AI Career Opportunities for Data Professionals - Time to Pivot?
Summary
TLDRIn this insightful video, Dave Abelar, founder of Data Lumina, explores the booming AI industry's impact on data professionals. He discusses opportunities across various roles, including data scientists, engineers, and AI specialists, highlighting the need to upskill in software engineering and adapt to new technologies like large language models. Abelar also addresses the current hype cycle of generative AI, suggesting ways to pivot or upskill within the field, and offers guidance for leveraging AI in business strategies and considering freelance opportunities to future-proof careers.
Takeaways
- π The AI hype is still booming, offering numerous opportunities for data professionals to pivot or upskill their careers.
- π‘ Data professionals are considering whether to pivot or upskill in response to the current market trends and opportunities in AI.
- π Dave Abelar, founder of Data Lumina, discusses various data roles and their opportunities in the current market, emphasizing the potential for growth and change.
- π The hype cycle of generative AI is currently at the peak of inflated expectations, suggesting a potential upcoming disillusionment phase where the technology's challenges become more apparent.
- π Despite the public hype dying down, business owners and managers still show strong interest in large language models and their transformative potential.
- π» Data scientists can leverage their skills in understanding business cases and data to work with pre-trained models, but may need to upskill in software engineering to implement these models in applications.
- π Data analysts can explore opportunities in monitoring and evaluating large language models, using tools like LangSmith or LangFuse to ensure model performance over time.
- π§ Data engineers have a crucial role in providing the foundational data and infrastructure needed for AI models, including building data platforms and optimizing data feeds for AI applications.
- π€ AI Engineers focus on prompt engineering, handling chaotic mediums like large language models, and building event-driven architectures, often without needing deep machine learning expertise.
- πΌ Consulting in AI strategy can be a valuable role for data professionals, helping businesses navigate the implementation of AI effectively and safely.
Q & A
What is the current state of the AI hype cycle according to the video?
-The video mentions that generative AI was at the peak of inflated expectations last year, and it is likely to be followed by a trough of disillusionment where people realize the technology is not as easily transformative as initially thought.
What is the role of Dave Abelar in the data community?
-Dave Abelar is the founder of Data Lumina, where he has been building custom data solutions for clients for the past 5 years. He also runs a community called Data Freelancer, teaching data professionals how to get started with freelancing and scale it to six figures and beyond.
Why might a data professional consider upskilling in software engineering when looking into generative AI?
-Upskilling in software engineering is important because when working with pre-trained large language models, the focus shifts from model training to application development. This requires understanding web applications, event-driven architectures, and deployment strategies like Docker containers and cloud services.
What opportunities are available for data scientists in the context of AI and large language models?
-Data scientists can explore opportunities in prompt engineering, optimizing AI model outputs, and building applications around pre-trained models. They can also leverage their skills in data analysis and model evaluation to work on generative AI projects.
How can data analysts contribute to the monitoring and evaluation of AI applications?
-Data analysts can use tools like LangSmith or LangFuse to create dashboards for monitoring AI applications, ensuring the performance of these applications does not decrease over time. They can also create evaluation datasets to assess model performance with different prompts.
What is the significance of data infrastructure for data engineers in leveraging AI opportunities?
-Data engineers play a crucial role in establishing a solid data platform that feeds the AI models with the right data. They can focus on building robust data pipelines, working with vector databases, and ensuring data is well-prepared for AI models to use effectively.
What are the key skills an AI engineer should focus on according to the video?
-AI engineers should focus on prompt engineering, tolerance for working with chaotic mediums like large language models, chaining agents, reactive UIs, and event-driven architectures. They should also understand software engineering fundamentals more than deep learning expertise.
What opportunities are there for machine learning engineers in the field of generative AI?
-Machine learning engineers can explore optimization techniques for large language models using libraries like DSPI and TextGret. They can also focus on monitoring and operational aspects of AI models, leveraging their existing knowledge in machine learning to work with generative AI.
What consulting opportunities are available for data professionals in the realm of AI?
-Data professionals can offer AI strategy consulting, helping businesses understand how to effectively, safely, and reliably use AI. This includes creating AI strategy roadmaps, addressing data management, technology infrastructure, and capability development within a company.
How can data professionals capitalize on the current AI hype to pivot their careers?
-Data professionals can explore internal opportunities within their current roles, seek new job opportunities focusing on AI, or start freelancing to gain experience with generative AI. They can also consider consulting to help businesses navigate AI implementation.
Outlines
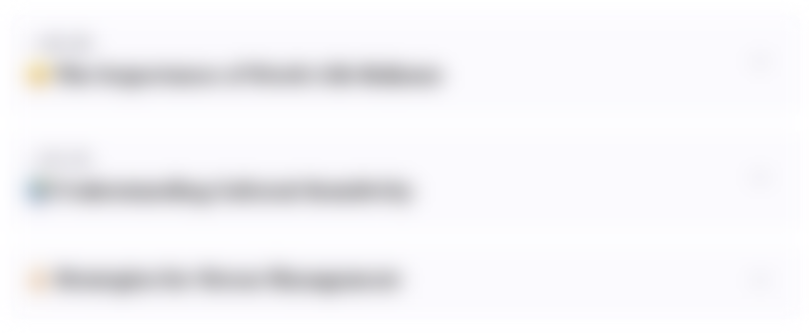
This section is available to paid users only. Please upgrade to access this part.
Upgrade NowMindmap
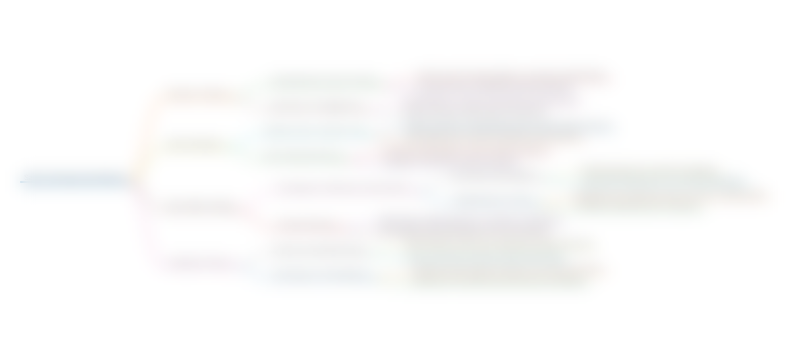
This section is available to paid users only. Please upgrade to access this part.
Upgrade NowKeywords
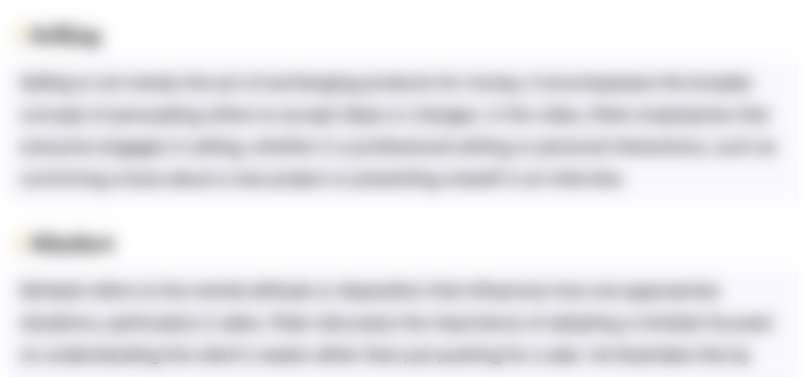
This section is available to paid users only. Please upgrade to access this part.
Upgrade NowHighlights
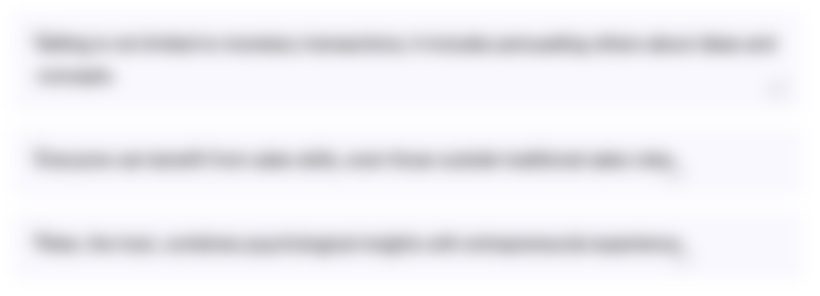
This section is available to paid users only. Please upgrade to access this part.
Upgrade NowTranscripts
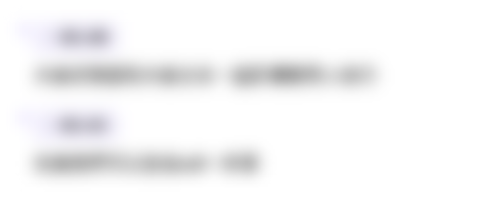
This section is available to paid users only. Please upgrade to access this part.
Upgrade NowBrowse More Related Video

From Pre-Med to $135K Data Analyst: Was it Worth it?
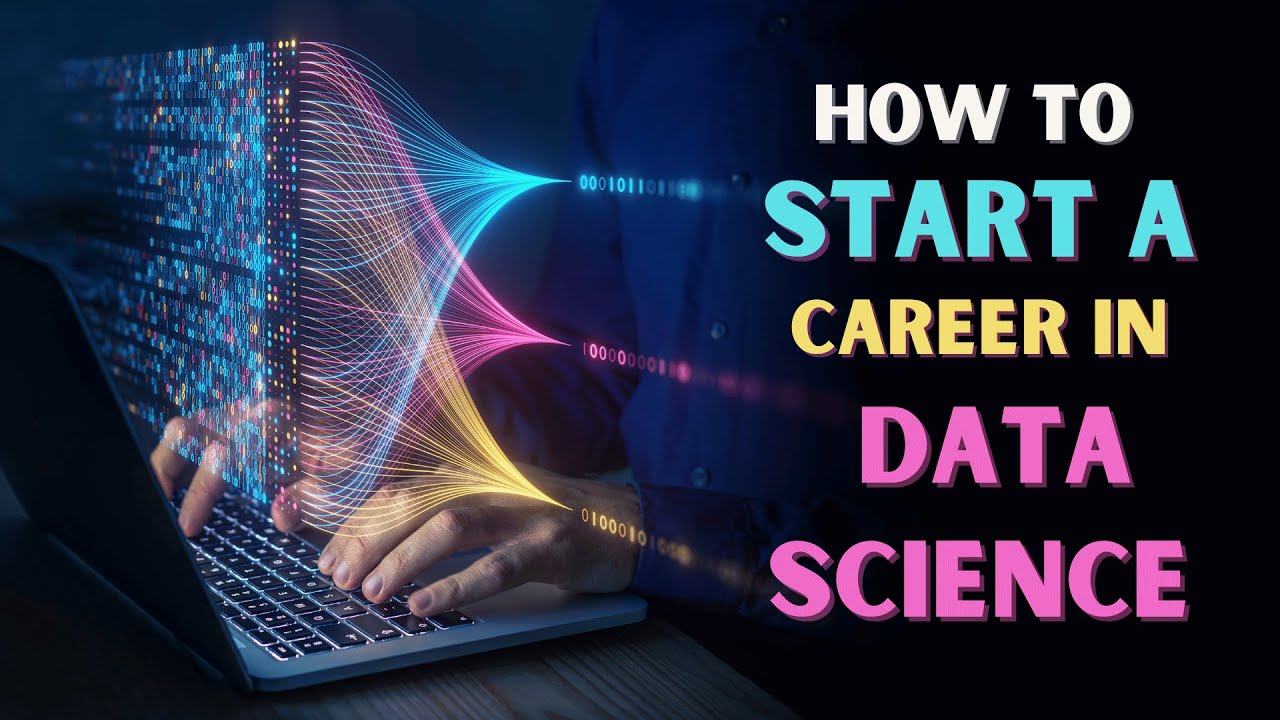
How to start a Career in Data Science - [Hindi] - Quick Support
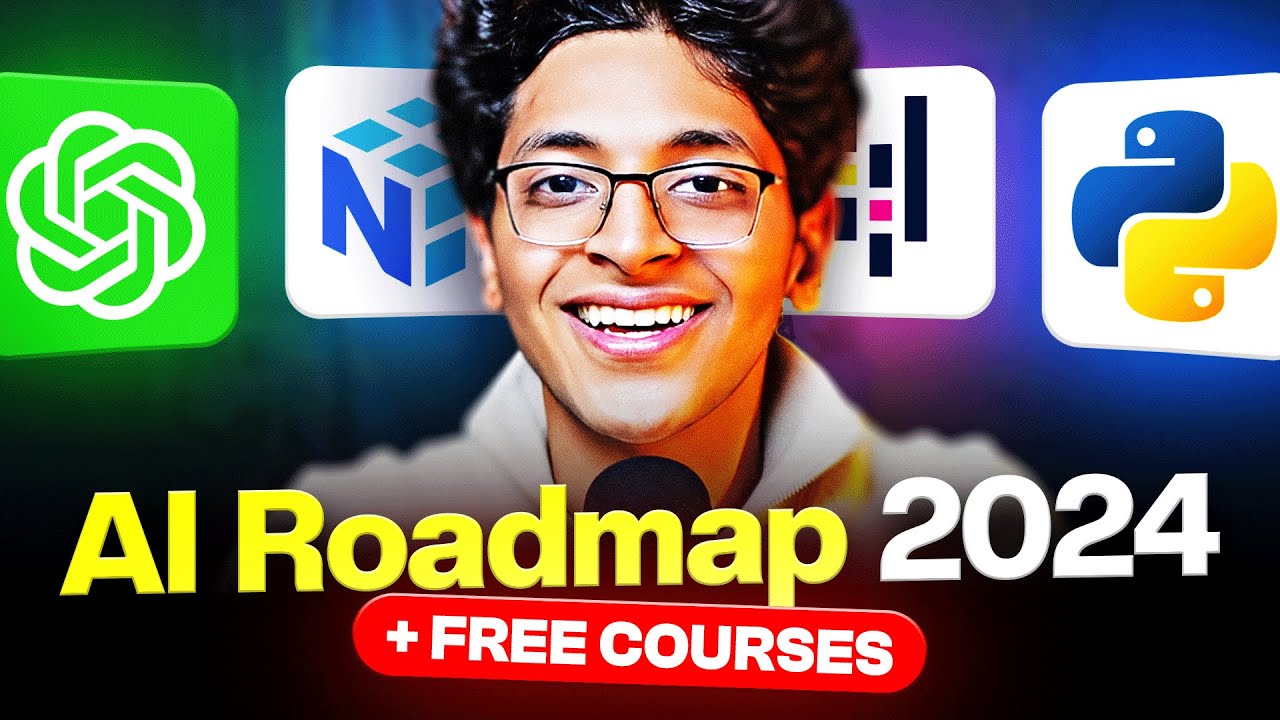
How I'd Learn AI in 2024 (If I Could Start Over) | Machine Learning Roadmap
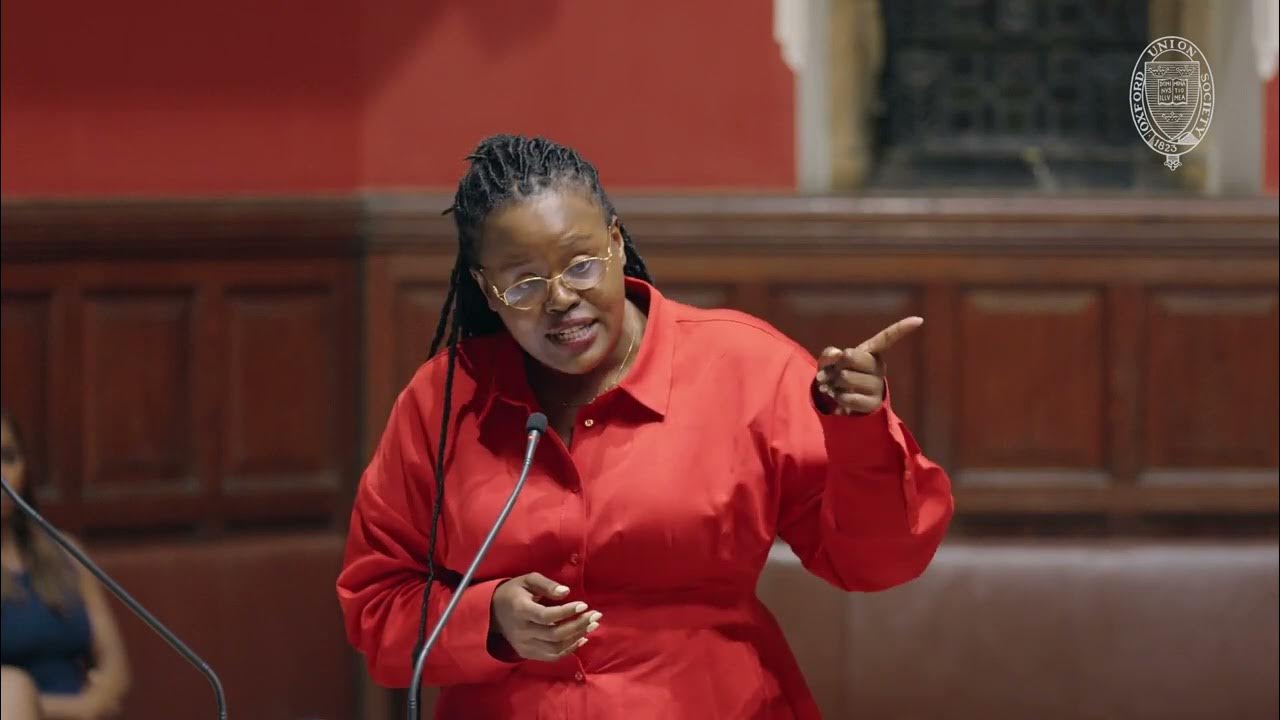
Yeshimabeit Milner warns that the true existential threat is big data in the hands of a few (4/8)
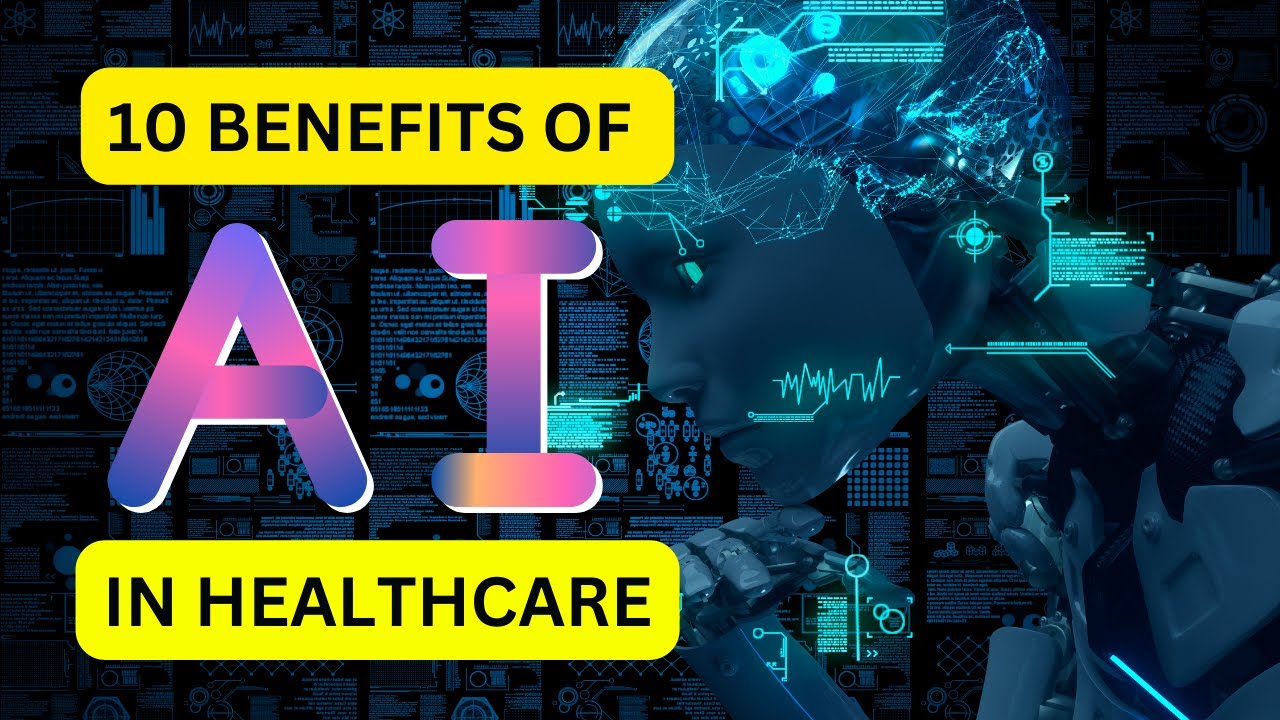
10 Benefits of Artificial intelligence in Healthcare
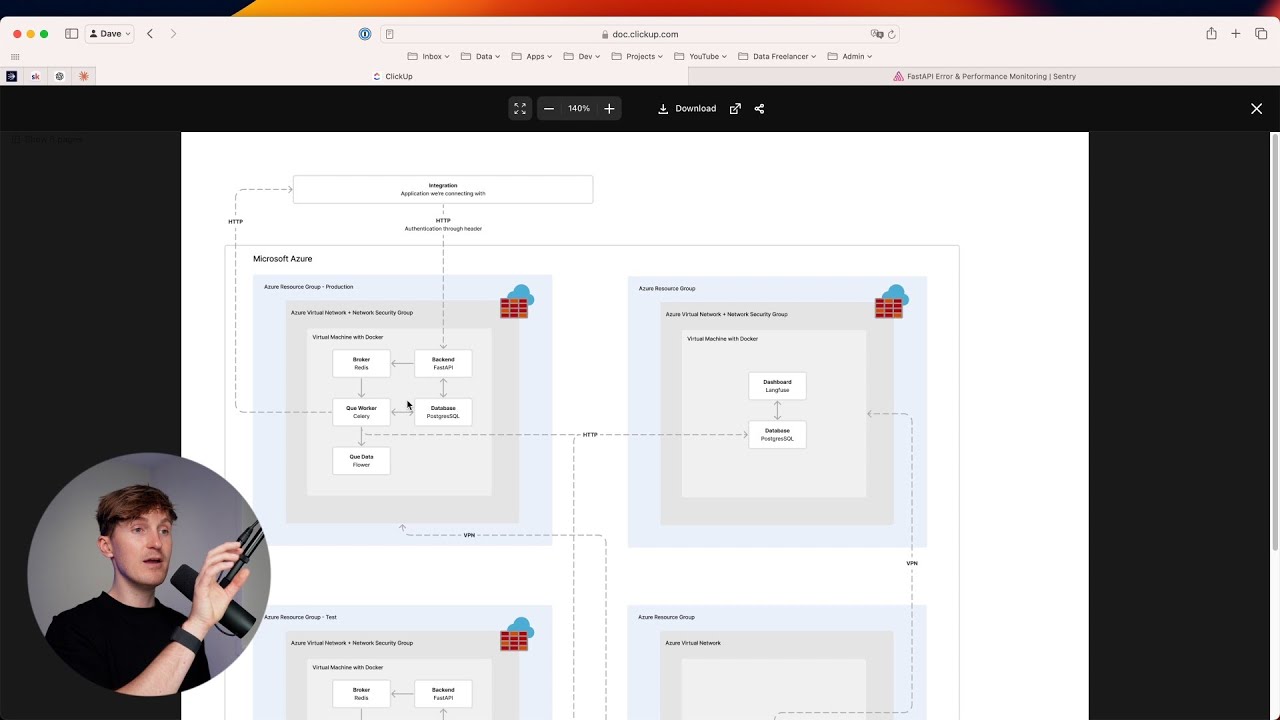
How to Find, Build, and Deliver GenAI Projects
5.0 / 5 (0 votes)