Biostatistics & Epidemiology - Use of Excel and Jamovi on Correlation Analysis
Summary
TLDRIn this tutorial, the speaker explains how to analyze correlations between variables using Pearsonโs R and Spearmanโs rho, depending on whether the data is normally distributed. The video demonstrates how to use Excel for correlation analysis and regression, while also introducing Jamovi as an alternative for non-normal data. The speaker walks through checking for normality using the Shapiro-Wilk test and provides clear steps for performing hypothesis testing and interpreting p-values. Ultimately, the conclusion is that there are no significant relationships between the analyzed variables, offering a practical guide to correlation analysis in both Excel and Jamovi.
Takeaways
- ๐ Pearson's R is used when both variables in the dataset are **normally distributed**, while Spearman's rho is used for **non-normal data**.
- ๐ The goal of the analysis is to check for **correlation** between two numerical variables, such as white blood cell count and red blood cell count.
- ๐ **Excel** is used to calculate Pearson's R for normally distributed data, but it has limitations when the data is non-normal. For non-normal data, **Jamovi** is recommended.
- ๐ Pearson's R values range between -1 and 1, where values close to -1 or 1 indicate a strong negative or positive relationship, respectively.
- ๐ **Significance testing** is essential: a p-value less than 0.05 indicates a significant relationship, while a p-value greater than 0.05 means no significant relationship.
- ๐ **Normality tests** like the Shapiro-Wilk test are used to check whether data follows a normal distribution. A p-value greater than 0.05 means the data is normally distributed.
- ๐ When using Excel, if the data is **not normally distributed**, Jamovi should be used to conduct Spearman's rho analysis for correlation.
- ๐ In **regression analysis**, the p-value is crucial for determining if the relationship observed between variables is statistically significant.
- ๐ The script demonstrates how to use both **Excel** and **Jamovi** for correlation analysis, with Excel being limited to normally distributed data and Jamovi handling non-parametric tests.
- ๐ **Lab experiment** involves testing three variable pairs for correlation, with the suggestion to choose **numerical variables** for meaningful analysis.
- ๐ If both variables in a pair are **not normally distributed**, Spearman's rho is automatically used to analyze their relationship instead of Pearson's R.
Q & A
What is the main focus of the discussion in the script?
-The main focus of the discussion is on the correlation between variables using Pearson's r and Spearman's rank correlation, specifically in the context of lab experiments where the goal is to determine if there is a significant relationship between numerical variables.
What types of variables are suitable for the correlation analysis discussed in the script?
-The variables must be numerical in nature. The script highlights that variables such as white blood cell count, red blood cell count, platelet count, and BMI are used, as they are numerical.
Why are gender and district not used in the correlation analysis?
-Gender and district are not used in the correlation analysis because they are categorical variables, whereas the analysis requires numerical data to determine the correlation.
When should Pearson's r be used instead of Spearman's rank correlation?
-Pearson's r should be used when the data is normally distributed. It is used to measure the linear relationship between two variables with a normal distribution.
What should be done if the data is not normally distributed?
-If the data is not normally distributed, Spearman's rank correlation should be used, as it is suitable for non-parametric data.
How can you check if the data is normally distributed using Excel?
-In Excel, you can assume normality for the data and use the built-in correlation tool. However, if the normality assumption is questionable, you should check the data using normality tests such as the Shapiro-Wilk test.
What is the significance of the P value in correlation analysis?
-The P value helps to determine if the relationship between variables is statistically significant. If the P value is less than the alpha value (0.05), the null hypothesis is rejected, indicating a significant relationship.
What does a negative Pearson's r value indicate in the context of correlation analysis?
-A negative Pearson's r value indicates a negative or inverse relationship between two variables. As one variable increases, the other decreases.
How do you determine if there is a significant relationship between variables using regression analysis in Excel?
-In regression analysis, you check the significance F value (P value). If the P value is less than the significance level (0.05), it suggests a significant relationship between the variables.
What is the difference between Pearson's r and Spearman's rank correlation?
-Pearson's r measures the strength of a linear relationship between two variables that are normally distributed, while Spearman's rank correlation is used for ordinal or non-normally distributed data to measure the strength of a monotonic relationship.
Outlines
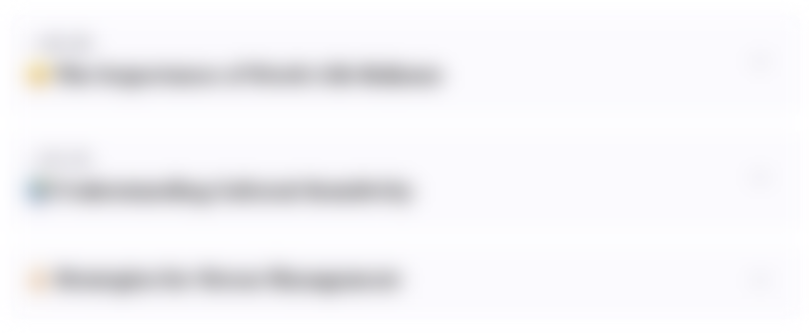
This section is available to paid users only. Please upgrade to access this part.
Upgrade NowMindmap
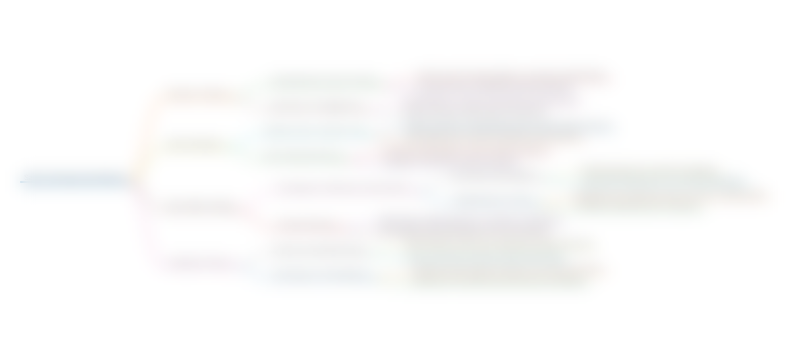
This section is available to paid users only. Please upgrade to access this part.
Upgrade NowKeywords
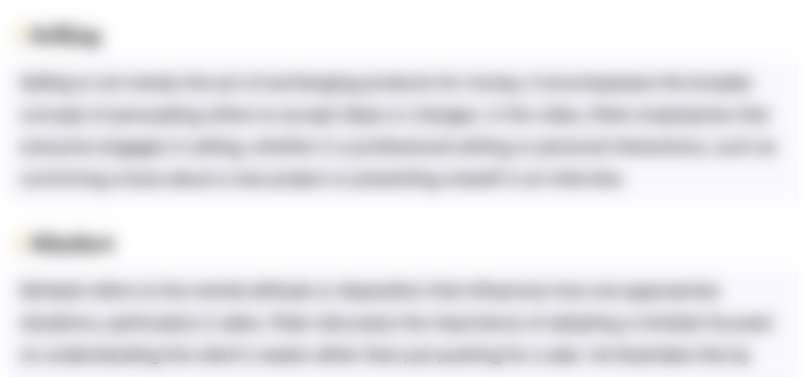
This section is available to paid users only. Please upgrade to access this part.
Upgrade NowHighlights
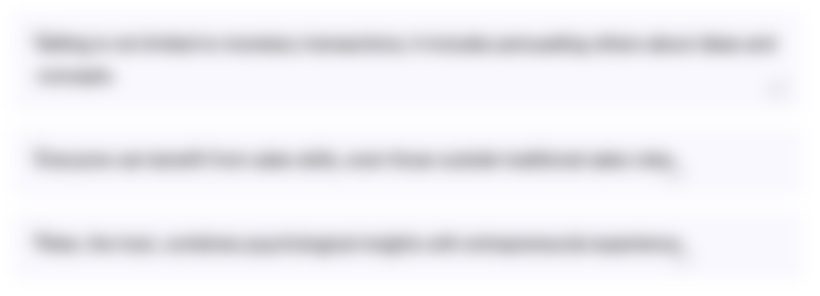
This section is available to paid users only. Please upgrade to access this part.
Upgrade NowTranscripts
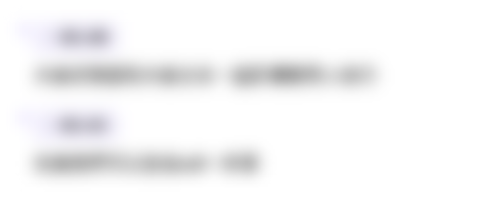
This section is available to paid users only. Please upgrade to access this part.
Upgrade NowBrowse More Related Video
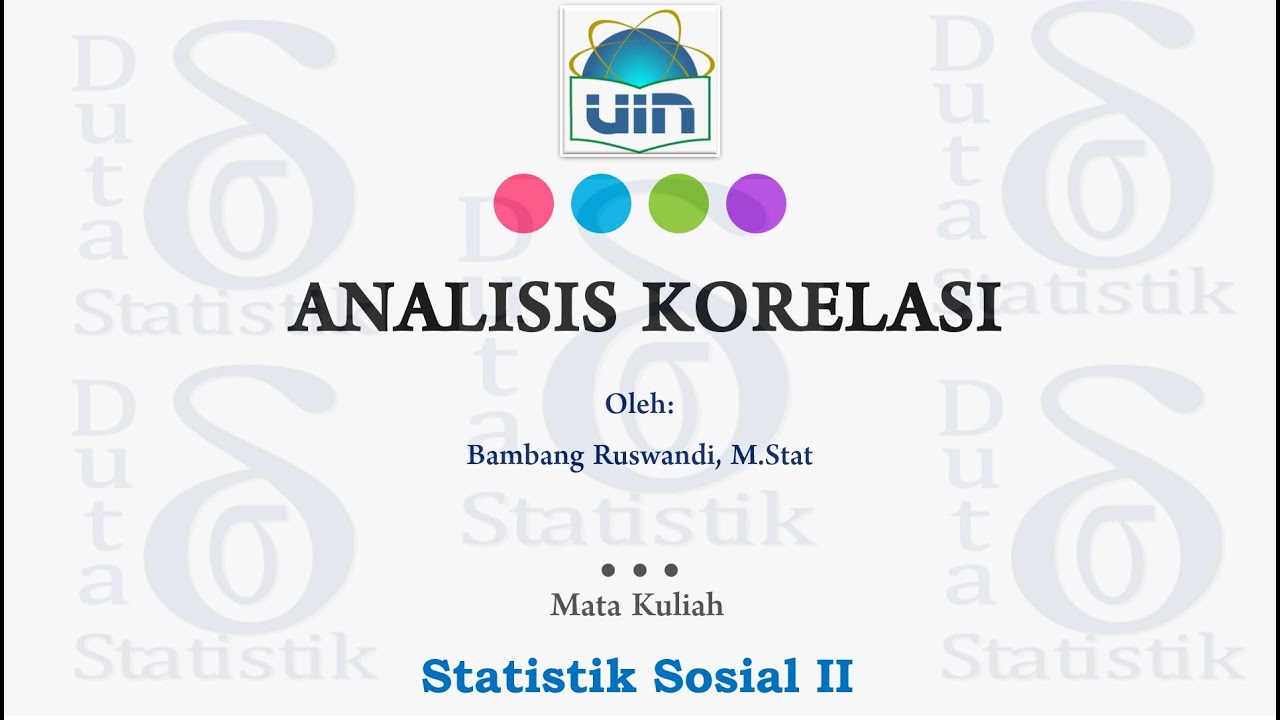
Pengantar Korelasi (Konsep Dasar Korelasi)
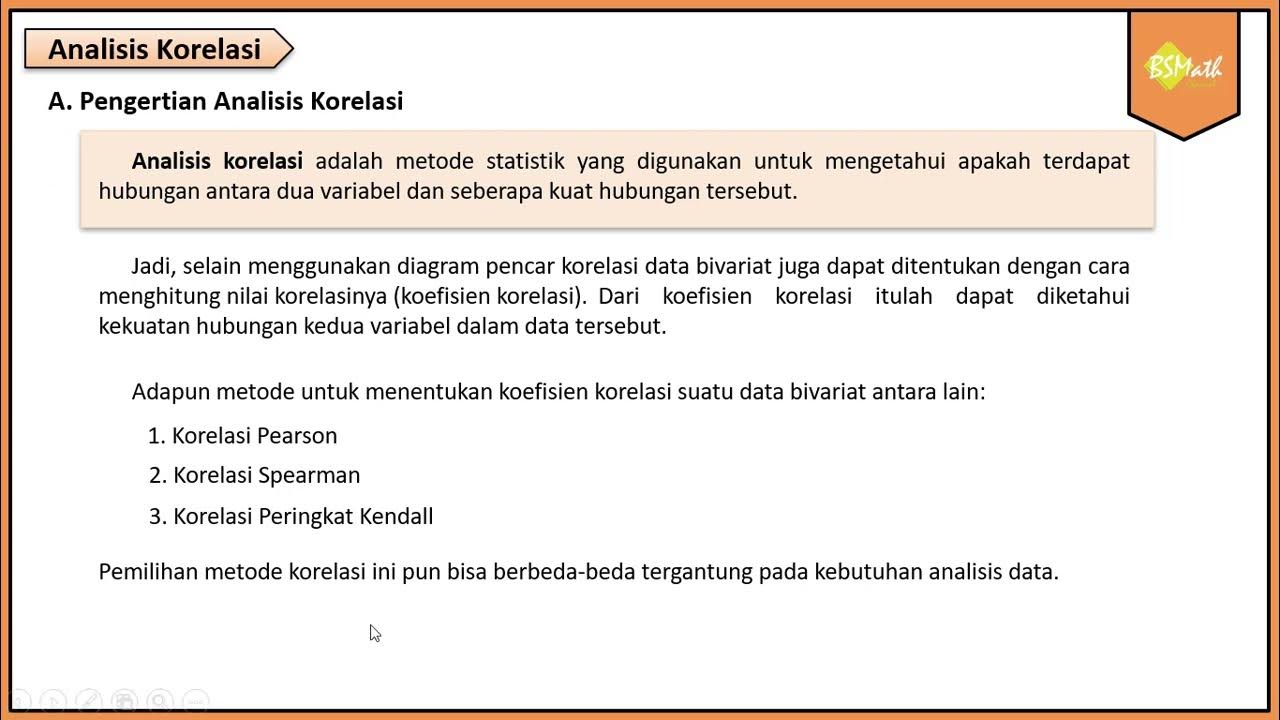
Analisis Korelasi - Matematika Wajib SMA Kelas XI Kurikulum Merdeka
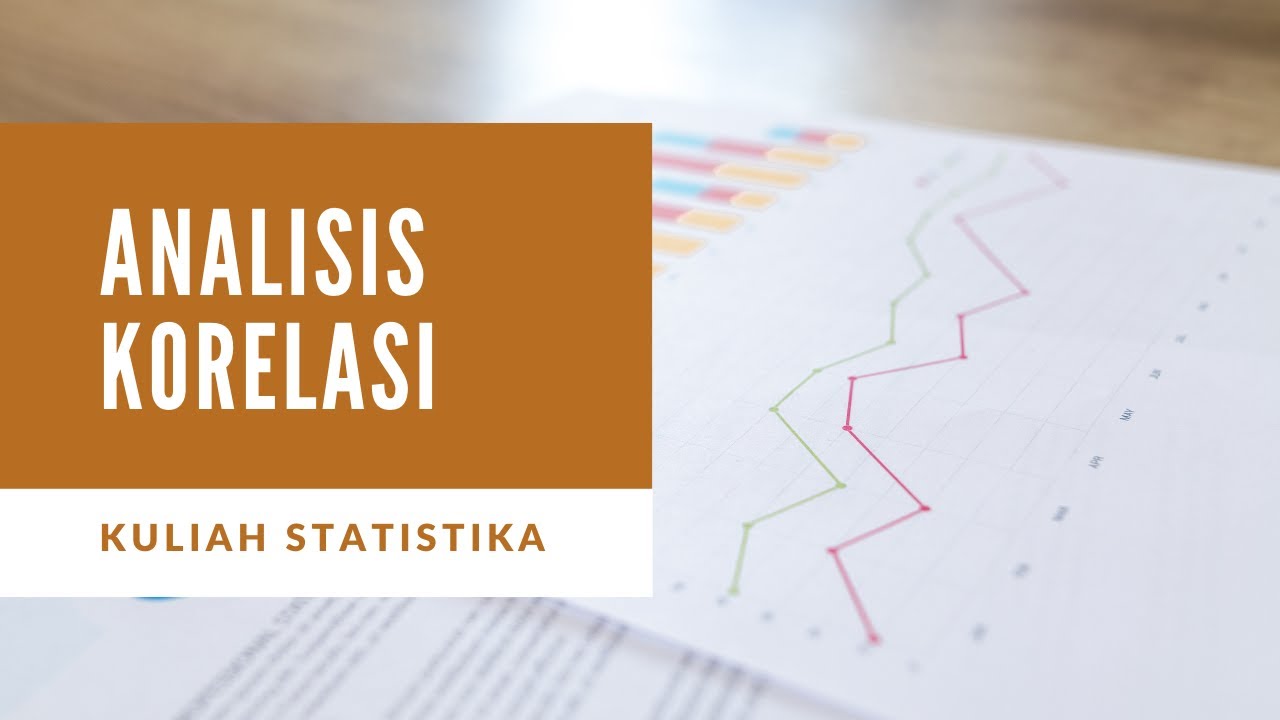
KULIAH STATISTIK - ANALISIS KORELASI
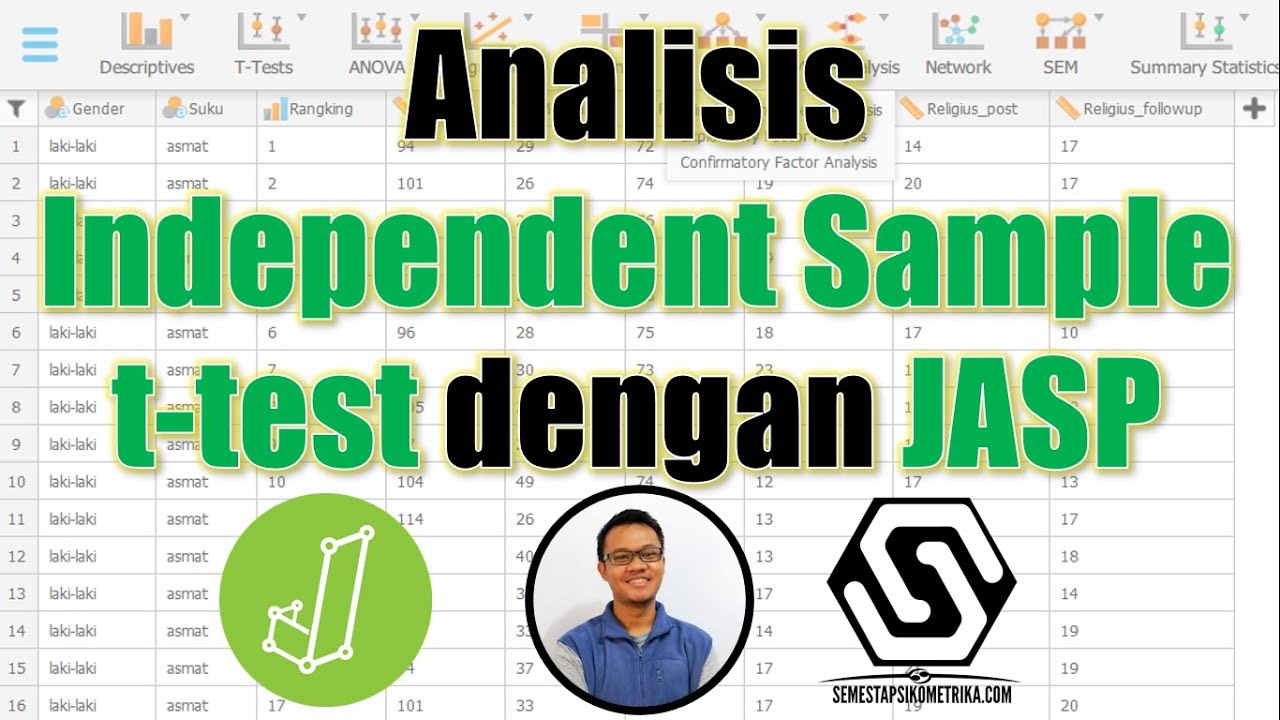
Tutorial Analisis Independent Sample t test dengan JASP

Uji Normalitas Lilifors Matkul STATISTIK 2
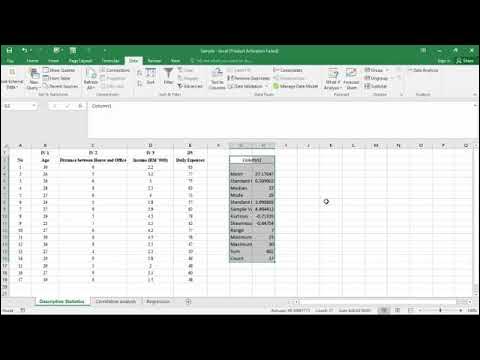
Descriptive Statistics, Correlation Analysis and Regression Analysis by Using Microsoft Excel
5.0 / 5 (0 votes)