How Google Translate Uses Math to Understand 134 Languages | WSJ Tech Behind
Summary
TLDRGoogle翻訳は過去20年間で技術的に完全に刷新され、130以上の言語に対応する洗練されたニューラルネットワークへと発展しました。言語をコンピュータが理解できる数学に変換し、単語に数値を割り当てます。これは単語間の関係を示すベクトルの数学的処理に基づいており、特にtransformerモデルが重要な役割を果たします。このモデルは単語をベクトルに変換し、それらを行列として扱い、単語間の関係を数学的に処理します。翻訳の精度を高めるためには、膨大な学習データから得られた機械学習が不可欠です。さらに、Googleの光学文字認識(OCR)技術もtransformerを用いて、画像からのテキスト抽出を精度を向上させています。しかし、言葉のコンテキストを完全に捉えることや、変則な物体表面の文字認識にはまだ課題があります。Googleは翻訳の微調整やさらなる言語の追加を目指しています。
Takeaways
- 🌐 Google翻訳は過去20年間で技術的に完全に刷新され、130以上の言語を扱う洗練された神経ネットワークへと発展しました。
- 🔍 Google翻訳は言語をコンピュータが理解できる数学に変換する働き方をしています。
- 📈 単語に数値を割り当て、意味を表すベクターを作成することで、コンピュータは単語間の関係を数学的に表現できます。
- 🧩 トランスフォーマーモデルは現代のAIの基礎であり、言語を数学に変える重要な役割を果たしています。
- 🔄 トランスフォーマーは単語の意味だけでなく、単語間の関係の重要性についても数学的に扱うことができます。
- 📊 Google翻訳は単語をベクターに変換し、それらを大きな表(行列)にまとめ、単語間の相互作用を数学的に解明します。
- 🤖 モデルは学習を通じて、数十億の例から得られた数値を調整し、最適な翻訳結果を生み出します。
- 🌐 翻訳のテストは全てを網羅することは不可能ですが、AIと専門家の翻訳者が正確性をチェックします。
- 🖼 Google Lensは光学文字認識(OCR)を使用して、画像からのテキストを抽出し翻訳します。
- 🎨 Google Lensは生成モデルを用いて、翻訳されたテキストを自然に見えるように背景に重ね合わせる処理を行います。
- 🌐 Googleはより多くの言語をサポートし、ユーザーが翻訳を調整できる機能を追加することを目指しています。
Q & A
Google翻訳はどのくらいの言語をサポートしていますか?
-Google翻訳は、130以上の言語をサポートしています。
Google翻訳の技術は過去20年間でどのように進化しましたか?
-過去20年間で、Google翻訳は基本的なパターンマッチングツールから、130以上の言語を扱う洗練された神経ネットワークへと進化しました。
Google翻訳はどのようにして言語をコンピュータが理解できる数学に変換するのですか?
-Google翻訳は、言語を数学に変換するために、単語に数値を割り当てます。これにより、コンピュータは単語の意味に関する数学を実行できます。
Google翻訳におけるトランスフォーマーモデルとは何ですか?
-トランスフォーマーモデルは、現代のすべてのAIのビルディングブロックであり、言語を数学に変換するプロセスにおいて重要な役割を果たします。
単語を数値として表現する際に重要なポイントは何ですか?
-単語に割り当てられた特定の数値は重要ではありませんが、重要なのは各単語が他の単語とどのように関連しているかです。
トランスフォーマーアーキテクチャはどのように翻訳を支援しますか?
-トランスフォーマーアーキテクチャは、単語の意味を表すだけでなく、単語同士の関係の重要性を数学的に扱うためのテーブルを作成し、そのテーブル全体に対して数学を実行します。
Google翻訳で翻訳する際にコンピュータはどのようにして単語の相互作用を理解するのですか?
-コンピュータは単語をベクトルに変換し、それらのベクトルを大きなテーブルまたは行列に入れ、各単語が他の単語とどのように相互作用するかを数学的に理解しようとします。
コンテキストベクトルとは何ですか?
-コンテキストベクトルは、単語の意味だけでなく、文全体の意味を表す数字のリストです。モデルが適切に機能している場合、文の単語の合計ではなく、その意味を表すものです。
光学文字認識(OCR)とは何であり、Google翻訳でどのように使われますか?
-光学文字認識(OCR)は、画像からテキストを抽出する技術であり、Google翻訳では外国語のスクリプトを入力できない多くの人々にとって情報をよりアクセス可能にします。
Google Lensはどのようにして文字を認識し、翻訳するのですか?
-Google Lensは、テキストの行とテキストの方向を特定し、その後特定の文字と単語を決定します。画像を単語に分割する代わりに、ピクセルのパッチ、つまりトークンに分割します。
翻訳の精度をどのようにテストし、保証していますか?
-翻訳の精度をテストするために、多くの数字と重みがランダムに選択され、コンピュータが数十億の例から学習し、その値を微調整します。デプロイ前に、エンジニアはAIの評価者と専門の人間翻訳者によって多数のテストを実行します。
Google翻訳はどのようにして2つの非英語言語間の翻訳を扱うのですか?
-モデルは英語との翻訳にトレーニングされているため、2つの非英語言語間の翻訳では、英語を経由する必要があります。例えば、日本語からズール語への翻訳では、日本語から英語へ、そして英語からズール語へ変換されます。
Google翻訳はどのようにして誤った翻訳を避けようとしていますか?
-Googleはユーザーが翻訳を微調整できる機能を追加し、翻訳をよりフォーマルまたはカジュアルにしたり、チリのスペイン語ではなくヨーロッパのスペイン語にしたりするなどのオプションを提供しています。
Google翻訳はどのくらいの言語をサポートすることを目指していますか?
-世界には推定される6,000から7,000の言語があり、Google翻訳の目標はそれらすべてをサポートすることです。
Outlines
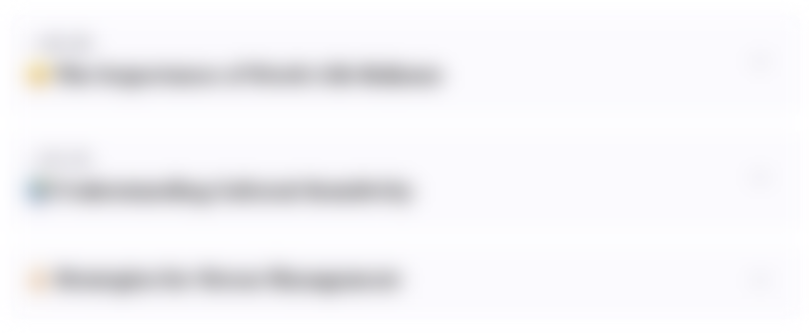
This section is available to paid users only. Please upgrade to access this part.
Upgrade NowMindmap
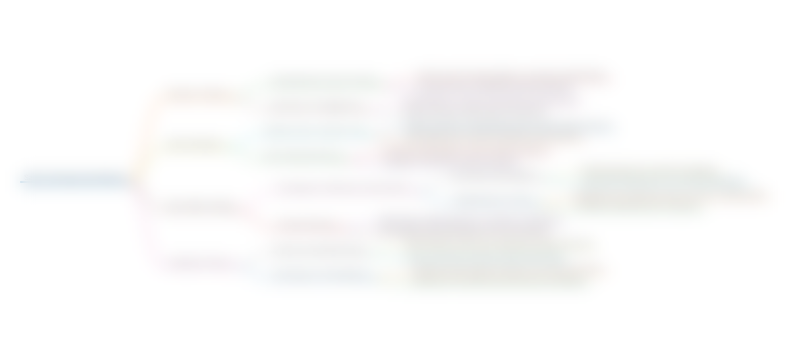
This section is available to paid users only. Please upgrade to access this part.
Upgrade NowKeywords
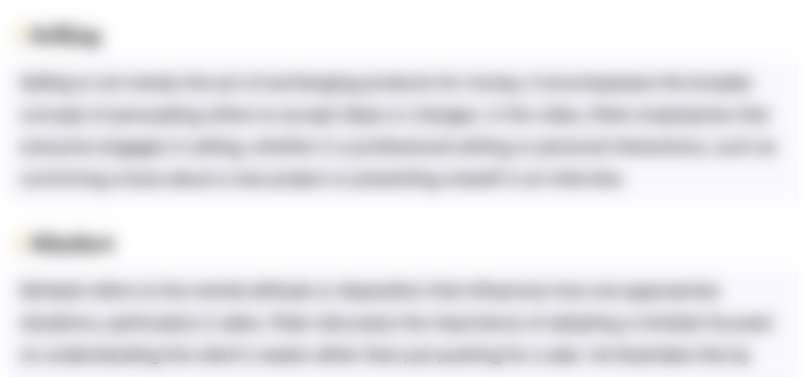
This section is available to paid users only. Please upgrade to access this part.
Upgrade NowHighlights
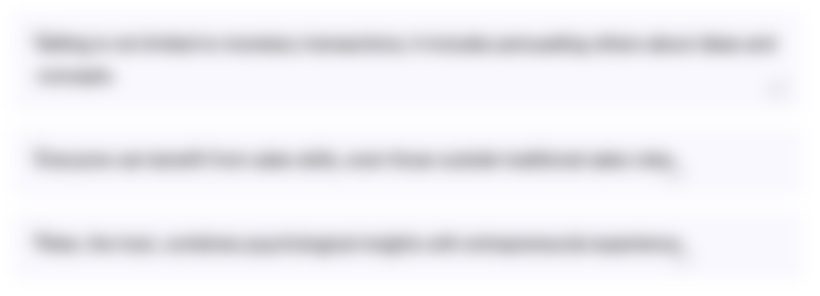
This section is available to paid users only. Please upgrade to access this part.
Upgrade NowTranscripts
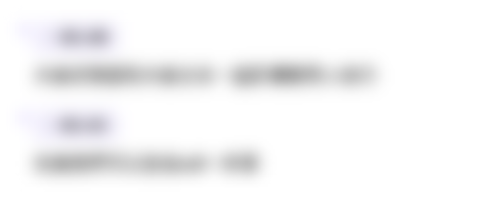
This section is available to paid users only. Please upgrade to access this part.
Upgrade NowBrowse More Related Video
5.0 / 5 (0 votes)