The future of AI in medicine | Conor Judge | TEDxGalway
Summary
TLDRThe speaker reflects on his journey from a young boy with a stammer in a drama competition to a medical consultant and lecturer, emphasizing the importance of data in solving medical mysteries. He introduces multimodal AI, which processes diverse data types, as a potential solution to healthcare inefficiencies exacerbated by technology. Highlighting examples of AI in healthcare, he discusses the need for trust, explainability, and clinical trials in implementing AI safely, advocating for a compassionate approach that integrates AI with human expertise.
Takeaways
- ๐ญ The speaker's connection to the stage began 26 years ago as a 12-year-old in a drama competition, playing a detective in a play about a hotel robbery.
- ๐ต๏ธโโ๏ธ The role of a detective in the play mirrors the speaker's current role as a medical consultant, both involving the collection of information to solve a mystery.
- โฑ๏ธ The speaker spends 70% of their time collecting information and 30% making decisions, a ratio that has been exacerbated by technology in the healthcare field.
- ๐ผ The introduction of electronic health records, designed for billing rather than efficiency, has increased administrative workload for doctors, reducing patient interaction time.
- ๐ค The speaker introduces the concept of multimodal AI, which processes various types of data, as a potential solution to the information overload in healthcare.
- ๐จโโ๏ธ The speaker differentiates between single-model AI, like machine learning and computer vision, and multimodal AI, which can process text, images, and numbers.
- ๐ฅ Examples of single-model AI in healthcare include 'Chest Link' for X-ray triage, an AI model for diagnosing eye diseases and predicting outcomes, and another for predicting Parkinson's disease from retinal images.
- ๐ฃ๏ธ Google's 'Med-PaM' is a large language model that has passed the US medical licensing exam, demonstrating the potential of AI in medical question answering.
- ๐ฒ The multimodal version of 'Chat GPT' and 'Med-PaM M' can analyze medical images and text, providing insights that sometimes surpass human radiologists' reports.
- ๐ก๏ธ For multimodal AI to be implemented safely, trust, explainability, and randomized clinical trials are essential to ensure its effectiveness and reliability.
- ๐ค The future of healthcare with AI should prioritize compassion and understanding, allowing doctors to spend more time with patients and improving health outcomes.
Q & A
What was the speaker's first experience on stage 26 years ago?
-The speaker's first experience on stage was at the town hall theater, participating in a drama competition for local schools where he played a detective in a play written by his best friend.
How does the speaker compare his role as a detective to his current profession?
-The speaker compares his role as a detective solving the mystery of who robbed a hotel to his current profession as a medical consultant and lecturer, where he solves medical mysteries by collecting and analyzing patient data.
What is the 70/30 imbalance the speaker refers to in healthcare delivery?
-The 70/30 imbalance refers to the speaker spending 70% of his time collecting information about patients and only 30% making decisions and communicating with them. This imbalance is common in healthcare and exacerbated by technology.
What is the main idea the speaker wants to share about artificial intelligence (AI) in healthcare?
-The speaker wants to introduce the responsible use of medical AI, specifically multimodal AI, as a potential solution to improve healthcare efficiency and reduce administrative workloads, allowing more time for patient care.
What is multimodal AI and how does it differ from single model AI?
-Multimodal AI can process and integrate different types of data such as text, images, and numbers, similar to how a doctor gathers various types of information from a patient. Single model AI, on the other hand, usually processes only one type of data at a time.
What are some examples of single model AI in healthcare mentioned by the speaker?
-Examples include OxyPitโs chest link for triaging chest x-rays and an AI model from University College London that diagnoses eye diseases and predicts conditions like Parkinson's disease from retinal images.
What is the significance of the Med-PaLM medical AI model mentioned by the speaker?
-Med-PaLM is a large language model trained to perform medical question answering, and it is notable for being the first AI to pass the US medical licensing exam with a passing score, demonstrating its potential in assisting medical professionals.
How does the speaker envision the future use of multimodal AI in healthcare?
-The speaker envisions multimodal AI being used to enhance healthcare delivery, especially in remote and underserved areas, by integrating various types of patient data to provide insights and support for medical professionals.
What are the key factors necessary for implementing multimodal AI safely in healthcare?
-The key factors are trust, explainability, and randomized clinical trials. Trust involves patients feeling comfortable with AI in their care, explainability ensures understanding of AI decisions, and clinical trials test AI models like medicines to ensure effectiveness and safety.
What is the 'eyeball test' and why is it important according to the speaker?
-The 'eyeball test' refers to the initial visual assessment of a patient by a medical professional. It's important because it provides context to diagnostic results and has been shown to be highly accurate in emergency settings. The speaker emphasizes that even with AI, the human element remains crucial.
Outlines
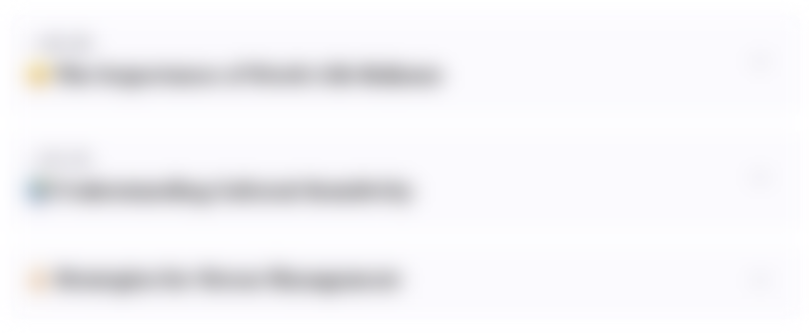
This section is available to paid users only. Please upgrade to access this part.
Upgrade NowMindmap
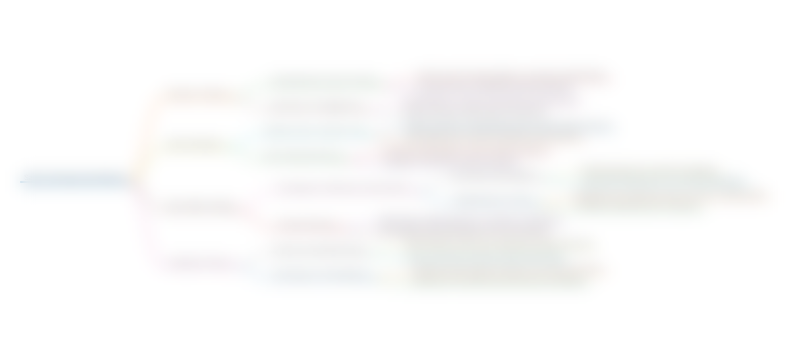
This section is available to paid users only. Please upgrade to access this part.
Upgrade NowKeywords
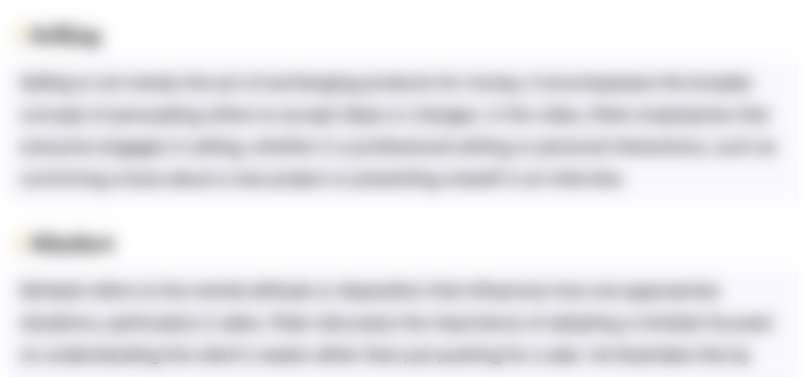
This section is available to paid users only. Please upgrade to access this part.
Upgrade NowHighlights
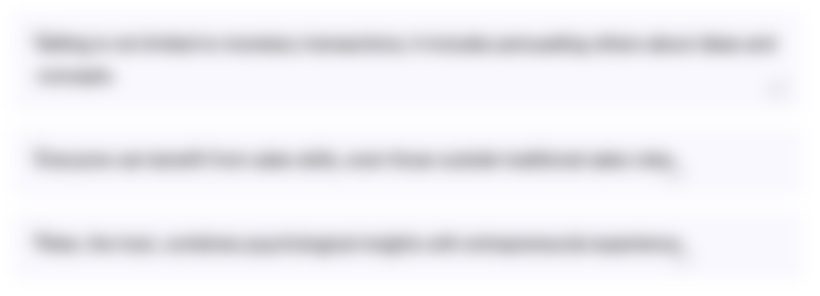
This section is available to paid users only. Please upgrade to access this part.
Upgrade NowTranscripts
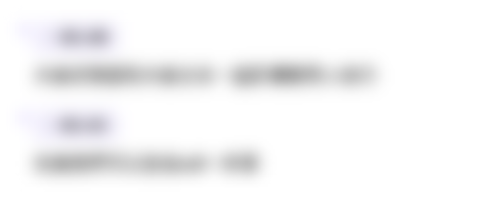
This section is available to paid users only. Please upgrade to access this part.
Upgrade NowBrowse More Related Video
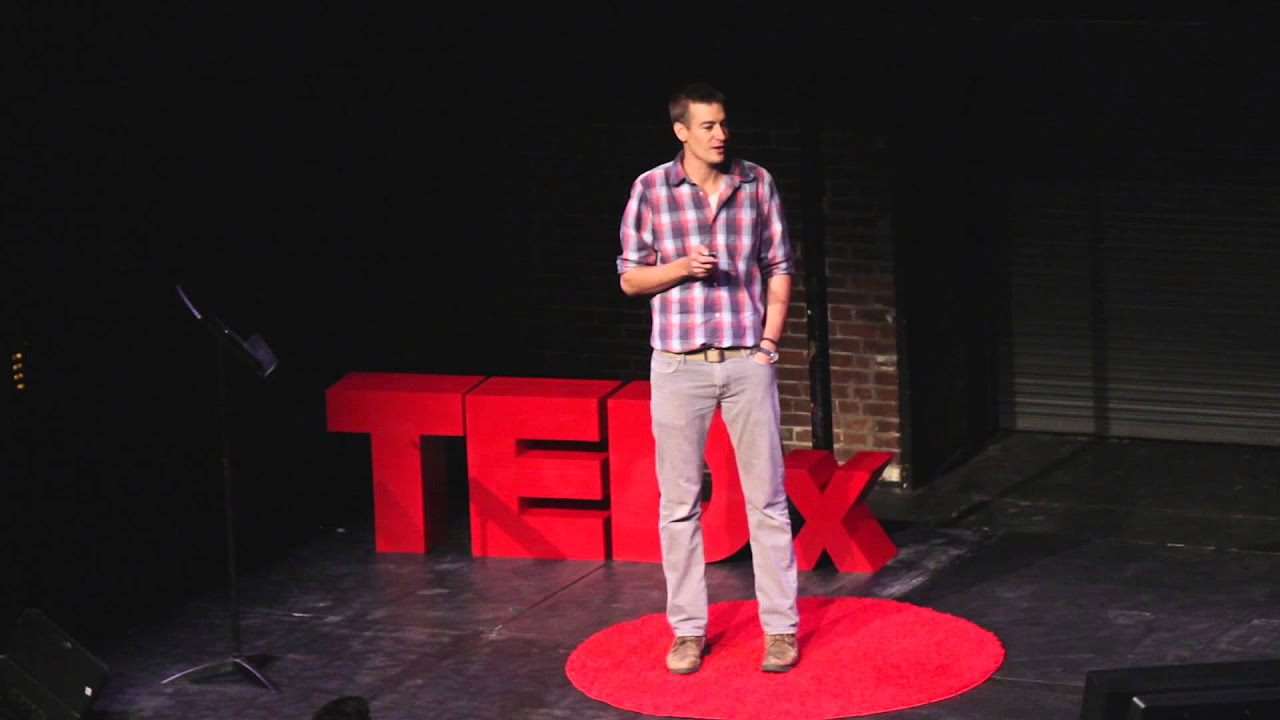
Why poverty has nothing to do with money: Ian Rosenberger at TEDxGrandviewAve
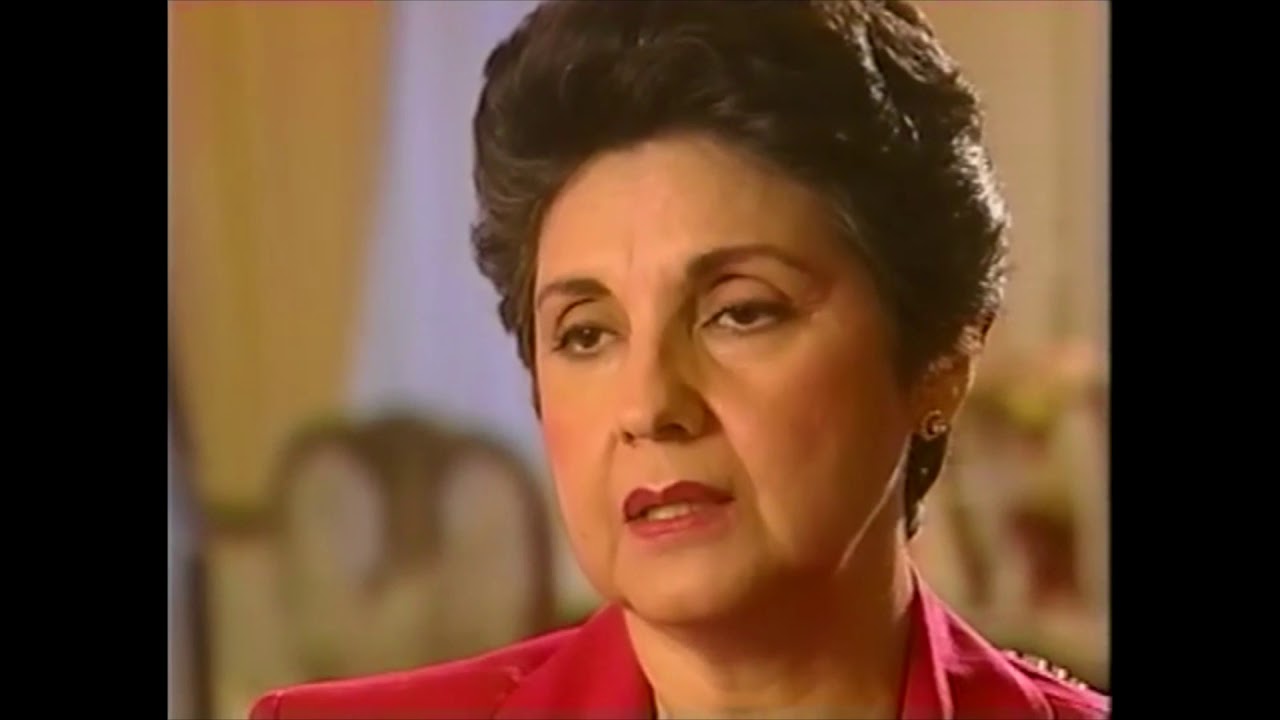
David Vetter Clip
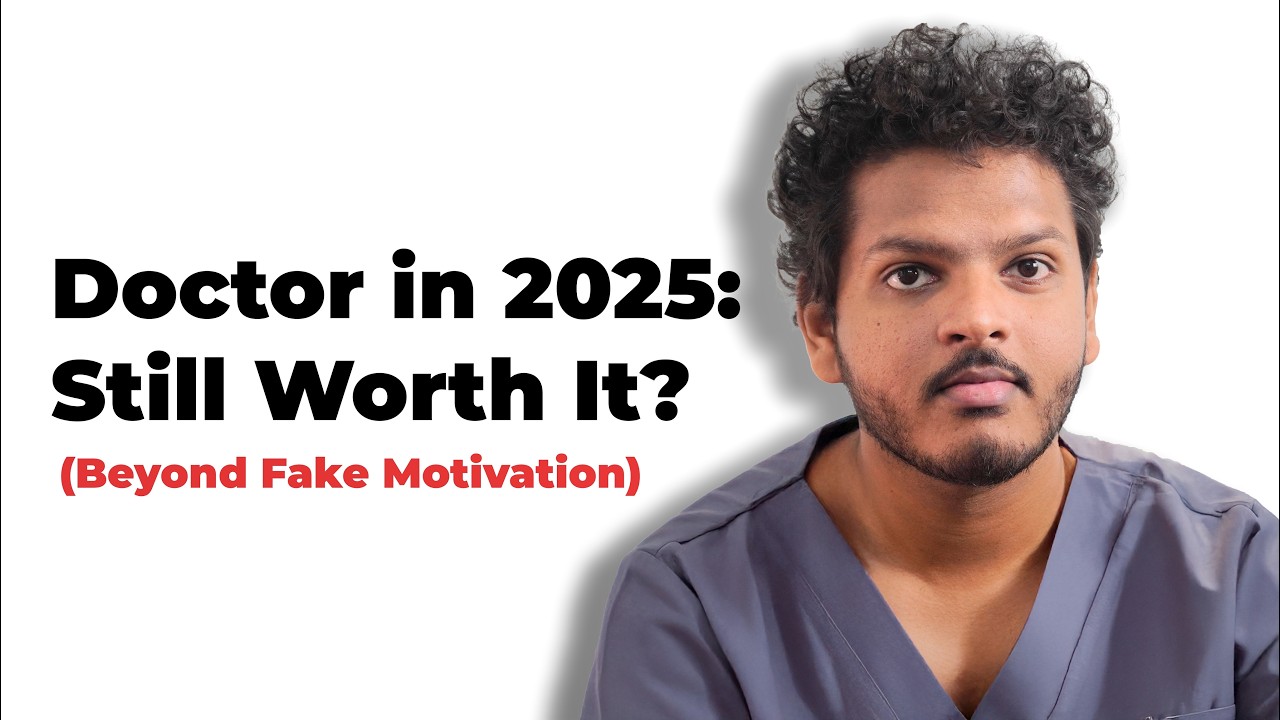
The Harsh Truth of Being a Doctor in India | Dr. Anuj Pachhel
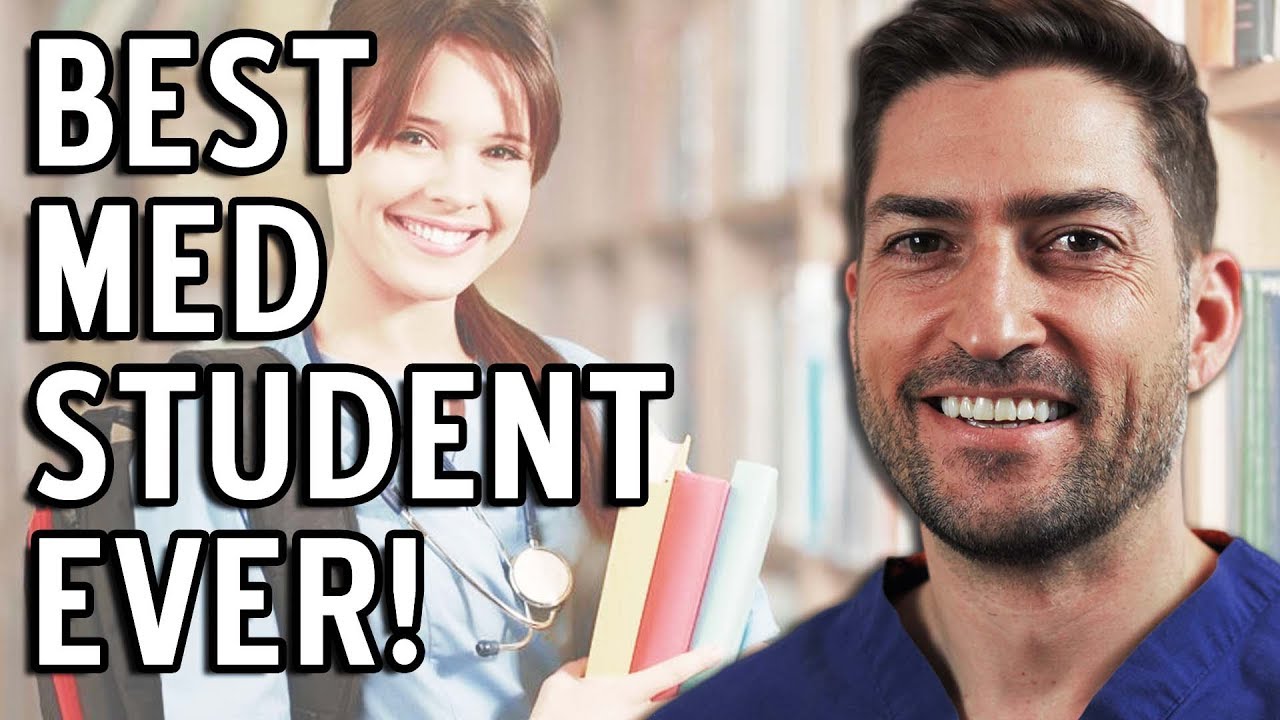
How to be the BEST MEDICAL STUDENT EVER!
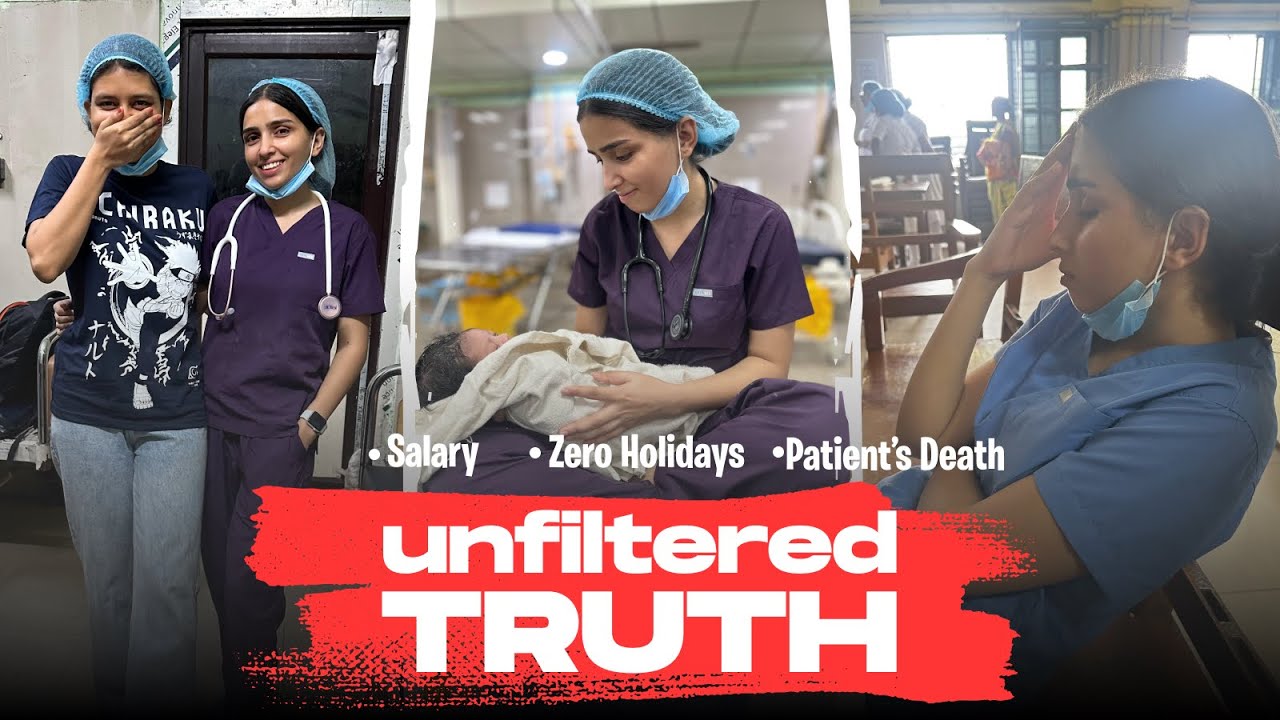
My Unfiltered experience as a Doctor-6 Months, No Sugarcoating.
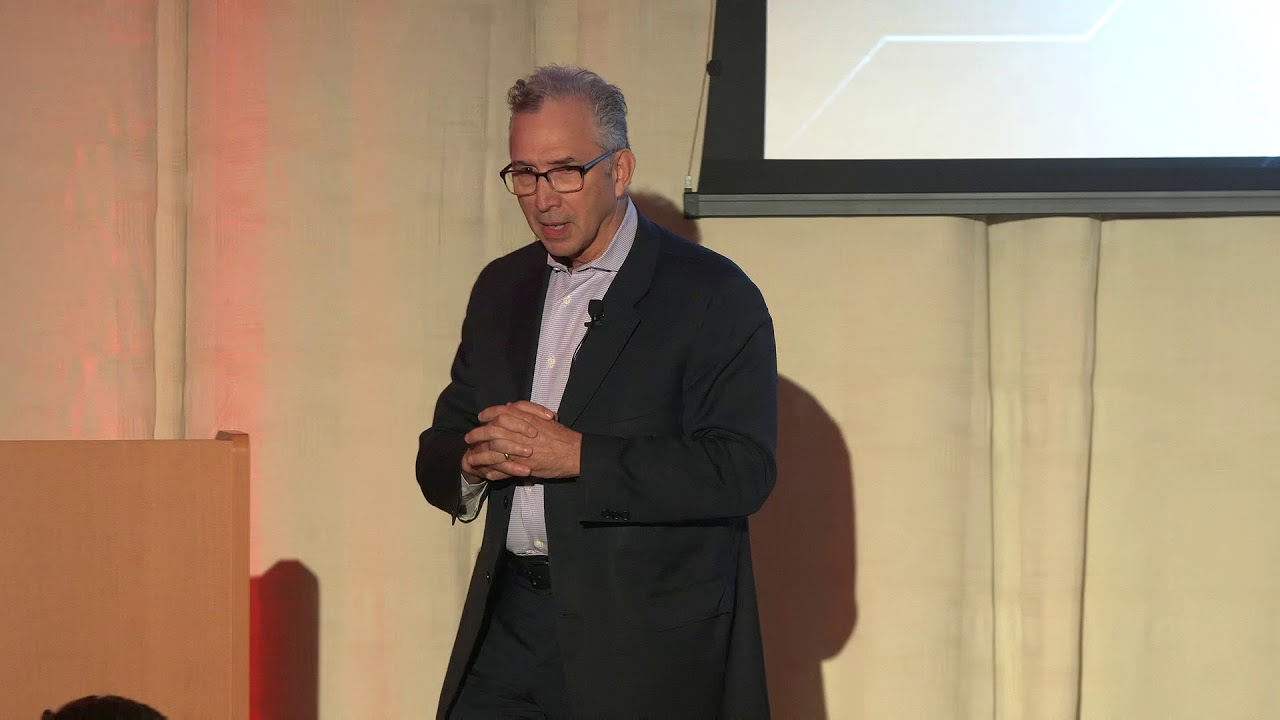
If You Are Going to be Bear, be a Grizzly | David Ansell | TEDxRushU
5.0 / 5 (0 votes)