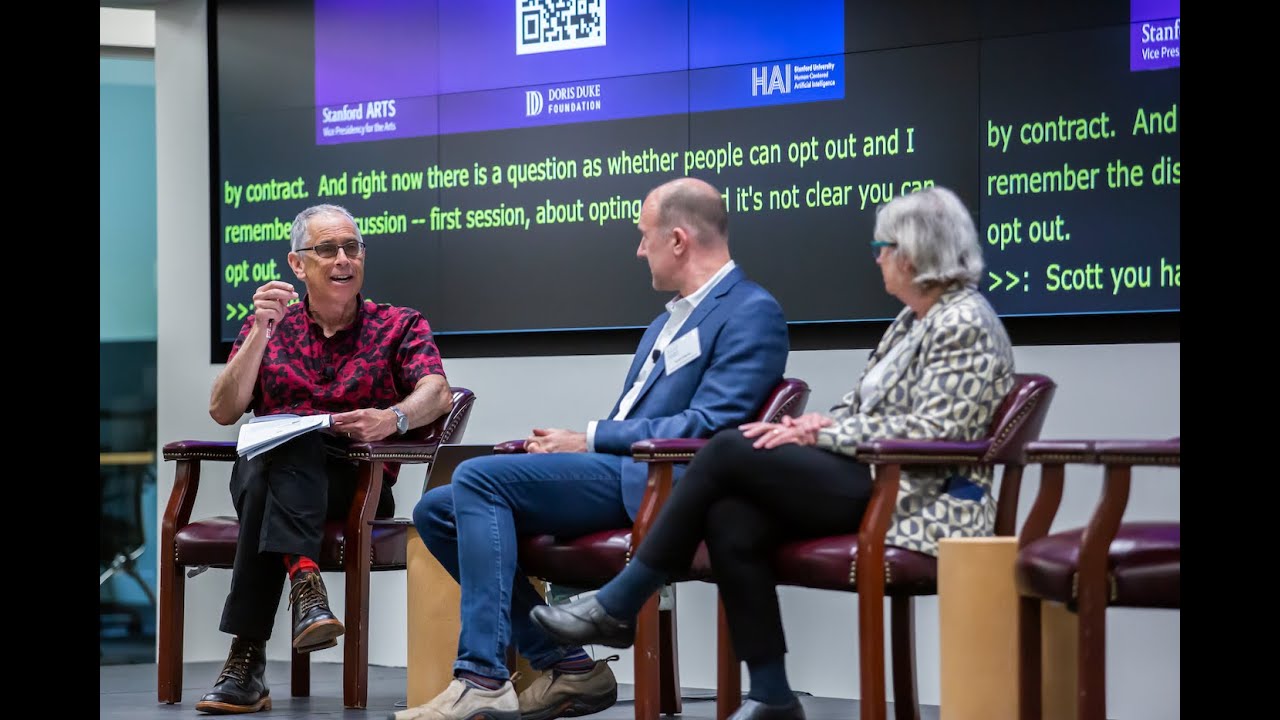
Creativity in the Age of AI: Generative AI Issues in Art Copyright & Open Source
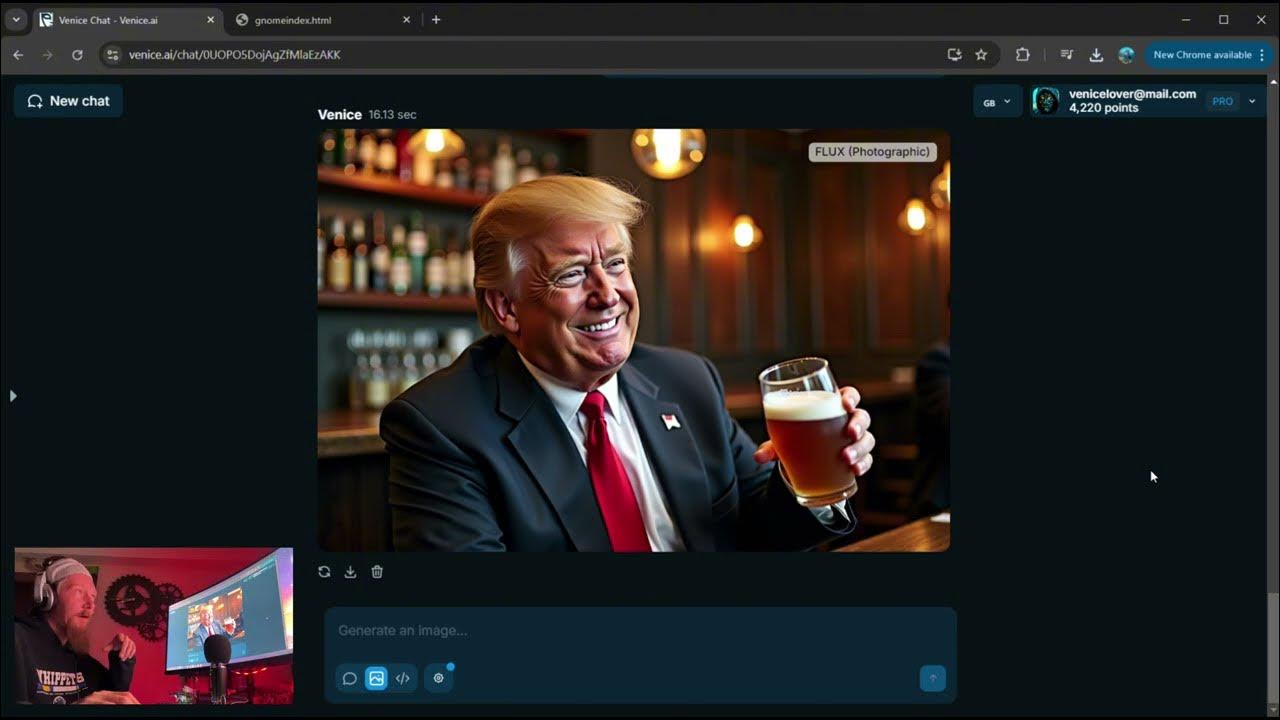
Venice AI Basic Tutorial
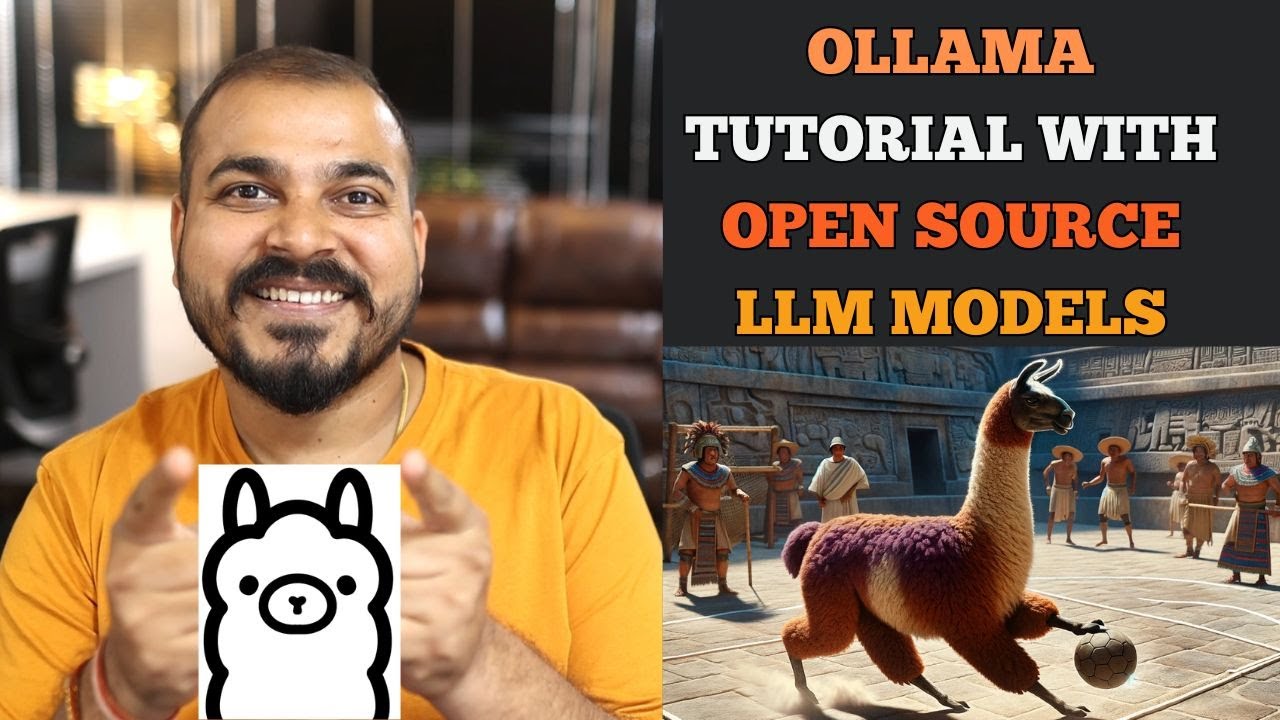
Ollama-Run large language models Locally-Run Llama 2, Code Llama, and other models
5.0 / 5 (0 votes)