Simplify data integration with Zero-ETL features (L300) | AWS Events
Summary
TLDRこのビデオスクリプトでは、データ分析における多岐にわたるデータ源を統合するZL(Zero ETL)ソリューションが紹介されています。リレーショナルデータベースやNoSQLデータベースからデータウェアハウスやデータレイクへのデータ移動を自動化し、分析のためのリアルタイムデータアクセスを提供します。スピーカーであるTasとAmanは、AWSサービス間のデータ統合の課題と、ZLが提供する解決策、その価値提案、および顧客事例について詳述しています。
Takeaways
- 🗃️ ZL(Zero ETL)は、データ分析のためのデータ統合を簡素化し、異なるデータベース間でのデータ移動を支援する一連の統合です。
- 🔍 ビジネスにとってデータは全てで、より多くのデータを持つほど分析が可能で洞察を得られますが、データは異なる場所に分散しています。
- 🤝 タスとアマンは、リレーショナルデータベースとNoSQLデータベースの専門家で、それぞれがZL統合の異なる側面について議論します。
- 🔄 ZLは、ストリーミングデータのコピーをサポートし、複数のソースから複数の宛先にデータを移動させることが可能です。
- 🚀 Amazon Aurora MySQLとRedshiftの間のZL統合により、リアルタイムで分析を実行できるようになります。
- 🛠️ ZLは、データパイプラインの構築、維持、監視、セキュリティ確保の複雑さを軽減し、ビジネスの分析ニーズに応えるための「分かりにくい作業」を削減します。
- 📈 Redshiftでの分析により、より迅速で高度な分析操作が可能となり、ビジネスの意思決定プロセスを強化します。
- 🔗 ZLは、DynamoDBからOpenSearchへのデータ移動をサポートし、NoSQLデータベースと検索サービスの間の同期を容易にします。
- 📊 Data Prepperというオープンソースのサーバーレス技術を活用して、ZLはETLプロセスを簡素化し、データの抽出、変換、ロードを自動化します。
- 📈 ZL統合は、DynamoDBからRedshiftへのデータのインポートもサポートしており、データウェアハウスでの分析が可能になります。
- 🔑 ZLはAWSが積極的に投資しているプロジェクトであり、将来的にはより多くのデータストアやデータソースとの統合が期待されています。
Q & A
ZLとはどのようなソリューションですか?
-ZLは、複数のソースから複数の宛先にストリーミングデータをコピーするための統合セットです。データのコピー、セキュリティ確保、および効率化を目的としています。
ZLが解決しようとしている問題とは何ですか?
-ZLは、ビジネスの分析面と操作面の間でデータを移動する際に必要となる、多数のパイプラインの構築、維持、監視、セキュリティ確保の複雑さを軽減することを目指しています。
Amazon Aurora MySQLとRedshift間のデータ転送に関して、ZLはどのように機能しますか?
-ZLは、Amazon Aurora MySQLからRedshiftへのデータ転送をリアルタイムで自動化し、操作データベースに追加の負荷をかけることなく、分析を可能にします。
データの低レイテンシ移転を実現するためにZLはどのような技術を使用していますか?
-ZLはAuroraのストレージ機能を最大限活用し、変更されたデータのみをキャプチャしてRedshiftに送信することで、レイテンシを数桁の秒間に抑えることを目標としています。
ZLが提供する統合の種類には何がありますか?
-ZLは、現在、Amazon Aurora MySQLとRedshift、Amazon DynamoDBとOpenSearchの間での統合を提供しています。他の統合はプレビュー段階または準備中です。
DynamoDBとOpenSearch間のZL統合にはどのような利点がありますか?
-DynamoDBとOpenSearch間のZL統合により、データの同期を維持しながら、検索機能を強化し、ユーザーエクスペリエンスを向上させることができます。
Data Prepperとは何であり、ZL統合でどのような役割を果たしていますか?
-Data PrepperはオープンソースのサーバーレスETLツールで、ZL統合でデータを抽出、変換、およびロードするプロセスを自動化します。
ZL統合を使用することでデータエンジニアにどのような利点がありますか?
-ZL統合を使用することで、データエンジニアはデータパイプラインのコードの維持やインフラの設定などの煩わしい作業から解放され、より迅速かつスケーラブルなデータストア間のセットアップが可能になります。
AWSは今後ZLにどのような投資を続ける予定ですか?
-AWSはZLへの投資を続けており、他のデータベースやデータソースとの統合も計画中です。AWSはZLのサポートを拡大し、より多くのユーザーニーズに対応する予定です。
ZL統合のフィードバックはどのように提供できますか?
-セッションの後またはAWSアカウントチームに連絡することで、ZL統合に関するフィードバックや要望を提供できます。AWSはユーザーからのフィードバックを活用してサービスを改善しています。
Outlines
😀 ゼロコードETLの紹介
この段落では、TasとAmanがゼロコードETL(ZL)の重要性とその機能について説明しています。Tasはリレーショナルデータベース専門家であり、AmanはDynamoDBの専門家です。彼らはデータ分析における多岐にわたるデータソースの課題と、ZLが提供する解決策について話しました。ZLはリレーショナルデータベースからストリーミングデータのコピーをサポートする一連の統合であり、分析エリアへのデータ移動を容易にします。また、彼らはこのプロセスを通じてデータの安全性を確保し、分析のためのデータパイプラインの複雑さを軽減することを目的としています。
😉 Amazon Aurora MySQLとRedshift間のZL統合
この段落では、Aurora MySQLからRedshiftへのデータ転送を自動化するZLの機能について詳しく説明しています。このプロセスはほぼリアルタイムで行われ、操作データベースに追加の負荷をかけることなく、Redshiftで分析が可能になります。また、ZLは複数のクラスターからデータを引き出し、Redshiftに送信することができるため、大量のデータを分析することができます。ZLはAuroraのストレージ機能を最大限に活用し、データの変更のみをキャプチャしてRedshiftに送信することで、低レイテンシを実現しています。
🎓 AmanによるDynamoDBとOpenSearch間のZL統合の解説
AmanはDynamoDBとOpenSearch間のZL統合について説明しています。この統合は、高スループットで低レイテンシのDynamoDBと柔軟性の高い検索機能を持つOpenSearchを組み合わせた場合に適しています。ZLは、Data PipelineやLambda関数を通じてDynamoDBの変更をOpenSearchに反映させるためのコードや設定を簡素化します。Data Prepperというオープンソースのサーバーレス技術を利用しており、YAMLテンプレートを使ってETLプロセスを定義できます。これにより、データの抽出、変換、およびロードが容易になり、データストア間の同期が効率的に行われます。
🛠️ DynamoDBからRedshiftへのZL統合の詳細
この段落では、DynamoDBからRedshiftへのデータのインポートについて説明しています。ZLは、DynamoDBのインクリメンタルエクスポート機能を利用して、変更されたデータのみを定期的にRedshiftに転送します。このプロセスは、データの差分を効率的に取得し、Redshiftでの分析を可能にします。ターゲットテーブルは、DynamoDBのスキーマレスな特性を考慮して作成され、パーティションキーとソートキーに基づいてRedshiftにデータをインポートします。この方法により、データエンジニアはデータパイプラインの維持にかかる労力を軽減できます。
🔍 ZL統合の能力とAWSの将来の計画
最後の段落では、ZL統合の能力がデータエンジニアにとってどのように有益であるかを強調しています。ZLは、データパイプラインの維持とコードの管理の複雑さを軽減し、データストア間の低レイテンシでスケーラブルなセットアップを実現します。AWSはZLの将来について積極的に投資しており、他のデータベースやデータソースとの統合も計画中です。フィードバックを歓迎し、ZL統合に関するリクエストや要望についてはAWSアカウントチームに連絡するよう呼びかけています。
📢 ZLのサポートとフィードバックの重要性
このセクションでは、ZL統合のサポートとフィードバックの重要性が強調されています。ZLはデータエンジニアにとって新しいツールであり、AWSはその開発を積極的に進めています。参加者は、ZL統合に関するフィードバックや要望をAWSチームに伝えることが期待されています。ZLのサポートと改善のために、ユーザーからの意見が非常に重要であると述べています。
Mindmap
Keywords
💡データ統合
💡ZL (Zero ETL)
💡Amazon Aurora MySQL
💡Redshift
💡DynamoDB
💡OpenSearch
💡Data Prepper
💡リアルタイム分析
💡データパイプライン
💡AWS
Highlights
Tas和Aman分别作为数据库专家和DynamoDB解决方案架构师,共同探讨了ZL集成解决方案。
ZL集成旨在解决数据分散在多个数据库中的问题,实现数据的统一分析。
数据集成的挑战在于需要构建、维护和监控将数据从操作数据库转移到分析数据库的多个管道。
ZL集成提供了一种减少未区分工作量的方法,确保数据安全地从一个数据库复制到另一个数据库。
介绍了Amazon Aurora MySQL到Redshift的数据传输集成,实现近实时分析而不影响操作数据库。
ZL集成利用Aurora存储功能和Redshift存储功能,通过增强的二进制日志捕获仅变化的数据。
展示了ZL集成如何帮助用户分析来自多个集群的大量数据,而无需额外的运维工作。
Aman讨论了DynamoDB到OpenSearch的集成,强调了使用正确的工具来完成正确的工作的重要性。
解释了如何使用Data Prepper作为无服务器技术来简化ETL过程,减少用户的工作量。
展示了ZL集成如何在YAML模板中定义提取、转换和加载数据的过程。
讨论了DynamoDB到Redshift的集成,强调了增量导出功能和对数据仓库的同步。
提供了关于如何将DynamoDB中的数据变化同步到Redshift的详细信息。
强调了ZL集成的监控能力,允许用户了解系统内部发生的情况。
提到了AWS对ZL集成未来的投资,以及对其他数据库或数据源集成的探索。
鼓励用户提供反馈,以改进ZL集成并满足他们对数据存储的需求。
总结了ZL集成的能力,特别是在减少数据工程师在维护数据管道方面的努力。
强调了ZL集成支持的数据库和数据源的多样性,以及AWS对新集成的持续开发。
最后,演讲者感谢了参与者,并邀请他们提供反馈以改善会议体验。
Transcripts
so we had few few talks before us and
almost in each of them zero ATL was
mentioned so let's unpack it
now my name is Tas I'm a database
specialist focused on relational engines
and I accompanied by Aman he's a Dynamo
DB specialist he will be helping us to
review the other part of the
presentation about ZL integration
so what we're going to talk about we
will review H what problem we're trying
to solve with this with this integration
with this
solution um we'll see what it all about
how it works and what its value
proposition and we will see how it used
by our customers and discuss it and we
have some call for Action
later so it's been mentioned multiple
times today that data is everything for
our business right so the more data we
have the more insight we get we can gain
from it but the data is located in
different places and we want to run
almost the same analysis on all the
sources of our data right we want to get
Trend analysis when to take fraud when
to understand that everything is good so
what what is a challenge let's see with
raise of hands how many of you need to
analyze data from multiple
databases
yeah and all right good amount of people
so you in the right
room and who need to build multiple
pipelines and maintain them for this
purpose all
right so we kind of understood
understanding now what is the complexity
with what what is the problem the data
our data sources our
operational data is located in
multiple databases or data sources and
we like to move them to our analytical
area it can be data warehouse it can be
data Lake um and we need to build and
maintain and of course Monitor and
secure all the pipelines that transfer
this data from operational side of the
business to the analytical side of the
business so this is the challenge
and this is the solution that we
suggesting for for this um and it's
called ZL so it's not one solution it's
set of integration that can copy data
help with copying of streaming data from
multiple sources to multiple destination
we'll be reviewing them um
shortly and we would like to eliminate
or r or dramatically reduce the amount
of undiff created work undifferentiated
work that you or your teams will be
investing into this process and to make
sure that it's copy the data in Secure
way from database to
database so those Integrations that are
available
currently H you can see that we have a
multiple sources like for relational
databases or MySQL or pogress and of
course no scale databases like Dynamo DB
and open search and we can move data
from through various pipelines to
various destinations and let's review
some of
them so first that we would like to
discuss is the integration between
Amazon Aurora MySQL and red
shift so what's what what's all about
what we are talking about we
currently providing this option to
transfer data without any effort on your
side to build or maintain pipelines in
near real time from
Amazon Aurora for MySQL to Red shift and
we can when we have this data in red
shift we can start analyzing it run our
models on top of it without any
additional load added to our operational
database and the latency is a big
question we're going to discuss it in
few
slides so how how we usually will tackle
this we will have our sources we would
like to have our data in analytical area
in in red shift so usually we will build
multiple pipelines and we will have to
maintain them and monitor them and
secure them and for this we need
resources people and Investments right
so all this is undifferentiated heavy
lifting that your business is not um
benefiting from this so we trying
to to take this into and offload from
you to us and we will be providing this
as a service so it will be much easier
to just spin up this integration between
your Rel database Aurora MySQL in this
case we have this ability in preview for
Aurora pogress so this one is coming
soon and it will be copying the data to
Red shift and this will allow you near
realtime analytics on your production
data without impacting your production
database or any need to upgrade it or
anything
else and of course we we aware that you
may have more than one source of
your of your of the data that you would
like to analyze so ZL can pull data from
multiple clusters and ship it to Red
shift without any addition that you need
to run so this will allow you to
analyze large amount of data in red
shift and with all the benefits that
come with this including faster queries
analytic operations that will be much
much more sophisticated and so
on so what we do H how we make this
magic work so we um we trying to offload
as much as possible of this toward
storage layer so our head nodes or
writers or compute nodes are not busy
with this so we utilizing as much as
possible Aurora storage capabilities and
r storage capabilities and we will be
enabling enhanced bin login on Aurora
side so we will be consistent in our
operation and we'll be capturing only
changes that happened on Aurora and
sending them to Red shift and from tests
that I was running in my environment
usually the data will be in red shift in
single digit seconds so the latency will
be really really low and I think it's
great achievement aent so of course it
may be different in your workloads
but because it's done on because it's
optimized and it's done mostly on
storage layer I hope that your workloads
will be benefiting from this pretty the
same way single digit second latency
between your production data and option
to analyze it in red
shift all right so this is like example
of what we see our customer doing with
this solution let's imagine that we are
um we are company that have multiple
streams of data H let's say data that is
structured and data is not nonstructured
and we have a lot of H data that is um
streaming into Aurora MySQL and we have
a lot of data streaming from um from
streams Kinesis data streams um let's
say we need to analyze both with low
latency and we'd like to have red shift
to hold them so we can offload this from
uh from you towards Zer ETL that will
help him to that will help to ship you
that will help you to ship your data
from MySQL to Red shift with really low
latency and without any need to build it
or maintain it or monitor it and of
course we will expose a lot of counters
so you will be able to see what's going
on and monitor it and and be aware of
what's going on in the
system all
right so just again uh to remind you
this is the integration that are
currently are
there Aurora to Red shift and Amazon
Dynamo DB uh to open search are
available the rest are in preview orora
pogress is in preview RDS from MyQ in
preview and Dynamo DB to Red shift is in
preview so get out there check them see
how they work and of course we'll be
really happy to hear your feedback
because we working on this this is the
project that we just started and we have
future for
this all right thank you and now I will
invite Aman to speak about noise scale
solution for this one
thank you am I audible okay so how many
of us use dwb already in our
applications
today okay I'm in the right room uh so
uh for some use cases you know my name
is Aman I'm a Dynamo DB Solutions
architect I've been with AWS for just
under 7 years um primarily focusing on
the no SQL Technologies uh some of the
customers that we see you know have use
cases where they want to combine uh
purpose build Solutions purpose build
data bases right it's it's all aimed at
using the right tool for the right job
so for example they'll have Dynamo DB
stored for their olp use case right that
high uh high high throughput low latency
access but they also need a lot of
flexibility for certain you parts of the
the data access so maybe think about uh
the Amazon retail store right we like we
like to eat our own doc food so think
about the Amazon retail store you go on
the website uh there is a search bar at
the top uh you start adding you know uh
start start typing for products so that
could be pillows that could be laptops
so and so forth and as in when you're
typing uh you see an auto complete
already right uh that cannot be powered
by Dynamo DB unfortunately but there is
a purpose buil solution for that which
is open search right so open search can
do your your fuzzy searching your
analytics your uh you know anomaly
detection your vector store so semantic
searches and so on and so forth so it is
the right tool for that particular job
so we see customers using both of these
stores together uh in cases where they
need the the fuzzy searching the rich
Tech search or the semantic search
capabilities but they also need the high
throughput loow latency access and this
is also something that we use on the am
Amazon retail store uh similar to uh you
know the services uh the the other
example where you know I've seen this
being used is let's say you're a retail
store right and you have customer
service uh on the desk in in the shop
shops there uh and they want to look at
customer profiles they want to fetch a
profile based on a customer standing in
the queue so the customer could give any
information maybe their first name last
name their contact information their you
know uh their address or what have you
and there may be mistakes in you know
the spellings and so on and so forth
that's where you need open search uh
because thenb will do a a string
matching basically uh but you if you
need those Rich Text uh search
capabilities that that's where open
search comes into the
picture so because we've seen customers
build this sort of pipeline where they
keep the data in sync between dyo DV and
open search uh you know we we saw that
and based on that there there's a
certain amount of effort that's required
in order to you know make it
possible so this is sort of the pipeline
that you know uh customers need to build
uh and I've built that myself so it's
it's really a lot of work uh if you look
at it I I'll start with number one so U
when you're first seeding your open
search server uh be it a cluster or a
serverless domain uh you basically need
to take an export right a full data dump
of your dyb data uh put that into S3 and
then write an application that reads
from that S3 data and seeds your open
search service be it a cluster or a
serverless domain right uh with that you
know you have taken an initial dump but
what happens to the data that's incoming
while you're doing that uh dump and and
load process so for that you rely on
dynamodb streams which is number four
there dynamodb streams is a change data
capture stream of every change that
happens on the table so you see a record
of what data changed how it changed uh
and you know what is the target state of
the data so that can be consumed by a
Lambda function and that Lambda function
can basically have the business logic uh
and the the code to basically ingest
that data into the open search domain or
cluster so maybe if there's there's an
insert there is an update there is a
delete of a record in d modb uh that
data needs to make its way through to
open search right so think about a
product use case a product catalog for
the retail website where all the
products are actually stored in dyn DB
but you need to support that top search
capability right and so you need open
search to power that but every time a
product description changes or certain
things in the product change uh those
changes are made into Dynamo DB table
but you need that uh to replicate into
the open search service right so this is
the whole process that goes behind
building such a data
pipeline now clearly this is a lot of
undifferentiated heavy lifting and this
is the word that you you know take home
for the day uh because we've said it so
many times uh and so the Z ATL
integration uh between dynamod and open
search uh leverages an open source uh
secure serverless uh technology called
Data prepper and data prepper is
basically uh you know it's not something
that's newly built it's been there for
years uh we're running something around
uh
2.7.0 release now so we've we' you know
it's it's out there it's open source uh
it's secure uh and it's also serverless
and so this is doing the ETL for you
where you do not need to think about the
initial data dump the export to S3 the
the chain data capture the Lambda
function and all that that goes behind
doing
it so all of that basically unref heavy
lifting uh translates into just writing
a yaml template right this is the z8l
integration between dynamodb and open
search so I'll break if if you're not
able to read it I'll break that down
into the e t and L uh phases right but
uh as a whole this is basically 26 lines
of a template that will do the Z ATL for
me the first part is the is the
extraction which is you know taking the
data from The Source uh which is your
Dynamo DB table so here you know I pass
the dyb uh table Arn or resource name uh
and I provide uh an S3 location where
the Zer ATL integration itself will do
the initial data dump right uh and I can
I can secure it with a particular role
tie to a particular role and have uh
permissions granted as per leas
privilege
principle the second part is the trans
formation so this is where I I can add
certain transformation uh functions
right the whole list is on the right uh
and basically what I'm doing here is I'm
using two individual attributes which is
user latitude and user longitude and
combining that into a location attribute
that the data prepper will add on my
behalf in real time right so you can do
a lot of stuff with anomaly detection
you can aggregate uh you can compress or
you can you can add new
uh data in it right uh and if you want
to uh vectorize it vectorize the data uh
you can also use a Bedrock connector on
your open search uh server or cluster uh
to basically vectorize that data as it
is coming
in the final part is the the load right
and this is where I provide a the target
uh open source domain or cluster
depending on where you're doing cluster
based or serverless uh and again uh I
can provide information about the index
where the data should go to I can
provide routes based on you know based
on certain characteristics within the
data which particular index should that
data end up in and it's not just one
cluster or domain that I can Target I
can Target multiple uh clusters of open
search to to index that data right uh
and one of the key things over here is
the document ID now this is the unique
identifier of the data in open search uh
and over here the the example uses a
primary key which is basically the
partition key and the sort key of
dynamod DB that you uniquely identify
each and every record right so uh
because we are also thinking about
keeping the two data stores in sync so
whenever a change happens in the product
in the Dynamo DB data uh it needs to be
propagated onto open search site so the
same product ID should reflect the new
change that happened right so for that
we need the same unique identifier as we
did in dynamodb so for that matter uh we
can just automate that process of
creating a document ID uh by leveraging
the the the metadata in open search so
when we when we set it up like this data
prepper goes to dynamodb gets the
partition key and sort key attribute
names all the column names combines that
and that becomes your unique identifier
for the document ID in open
search now all of that undifferentiated
heavy lifting or the whole architecture
becomes much more simpler with just the
Z ADL
integration so not only uh open search
but we see customers also you know
obviously leveraging the likes of red
shift for their analytics use cases so a
lot of times again data Engineers uh
would need to set up pipelines where
they export data from Dynamo DB uh put
that into you know run some
Transformations on it put that into to
Red shift and then run their reports or
their uh you know analysis pipelines
basically so for that uh We've launched
a uh a Dynamo db2 red shift 08l
integration as well this is currently in
preview mind you so if there are certain
things that you know uh you see that
maybe they're not great let us know we
we want to hear feedback so basically
the thing that powers the 08l
integration between DB and and red shift
are incremental exports by by dynamodb
so dynamodb supports incremental exports
which is basically you provide a start
time and an end time and it will return
you all the data that changed between
those times right so if you need the
Delta of changes you can call it as many
times you like and you're only charged
for the amount of data that's uh shared
with you not the you know the whole data
dump or the whole table data size so
what the Z ATL integration does here it
it takes uh an Inc export roughly every
15 minutes and then uses that output uh
to power uh to ingest into red shift so
you decide a red shift cluster or
serverless uh you know resource and it
will do the the syncing between dynamodb
and red
shift now uh this is uh the target table
that's created for my integration uh
that was running so it was an e-commerce
uh table because you know Amazon uh and
so the schema of that that Target table
is basically a partition key name a sort
key name and a value uh column so uh you
know because dmod is schema less and uh
you know it's a no SQL database uh the
only mandatory parts of your record in
Dynamo DB are the partition key and the
sort key or the primary key uh as a
whole right so a single item in the Dy
table can have let's say five columns or
five attributes the the next item in the
same table could have 200 so because of
the schema less nature uh it's sort of
uh tricky to you know map that in real
time into a schema uh enforcer enforcing
system such as red shift so the way it
it works uh is that we decide a a
primary key uh same as the Dynamo DB
table in in red shift so there's a
partition key my partition key name was
PK my sord key name was SK and then
there is a a raw Json value in the in
the third column that's the value uh
column itself so on the right is
something that uh you know this is how
the data would appear uh in in red shift
now it is up to you to either use the
same table that gets created
automatically uh into your reporting
pipelines in your reporting queries
where you need to probably uh map the
value Json attribute as per your uh you
know analytical queries or you move this
data from this particular red shift
table to your production table and
sanitize it while you're doing it right
so that's the work the data is already
there in Tad shift you either decide to
update your queries to you know reflect
to point to this particular table or you
the table that you're already using you
basically move data from this table to
that
one in terms of my test uh and you know
again your mileage May vary uh but
basically I was loading the same
e-commerce data uh randomized into uh
into dynamodb which was making its way
onto red shift and so there was a the
the cloud metrics offered by this
integration uh are basically include a
lag between the time data was ingested
into red shift and the time uh it is
available for quering uh which was
roughly about 20 25 minutes so on on the
left you see the the lag there which is
pretty good if you think about you know
your analy IAL database right your data
warehouse basically uh and on the right
side is the data transfer the raw bites
transferred uh so there there are many
more cloudwatch metrics not only for the
red shift integration but also for the
open search integration which give you
an idea of what was the time it took end
to end uh and that can help you you know
estimate whether it's good for you
whether you need it to be fast whether
it's too quick uh well nobody uh hates
uh things being too quick uh but
anyway so I want to I want you to take
away from this session uh the
capabilities of Z ATL right if you're
data Engineers you're already uh you
maintaining data pipelines uh there's a
lot of effort that goes behind uh
maintaining the code setting up the
infrastructure uh maintaining that
infrastructure and maintaining the code
again so and and making it bug free is
almost impossible right all of those
things get taken away with the Zer ATL
Integrations that that are being
supported by for purpose buil databases
uh and so this can allow you low latency
High throughput scalable uh sort of
setup between your data stores uh where
you can root the use the right tool for
the right job but also extract insights
in near real
time so these are the the Integrations
you know that I want to put up again for
you to take uh uh grasp it for a few
seconds uh these are the Integrations
that are supported uh I I did not cover
open source to S3 but basically in the
same uh yaml template that we saw we can
also add an S3 bucket where open search
data will be uh dumped
into and finally uh this is not the only
set of Integrations that are available
uh well not will be available uh AWS is
investing in a Z ATL future heavily
right so if there's any other
Integrations that you uh you know today
set up data pipelines for uh let us know
we we're we're already uh in progress uh
there's there's a number of projects in
progress that are integrating other
database or other data sources uh but
the whole idea is that z8l is not going
anywhere it's only getting started uh
you know feel free to reach out to us
after the session or your AWS account
teams if there are certain requests that
you have about Z ATL Integrations about
your data stores uh and let us know we'd
love to
learn with that I'd like to thank you uh
for being here with uh with us here
today uh and I would appreciate if you
let us know how we how we did with the
feedback thank you
Browse More Related Video
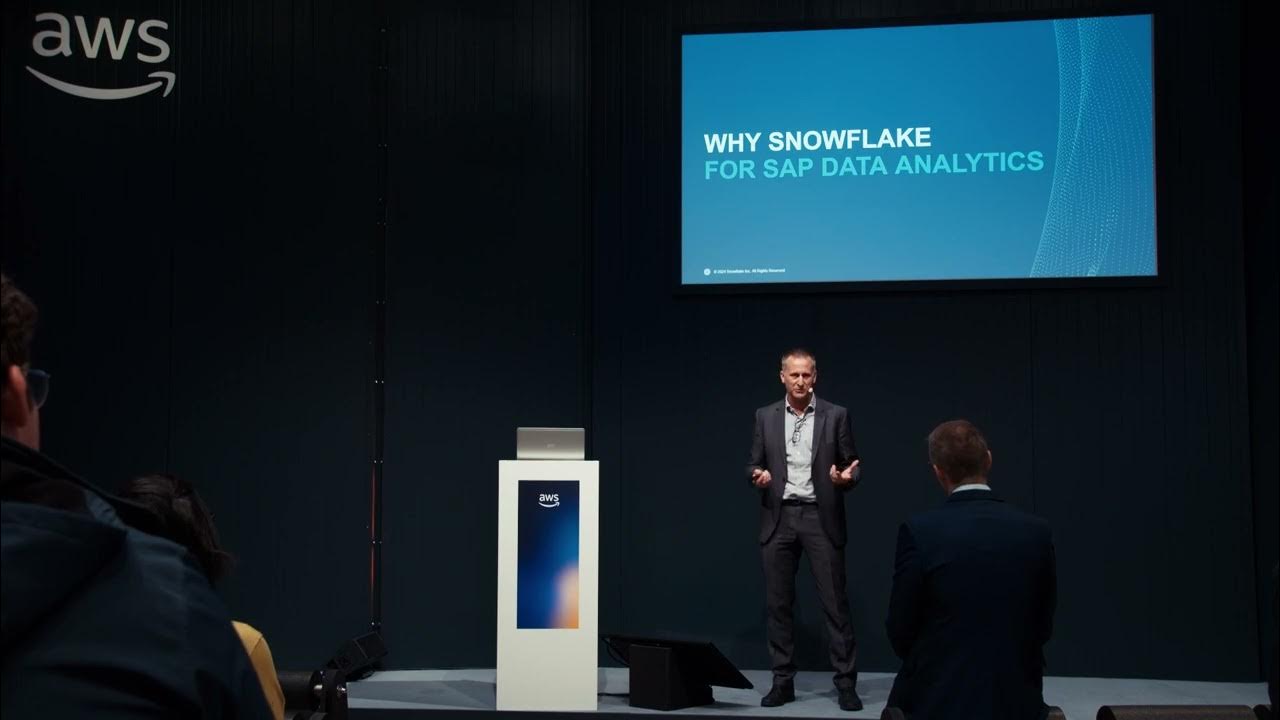
Hannover Messe 2024: Generate business value from your SAP data

How to use generative AI & Amazon Security Lake for threat analysis | AWS OnAir-S05
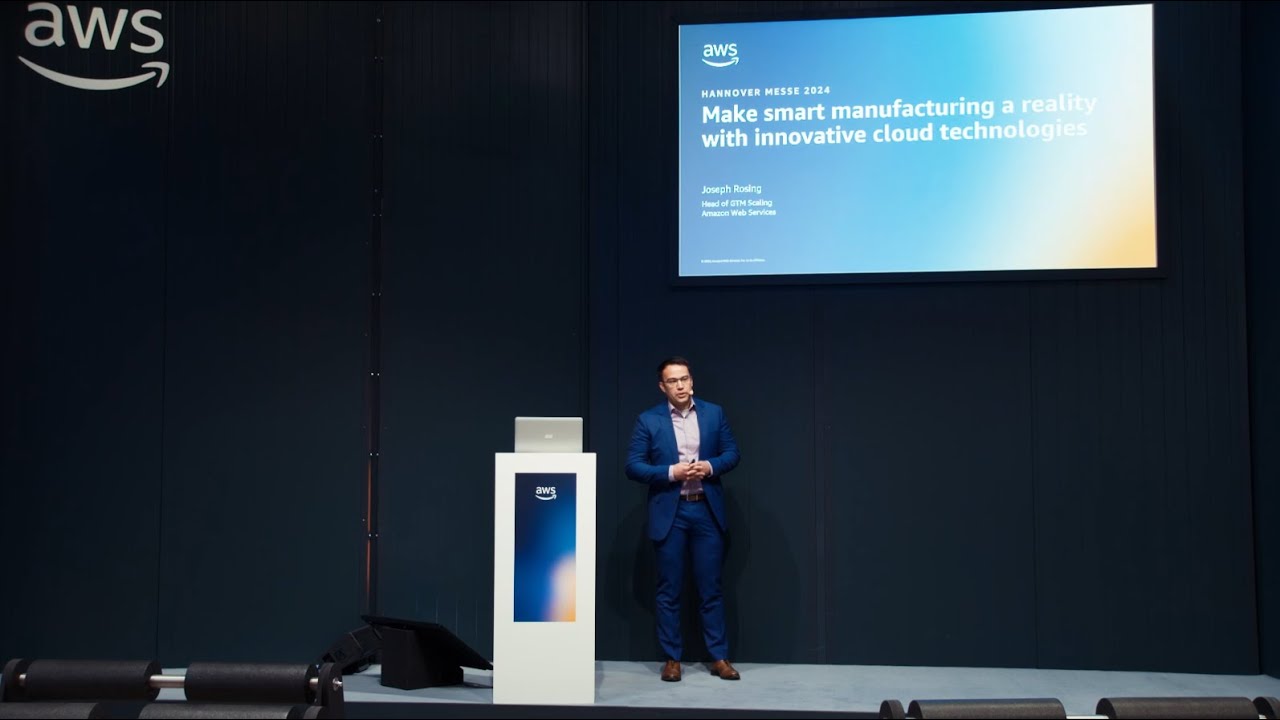
Hannover Messe 2024: Make smart manufacturing a reality with innovative cloud technologies
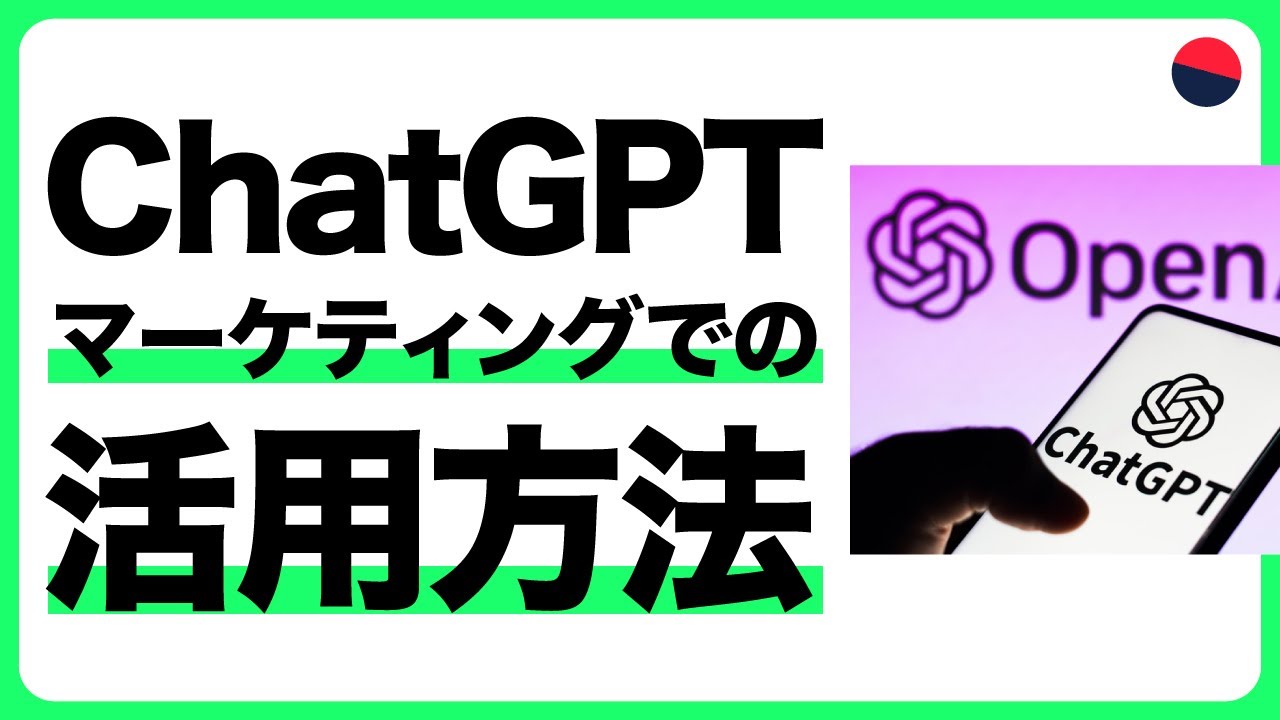
【ChatGPT活用術】SEO対策もサイト制作もデータ分析も、全て出来る使い方(GPT-4o)
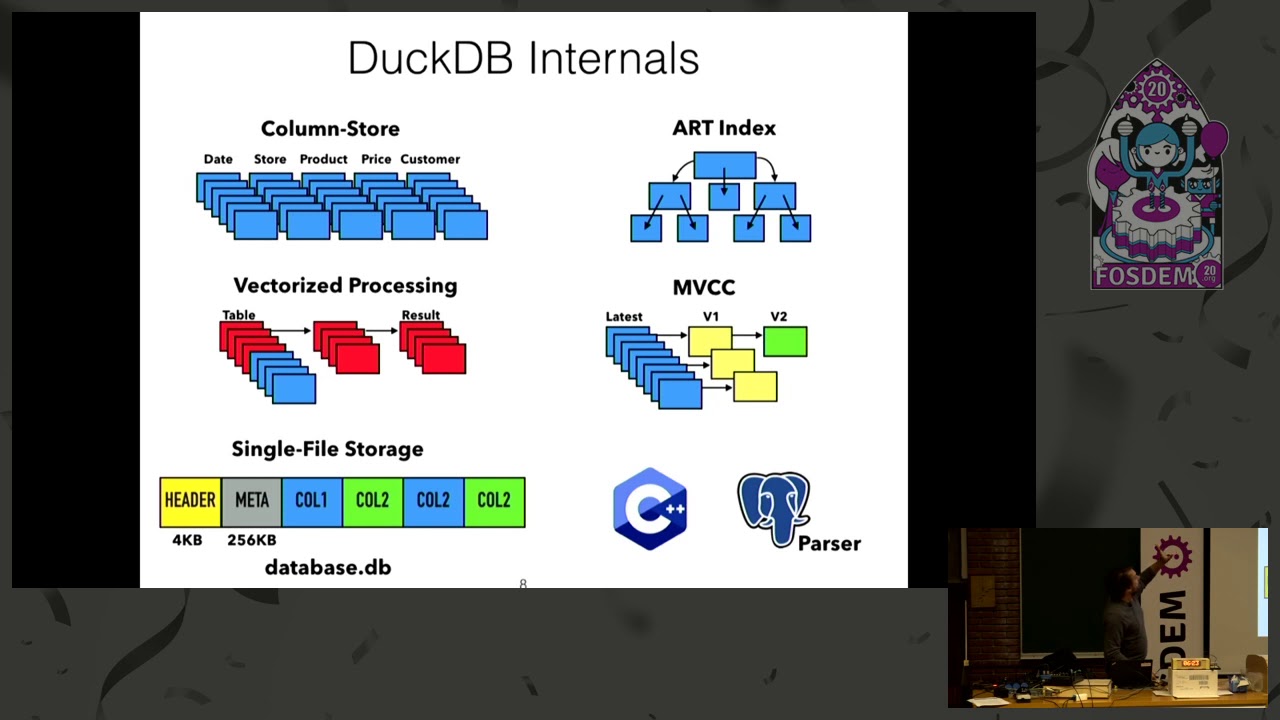
DuckDB An Embeddable Analytical Database
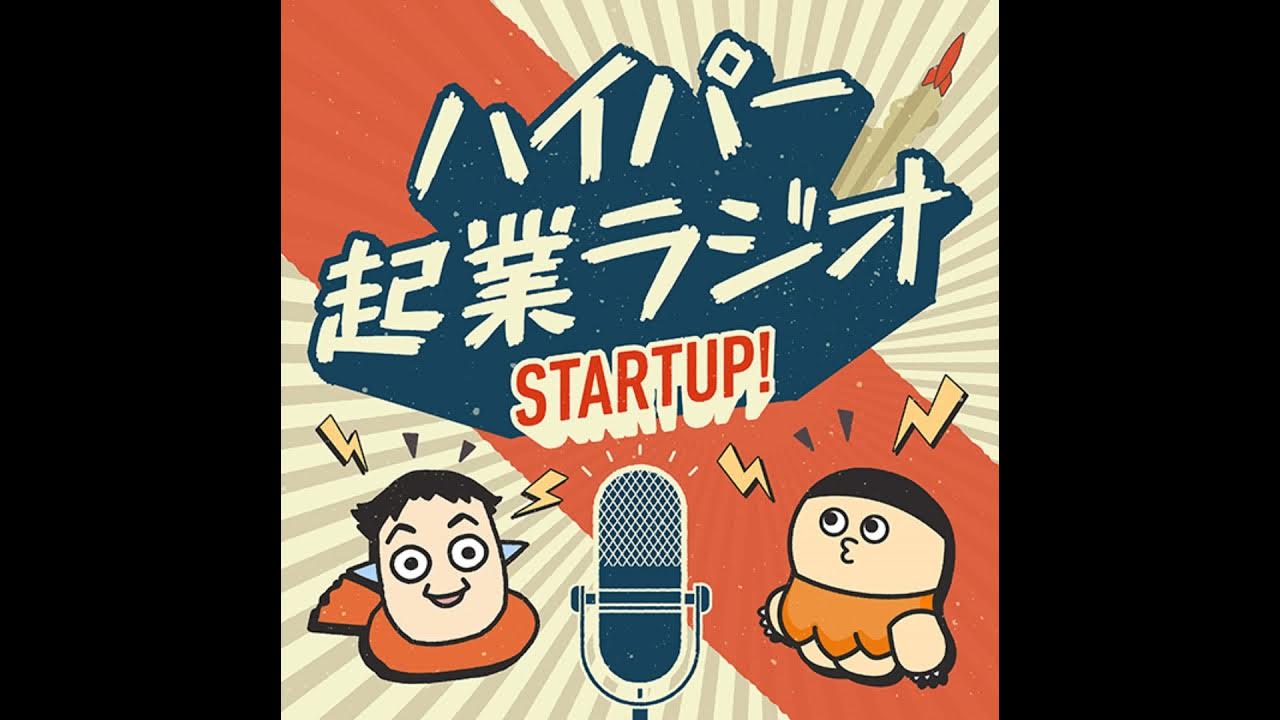
[番外編 #02] ペルソナ、ちゃんと使えてる?番外解説編(前編)
5.0 / 5 (0 votes)