How to Select the Correct Predictive Modeling Technique | Machine Learning Training | Edureka
Summary
TLDRIn this tutorial, the speaker introduces predictive analysis, a key technique in data science used to forecast future outcomes based on historical data. The video covers various predictive modeling techniques including regression, classification, clustering, time series, and forecasting. It explains how to choose the right technique based on factors like target variables, data linearity, and dataset size. The speaker also highlights real-world applications of predictive analysis in fields such as finance, healthcare, government, and sports. The tutorial is designed to help viewers understand when and how to use these techniques for effective decision-making.
Takeaways
- ๐ Predictive analysis is a data-driven approach used to predict future events or outcomes based on past data and patterns.
- ๐ Regression is used to predict continuous variables (e.g., salary, area), with techniques like simple linear regression and logistic regression.
- ๐ Logistic regression is used for binary classification problems, where the target variable is categorical (e.g., yes/no, true/false).
- ๐ Classification is the process of categorizing data into classes or categories, such as heart disease detection which involves binary classification.
- ๐ Clustering involves grouping data points based on similarity, where points in the same group are as similar as possible and points in different groups are as different as possible.
- ๐ Time series models use past data to predict future data points, accounting for trends and seasonal factors.
- ๐ Forecasting uses historical data to make predictions about future outcomes or metrics, such as stock price prediction.
- ๐ Choosing the right predictive technique depends on the target variable (continuous or categorical) and the nature of the data (linear or non-linear).
- ๐ Factors to consider when selecting a model include the size of the data, the risk of overfitting, and whether the data is linearly separable.
- ๐ Machine learning models for predictive analysis include linear regression, logistic regression, k-means clustering, neural networks, decision trees, and time series models.
- ๐ Predictive analysis has various applications in finance (e.g., fraud detection, stock prediction), healthcare (e.g., disease detection), government (e.g., policy impact prediction), and other sectors.
Q & A
What is predictive analysis?
-Predictive analysis is a branch of data analysis that uses data-driven techniques to predict future events or outcomes by finding relevant patterns in large datasets. It is used to make informed decisions in various business areas such as risk detection, market analysis, and forecasting.
What are the primary techniques used in predictive analysis?
-The main techniques used in predictive analysis include regression, classification, clustering, time series analysis, and forecasting. These techniques help in building models to predict continuous or categorical outcomes, group similar data points, and forecast future trends based on historical data.
How does regression analysis work in predictive modeling?
-Regression analysis is used to predict a dependent variable based on independent variables. In the case of simple linear regression, it predicts continuous outcomes by modeling a linear relationship between variables. Logistic regression, a type of regression, is used for predicting binary outcomes (e.g., yes/no, true/false).
What is the difference between classification and clustering in predictive modeling?
-Classification is used to predict which category a new data point belongs to, typically with predefined classes. It is used for problems where the target variable is categorical (e.g., heart disease detection). Clustering, on the other hand, is used to group similar data points together based on their features, without predefined categories, often used for unsupervised learning.
What is time series analysis and when is it used?
-Time series analysis involves analyzing data points ordered by time to predict future values based on past trends. It is used for forecasting purposes, such as predicting stock prices or weather patterns. Time series models take into account seasonality and events that could impact the predictions.
How does forecasting differ from other predictive analysis techniques?
-Forecasting is specifically used to predict future outcomes based on historical data, typically for time-dependent variables. While time series analysis focuses on analyzing data trends over time, forecasting models are directly used to predict future values based on patterns from the past.
What factors should be considered when choosing a predictive model?
-Key factors include the type of target variable (continuous or categorical), the size and quality of the data, whether the data is linearly separable, and whether the model may suffer from overfitting or underfitting. Additionally, understanding the relationship between input and output variables helps in choosing the most appropriate technique.
What are some machine learning models used in predictive analysis?
-Common machine learning models include linear regression (for continuous target variables), logistic regression (for binary classification), k-means clustering (for grouping data), neural networks (for pattern recognition), decision trees (for mapping out outcomes), and time series models (for forecasting future data).
Can you give examples of applications of predictive analysis in real-world scenarios?
-Predictive analysis is widely used in fields such as finance (for fraud detection and stock market prediction), government (for policy impact prediction and disaster management), healthcare (for early disease detection), manufacturing (for predicting production failures), and telecom (for customer segmentation and support).
How do decision trees contribute to predictive analysis?
-Decision trees are used to model decisions and their possible consequences. They map out possible outcomes based on different choices, weighing the costs and benefits. This makes them useful for both classification and regression tasks, where the model needs to predict the best possible outcome from a series of options.
Outlines
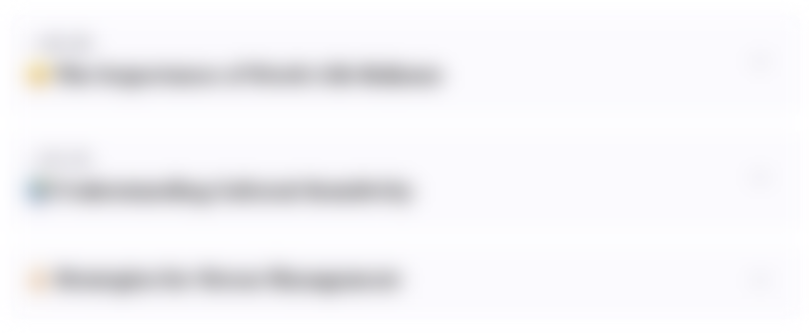
This section is available to paid users only. Please upgrade to access this part.
Upgrade NowMindmap
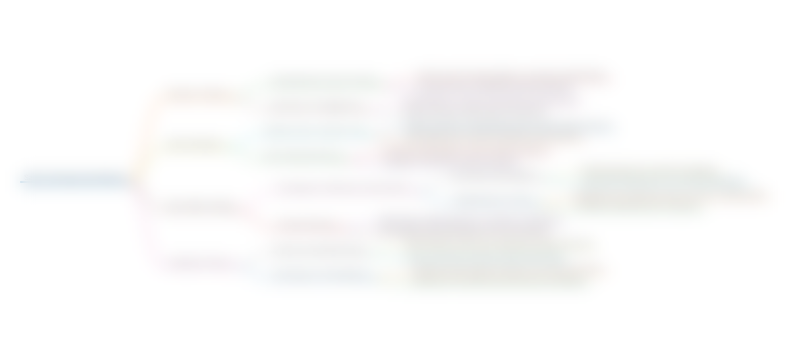
This section is available to paid users only. Please upgrade to access this part.
Upgrade NowKeywords
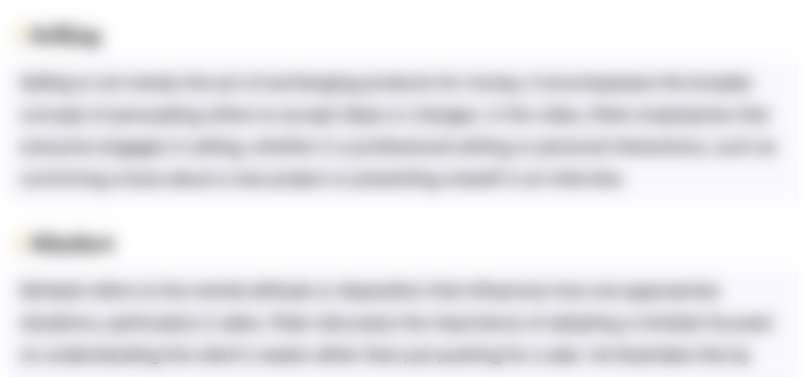
This section is available to paid users only. Please upgrade to access this part.
Upgrade NowHighlights
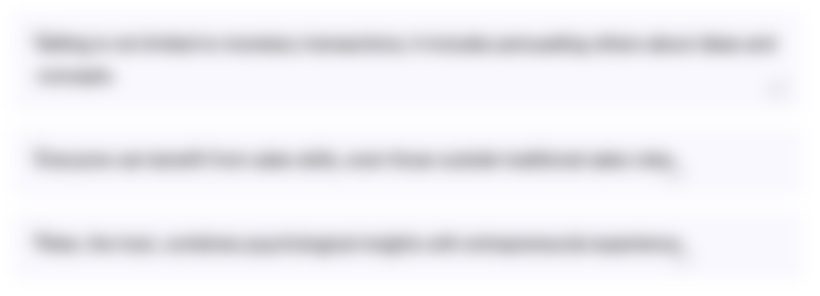
This section is available to paid users only. Please upgrade to access this part.
Upgrade NowTranscripts
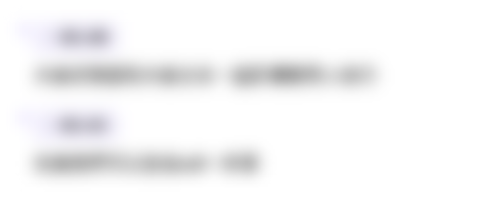
This section is available to paid users only. Please upgrade to access this part.
Upgrade NowBrowse More Related Video
5.0 / 5 (0 votes)