Ilya Sutskever | This will all happen next year | I totally believe | AI is come
Summary
TLDR这段视频剧本探讨了多模态学习的重要性及其对神经网络发展的影响。多模态学习不仅增强了神经网络的视觉理解能力,也通过图像而非仅文本来更全面地了解世界。讨论了人类一生中接收到的词汇量有限,因此需要通过视觉等多种信息源来丰富知识。此外,还提到了AI生成测试以训练其他AI的潜力,以及未来AI自我提升的可能性。最后,讨论了大型语言模型的可靠性和未来发展,强调了提高系统可信度和遵循用户意图的重要性。
Takeaways
- 📈 多模态性对于神经网络尤其有用,因为世界是非常视觉化的,人类是视觉动物,大约有三分之一的视觉皮层致力于视觉。
- 🧠 人类一生中大约只能听到十亿个单词,这强调了从视觉等其他信息源学习的重要性。
- 🌐 神经网络可以从文本中学习世界信息,即使没有直接的视觉输入,也能了解颜色等概念。
- 🔍 通过视觉学习,我们可以了解世界构造、物理和动画,而音频可以为模型学习提供额外的信息源。
- 📊 在数学竞赛问题中,视觉输入显著提高了神经网络解决问题的准确率。
- 🤖 神经网络通过视觉和文本学习,能够进行视觉推理和沟通,未来可能通过图像而非文字来解释问题。
- 🔑 未来语言模型的发展将集中在提高系统的可靠性和信任度,确保其输出的准确性和完整性。
- 🔄 神经网络可能会通过生成自己的数据来训练自己,类似于人类通过自我反思和睡眠中的大脑活动来学习。
- 🚀 GPT-4在可靠性、解决数学问题的能力以及遵循指令方面表现出色,特别是在视觉方面解释笑话和模因的能力。
- 🎯 神经网络的发展证明了早期关于人工神经元和学习算法的概念是正确的,这是过去20年中最大的惊喜。
- 🌟 神经网络的训练和数据量在过去10年里增长了一百万倍,这是计算机科学领域难以置信的成就。
Q & A
多模态在神经网络中的重要性是什么?
-多模态对于神经网络非常重要,因为它增加了视觉信息的输入,使网络能够更好地理解和解释世界。人类是非常视觉化的生物,大脑中约有三分之一的皮层专门用于视觉处理,因此多模态可以显著提升神经网络的实用性。
为什么说通过图像学习可以让我们更深入地了解世界?
-通过图像学习,我们不仅可以从文本中获取信息,还能从视觉信息中获得额外的知识。例如,颜色的相似性,即使没有直接的视觉经验,文本信息也能间接地告诉我们红色与橙色比与蓝色更相似。
人类一生中大约能听到多少单词?
-人类一生中大约能听到十亿个单词。这个数字可能看起来很多,但实际上并不大,因为十亿秒大约是30年,而我们每天有一半的时间在睡觉,所以每秒听到的单词数量并不多。
为什么说神经网络可以从大量文本中学习到世界的知识?
-尽管神经网络可能从未直接看到过任何东西,但它们可以从大量的文本数据中学习到关于世界的知识。这是因为文本中包含了关于世界的间接信息,即使这些信息不是直接的视觉信息。
为什么说多模态学习可以提高神经网络的实用性?
-多模态学习可以提高神经网络的实用性,因为它允许网络从多种信息源中学习,而不仅仅是文本。例如,通过视觉信息,神经网络可以学习到颜色、形状和物体之间的关系等概念。
为什么说音频信息对于神经网络的学习也是有用的?
-音频信息是另一种信息源,它可以帮助神经网络理解语言的情感和语境,比如区分讽刺和热情的语气。虽然音频可能不如图像或视频信息那么丰富,但它仍然是一个有价值的补充信息源。
GPT-3和GPT-4在处理数学问题时的表现有何不同?
-GPT-4在处理数学问题时的表现显著优于GPT-3。例如,在AMC2数学竞赛中,GPT-4在添加视觉信息后,解决问题的成功率从2%提高到了40%。这说明视觉信息对于提高神经网络解决问题的能力至关重要。
为什么说神经网络的可靠性是未来研究的重要方向?
-神经网络的可靠性是指它们能够被信任并准确无误地完成任务的能力。如果神经网络能够可靠地识别重要信息并遵循用户的意图,那么它们的实用性将大大提高。
GPT-4在哪些方面表现出了令人惊讶的技能?
-GPT-4在多个方面表现出了令人惊讶的技能,包括解决复杂的数学问题、创作诗歌、解释笑话和模因,以及在视觉方面对复杂图像和图表的解释能力。
为什么说神经网络的自我生成数据可能是未来训练AI的重要部分?
-自我生成数据可以让AI在没有新外部数据的情况下继续学习和进步。类似于人类通过自我反思和思考问题来训练大脑,AI也可以通过生成对抗性内容或解决新问题来提高自身能力。
未来一两年内,大型语言模型的哪些领域可能会有显著进步?
-在未来一两年内,我们可以期待大型语言模型在可靠性和用户意图理解方面取得显著进步,这将使技术更加值得信赖,并能够应用于更多领域。
Outlines
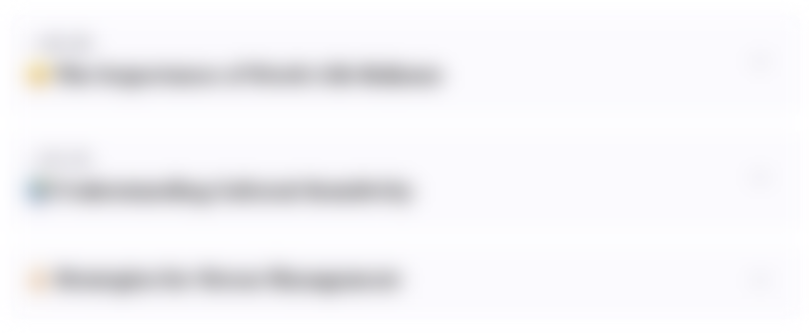
This section is available to paid users only. Please upgrade to access this part.
Upgrade NowMindmap
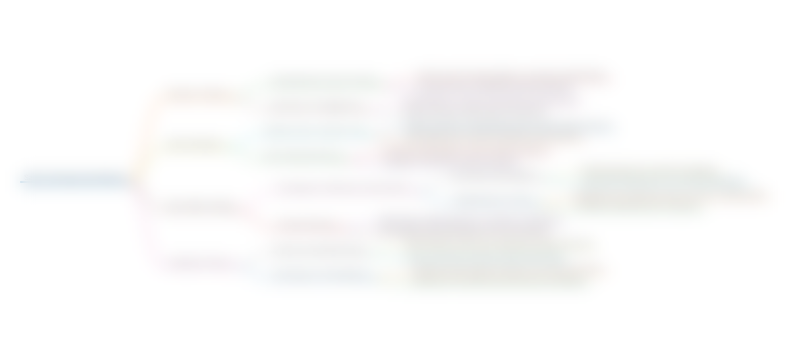
This section is available to paid users only. Please upgrade to access this part.
Upgrade NowKeywords
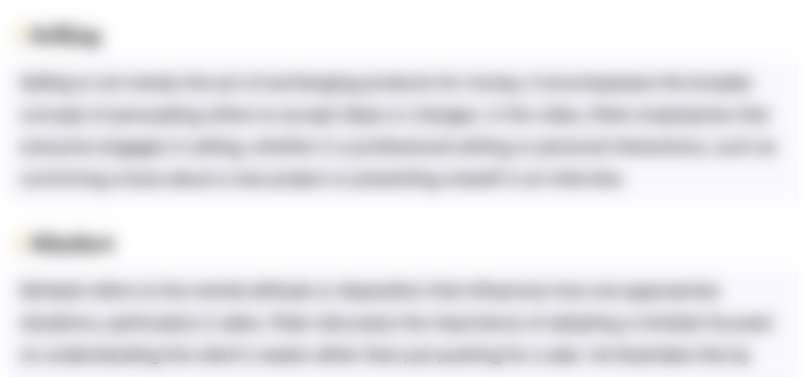
This section is available to paid users only. Please upgrade to access this part.
Upgrade NowHighlights
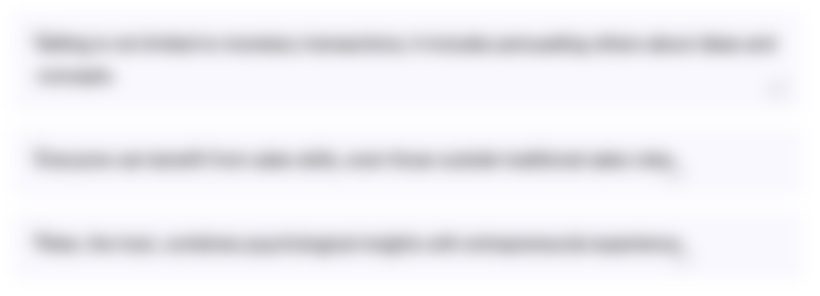
This section is available to paid users only. Please upgrade to access this part.
Upgrade NowTranscripts
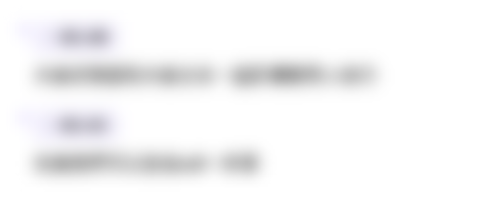
This section is available to paid users only. Please upgrade to access this part.
Upgrade NowBrowse More Related Video
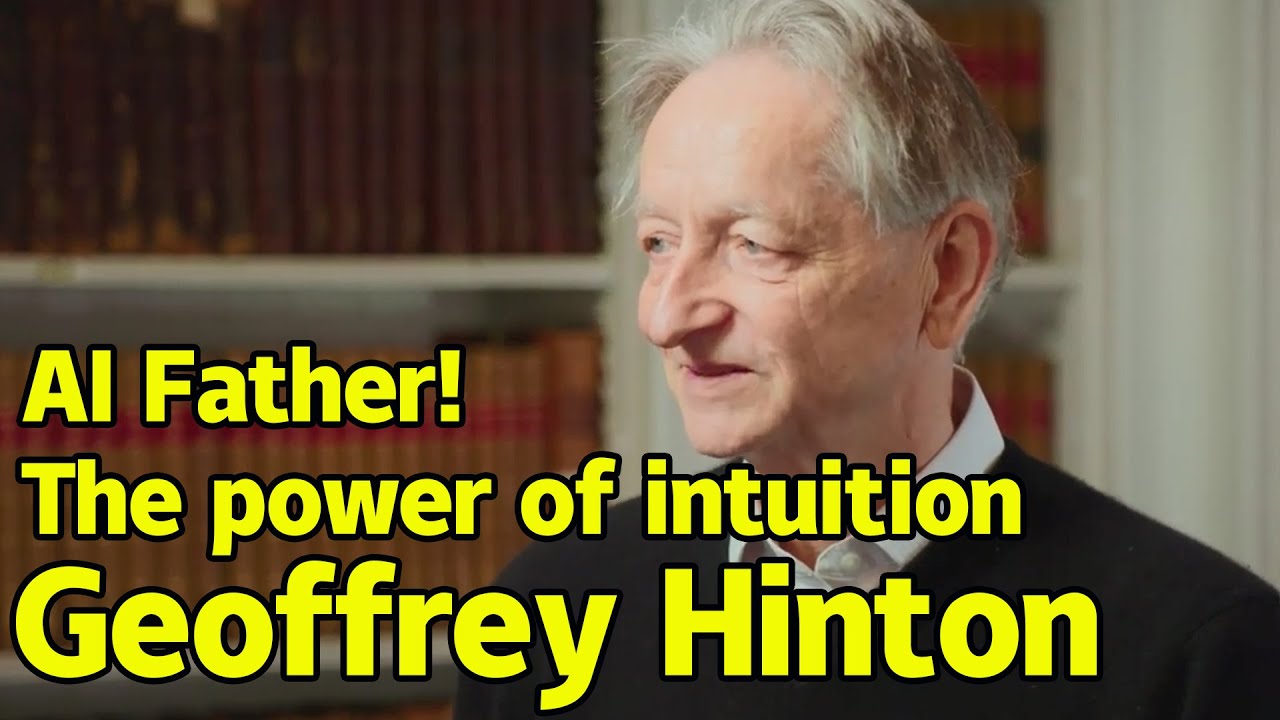
Geoffrey Hinton | Ilya's AI tutor Talent and intuition have already led to today’s large AI models
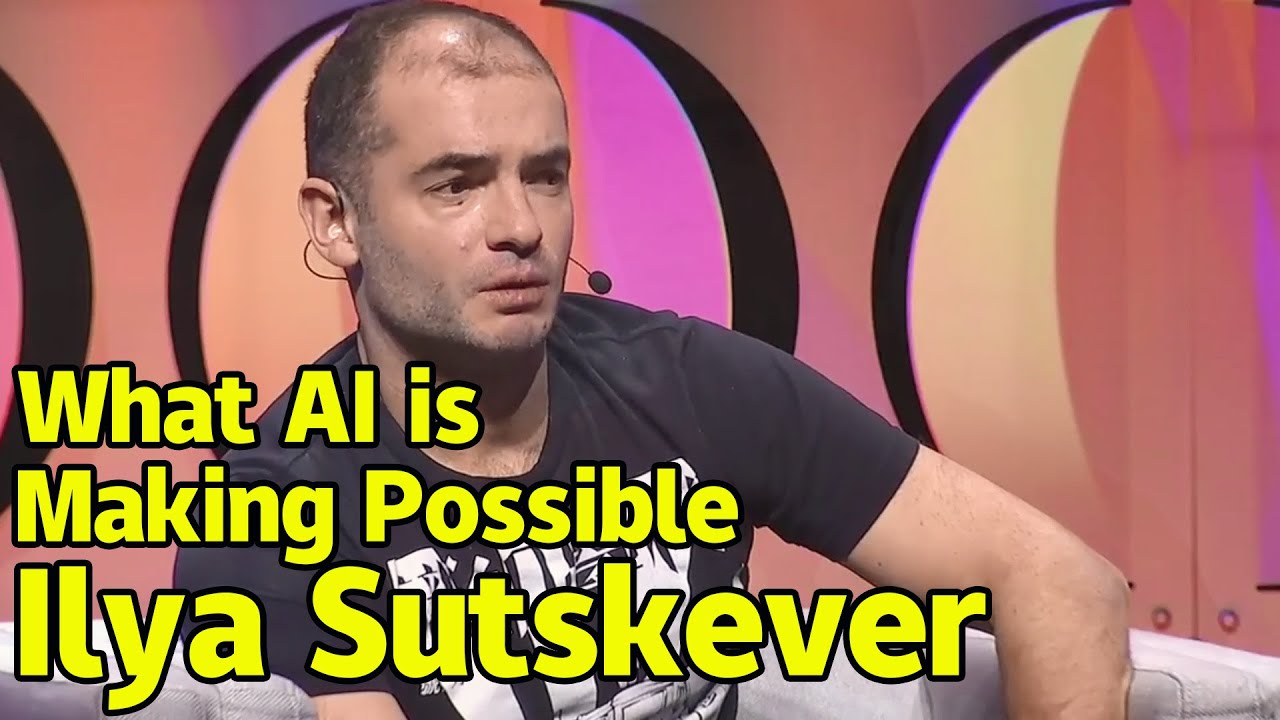
Ilya Sutskever | AI will be omnipotent in the future | Everything is impossible becomes possible
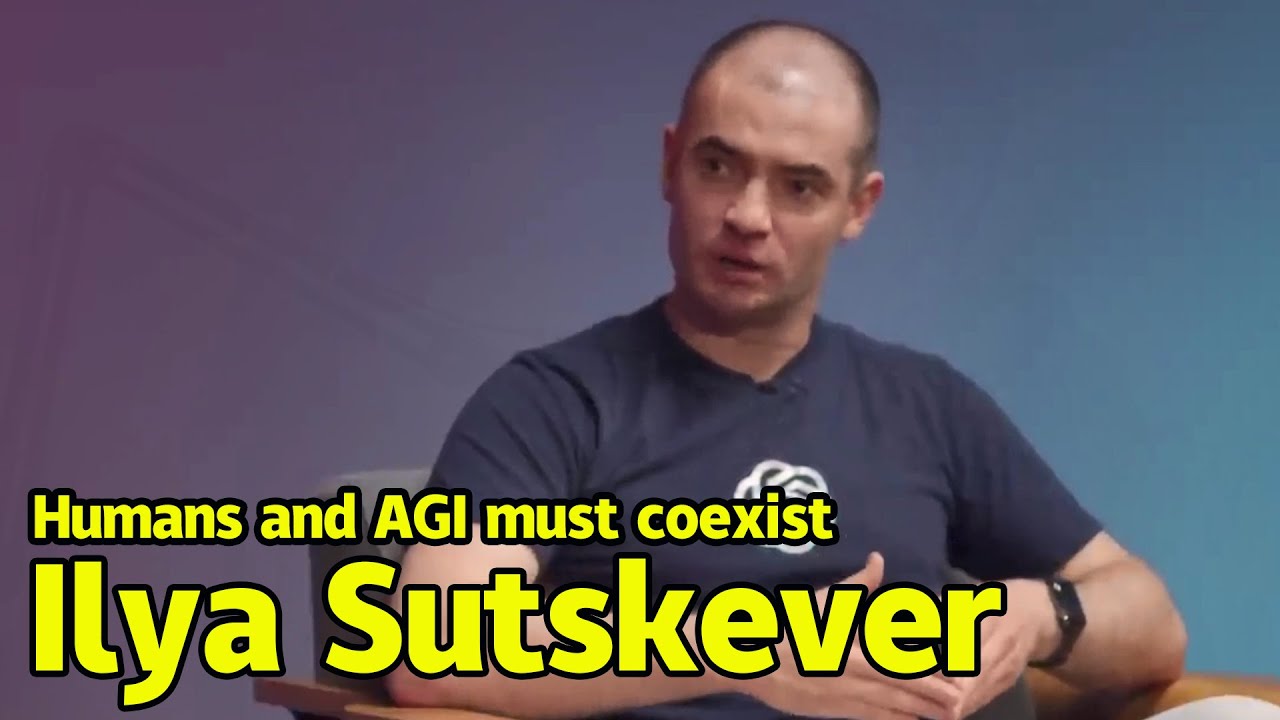
Ilya Sutskever | The future of AGI will be like what you see in the movies
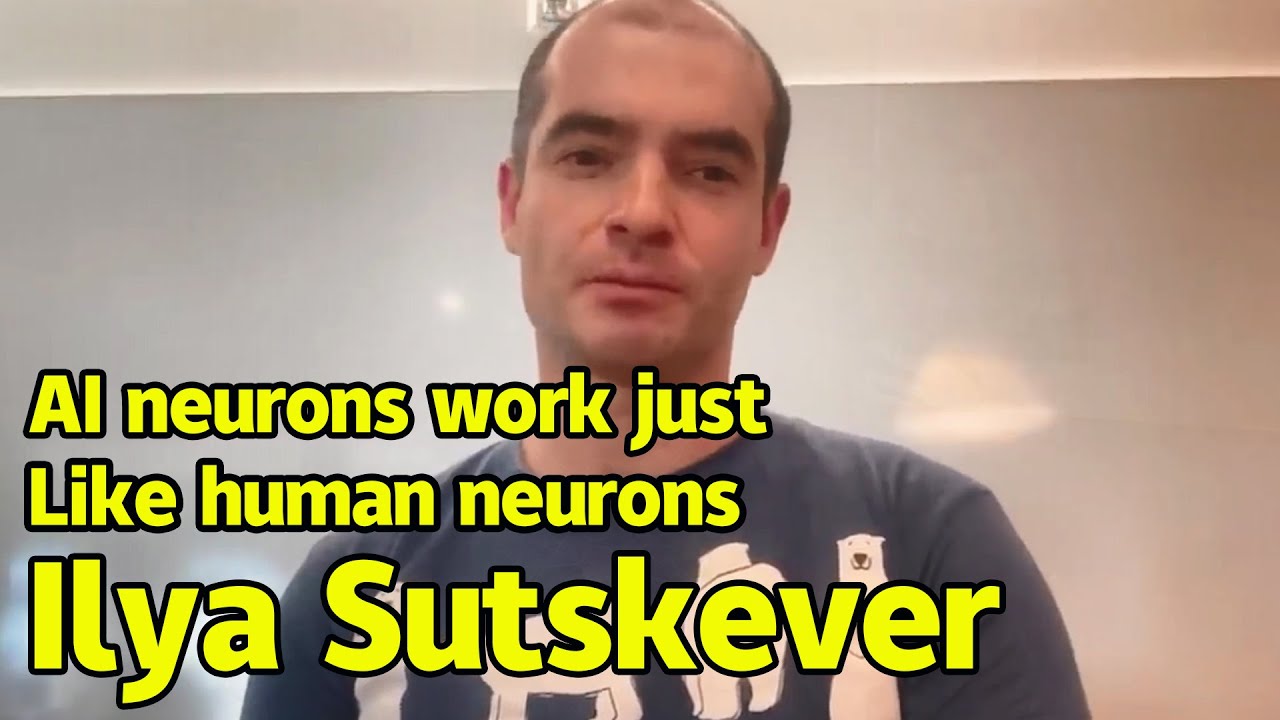
Ilya Sutskever | AI neurons work just like human neurons | AGI will be conscious like humans

Highlights of the Fireside Chat with Ilya Sutskever & Jensen Huang: AI Today & Vision of the Future
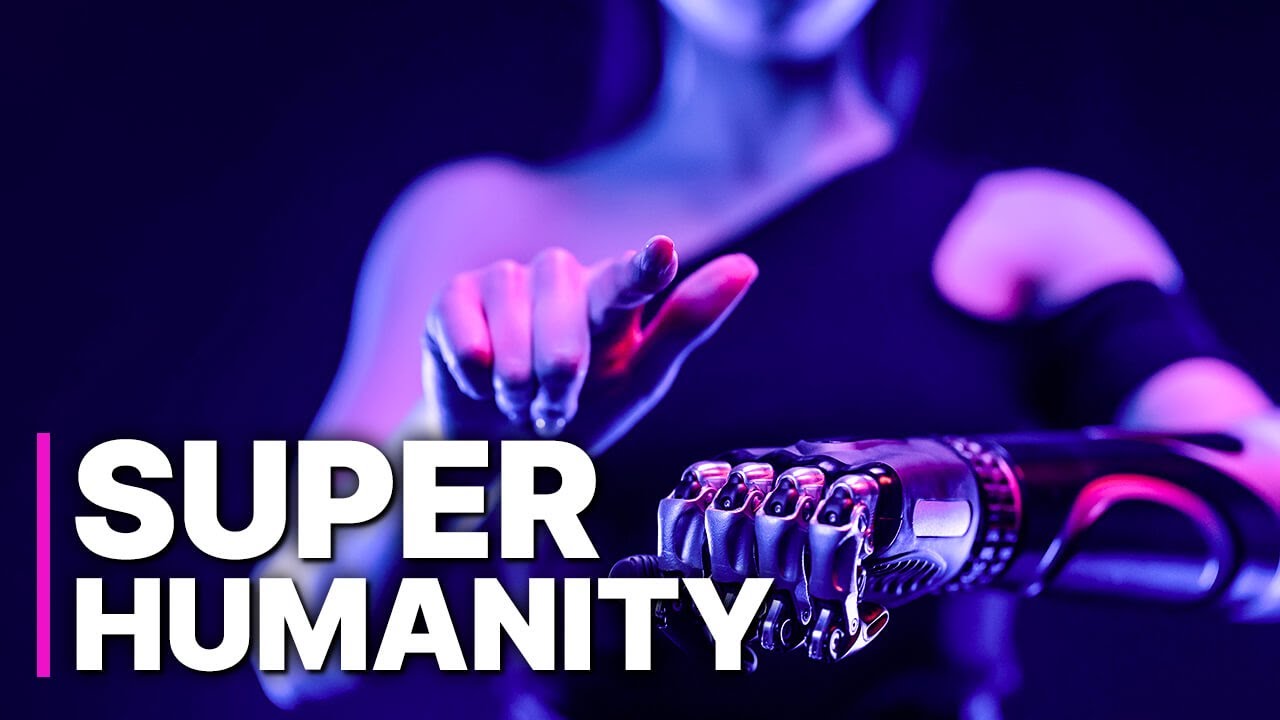
Super Humanity | Transhumanism
5.0 / 5 (0 votes)