Beer and Diaper case study | Data Analytics in retail | Data Mining | SQL | Business Intelligence
Summary
TLDRThis video explores the intriguing correlation between beer and diaper sales, first discovered in the early 1990s. The case study demonstrates how data mining, or querying, can uncover unexpected patterns in consumer behavior, like young men buying beer and diapers together on Friday afternoons. The story also highlights the differences between business intelligence and advanced analytics, showing that while correlations can be insightful, they must be validated and applied in context. Ultimately, the case emphasizes the evolving role of data analytics in retail, from improving product placement to optimizing inventory and marketing strategies.
Takeaways
- ๐ The beer and diapers correlation was discovered in the early 90s by Karen Heath and her team, who used data queries to identify patterns in retail sales data.
- ๐ค The unexpected correlation between beer and diapers, found on Friday afternoons, surprised many and quickly became a famous example of data analysis.
- ๐ป Data mining involves using algorithms to find patterns in data without preconceived ideas, while querying starts with a hypothesis that is tested against the data.
- ๐ Retailers used insights from data analysis to enhance product placement and improve sales, such as putting diapers and beer next to each other on shelves.
- ๐ The original beer and diapers story illustrates the potential power of data mining but also highlights how correlations might not always be meaningful or applicable universally.
- ๐ก The story emphasizes that businesses need to validate correlations within the specific context of their own data and environment to ensure insights are actionable.
- ๐๏ธ Retailers started using loyalty card and point-of-sale data to gain deeper insights into customer behavior, leading to more effective marketing and stocking strategies.
- โ๏ธ The story about Osco Drug illustrates that removing slow-moving products from shelves can improve consumer perceptions of product availability and store selection.
- ๐ In 1998, IBM aired a commercial using the beer and diapers example, showing how the story had permeated popular culture and was being used to sell the idea of advanced data analytics.
- ๐ Even when a correlation is found, it can be impacted by external factors like cross-promotion, which can distort the relationship between products in the data.
- ๐ค The beer and diapers case shows the difference between simple business intelligence and advanced analytics, where models and context are key to understanding and applying data insights.
Q & A
What is the main topic of the case study in the video?
-The case study explores the unexpected correlation between beer and diaper sales, and how this story highlights the power of data analysis and data mining in retail business decisions.
Who was responsible for discovering the correlation between beer and diaper sales?
-Karen Heath, an industry consultant with Teradata, discovered the correlation between beer and diaper sales in 1992 while analyzing retail sales data.
What data was combined to uncover the beer and diaper correlation?
-Walmart combined demographic data from its loyalty card system with point-of-sale system data to uncover correlations in customer purchasing behavior, including the beer and diaper connection.
What did Karen Heath and her team use to uncover the correlation between beer and diapers?
-Karen Heath and her team used SQL queries against the retailer's data warehouse to find products that were frequently bought together, including beer and diapers.
Why did the beer and diaper correlation become famous?
-The beer and diaper correlation became famous because it was an unexpected finding. The connection between these two products seemed counterintuitive and was an example of how data mining can reveal surprising insights.
What is the difference between querying data and data mining, as explained in the video?
-Querying data involves asking specific questions or testing hypotheses, whereas data mining is an automated process that seeks patterns or correlations in data without preconceived ideas or hypotheses.
Why was the beer and diaper story not a pure example of data mining?
-The beer and diaper correlation was discovered through simple querying of data, not by a data mining algorithm. Data mining involves looking for patterns without a specific hypothesis, which was not the case here.
What challenge did retailers face when trying to validate the beer and diaper correlation?
-Retailers faced the challenge of cross-promotion, where the correlation might have been influenced by the placement of beer and diapers next to each other on the shelves, making it difficult to determine if there was a true, underlying relationship.
What lesson does the beer and diaper case study teach about business intelligence?
-The case study highlights the importance of validating analytics models before applying them in real-world business settings. It also emphasizes the distinction between business intelligence, which is about analyzing existing data, and data mining, which seeks to uncover unknown patterns.
How has predictive analytics improved retail operations compared to the 1990s?
-Today, predictive analytics enables retailers to better understand consumer buying behavior, optimize store layouts, manage inventory more efficiently, and offer targeted promotions, which significantly improves sales and the customer experience.
Outlines
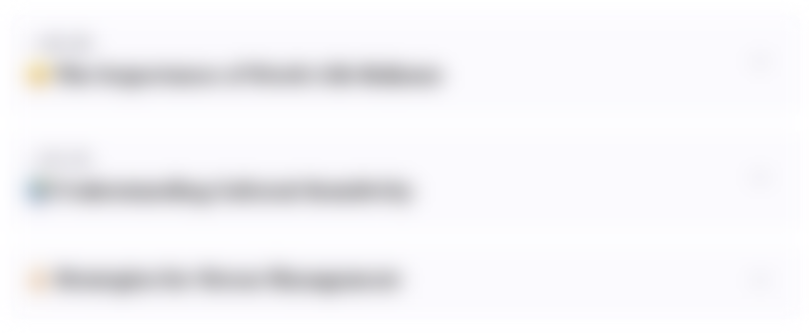
This section is available to paid users only. Please upgrade to access this part.
Upgrade NowMindmap
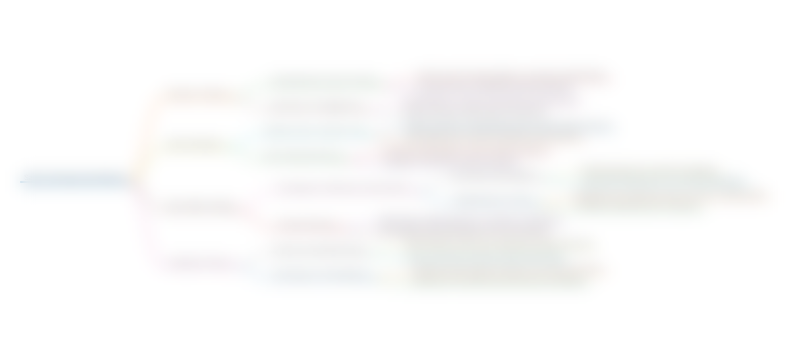
This section is available to paid users only. Please upgrade to access this part.
Upgrade NowKeywords
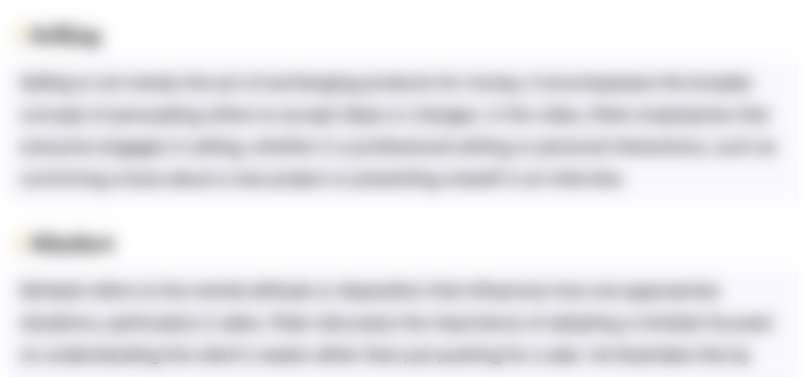
This section is available to paid users only. Please upgrade to access this part.
Upgrade NowHighlights
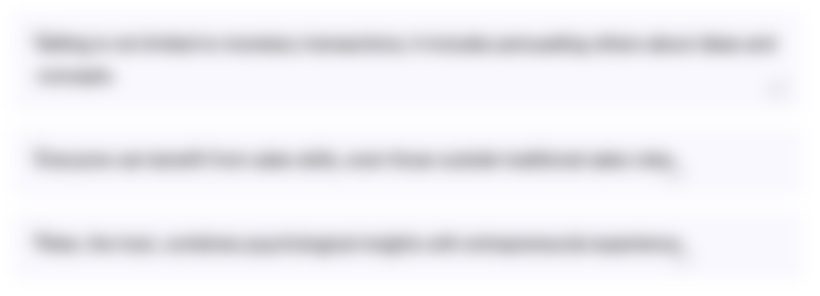
This section is available to paid users only. Please upgrade to access this part.
Upgrade NowTranscripts
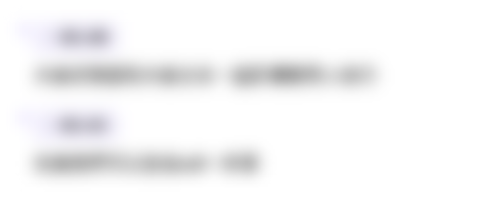
This section is available to paid users only. Please upgrade to access this part.
Upgrade NowBrowse More Related Video
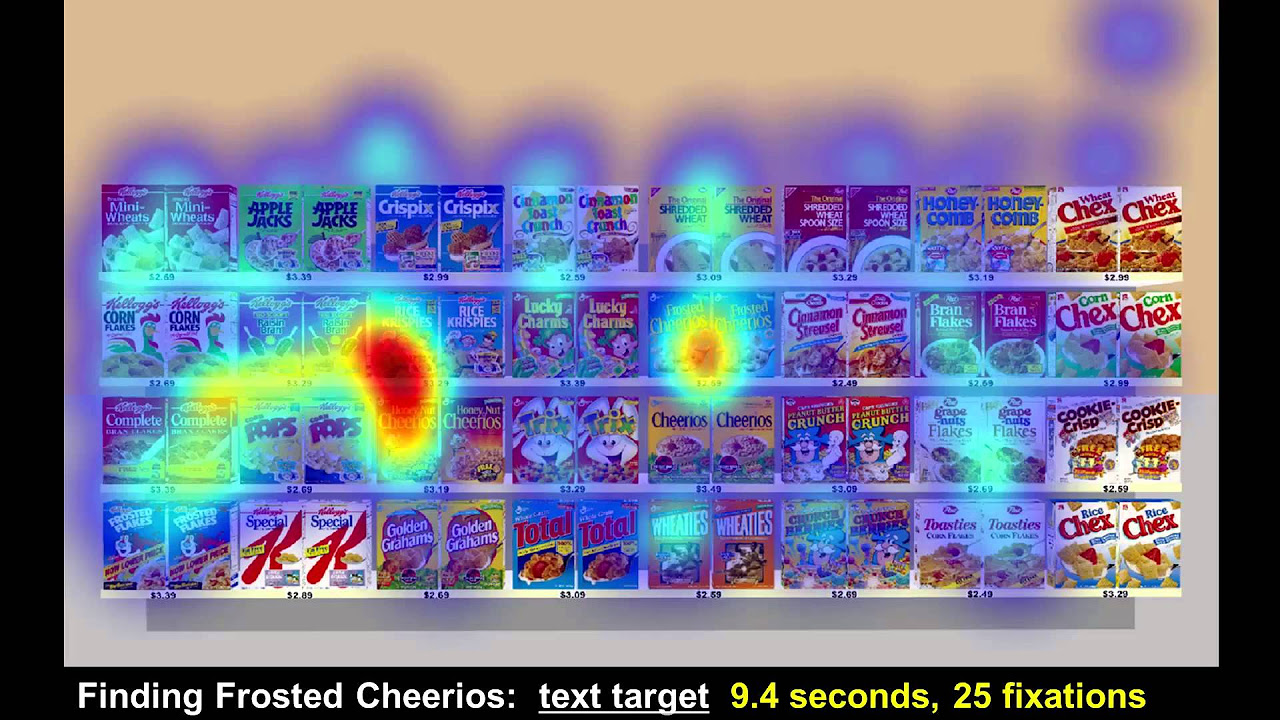
How stores track your shopping behavior | Ray Burke | TEDxIndianapolis
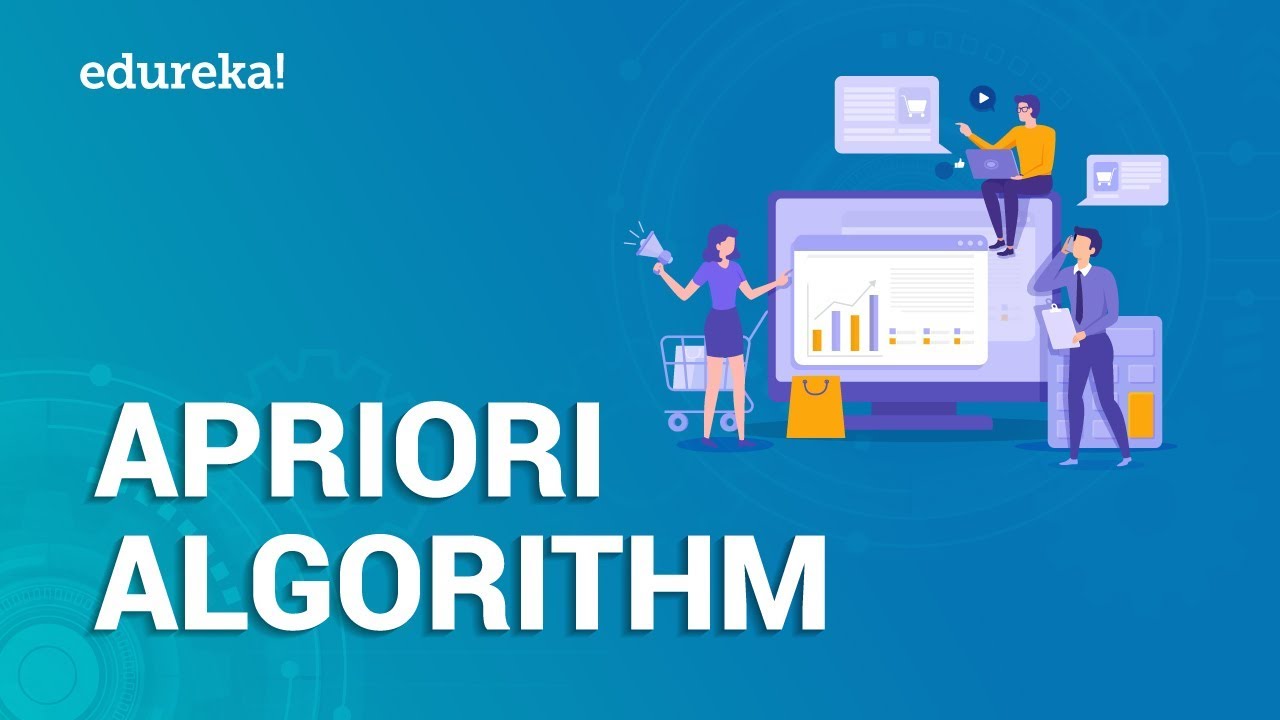
Apriori Algorithm Explained | Association Rule Mining | Finding Frequent Itemset | Edureka

Types of Business Analytics (Descriptive, Predictive, and Prescriptive)
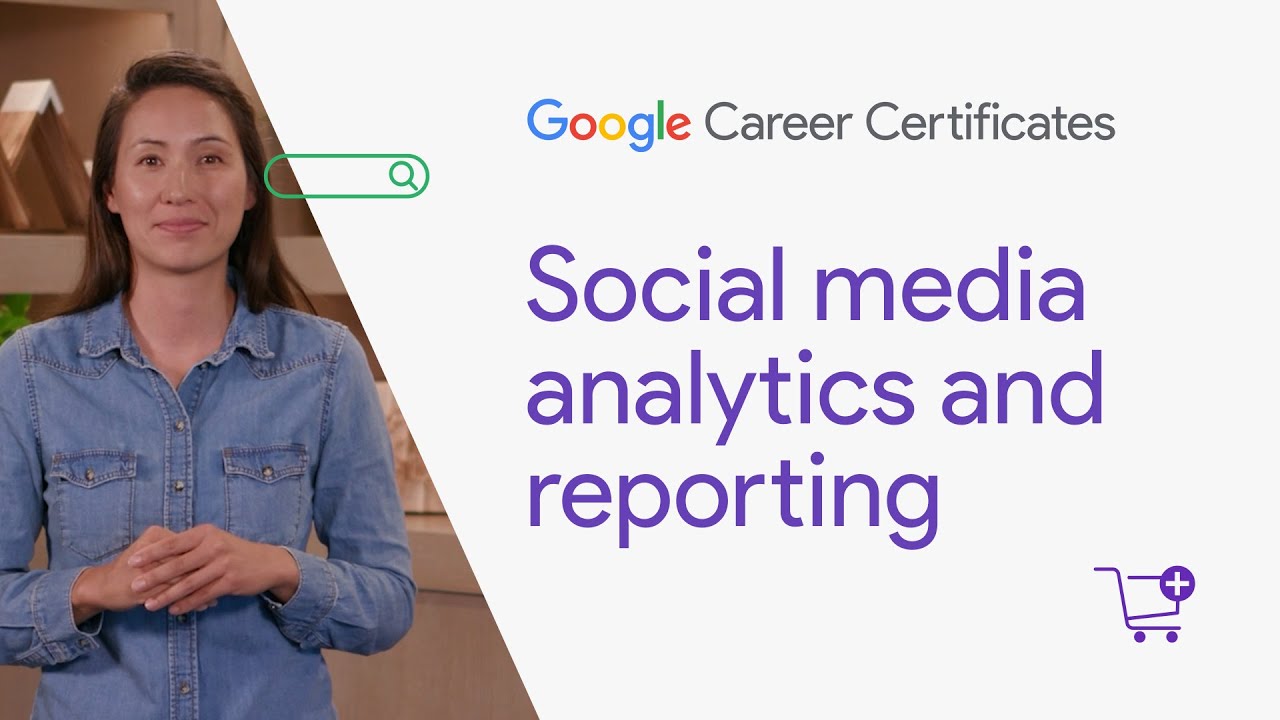
Social media analytics and reporting | Google Digital Marketing & E-commerce Certificate
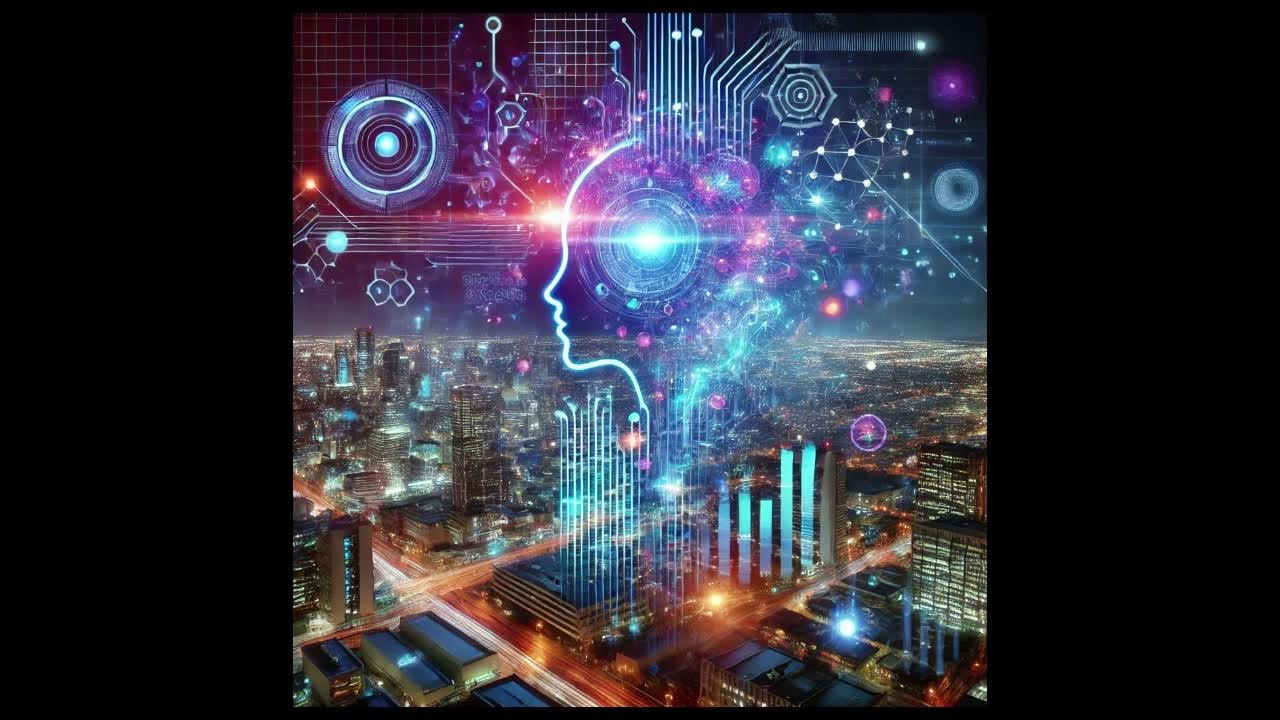
The Power of Big Data in Consumer Behavior

Numpy Boolean Arrays - Data Analysis with Python Course
5.0 / 5 (0 votes)