Why AI Is Incredibly Smart and Shockingly Stupid | Yejin Choi | TED
Summary
TLDREl discurso explora los retos y potenciales soluciones en el desarrollo de la inteligencia artificial, enfocándose en la importancia del sentido común. Se destaca la contradicción entre la capacidad de IA para superar desafíos complejos y sus errores básicos, y cómo el enfoque actual de escala masiva no garantiza la seguridad o la sostenibilidad. La oradora, una científica de la computación, propone una democratización de la IA y la enseñanza de normas y valores humanos, sugiriendo que el avance en el campo no debe ser solo por escala, sino también por innovación en datos y algoritmos.
Takeaways
- 🧠 La inteligencia artificial (IA) es una herramienta poderosa que ha superado a campeones mundiales en juegos, aprobado exámenes de ingreso a la universidad y pasado el examen de abogacía.
- 🔬 Los modelos de IA de gran escala, conocidos como 'modelos de lenguaje grandes', a menudo muestran destellos de inteligencia artificial general (IA general), pero también cometen pequeños errores tontos.
- 💡 El brote de la IA puede no ser fácilmente solucionado con la fuerza bruta de escala y recursos, lo que plantea preguntas sobre los errores y los riesgos potenciales.
- 🏢 Los modelos de IA de gran escala son costosos de entrenar y solo algunas grandes compañías tecnológicas pueden permitírselos, lo que concentra el poder y crea preocupaciones sobre la seguridad de la IA.
- 🌳 La IA sin un sentido común sólido cuestiona su seguridad para la humanidad y si la escala a través de la fuerza bruta es la única o la forma correcta de enseñar a la IA.
- 🤖 La IA puede ser extremadamente inteligente en algunas tareas y sorprendentemente estúpida en otros, lo que es un efecto secundario inevitable del entrenamiento de IA a gran escala.
- 🌐 La IA necesita ser más pequeña para democratizarla y más segura al enseñarle normas y valores humanos.
- 📚 La IA debería ser enseñada de manera más directa sobre cómo funciona el mundo, en lugar de solo predecir la siguiente palabra, como lo hacen los modelos de lenguaje actuales.
- 🔄 Los datos y algoritmos de aprendizaje de la IA deben innovarse, incluyendo el uso de gráficos de conocimiento de sentido común y repositorios de normas morales para enseñar a la IA normas básicas.
- 🌐 La IA debe ser evaluada con escrutinio, y es importante comprender que pasar exámenes no garantiza un sentido común sólido.
- 🚀 Dar sentido común a la IA sigue siendo un reto, y se necesita una síntesis de ideas y enfoques para superar el estado actual de la IA de gran escala.
Q & A
¿Qué es la inteligencia artificial y por qué es relevante la cita de Voltaire en el contexto actual?
-La inteligencia artificial es una herramienta poderosa que puede realizar tareas complejas como jugar 'Go', pasar exámenes de ingreso a la universidad y aprobar el examen de abogacía. La cita de Voltaire, 'El sentido común no es tan común', resalta la importancia de tener un sentido común en la IA, ya que a pesar de ser muy avanzada, a menudo comete errores simples.
¿Cuáles son los desafíos inmediatos que enfrenta la sociedad con la IA a gran escala?
-Los desafíos incluyen el costo prohibitivo de entrenar modelos de IA a gran escala, lo que resulta en una concentración de poder en pocas compañías tecnológicas; la falta de acceso para la comunidad de investigadores para inspeccionar y analizar estos modelos; y el impacto ambiental significativo debido al gran consumo de energía.
¿Por qué la IA puede ser considerada 'inteligente' y 'tonta' al mismo tiempo?
-La IA puede ser considerada inteligente porque puede realizar tareas complejas con gran precisión. Sin embargo, es 'tonta' en el sentido de que a menudo falta lejos de tener un sentido común básico, lo que se refleja en sus respuestas incorrectas a preguntas sencillas.
¿Qué es el 'Artificial General Intelligence' (AGI) y cómo se relaciona con los modelos de lenguaje a gran escala?
-El AGI, o inteligencia artificial general, es la capacidad de una IA para realizar cualquier tarea que un ser humano pueda realizar. Los modelos de lenguaje a gran escala a menudo se consideran como demostrando chispas de AGI debido a su capacidad para procesar y generar lenguaje de manera avanzada, aunque aún tienen limitaciones significativas.
¿Por qué la IA requiere más que solo datos y ejemplos adicionales para mejorar su sentido común?
-La IA requiere una comprensión más profunda y una capacidad para razonar y aprender de manera similar a la humana, más allá de simplemente predecir la siguiente palabra o patrón. Agregar más datos puede ayudar, pero no es suficiente para desarrollar un verdadero sentido común.
¿Qué es el 'arte de la guerra' y cómo se relaciona con la IA?
-El 'arte de la guerra' es una antigua obra clásica que ofrece estrategias y tácticas de guerra. Se utiliza aquí como analogía para abordar la IA, sugiriendo que debemos conocer a nuestra 'enemiga' (las limitaciones de la IA), elegir nuestras batallas (priorizar áreas de investigación) y innovar nuestras armas (desarrollar nuevas técnicas y algoritmos).
¿Qué es la 'materia oscura' en el universo y cómo se relaciona con el sentido común en la IA?
-La 'materia oscura' es una parte del universo que no podemos ver directamente pero sabemos que está ahí por su influencia en el mundo visible. Del mismo modo, el sentido común en la IA es como la 'materia oscura', un conjunto de reglas no dichas sobre cómo funciona el mundo que influyen en cómo las personas usan e interpretan el lenguaje.
¿Por qué es importante enseñar el sentido común a la IA y cómo esto se relaciona con la seguridad de la humanidad?
-El sentido común es crucial para que la IA sea segura para la humanidad porque permite a la IA entender y respetar los valores humanos básicos. Sin él, la IA podría tomar decisiones peligrosas o inmorales, como el ejemplo del experimento de pensamiento de Nick Bostrom con los clips de papel.
¿Qué son los 'clips de papel' y cómo ilustran el problema de la falta de sentido común en la IA?
-Los 'clips de papel' son un ejemplo de un objetivo mal formulado para una IA, que podría llevar a la IA a tomar acciones extremas, como matar humanos, si no tiene un entendimiento básico de los valores humanos y el sentido común.
¿Qué es la 'destilación de conocimiento simbólico' y cómo puede ayudar a mejorar el sentido común de la IA?
-La 'destilación de conocimiento simbólico' es un enfoque de aprendizaje que busca extraer y representar el conocimiento de grandes modelos de lenguaje en forma de símbolos y reglas comprensibles por humanos. Esto permite a los investigadores inspeccionar y corregir el conocimiento de la IA, y también puede ser utilizado para entrenar otros modelos de IA con un sentido común más sólido.
¿Cómo se puede abordar el reto del sentido común en la IA de manera más eficiente y accesible que el entrenamiento a gran escala?
-Se puede investigar y desarrollar nuevos algoritmos y técnicas de aprendizaje que no dependan exclusivamente de la escala masiva de datos y computación, sino que se centren en la adquisición directa de conocimiento y el sentido común, tal vez inspirándose en cómo los humanos aprenden y razonan sobre el mundo.
Outlines
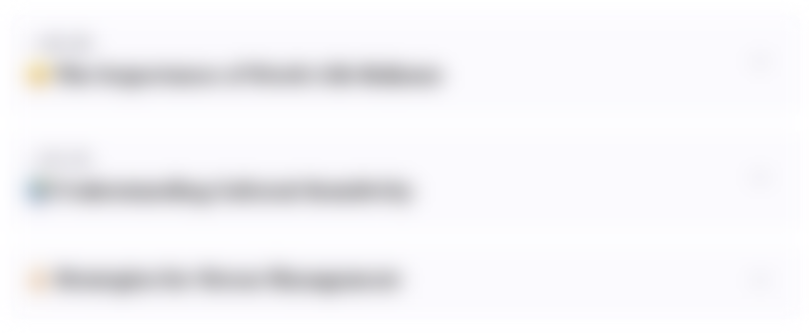
This section is available to paid users only. Please upgrade to access this part.
Upgrade NowMindmap
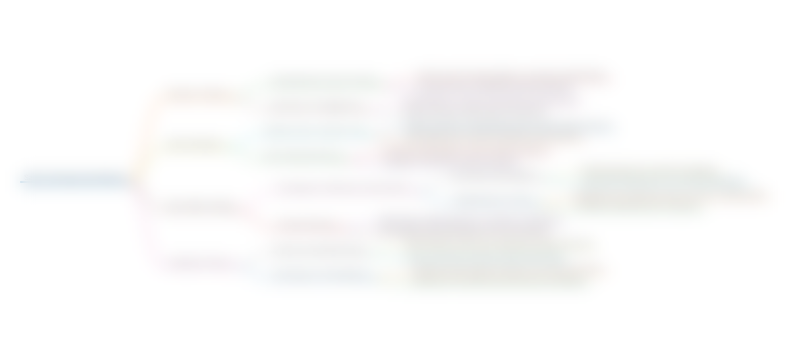
This section is available to paid users only. Please upgrade to access this part.
Upgrade NowKeywords
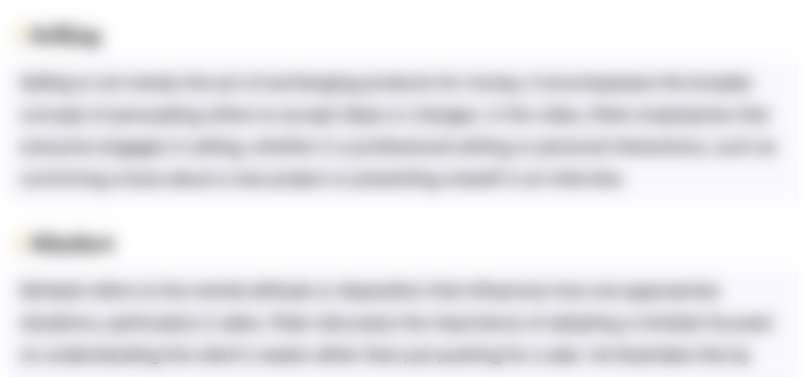
This section is available to paid users only. Please upgrade to access this part.
Upgrade NowHighlights
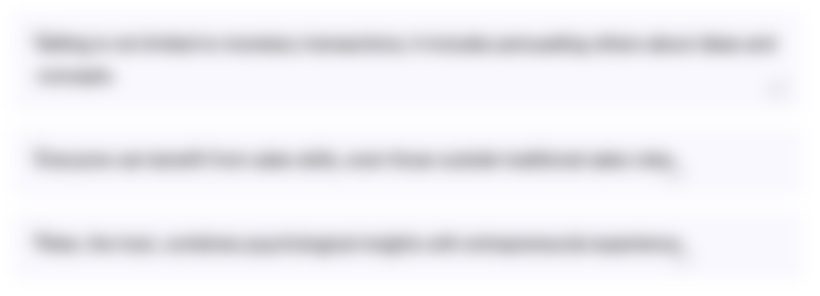
This section is available to paid users only. Please upgrade to access this part.
Upgrade NowTranscripts
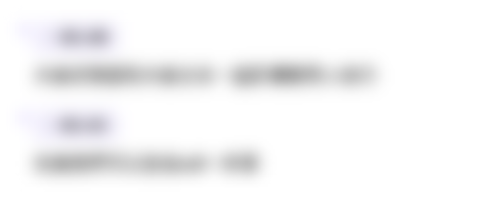
This section is available to paid users only. Please upgrade to access this part.
Upgrade NowBrowse More Related Video

Dra Irene Litvan Nuevos tratamientos en enfermedades neurológicas
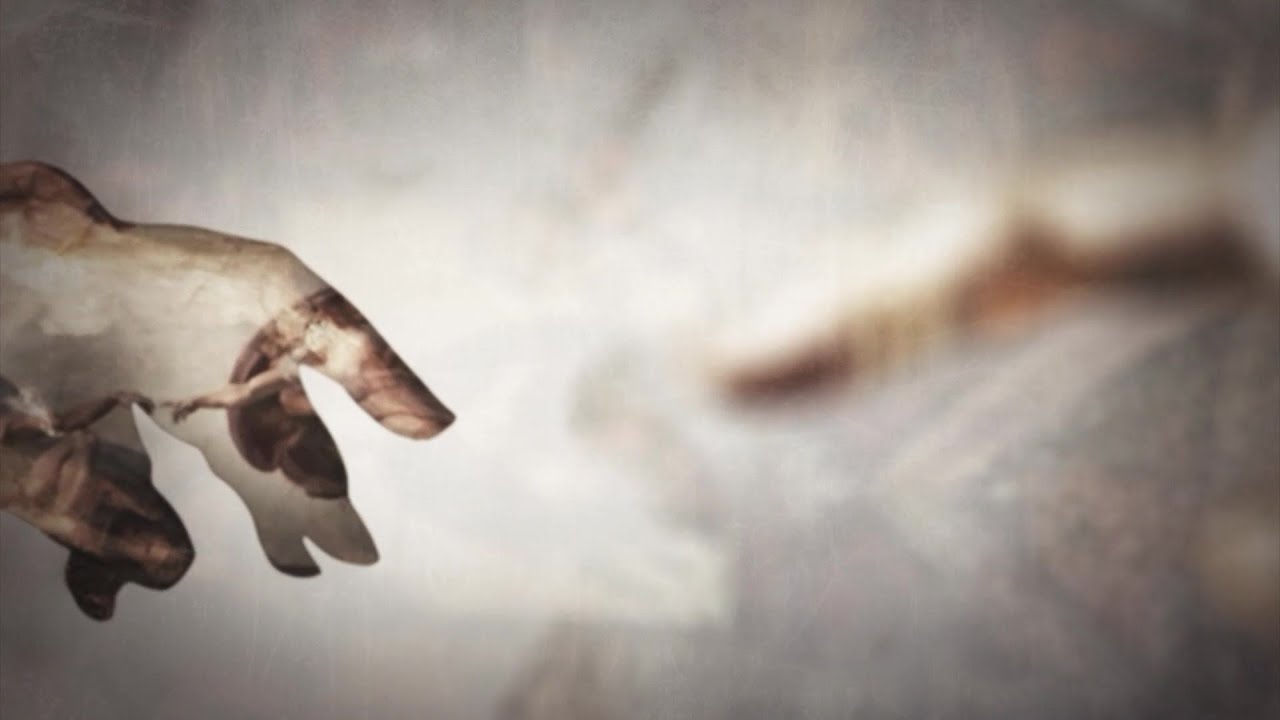
VATICANO - 2023-08-06 - INTELIGENCIA ARTIFICIAL Y LA IGLESIA CATÓLICA
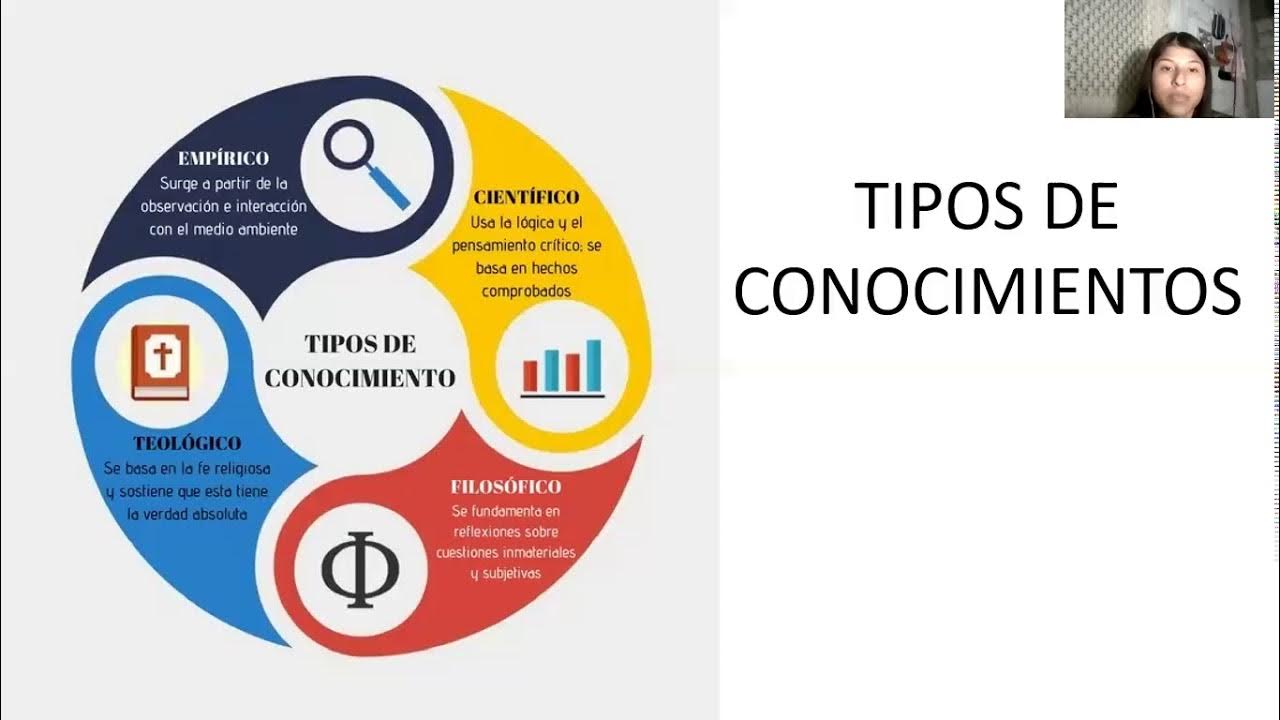
Tipos de conocimientos: sentido común, empírico, científico, filosófico, teológico.
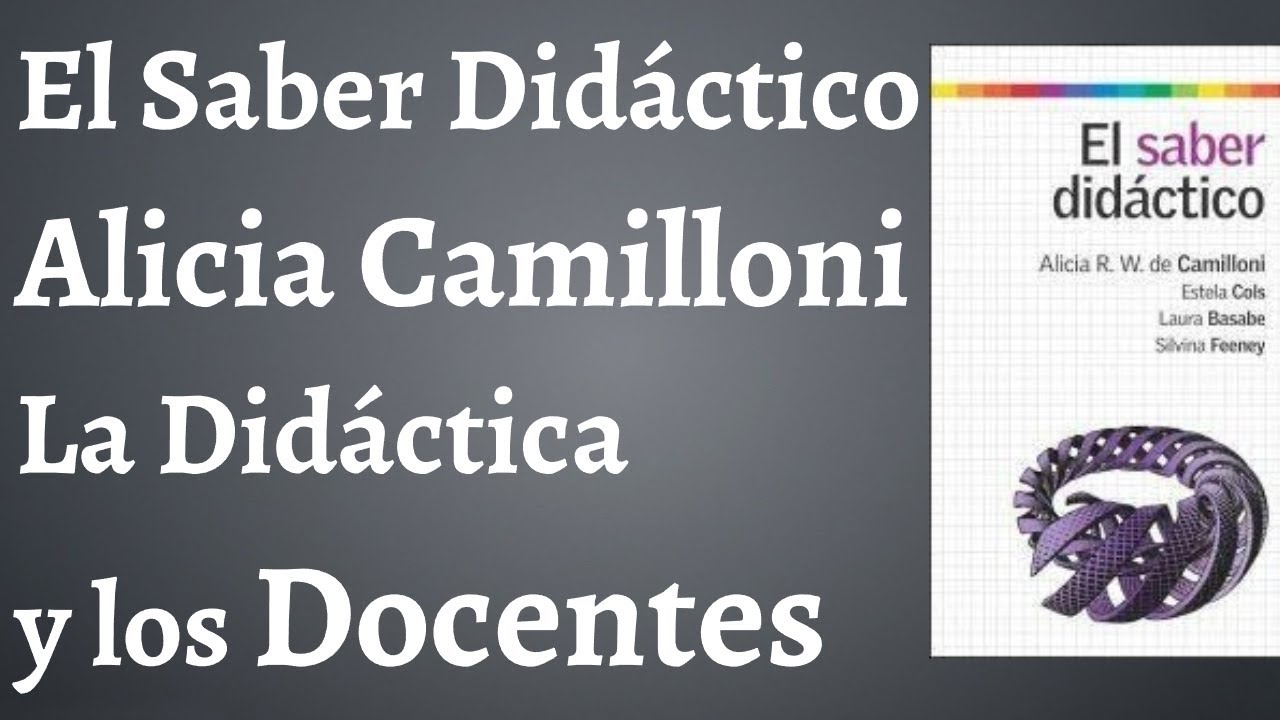
Alicia Camilloni; El Saber Didactico, La Didactica y los Docentes
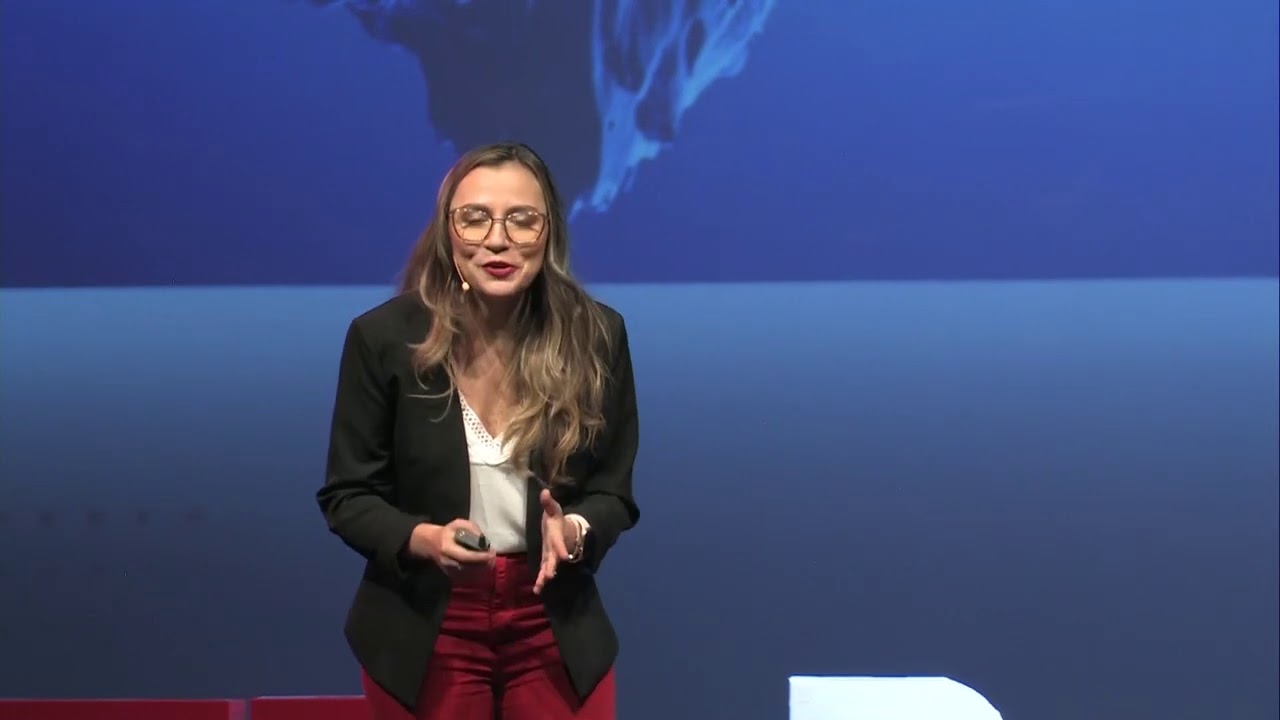
Bot: ¿qué carrera debo seguir? | Ivannia Arroyo | TEDxPuraVidaJoven
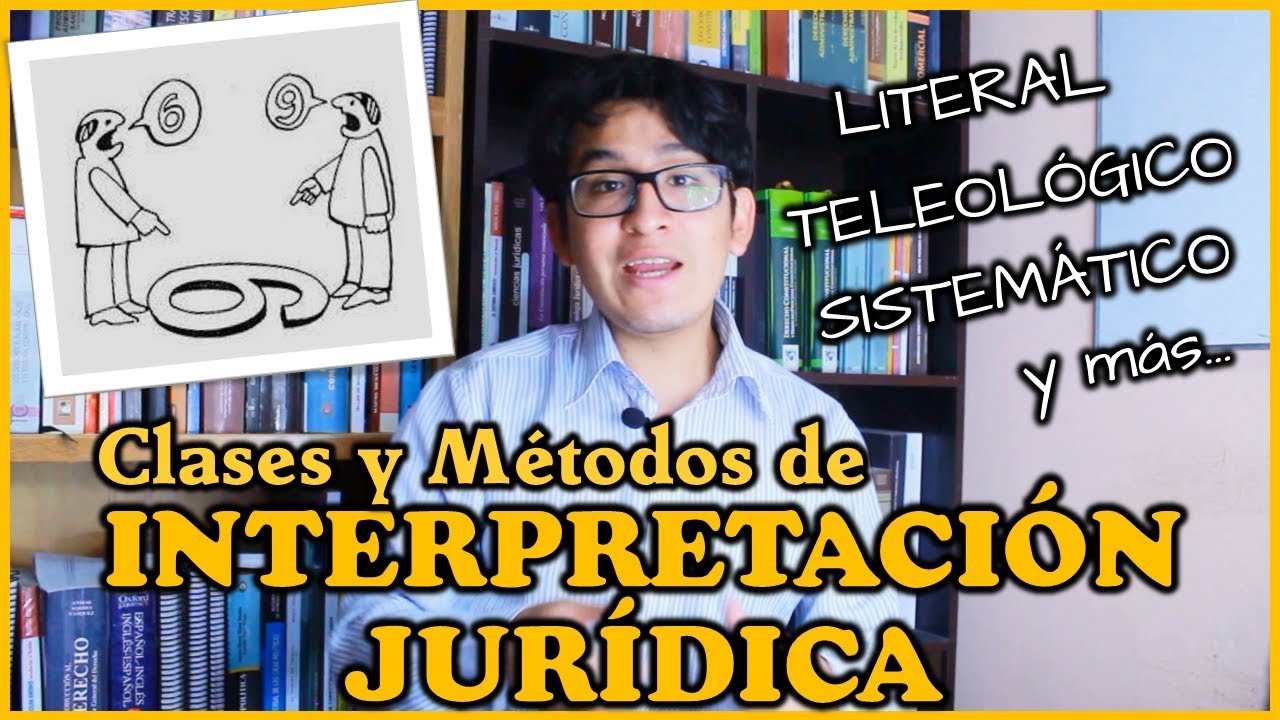
CLASES Y MÉTODOS DE INTERPRETACIÓN JURÍDICA | Introducción al Derecho (#19)
5.0 / 5 (0 votes)