Computers Can Predict When You're Going to Die… Here's How
Summary
TLDRThe video script explores the concept of predictive analytics, a branch of mathematics that uses historical data to forecast future outcomes. It delves into the history of predictive analytics, starting with the 1600s when Lloyd's of London used data to predict the risk of sea voyages, leading to the birth of the insurance industry. The script then transitions to the present, highlighting how predictive analytics is pervasive in various sectors, from shopping to politics. The narrative takes a personal turn as the host, Joe, discusses death anxiety and the possibility of predicting life expectancy using algorithms. He introduces an AI model that predicts mortality with high accuracy by analyzing multi-factor datasets. The script concludes with a reflection on the power of human choice and the potential of predictive analytics to enhance life, encouraging viewers to stay curious and make the most of their time.
Takeaways
- 📉 Joe's initial reaction to comments on his age leads to a humorous reflection on death and an introduction to life-extending efforts.
- 📚 A 2022 survey reveals that a significant portion of Americans and Gen Z individuals think about death frequently, highlighting the prevalence of death anxiety.
- 💰 The global health and wellness market is worth $1.8 trillion, indicating the extent to which society is invested in extending life.
- 📈 Predictive analytics, a branch of mathematics, uses historical data to predict future outcomes and is widely used across various sectors.
- ⛵ The origins of predictive analytics can be traced back to the 1600s with the advent of maritime insurance by the Lloyd's Company of London.
- 🧮 Predictive analytics involves creating a 'statistical Franken-human' by aggregating data from numerous individuals to predict outcomes for a collective rather than an individual.
- 📱 Our daily activities generate a wealth of data that can be used to predict our behaviors and outcomes, such as where we are likely to be at any given time.
- 🔢 The law of large numbers is a mathematical theory that underpins predictive analytics, stating that larger data samples are more likely to reflect actual averages.
- 🎰 The complexity of predicting future events is simplified by considering multiple factors and their influence on outcomes, much like analyzing the contents of a bag of marbles.
- 🤖 Machine learning is employed to analyze increasingly complex factors and determine which ones are significant in predicting future events.
- 🧳 An AI mortality model trained on Danish data was able to predict survival with a high degree of accuracy, underscoring the potential of predictive analytics in life expectancy.
- 🚀 Despite the accuracy of predictive models, unpredictable 'black swan' events can still occur, emphasizing the limits of these models and the importance of human agency in shaping our futures.
Q & A
What is the main theme of the video?
-The main theme of the video is the concept of death anxiety and the use of predictive analytics to estimate life expectancy and risks associated with various factors.
What does the term 'thanatophobia' refer to?
-Thanatophobia is the fear of death, a perfectly natural human feeling that many people experience.
How does the global health and wellness market size relate to the video's content?
-The size of the global health and wellness market, estimated at $1.8 trillion, illustrates the extent to which people are willing to invest in products and services to extend life and improve health, reflecting the human desire to 'cheat death.'
What historical example is given to show the use of predictive analytics?
-The historical example given is the Lloyd's Company of London, which used past data to predict the risk of sea voyages and offered insurance based on those predictions, effectively birthing the insurance industry.
How does the law of large numbers play a role in predictive analytics?
-The law of large numbers is a mathematical theory stating that the larger the data sample, the more likely it is that the average of that sample will reflect what actually happens. This principle is used in predictive analytics to create more accurate predictions by analyzing larger groups of data points.
What is the significance of machine learning in improving predictive analytics?
-Machine learning allows for the analysis of more complex factors and can determine which factors are actually important in predicting outcomes. It can process all possible factors, free from human bias, and select and weight factors more objectively.
What is the accuracy of the AI predictive mortality model mentioned in the video?
-The AI predictive mortality model mentioned in the video was able to guess correctly 8 out of 10 times when tested with a set of people where half survived and half died.
How does the actuary in the video estimate Joe's life expectancy?
-The actuary uses a longevity illustrator and factors such as Joe's age, gender, and habits (like not smoking) to estimate his life expectancy to be around 86 years, with a 37% probability of living to age 90 and an 8% chance of living to age 100.
What is the role of black swan events in the context of predictive analytics?
-Black swan events are outlier events that are moments of totally unpredictable chaos. They represent the unpredictable elements that can occur despite the most accurate predictive analytics, emphasizing the inherent uncertainty in forecasting the future.
What is the conclusion the presenter draws about the power of individual choices?
-The presenter concludes that despite the accuracy of mathematical tools and predictive analytics in forecasting our lives and actions, individuals still have the power to make choices that can change those predictions and lead to different outcomes.
What is the purpose of the Patreon mention at the end of the video?
-The Patreon mention is a call to action for viewers to support the show financially. By signing up for Patreon, viewers can gain early access to videos and support the content creation process, effectively taking some control back from predictive analytics algorithms.
Outlines
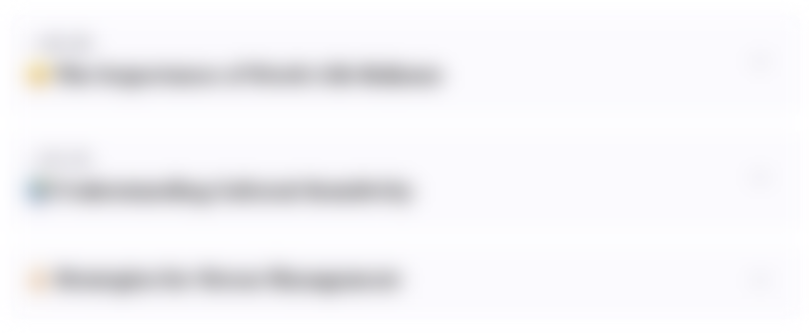
This section is available to paid users only. Please upgrade to access this part.
Upgrade NowMindmap
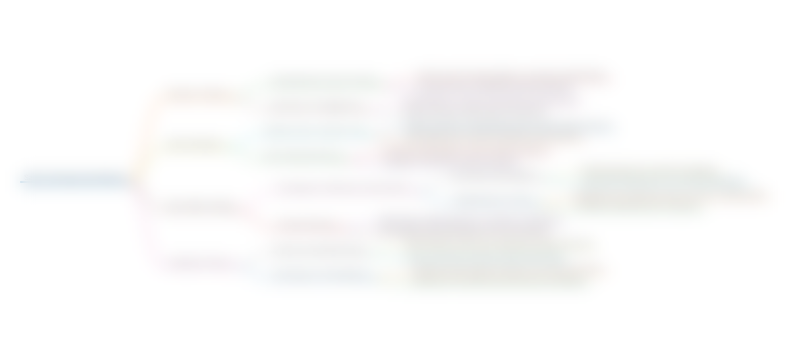
This section is available to paid users only. Please upgrade to access this part.
Upgrade NowKeywords
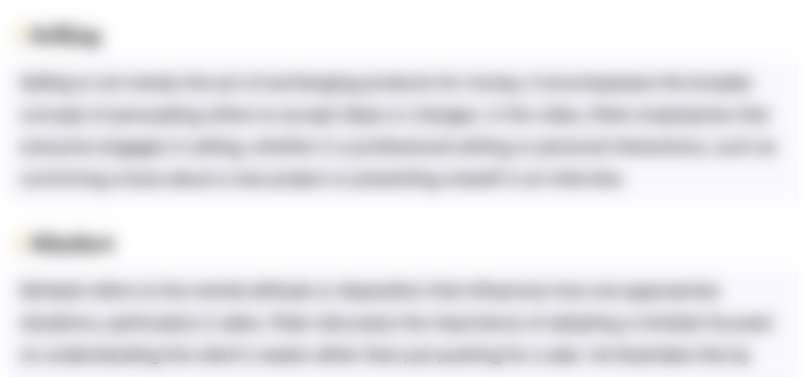
This section is available to paid users only. Please upgrade to access this part.
Upgrade NowHighlights
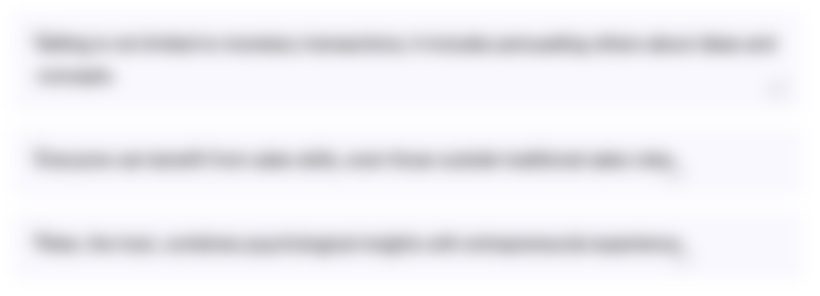
This section is available to paid users only. Please upgrade to access this part.
Upgrade NowTranscripts
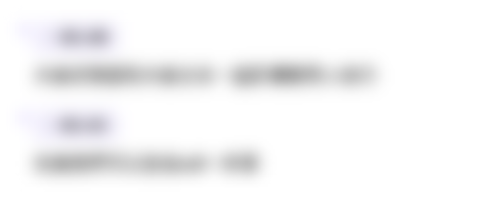
This section is available to paid users only. Please upgrade to access this part.
Upgrade NowBrowse More Related Video
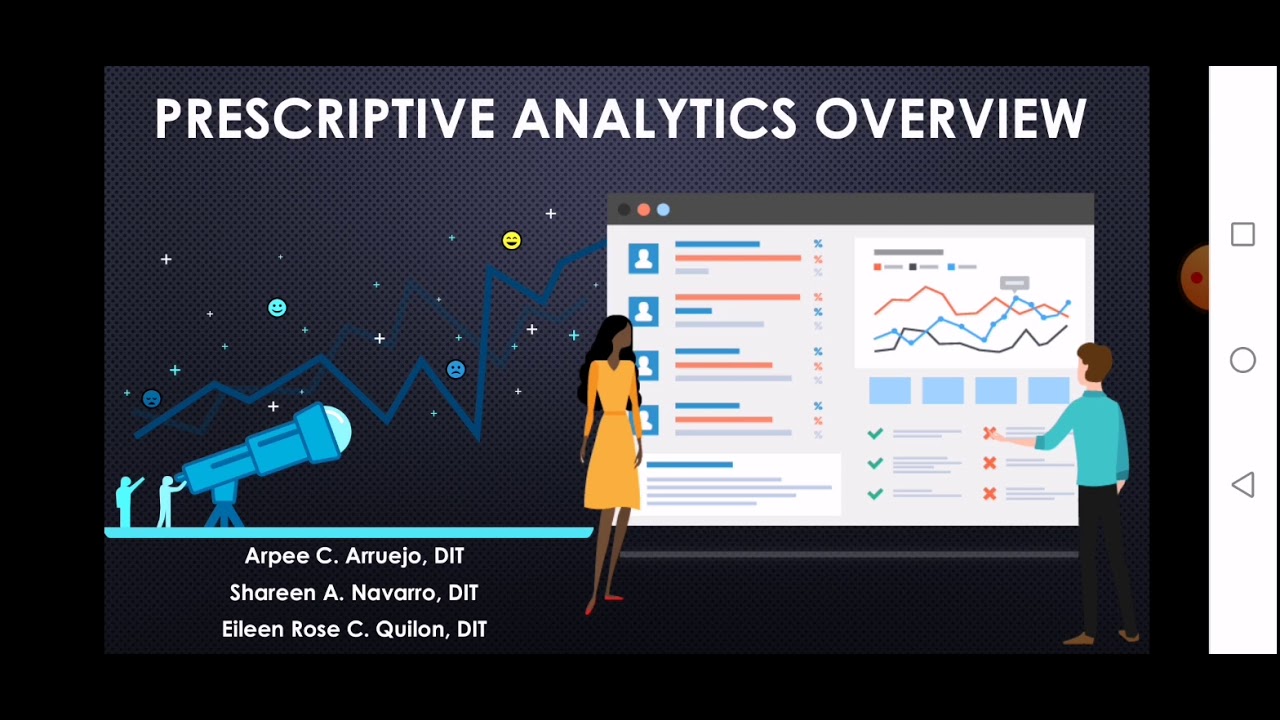
Prescriptive Analytics Overview
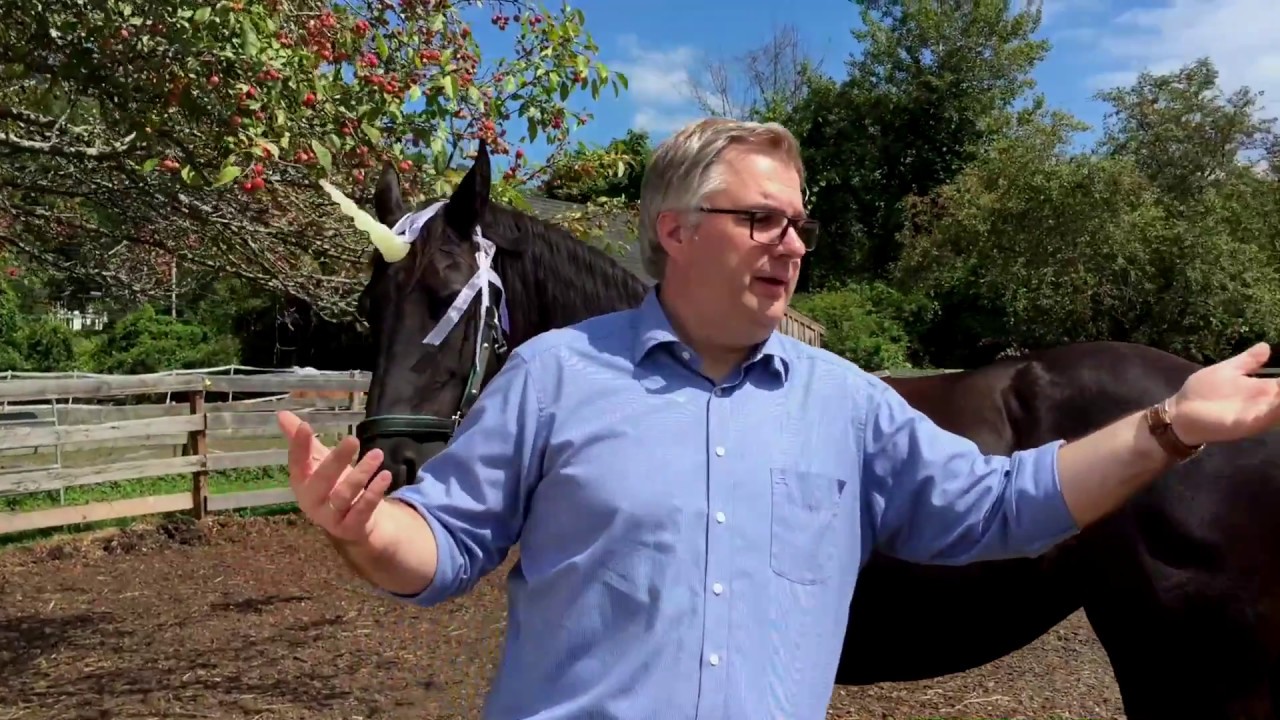
[S2E1] Prescriptive Analytics | 5 Minutes With Ingo

Types of Business Analytics (Descriptive, Predictive, and Prescriptive)
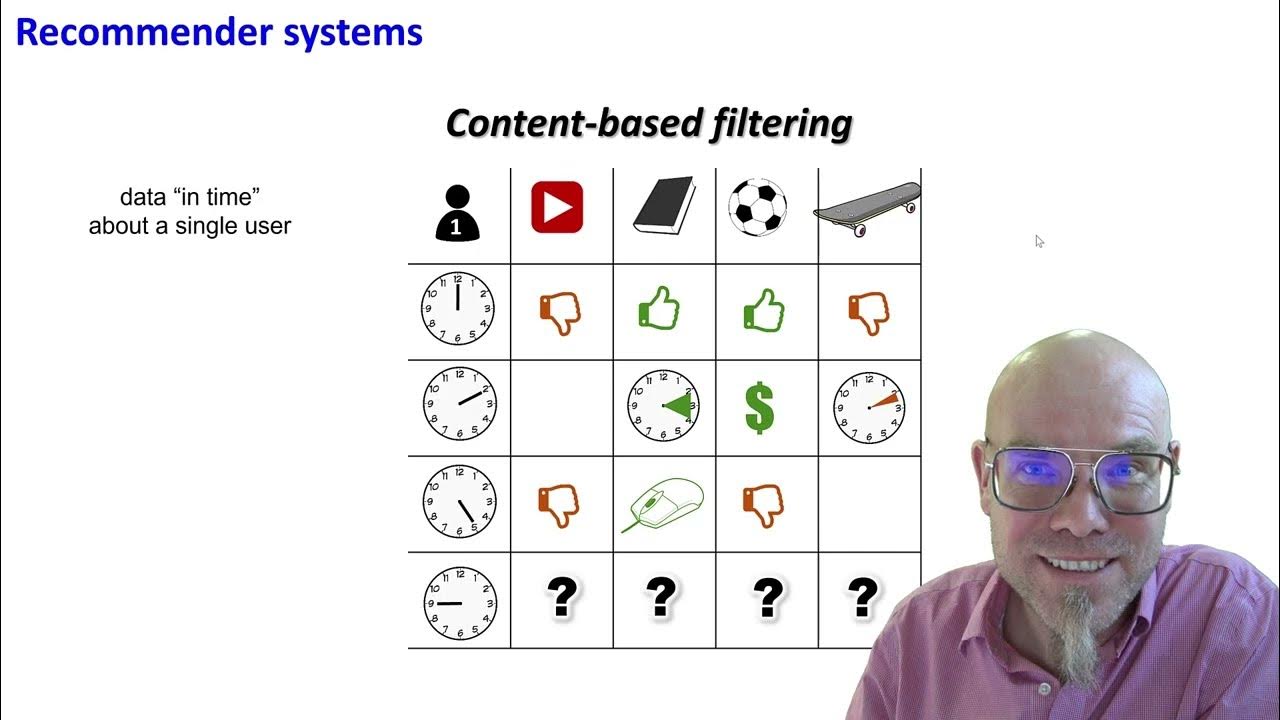
DTSC: 3.3 Prediction Machines and their recommender engines (or: what algorithms know from our past)
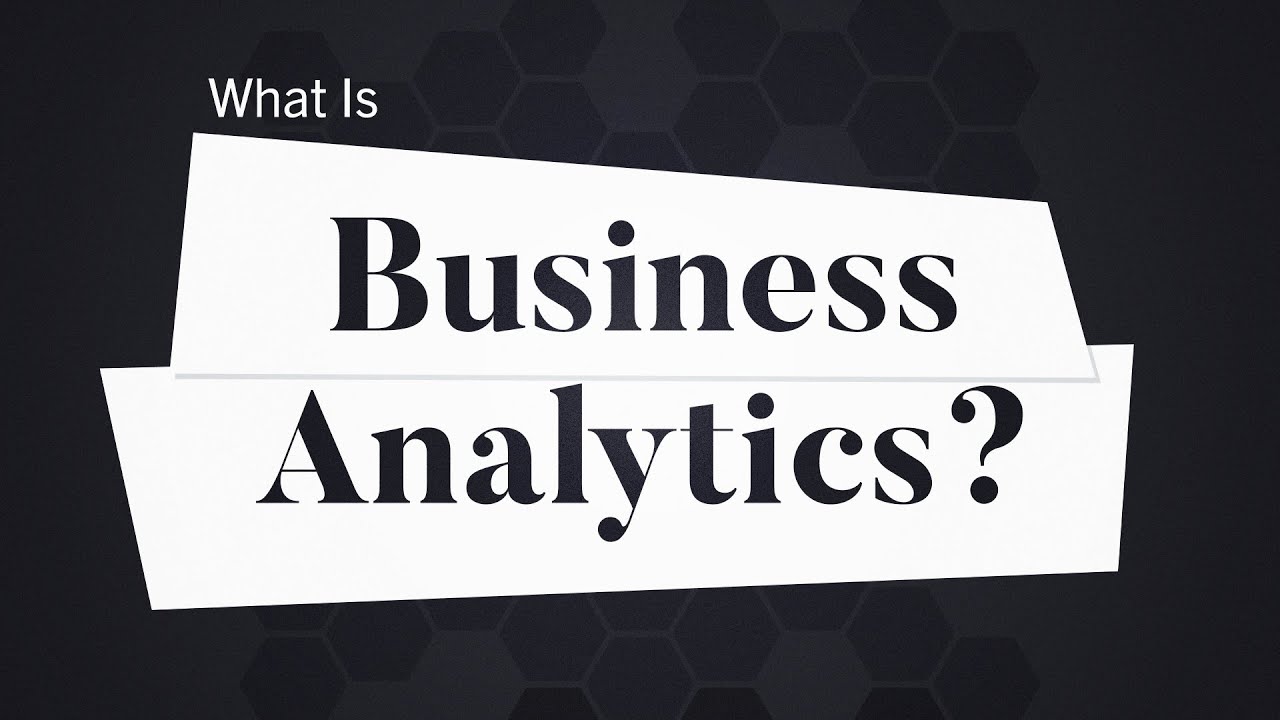
What Is Business Analytics? | Business: Explained

What do tech companies know about your children? | Veronica Barassi | TEDxMileHigh
5.0 / 5 (0 votes)