Ilya Sutskever | The future of AGI will be like what you see in the movies
Summary
TLDR这段视频剧本探讨了神经网络的复杂性和其在人工智能领域的应用。讨论了神经网络难以精确解释的特性,以及这种特性如何成为构建真正智能的潜在优势。剧本还回顾了神经网络的发展历程,包括早期的挑战和近年来的快速发展。此外,还讨论了OpenAI的成立初衷,以及对未来人工智能技术,特别是在编程、法律和其他白领职业中应用的展望。
Takeaways
- 🧠 神经网络的编写与传统编程不同,它涉及在循环内编写复杂方程,运行后难以精确理解其功能,这与神经网络的不可解释性相关。
- 🤖 神经网络的不可解释性可能是其特性而非缺陷,因为真正的智能本身就难以理解,正如人类自身的认知功能一样。
- 📈 神经网络的成功部分归因于它们在数学上的难以推理,这与早期AI领域的其他方法形成对比。
- 🕵️♂️ 早期对神经网络的研究者需要长期坚持,因为当时AI领域并不看好神经网络,直到2010年左右才开始受到关注。
- 🚀 神经网络的潜力被重新认识,随着计算能力的提升,人们对其潜力的期待也随之增加。
- 🌐 OpenAI的成立是出于将科学与工程紧密结合的愿景,以及对AI技术潜在影响的深刻理解。
- 🛡️ OpenAI的目标之一是确保AI技术的安全和有益使用,同时关注政策和伦理问题。
- 🔮 神经网络的未来发展不仅关乎技术进步,还涉及解决由AI带来的挑战和问题。
- 🔢 神经网络模型的扩展性表明,随着计算能力的增加和数据集的扩大,模型的性能也会随之提高。
- 💡 神经网络的泛化能力是其核心优势之一,但当前仍需大量数据来补偿其泛化能力的不足。
- 🎨 神经网络在创造性任务上的表现出乎意料,这可能与它们的生成模型特性有关,这些模型在艺术创作方面显示出巨大潜力。
Q & A
为什么作者认为神经网络的难以理解并不是一个缺陷,而是一个特性?
-作者认为,如果我们想要构建智能,那么它不应该简单易懂。正如我们无法解释自己如何进行认知功能,如视觉、听觉和语言理解一样,如果计算机能够产生同样难以理解的对象,这可能意味着我们正走在正确的道路上。
作者在什么时期开始对神经网络产生兴趣,并与Jeff Hinton合作?
-作者在2000年代初开始对神经网络感兴趣,并在2003年开始与Jeff Hinton合作。
为什么作者认为神经网络的成功部分是因为它们难以用数学来推理?
-作者认为,神经网络之所以成功,部分原因是因为它们难以用数学来推理,这使得它们能够避免陷入数学证明的陷阱,专注于实际的进展。
作者对于人工智能未来的希望是什么?
-作者希望在未来,我们不仅能构建强大且有用的AI,而且能够实现通用人工智能(AGI),并确保它能够被用来解决大量问题并创造出许多令人惊叹的应用。
为什么作者决定离开Google并创立OpenAI?
-作者离开Google并创立OpenAI的动机之一是他认为将科学和工程融合在一起是AI发展的正确方式。另一个动机是他对AI技术有了更成熟的认识,希望建立一个能够意识到AI带来的挑战并致力于解决这些问题的公司。
OpenAI的初始愿景和目标是什么?
-OpenAI的初始愿景是将科学和工程融合在一起,消除两者之间的界限,以科学的思想来指导工程实践,以工程的严谨性来推动科学发展。同时,作者希望OpenAI能够意识到AI的复杂性,并致力于使AI安全、有益。
为什么作者认为将科学和工程结合在一起是重要的?
-作者认为,随着AI领域的成熟,小规模的调整已经不足以推动实质性的进步。将科学和工程结合在一起可以确保科学发现得到精心的工程实施,同时确保工程实践得到科学思想的指导。
为什么作者认为数据的可用性对于神经网络的性能至关重要?
-作者指出,神经网络需要大量的数据来训练,以便更好地泛化。在数据丰富的领域,如语言处理,神经网络可以取得很好的效果。但在数据较少的领域,如法律,神经网络可能无法达到同样的性能水平。
作者如何看待神经网络在编程领域的应用,例如Codex模型?
-作者对Codex模型感到兴奋,因为它展示了神经网络能够从自然语言转换到代码,这是AI领域的一个重要进步。Codex模型能够理解自然语言描述的需求,并生成可运行的代码,这可能会改变编程职业的性质。
为什么作者认为神经网络在创造性任务上的表现可能优于简单机器人任务?
-作者认为,神经网络在创造性任务上的表现可能优于简单机器人任务,因为创造性任务如图像和文本生成有大量的数字数据可供学习,而机器人任务受限于现实世界中的数据收集。
作者如何看待神经网络的泛化能力?
-作者认为,神经网络的泛化能力是其核心优势之一。泛化能力使得神经网络能够在面对新的、不同于训练数据的情况时做出正确的反应。然而,这种能力尚未达到人类水平,因此需要大量的数据来补偿。
作者对神经网络在未来的发展有哪些期望?
-作者期望神经网络能够继续提高其泛化能力,减少对大量数据的依赖。他还期望神经网络能够更好地理解和生成代码,从而改变编程和其他白领职业的性质。
为什么作者认为神经网络的泛化能力对于创造性应用特别重要?
-作者认为,创造性应用特别适合神经网络,因为生成模型在机器学习中扮演着核心角色,它们生成新的、合理的数据的方式与艺术创作过程有相似之处。
Outlines
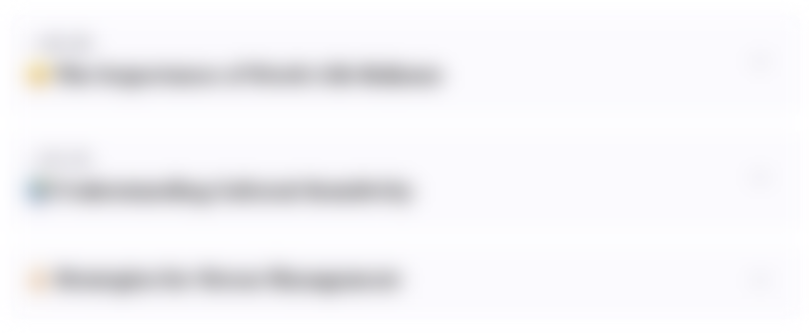
This section is available to paid users only. Please upgrade to access this part.
Upgrade NowMindmap
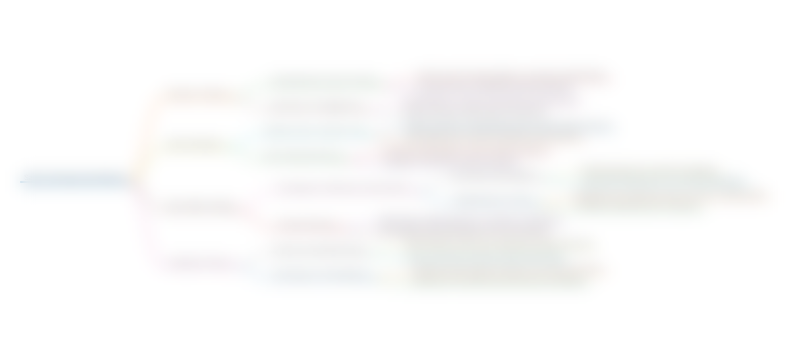
This section is available to paid users only. Please upgrade to access this part.
Upgrade NowKeywords
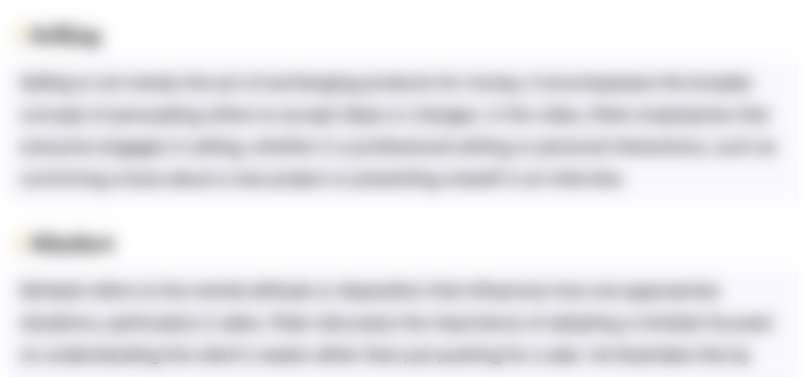
This section is available to paid users only. Please upgrade to access this part.
Upgrade NowHighlights
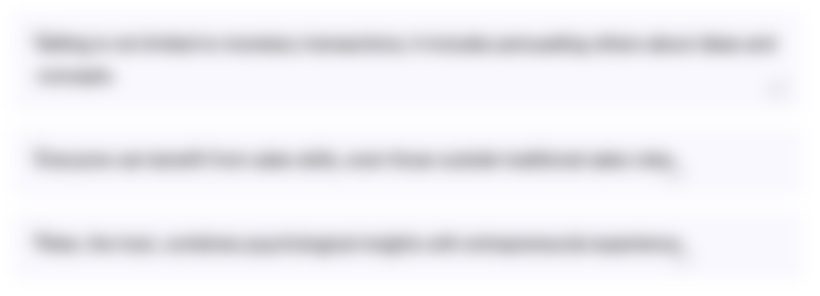
This section is available to paid users only. Please upgrade to access this part.
Upgrade NowTranscripts
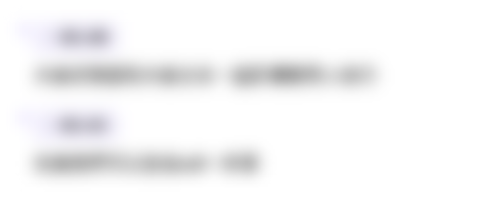
This section is available to paid users only. Please upgrade to access this part.
Upgrade NowBrowse More Related Video

Geoffrey Hinton: The Foundations of Deep Learning
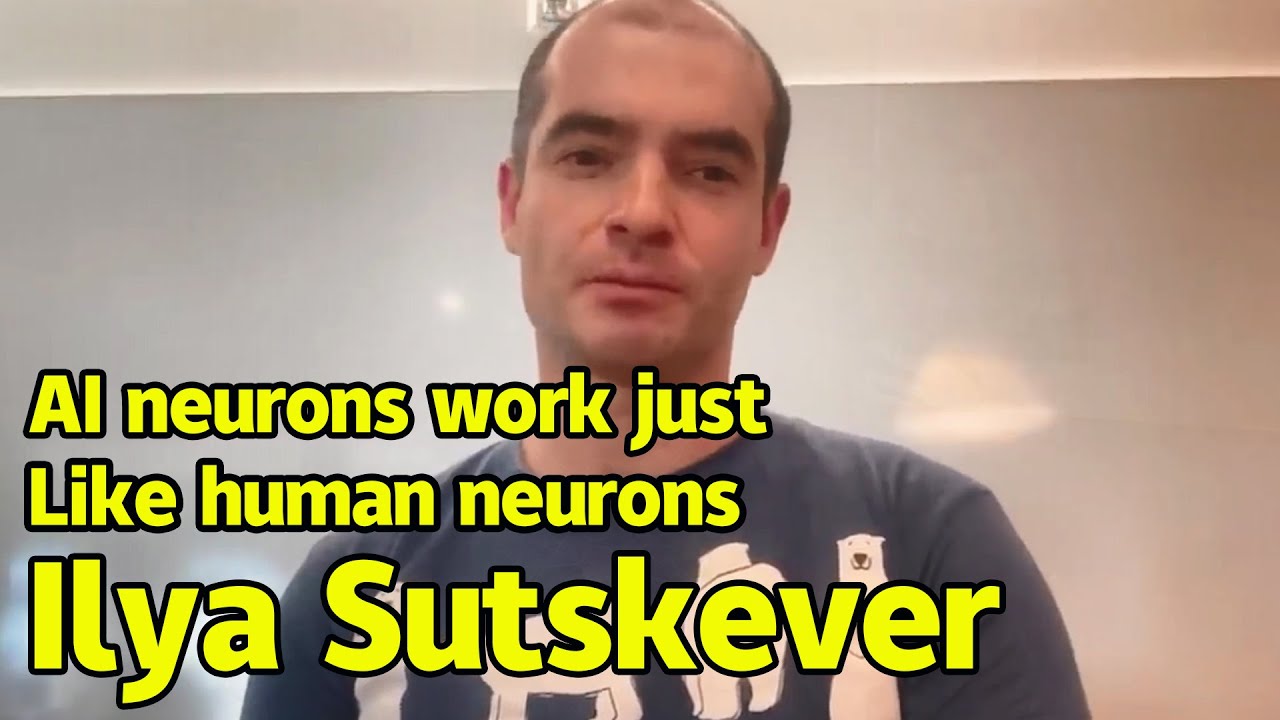
Ilya Sutskever | AI neurons work just like human neurons | AGI will be conscious like humans
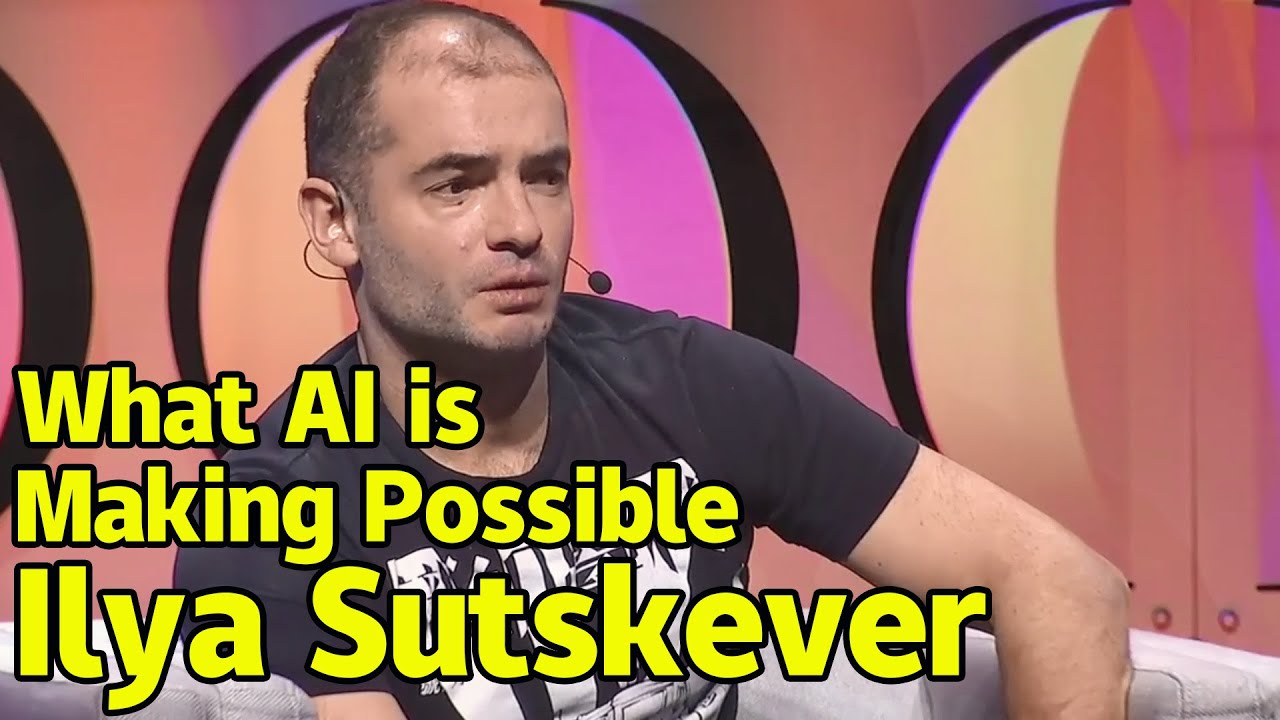
Ilya Sutskever | AI will be omnipotent in the future | Everything is impossible becomes possible
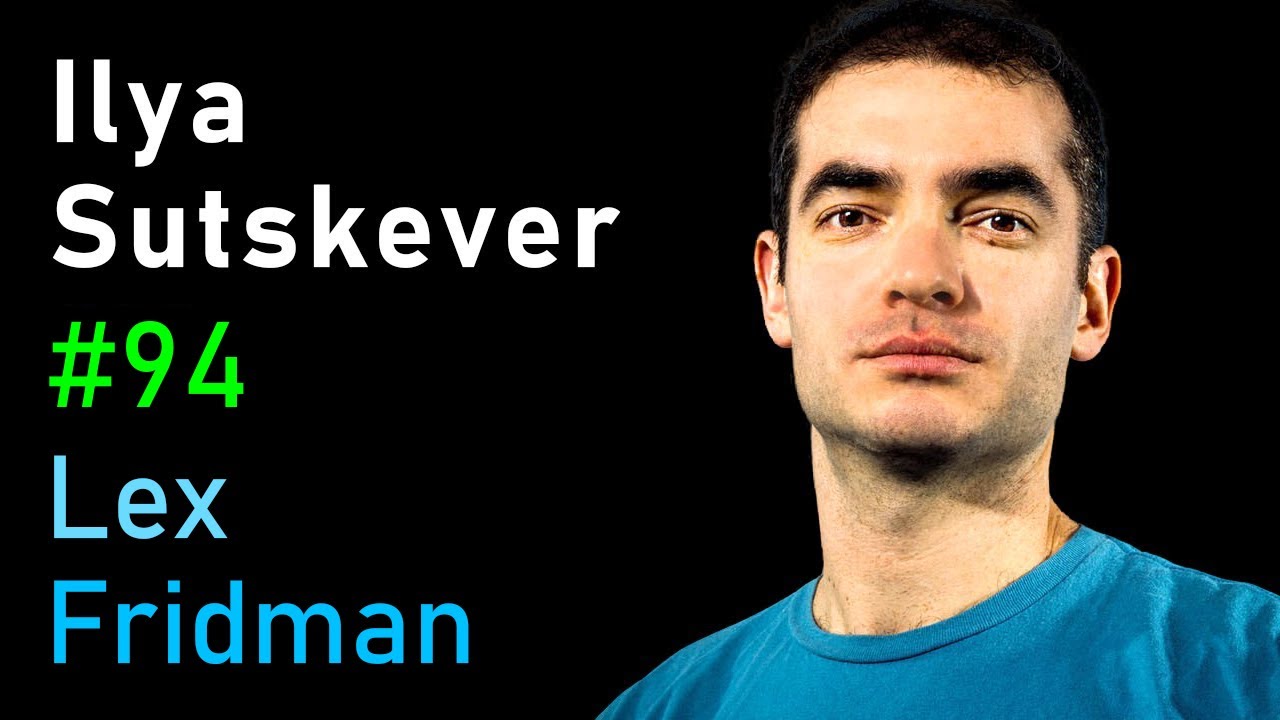
Ilya Sutskever: Deep Learning | Lex Fridman Podcast #94
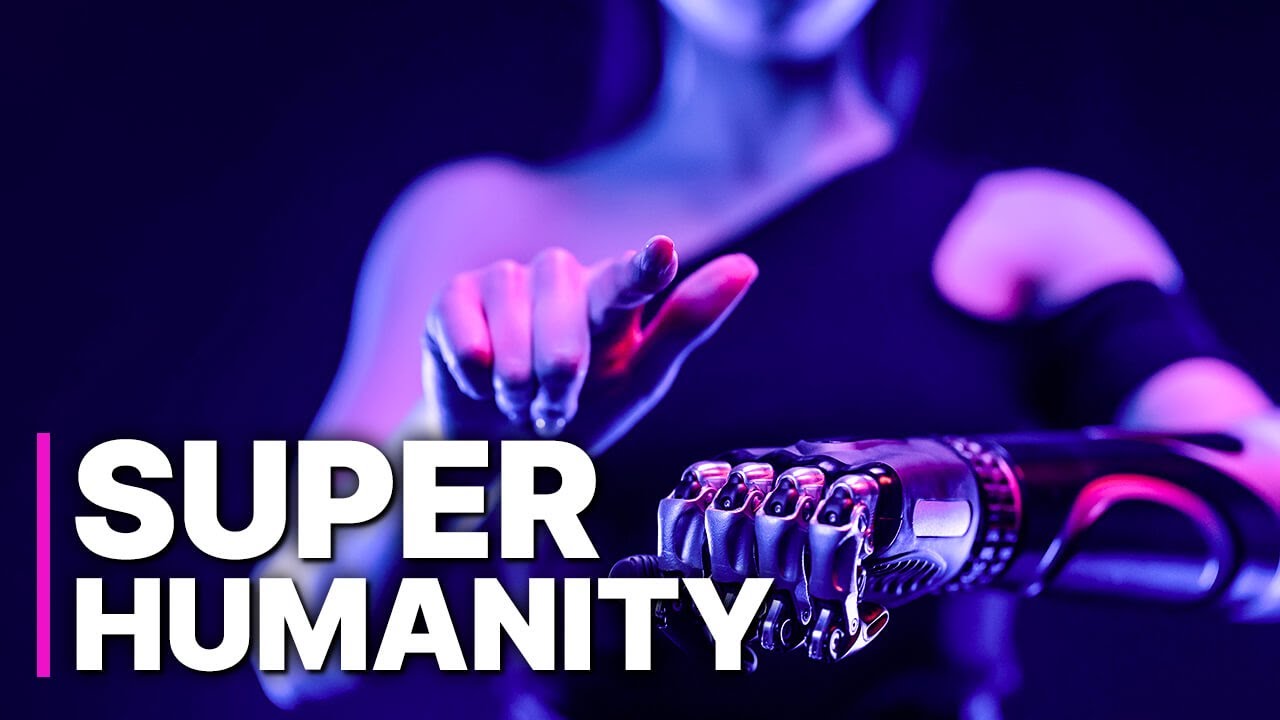
Super Humanity | Transhumanism
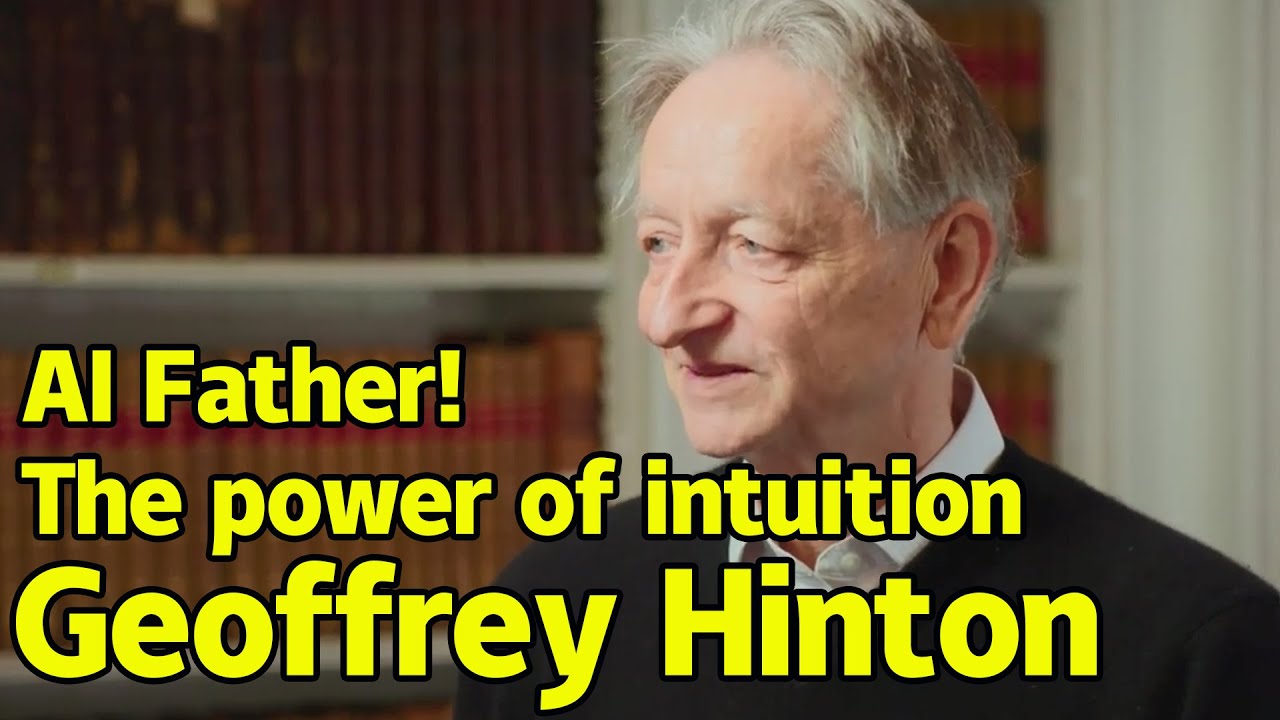
Geoffrey Hinton | Ilya's AI tutor Talent and intuition have already led to today’s large AI models
5.0 / 5 (0 votes)