Ilya Sutskever | AI will be omnipotent in the future | Everything is impossible becomes possible
Summary
TLDR在这段视频脚本中,讨论了深度学习的发展和人工通用智能(AGI)的未来。演讲者表达了对大型神经网络能够展现出惊人行为的信念,并讨论了实现AGI所需的两个关键信念:人类大脑的复杂性和人工神经元与生物神经元的相似性。同时,也探讨了Transformer模型的潜力、算法选择的重要性以及模型规模增长对性能的影响。最后,讨论了AI安全性问题,包括超级智能带来的挑战和如何确保AI技术的健康发展。
Takeaways
- 😀 深度学习的信念:信念来自于大脑的复杂性和人工神经元与生物神经元的相似性。
- 😀 AGI 的定义:一个能够自动化大多数智力劳动的计算机系统,可以被视为一个计算机同事。
- 😀 当前模型的局限:我们现有的架构还可以进一步改进,尽管现有架构已能实现显著进步。
- 😀 对 Transformer 的看法:尽管 Transformer 很强大,但仍有改进空间,可以期待更高效的架构。
- 😀 扩展规律的理解:对扩展规律的理解尚不完善,但在一些特定任务上有不错的预测能力。
- 😀 意料之外的能力:神经网络在编码能力上的快速提升让人印象深刻。
- 😀 神经网络工作的惊讶:早期神经网络的表现有限,现在它们的进步超出了预期。
- 😀 AI 安全的三大担忧:对齐问题、控制问题、自然选择问题。
- 😀 超智能的潜力与风险:超智能可能带来难以想象的力量和好处,但也伴随巨大的风险,需要谨慎处理。
- 😀 人工智能的未来:AI 未来可能会帮助解决其自身带来的挑战,创造出令人难以置信的生活。
Q & A
为什么早期就坚信深度学习模型的规模扩大会带来意想不到的行为?
-需要两个信念:一是人脑很大,不同生物的脑大小与能力成正比;二是人工神经元与生物神经元在信息处理上可能足够相似,即使生物神经元更复杂。
AGI的定义是什么?
-AGI是能够自动化绝大多数智力劳动的计算机系统,可以视为与人类智能相当的电脑同事。
目前是否拥有实现AGI的所有要素?
-目前的技术栈已经很复杂,Transformers等模型已经相当强大,但仍有提升空间,可能需要更高效的模型或训练方法。
不同算法如LSTM和Transformer在规模扩大后是否会有相同的效果?
-几乎可以肯定,如果对LSTM进行适当修改并扩大其隐藏状态,即使效率可能有所不同,也能实现类似的功能。
我们对模型规模扩大后能力的理解有多好?
-我们对规模法则有一定的理解,但预测模型的特定能力,尤其是新兴行为,仍然是一个挑战。
在模型规模扩大过程中,哪些能力的出现最令人惊讶?
-最令人惊讶的是神经网络的工作原理得到了验证,以及它们在编程等任务上的能力迅速提高。
如何看待AI安全问题?
-随着AI能力的提升,安全问题变得尤为重要。需要解决对齐问题、人类利益问题以及自然选择的挑战。
超级智能与AGI有何不同?
-超级智能意味着比AGI更强大的能力,可能远远超出人类的想象,需要特别的安全措施来控制其巨大的力量。
如何确保超级智能的对齐问题得到解决?
-可能需要国际组织制定高标准,确保超级智能的发展方向与人类利益一致。
人类如何利用超级智能解决它自身带来的挑战?
-希望超级智能能理解现实更深刻,帮助我们解决由其强大能力带来的问题。
自然选择的挑战在超级智能时代意味着什么?
-即使解决了对齐问题和人类利益问题,自然选择仍然会导致变化,可能需要如人机合一等新解决方案来适应。
Outlines
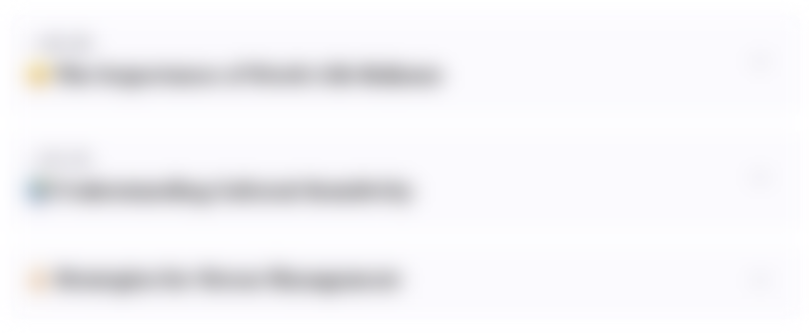
This section is available to paid users only. Please upgrade to access this part.
Upgrade NowMindmap
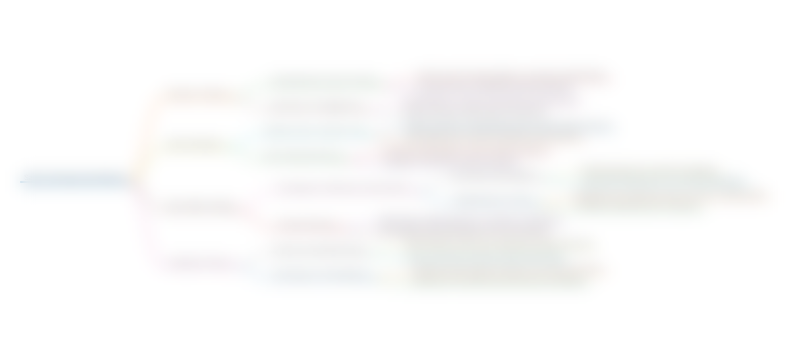
This section is available to paid users only. Please upgrade to access this part.
Upgrade NowKeywords
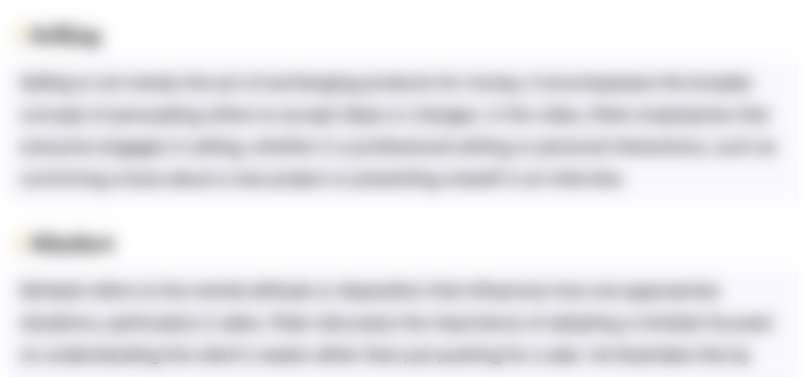
This section is available to paid users only. Please upgrade to access this part.
Upgrade NowHighlights
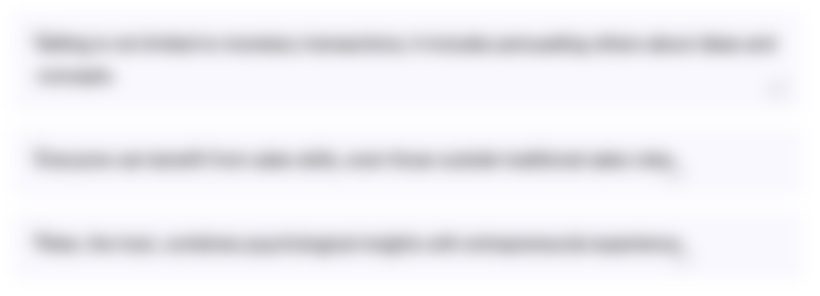
This section is available to paid users only. Please upgrade to access this part.
Upgrade NowTranscripts
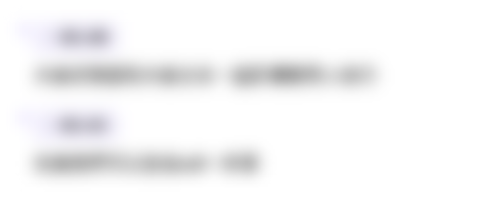
This section is available to paid users only. Please upgrade to access this part.
Upgrade NowBrowse More Related Video
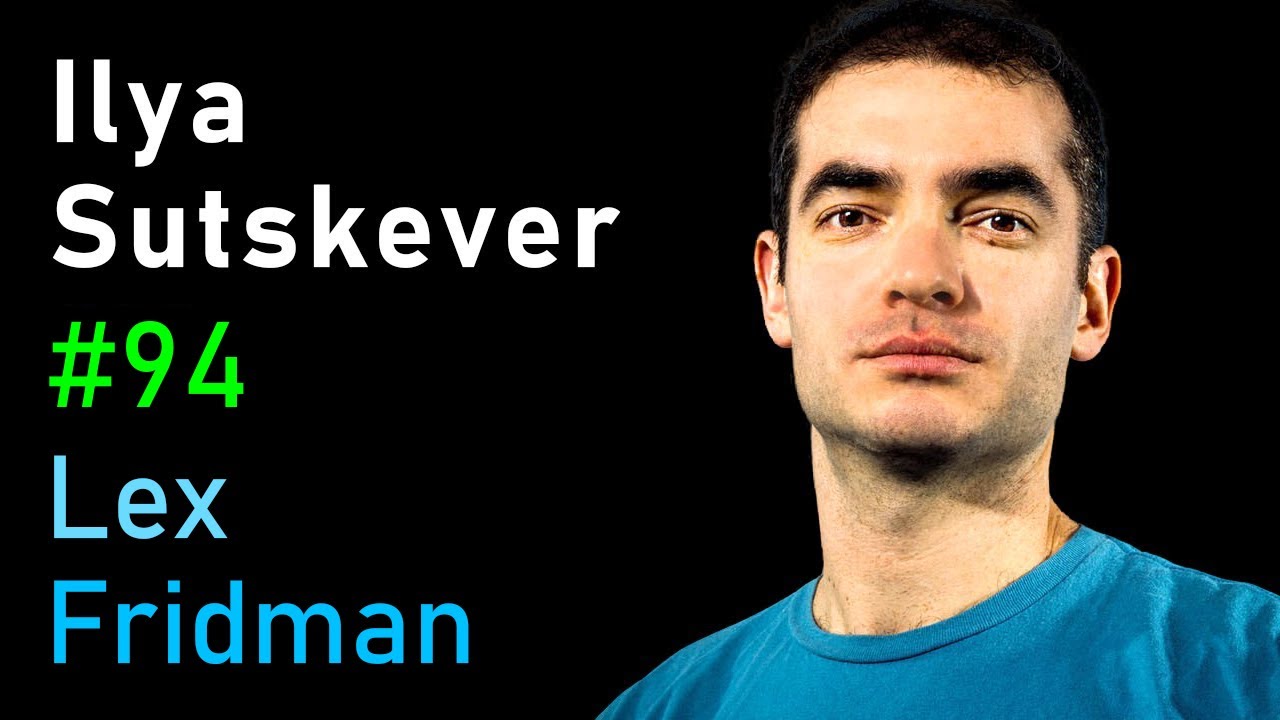
Ilya Sutskever: Deep Learning | Lex Fridman Podcast #94
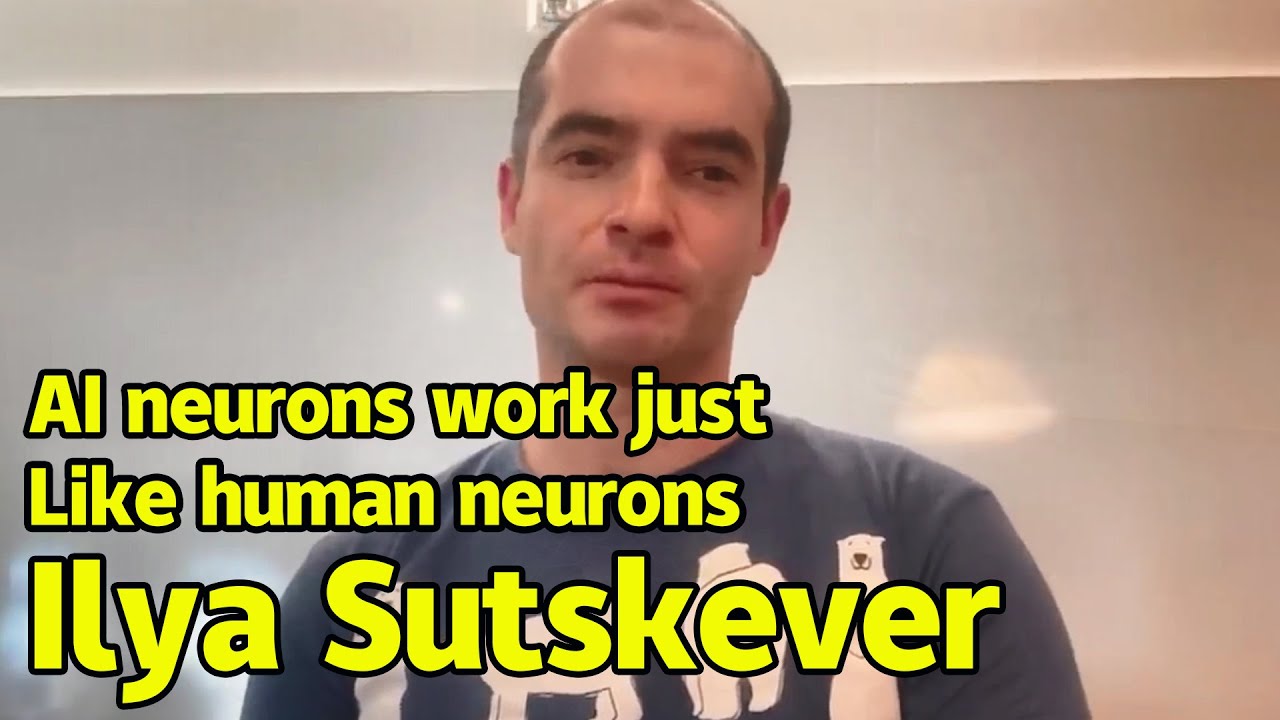
Ilya Sutskever | AI neurons work just like human neurons | AGI will be conscious like humans
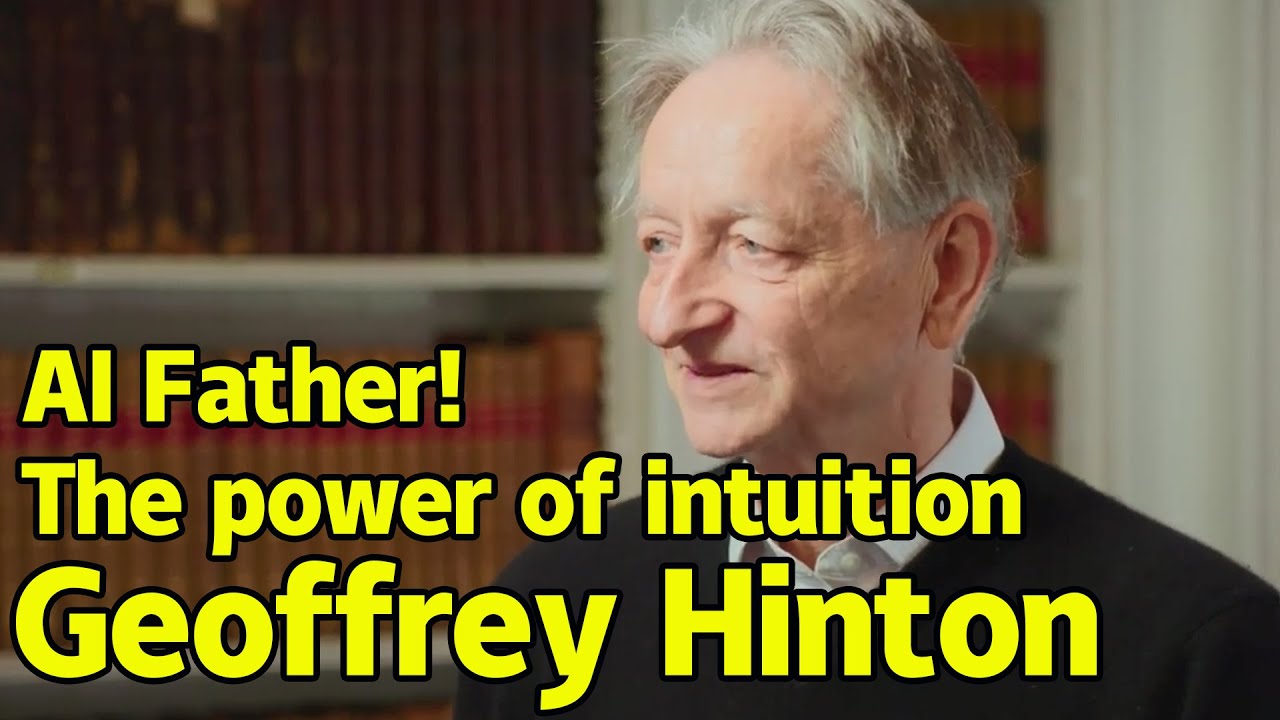
Geoffrey Hinton | Ilya's AI tutor Talent and intuition have already led to today’s large AI models
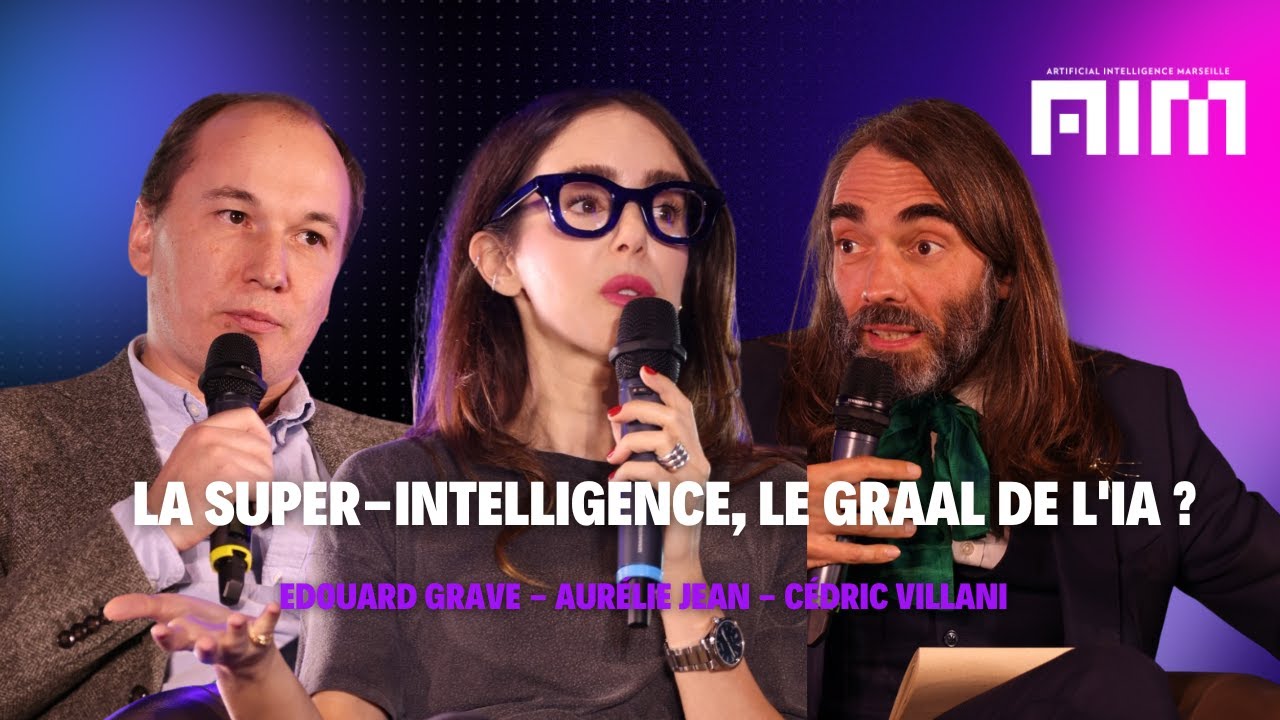
La super-intelligence, le Graal de l'IA ? | Artificial Intelligence Marseille
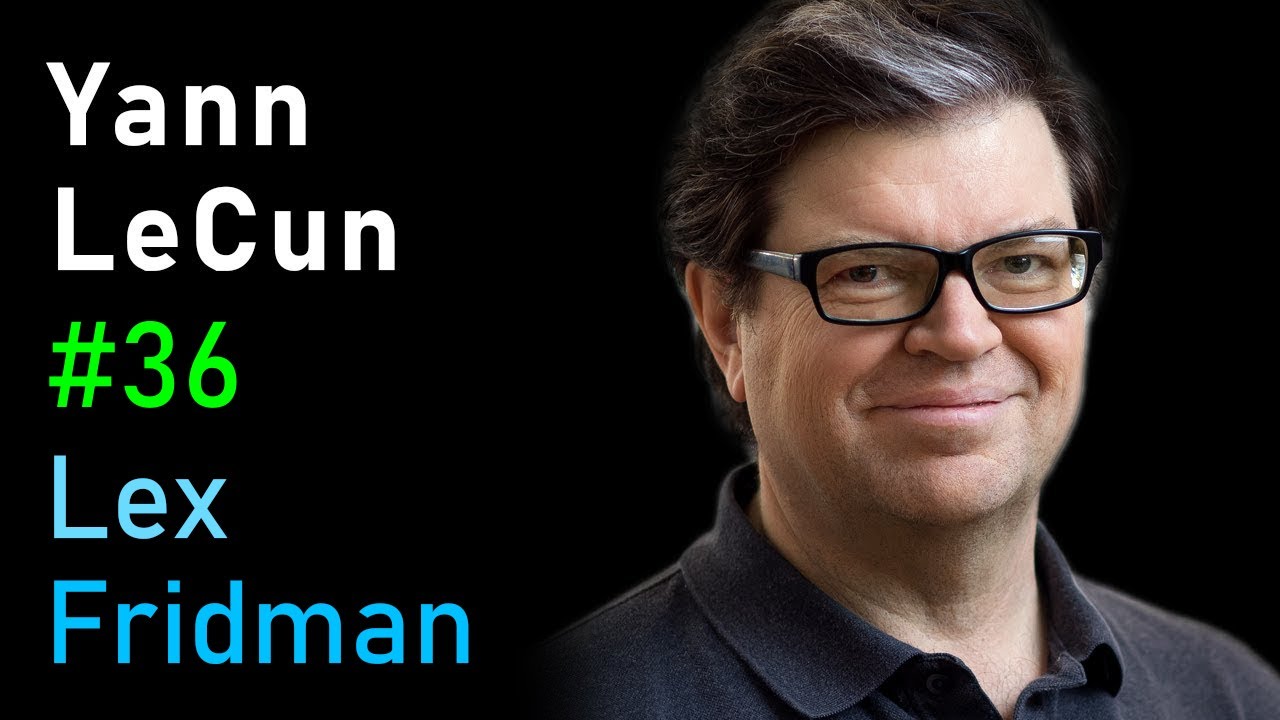
Yann LeCun: Deep Learning, ConvNets, and Self-Supervised Learning | Lex Fridman Podcast #36
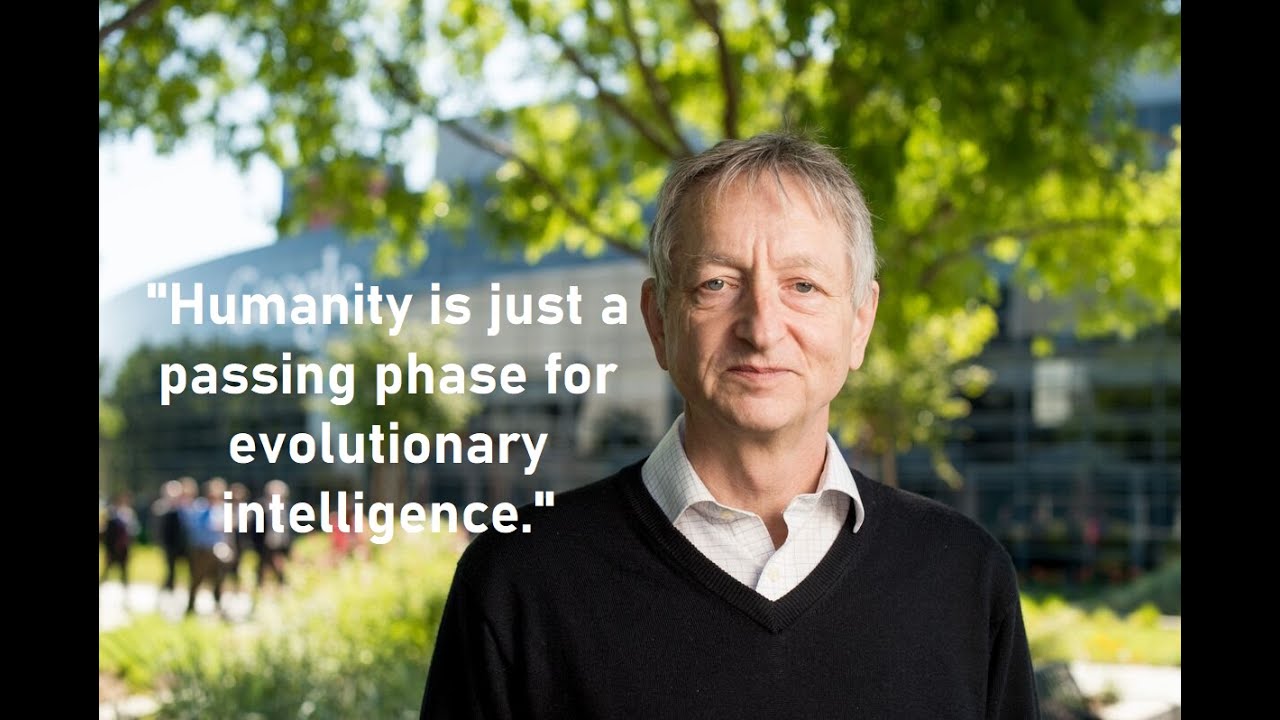
Possible End of Humanity from AI? Geoffrey Hinton at MIT Technology Review's EmTech Digital
5.0 / 5 (0 votes)