04 Correlation in SPSS – SPSS for Beginners
Summary
TLDRThis tutorial from the RStats Institute introduces the basics of correlation analysis in SPSS for beginners. It explains how to perform a Pearson's correlation to examine the relationship between two variables, such as height and weight, using a sample dataset. The video covers setting up data, interpreting the correlation matrix, and understanding significance levels. It also demonstrates how to include a third variable in the correlation, resulting in a point biserial correlation, and how to visualize correlations using a scatter plot with the Chart Builder tool. The tutorial concludes by highlighting the significance of correlation in statistical analysis and its applications in further statistical techniques like regression.
Takeaways
- 📚 The video is part of a series on SPSS for beginners by the RStats Institute at Missouri State University.
- 🔍 It focuses on teaching the basics of correlation analysis in SPSS, specifically Pearson's r, which measures the relationship between two variables.
- ⚖️ Pearson's r ranges from -1 to +1, with 0 indicating no relationship, and values closer to +1 or -1 indicating a stronger relationship.
- 📈 The example used in the video involves analyzing the relationship between height and weight, with each person's height paired with their weight.
- 🔎 The video demonstrates how to perform a correlation analysis in SPSS, including selecting the 'Bivariate' option and choosing the variables to correlate.
- 📊 The output of a correlation analysis in SPSS is a 'correlation matrix', which shows the correlation coefficients between variables.
- 🔑 The significance of the correlation is indicated by the p-value; if it's less than .05, the correlation is statistically significant.
- 👥 The video mentions that SPSS will ignore cases with missing values, which is important for understanding the sample size used in the analysis.
- 📊 The video also introduces the concept of a scatter plot as a visual representation of the correlation between two variables.
- 📝 The script suggests that viewers watch additional videos from the RStats Institute for more information on theory, analysis, and APA style reporting.
- 📈 Lastly, the video briefly mentions other statistical techniques such as point biserial correlation, regression, and t-tests for analyzing differences between variables.
Q & A
What is the main focus of the fourth video in the SPSS for beginners series?
-The main focus of the fourth video is to teach viewers how to perform simple analyses, specifically how to calculate and interpret Pearson's correlation in SPSS.
What is Pearson's r and what does it measure?
-Pearson's r is a statistical measure that describes the relationship between two variables. It ranges between -1 and +1, where 0 indicates no relationship, and values closer to +1 or -1 indicate a stronger relationship.
Why is it important that each person's height is paired with their own weight in the dataset?
-It is important because the analysis requires each pair of scores to stay together to make sense. Pairing one person's height with another person's weight would not be meaningful in the context of the analysis.
How does SPSS handle cases with missing values during a correlation analysis?
-SPSS will simply ignore cases with missing values. It does not include them in the analysis, ensuring that only complete pairs of scores are considered.
What is the significance of the correlation coefficient being flagged with asterisks in SPSS?
-Asterisks indicate that the correlation is statistically significant, meaning it is significantly different from 0. The specific significance level is denoted by the number of asterisks.
What is a 'correlation matrix' and what does it show?
-A correlation matrix is a table that shows the correlation coefficients for every combination of variables. It includes both the correlation between different variables and the perfect correlation of a variable with itself.
What is the significance level mentioned in the script, and what does a level smaller than .05 indicate?
-The significance level is a threshold used to determine if a result is statistically significant. A level smaller than .05 indicates that the correlation is statistically significant, meaning it is unlikely to have occurred by chance.
What is a point biserial correlation and when is it used?
-A point biserial correlation is a type of correlation used when one variable is continuous and the other is a dichotomous nominal variable. It is used in the script to correlate height, weight, and gender.
What does the scatter plot represent and how is it created in SPSS?
-A scatter plot represents the relationship between two variables, with each point corresponding to an individual's scores on the two variables. In SPSS, it is created using the Chart Builder tool by dragging variables to the x and y-axis drop zones.
What other statistical techniques are mentioned in the script that could be used to explore relationships between variables further?
-The script mentions other techniques such as simple regression and multiple regression for predicting variables, as well as t-tests for examining differences between variables.
Outlines
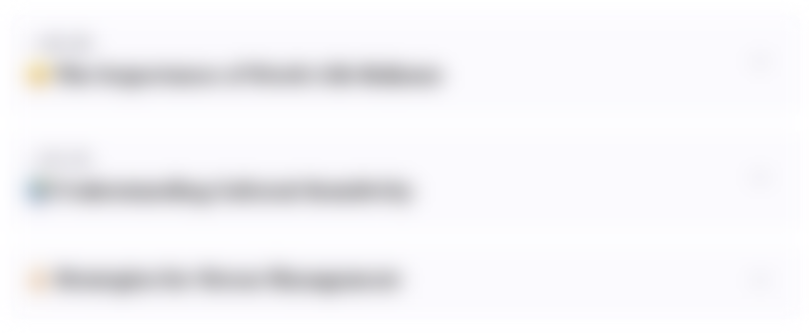
This section is available to paid users only. Please upgrade to access this part.
Upgrade NowMindmap
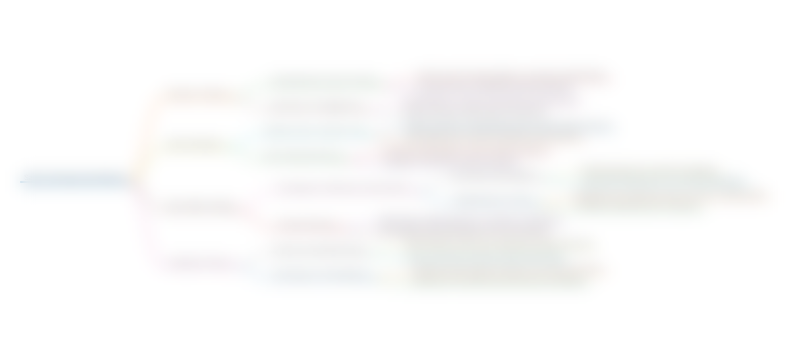
This section is available to paid users only. Please upgrade to access this part.
Upgrade NowKeywords
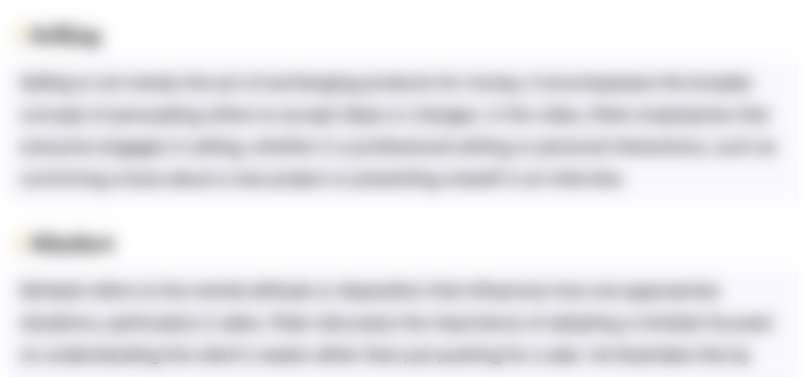
This section is available to paid users only. Please upgrade to access this part.
Upgrade NowHighlights
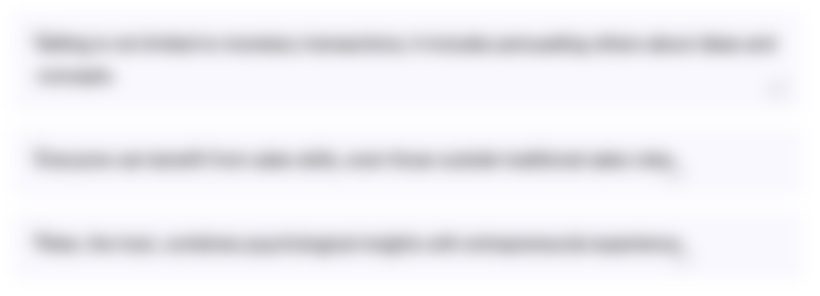
This section is available to paid users only. Please upgrade to access this part.
Upgrade NowTranscripts
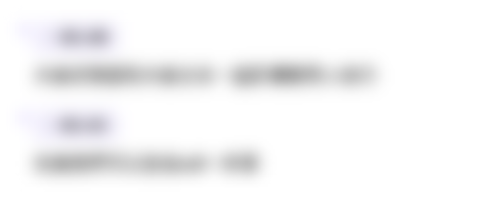
This section is available to paid users only. Please upgrade to access this part.
Upgrade NowBrowse More Related Video
5.0 / 5 (0 votes)