Generative AI - Driving Resilient Supply Chains
Summary
TLDR本次网络研讨会由C3 AI公司主办,介绍了其供应链套件和创新应用——C3生成式AI。C3 AI的供应链套件是一系列针对供应链规划和执行的企业AI应用,涵盖从采购优化到需求预测、生产调度优化、库存优化和供应链风险管理等用例。特别引人注目的是C3供应链数字孪生技术,它整合了来自不同ERP系统和其他企业数据源的供应链数据,提供全面的供应链视图。C3生成式AI作为一个AI驱动的知识助手,允许用户通过自然语言查询访问企业信息,提高了决策效率并减少了数据隐私风险。演示部分展示了C3 AI如何帮助用户通过生成式AI快速获取供应链信息,并采取行动以缓解潜在问题。
Takeaways
- 🚂 数字化转型是企业生存的根本问题,关乎是否能够适应时代发展。
- 🛠️ C3 AI 提供了一整套端到端的企业AI应用,涵盖供应链规划和执行的各个方面。
- 🌐 C3 AI 的供应链数字孪生技术整合了ERP系统、企业数据源和外部数据,为供应链管理提供统一的数字视图。
- 🔮 C3 AI 利用生成式AI技术,帮助企业快速访问和分析大量企业信息,提高决策效率。
- 🛡️ C3 AI 的生成式AI架构通过知识嵌入和检索模型保护原始数据,避免了标准大型语言模型(LLM)的数据安全风险。
- 📊 C3 AI 应用提供可预测的软件套件,帮助企业在全球范围内实现细粒度的可见性,并应用不同类型的AI模型。
- 👥 C3 AI 为供应链中不同角色的用户提供完整的工作流程,包括需求规划师、库存经理、供应商关系经理和采购经理。
- 📈 C3 AI 供应链应用的客户包括航空航天、国防、石油和天然气、零售、消费品、制造业和医疗保健等行业的大型组织。
- 🔄 C3 AI 的生成式AI产品允许用户通过自然语言查询访问跨各种数据库和信息源的数据,提高用户效率。
- 📚 C3 AI 通过案例研究展示了其供应链解决方案如何帮助农业生产商优化供应链,提高准时交货率和服务水平。
- 🔑 C3 AI 通过提供访问控制,确保数据隐私,并防止敏感信息泄露给未经授权的用户或竞争对手。
Q & A
什么是C3 AI供应链套件的核心组成部分?
-C3 AI供应链套件的核心组成部分是C3供应链数字孪生(Digital Twin),它提供了对所有供应链操作的细粒度和时间历史记录,整合了来自不同ERP系统、企业数据源和外部数据源的数据。
C3 AI如何利用人工智能和自动化来改变世界?
-C3 AI通过使用包括AI在内的技术,使人们能够创造价值并为客户提供结果,从而利用科学和自动化的潜力来改变整个世界。
C3 AI供应链套件如何帮助企业提高效率?
-C3 AI供应链套件通过提供端到端的企业AI应用程序,涵盖从采购优化到需求预测、生产计划优化、库存优化以及供应链风险识别等多个方面,帮助企业提高效率。
C3 AI的供应链数字孪生如何处理不同ERP系统的数据?
-C3 AI的供应链数字孪生能够整合不同的ERP系统,无论是Oracle、SAP还是其他系统,将其统一到一个共同的数字视图中,为企业提供完整的供应链数据视图。
C3 AI如何确保数据的安全性和访问控制?
-C3 AI通过将大型语言模型(LLM)与数据检索模型分离,确保LLM无法直接访问原始数据,从而保护数据安全。此外,C3 AI还实施了企业级访问控制,确保用户只能访问其被授权的数据。
C3 AI的生成性AI与传统的LLM有何不同?
-C3 AI的生成性AI通过知识嵌入和检索模型来提供答案,而不是直接让LLM访问数据。这种方法提供了可追溯性、确定性的答案,并且没有信息泄露的风险。
C3 AI生成性AI如何帮助用户更有效地获取信息?
-C3 AI生成性AI作为一个AI驱动的知识助手,允许用户通过聊天或搜索形式提问,系统会将问题转换为查询,并从检索模型中获取数据,以人类可读的格式提供答案。
C3 AI生成性AI如何处理数据缺失的情况?
-如果查询的数据缺失,C3 AI的检索模型将返回没有数据的查询结果,并通过LLM告知用户没有数据或无法访问相关信息。
C3 AI生成性AI如何与现有的供应链解决方案集成?
-C3 AI生成性AI可以集成到现有的供应链解决方案中,通过查询和分析现有系统中的数据,为用户提供更深入的洞察和决策支持。
C3 AI的实施周期通常需要多长时间?
-C3 AI的实施通常从3到6个月的试点项目开始,在这段时间内,将完成数据集成、模型训练和用户工作流程配置,确保在项目结束时拥有一个生产就绪的应用。
Outlines
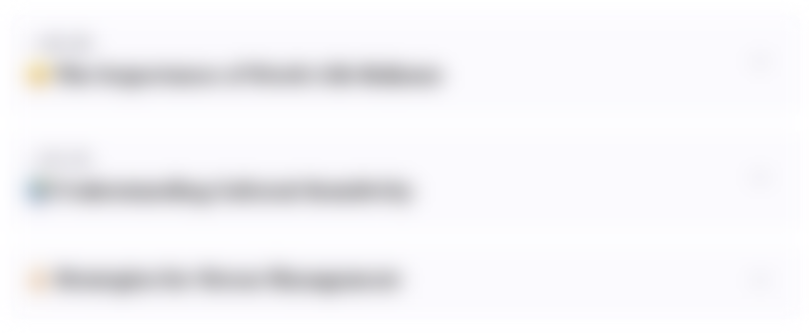
This section is available to paid users only. Please upgrade to access this part.
Upgrade NowMindmap
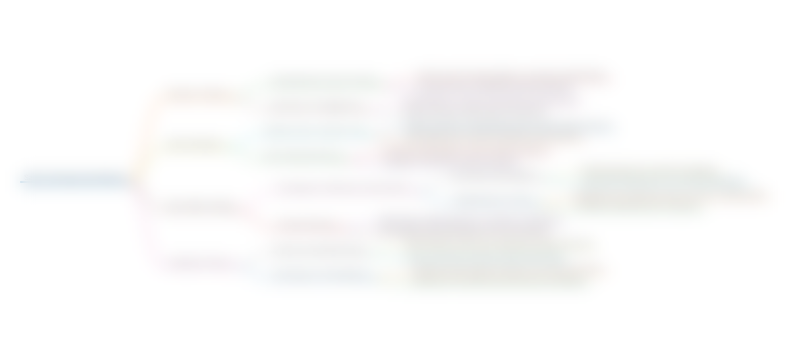
This section is available to paid users only. Please upgrade to access this part.
Upgrade NowKeywords
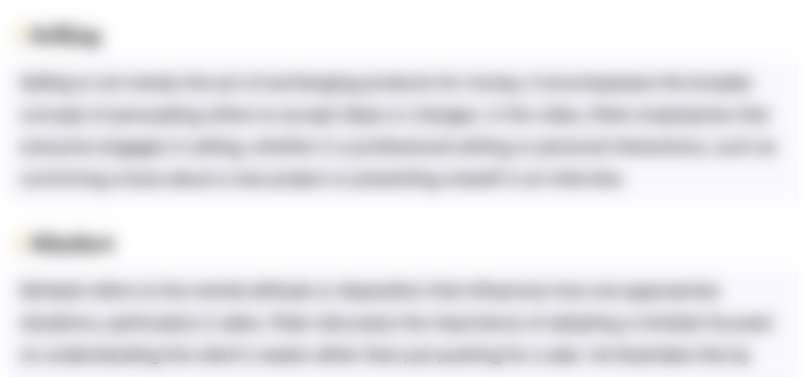
This section is available to paid users only. Please upgrade to access this part.
Upgrade NowHighlights
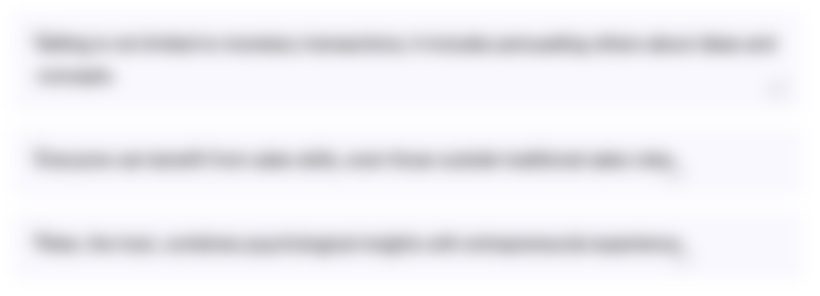
This section is available to paid users only. Please upgrade to access this part.
Upgrade NowTranscripts
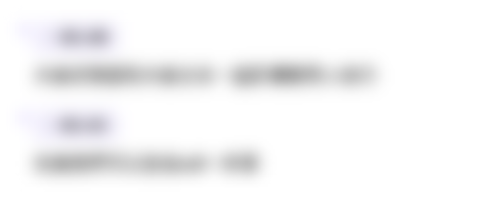
This section is available to paid users only. Please upgrade to access this part.
Upgrade NowBrowse More Related Video
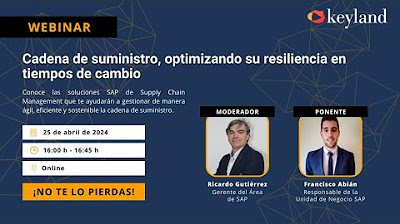
Webinar | Cadena de suministro, optimizando su resiliencia en tiempos de cambio

AI-Driven Supply Chains: 3 Cases | MIT SCALE Webinar | English
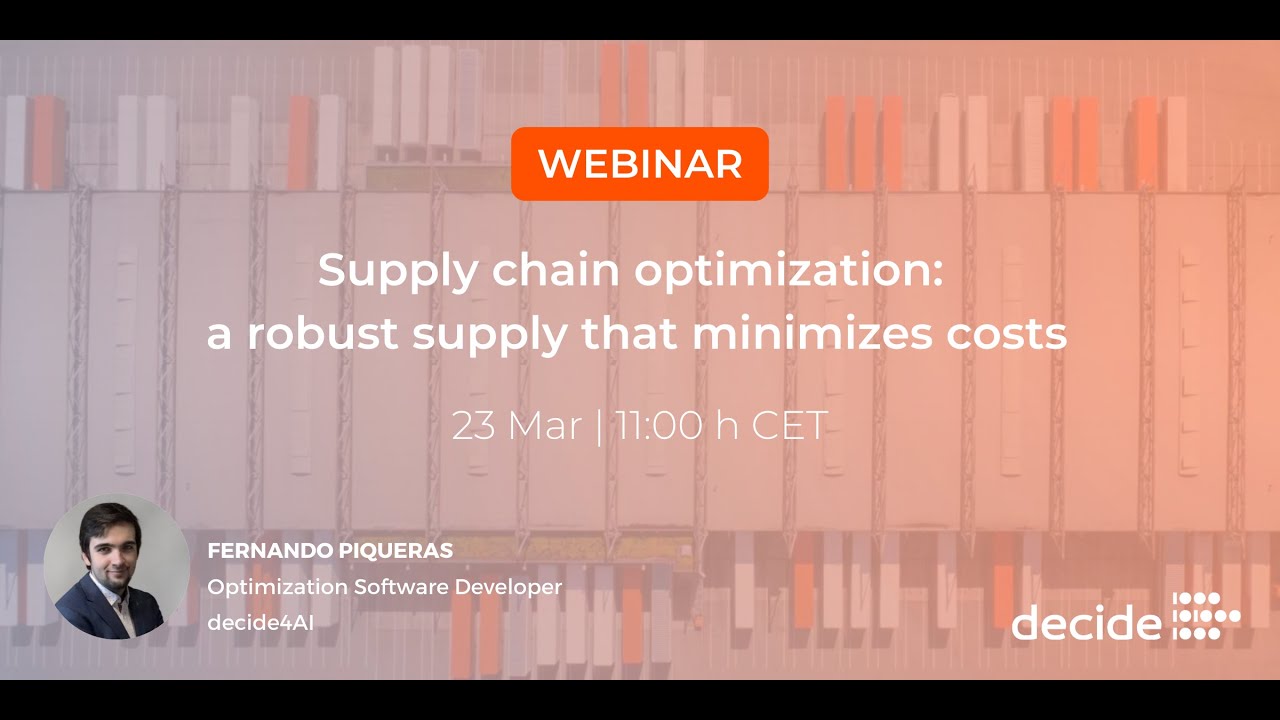
Webinar - Supply Chain Optimization: A Robust Supply That Minimizes Costs
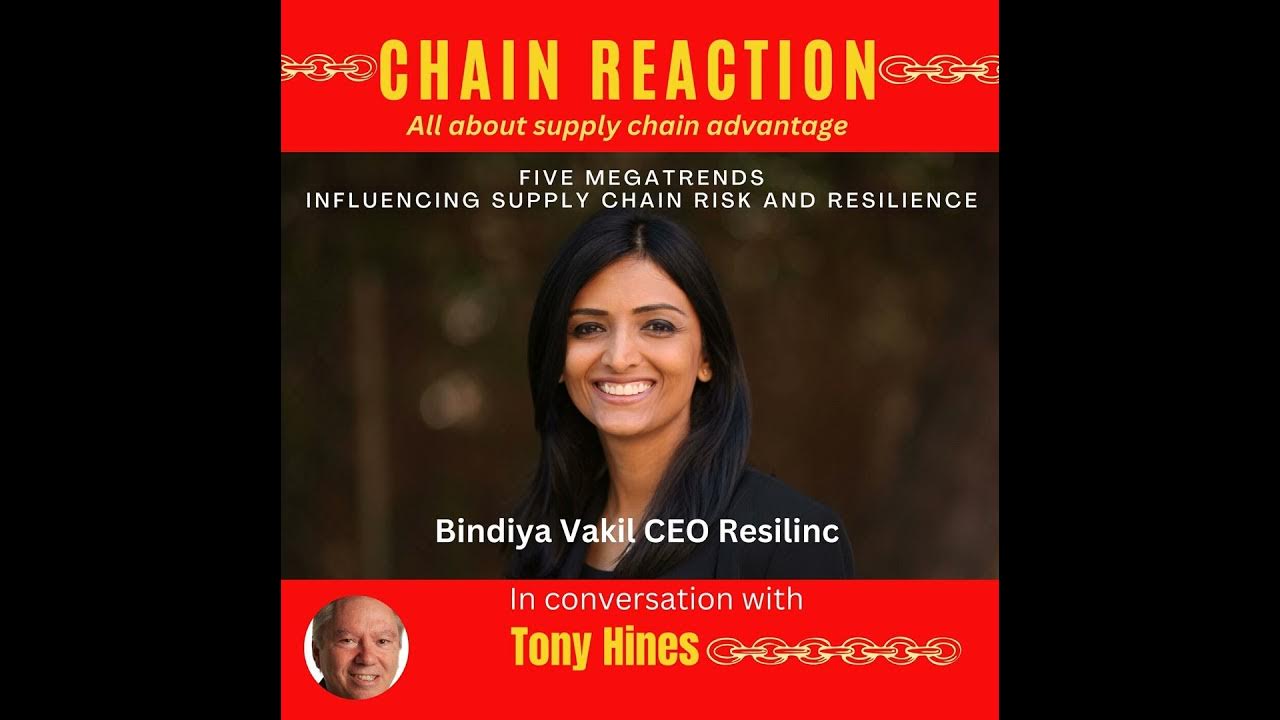
Five Megatrends Influencing Supply Chain Risk And Resilience with Bindiya Vakil

"Generación de Resiliencia en la Cadena de Suministro"
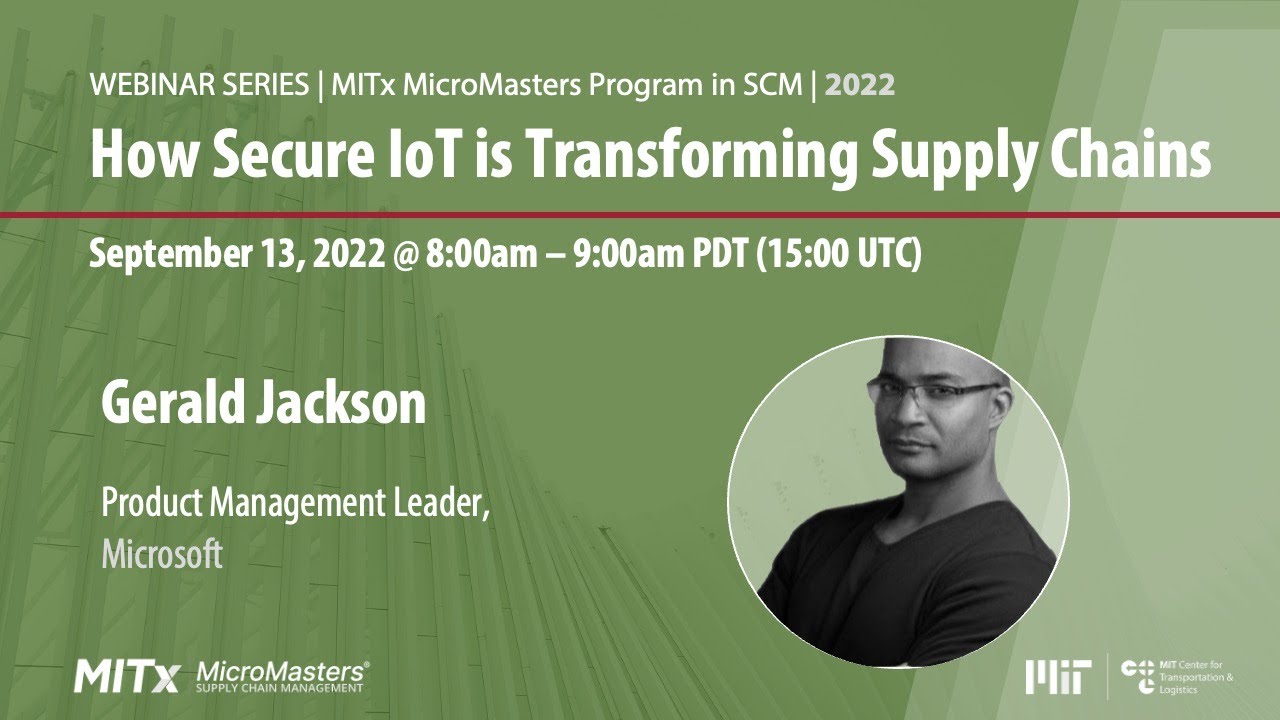
How Secure IoT is Transforming Supply Chains
5.0 / 5 (0 votes)