DALL·E 2 Explained - model architecture, results and comparison
Summary
TLDRDALL·E 2, developed by OpenAI, represents a breakthrough in AI image generation using advanced diffusion models. By leveraging the CLIP model to generate shared text and image embeddings, DALL·E 2 employs a Prior and Decoder network to convert textual descriptions into realistic images. The Decoder uses a modified glide diffusion model, which progressively refines images through upsampling techniques. The model also allows for creative image manipulation in latent space, offering flexibility in generating variations. Compared to other methods, DALL·E 2 shows superior diversity and image quality, as demonstrated by human evaluations and impressive FID scores.
Takeaways
- 😀 DALL·E 2 combines contrastive language-image pre-training (CLIP) with a diffusion model to generate high-quality images.
- 😀 The UNCLIP architecture consists of a Prior Network and a Decoder Network, which work together to map text and image embeddings.
- 😀 CLIP learns the relationship between text and images by generating embeddings that allow the model to predict which text corresponds to a given image.
- 😀 The Prior Network generates image embeddings (zi) from text embeddings (zt) and learns the mapping between them during training.
- 😀 The Decoder Network uses a modified version of the GLIDE diffusion model, which refines noisy images to produce realistic outputs.
- 😀 Diffusion models, like GLIDE, are preferred over GANs and VAEs for their superior image quality in DALL·E 2.
- 😀 The model utilizes upsampling techniques to progressively increase image resolution, from 64x64 to 1024x1024.
- 😀 By manipulating latent vectors, the model can generate variations of images or blend features from two different images.
- 😀 The ability to control image generation using text inputs allows for fine-grained modifications of visual content, such as changing seasons in landscape images.
- 😀 Evaluation results show that using the Prior Network with both text and image embeddings produces better diversity and image quality than using text alone.
- 😀 DALL·E 2 achieves impressive FID scores, outperforming other state-of-the-art image generation methods in terms of image fidelity and diversity.
Q & A
What is DALL·E 2 and how does it differ from previous image generation models?
-DALL·E 2 is a model from OpenAI that uses diffusion models to generate highly realistic images from textual descriptions. It differs from previous models by leveraging a combination of a prior network and a decoder network, along with trained clip embeddings to generate both images and text embeddings. This allows it to produce better image quality compared to older models like GANs or autoencoders.
What is the role of the 'clip' model in DALL·E 2?
-The 'clip' model in DALL·E 2 is used to learn the relationship between text and images. It produces embeddings for both the input image and the corresponding text. These embeddings, representing features of the image and text, are then fed into the prior network to generate new images based on text inputs.
What are the two main components of DALL·E 2's architecture?
-DALL·E 2 consists of two main components: the prior network and the decoder network. The prior network processes text and image embeddings and learns the mapping between them, while the decoder network generates images based on those embeddings, with the potential to include text captions as input.
How does the prior network work in DALL·E 2?
-The prior network in DALL·E 2 takes in text embeddings (from the clip model) and image embeddings, learning to map them to each other during training. This enables the system to generate images that are consistent with the provided textual description.
What is the difference between the auto-regressive prior and the diffusion prior in DALL·E 2?
-The auto-regressive prior in DALL·E 2 uses PCA (Principal Component Analysis) to reduce the dimensionality of clip embeddings, while the diffusion prior uses a diffusion model to generate images. The diffusion prior has shown better image quality compared to the auto-regressive approach, making it the preferred choice for image generation.
What role do upsampler models play in DALL·E 2's image generation process?
-Upsampler models in DALL·E 2 are used to gradually increase the resolution of generated images. They begin with a low resolution (64x64), progressively enhancing the image quality until reaching a higher resolution (1024x1024), which is where the final results are presented.
What is the purpose of the 'latent space' and how is it used in DALL·E 2?
-The 'latent space' in DALL·E 2 refers to a space where the noise vectors (latent vectors) are used to encode information about an image. The model manipulates these vectors to generate variations of an image or to transition from one image to another. This allows the model to create consistent and semantically meaningful variations of the input images.
How does DALL·E 2 manipulate images using the latent vectors?
-DALL·E 2 uses latent vectors to manipulate images by sweeping along the surface of a sphere in the latent space. This allows it to generate intermediate images that are a blend of two original images, showing gradual transitions between them.
Why is the use of text input significant in DALL·E 2's image generation process?
-The use of text input in DALL·E 2 is significant because the clip model ensures that the text and image embeddings share the same latent space. This allows the model to generate images that closely align with specific textual descriptions, enabling precise control over the generated images based on slight changes in the text.
What advantages does DALL·E 2's prior network offer over simpler methods like using only the text caption?
-The prior network in DALL·E 2 enhances image diversity and correlation with the text input compared to methods that only use the text caption. Using both text and image embeddings together results in better semantic alignment and image diversity, leading to more realistic and diverse image generations.
Outlines
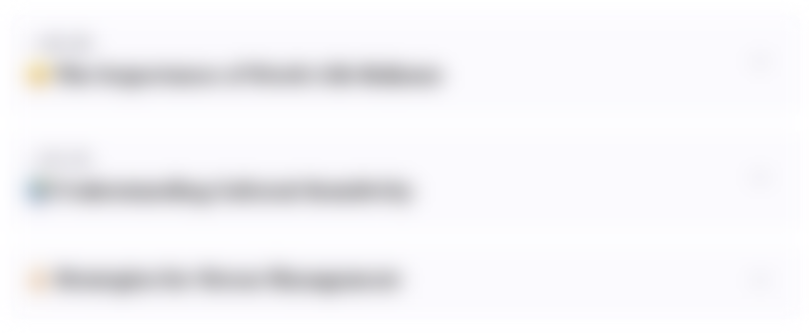
This section is available to paid users only. Please upgrade to access this part.
Upgrade NowMindmap
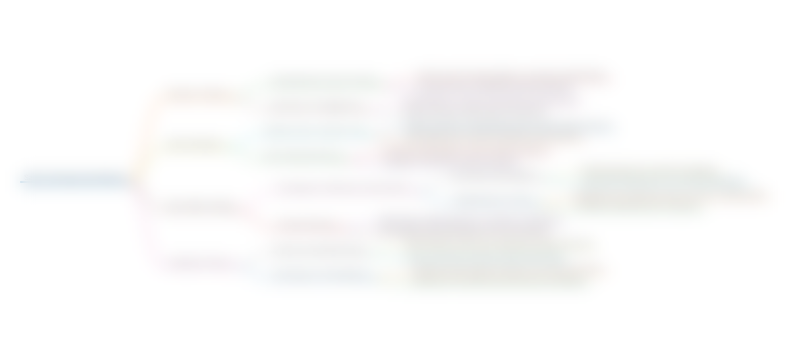
This section is available to paid users only. Please upgrade to access this part.
Upgrade NowKeywords
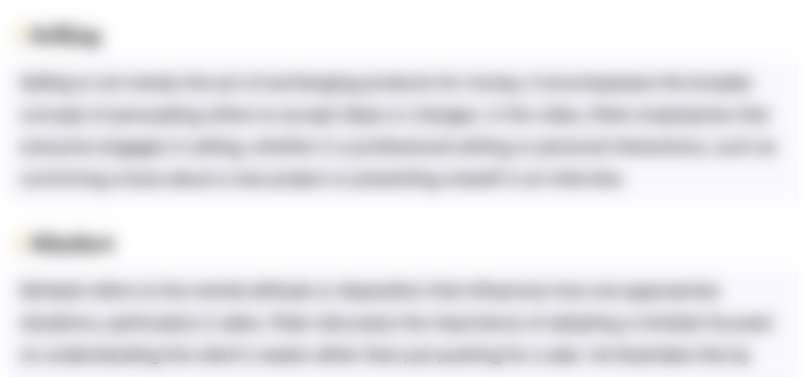
This section is available to paid users only. Please upgrade to access this part.
Upgrade NowHighlights
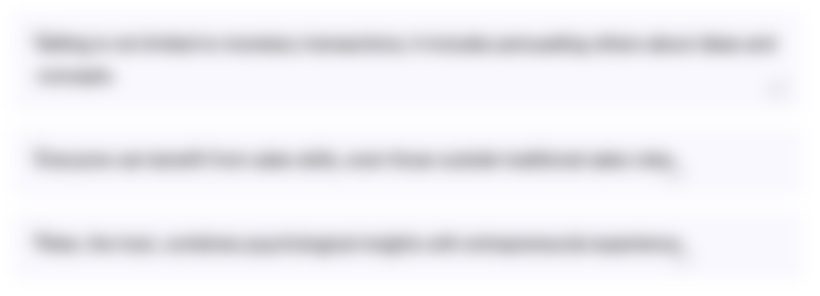
This section is available to paid users only. Please upgrade to access this part.
Upgrade NowTranscripts
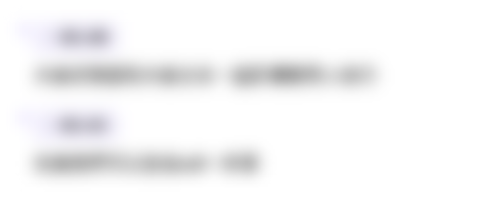
This section is available to paid users only. Please upgrade to access this part.
Upgrade Now5.0 / 5 (0 votes)