Webinar - Supply Chain Optimization: A Robust Supply That Minimizes Costs
Summary
TLDR本次演讲主要围绕供应链优化展开,介绍了一种旨在最小化成本并适应需求不确定性的鲁棒供应链模型。演讲者Fernando Piqueras,作为City of RAI的商业研究开发者,与FICO合作开发了一个决策支持系统,该系统使用FICO Express Insight平台进行库存管理。通过蒙特卡洛模拟和线性规划(MILP)技术,系统能够预测客户需求,优化库存水平,并在不同情况下做出最佳的订购和运输决策。此外,演讲还展示了如何通过用户友好的界面进行数据管理、模拟执行和结果分析,以及如何通过场景管理器快速比较不同策略的影响。整个演示强调了在不确定性环境下,如何利用先进的分析工具来提高供应链的灵活性和效率。
Takeaways
- 📈 **供应链优化目标**:创建一个能够应对需求不确定性的稳健供应链,以最小化成本并最大化需求满足率。
- 🤖 **决策支持系统**:开发了一个基于FICO Express Insight平台的决策支持系统,用于库存管理。
- 👨💼 **演讲者背景**:Fernando Piqueras作为业务研究开发人员,拥有在零售、运输和物流行业的丰富经验。
- 📚 **合作与技术**:与FICO合作,利用其领先的分析解决方案,通过操作研究技术提供定制解决方案。
- 🔄 **灵活性与敏捷性**:平台能够快速适应变化,为业务提供最佳策略,并加速实现业务目标。
- 📊 **多场景模拟**:通过蒙特卡洛模拟,能够可视化多种可能的业务结果,帮助分析师和业务用户进行决策。
- 📦 **库存管理决策**:需要决定向供应商订购的产品数量以及从哪个仓库向客户交付产品。
- 📈 **成本效益分析**:考虑了最小化库存成本和分销成本,包括订单处理和产品存储成本。
- 🗺️ **地理分布考虑**:模型考虑了地理位置,以确定最短距离和最低成本的配送路线。
- 📊 **关键绩效指标(KPIs)**:通过定义KPIs来衡量解决方案的表现,包括成本和服务水平协议(SLA)。
- 🔧 **实时模拟与分析**:应用允许用户进行实时模拟,观察不同决策对供应链和成本的影响。
Q & A
什么是供应链优化的主要目标?
-供应链优化的主要目标是创建一个能够应对需求不确定性的稳健供应链,以最大化需求满足率,同时最小化库存成本和分销成本。
Fernando Piqueras 在哪个领域工作,并且他的主要工作内容是什么?
-Fernando Piqueras 是一位商业研究开发者,他在 City of RAI 工作,专注于使用运筹学技术为客户创建定制解决方案,涉及零售、运输和物流等多个项目。
FICO 在供应链优化中扮演了什么角色?
-FICO 是世界领先的分析解决方案和服务提供商,它通过其平台帮助组织更好地理解客户,专注于优化业务流程。在供应链优化中,FICO 提供了技术支持,使得项目能够快速扩展并迅速应用于业务。
在供应链优化中,为什么需要一个决策支持系统?
-决策支持系统对于库存管理至关重要,因为它可以帮助应对需求的不确定性,提供基于数据的决策支持,从而提高供应链的灵活性和响应能力。
在供应链优化问题中,有哪些关键的决策变量?
-关键的决策变量包括向供应商下达的订单量、仓库的存储量、从哪个仓库向哪个客户配送产品,以及如何处理产品从供应商到仓库、从仓库到客户的运输。
如何使用 FICO 平台来开发供应链优化的解决方案?
-使用 FICO 平台,可以在没有先验知识的情况下从零开始开发解决方案。通过 FICO Express Optimization 软件,可以解决和优化混合整数线性规划(MILP)模型,同时 FICO Express Insight 提供了用户界面,允许用户管理数据并可视化可能的业务场景。
在供应链优化中,如何处理地理分布对成本和配送时间的影响?
-在供应链优化模型中,需要考虑供应商、仓库和客户之间的地理分布,以确定最短的运输距离,从而最小化运输成本和配送时间。
在供应链优化的第一阶段和第二阶段中,分别需要做出哪些决策?
-第一阶段主要是根据需求预测向供应商下达订单,决定每个仓库在特定时间内对特定产品的订单量。第二阶段是在已知实际需求后,决定从哪个仓库向哪个客户配送多少产品。
如何通过 FICO Express Insight 平台进行实时供应链优化演示?
-通过 FICO Express Insight 平台,用户可以登录到服务器,通过不同的视图(如输入数据视图、输出结果视图、分析视图)来管理数据、执行模型、查看结果,并进行实时演示。平台提供了交互式的用户界面,允许用户加载数据、运行模拟、查看订单和配送情况,并对不同场景进行分析。
在供应链优化的实时演示中,如何评估解决方案的效果?
-通过关键绩效指标(KPIs)来评估解决方案的效果。这些 KPIs 包括总成本(如订单成本、配送成本、库存成本)、服务水平协议(SLA,即满足需求的比例)等。用户可以在 FICO Express Insight 平台上查看这些 KPIs,并根据需要自定义它们。
在供应链优化中,如何考虑不同的业务场景和假设情况?
-通过创建不同的场景,用户可以模拟不同的业务场景和假设情况,如不同的需求预测、仓库配置变化或地理分布调整。FICO 平台允许用户快速修改参数并重新运行模型,以评估不同决策对供应链优化的影响。
Outlines
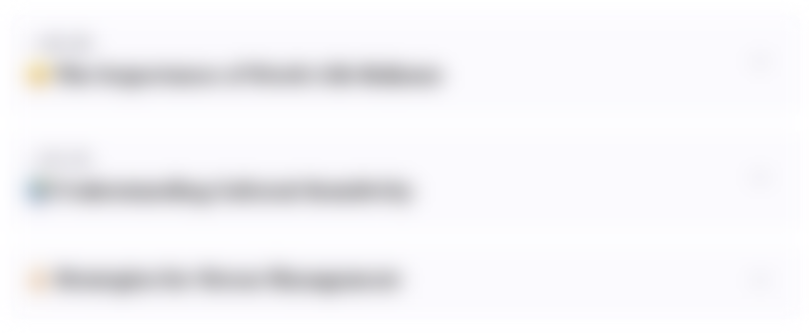
This section is available to paid users only. Please upgrade to access this part.
Upgrade NowMindmap
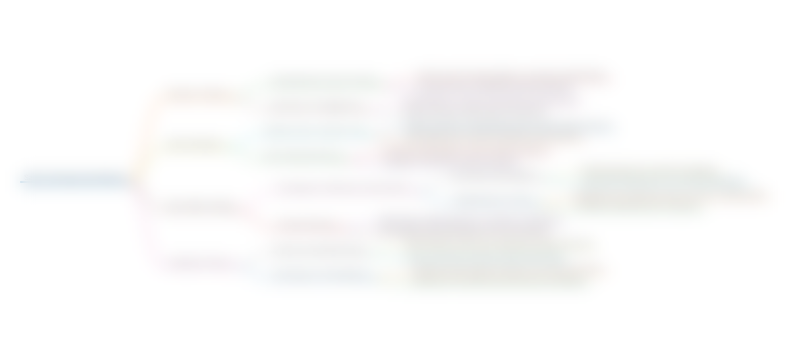
This section is available to paid users only. Please upgrade to access this part.
Upgrade NowKeywords
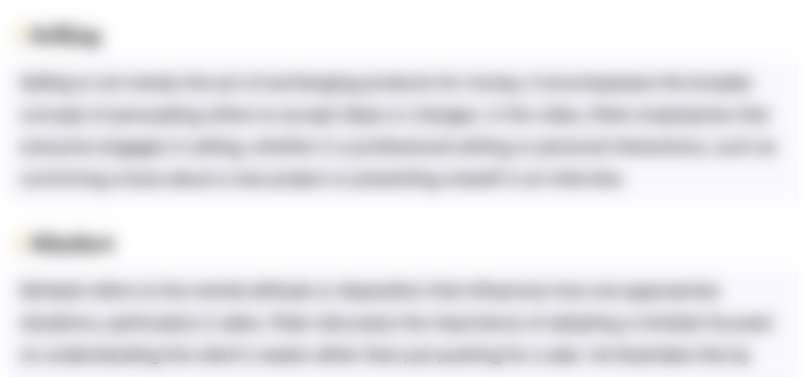
This section is available to paid users only. Please upgrade to access this part.
Upgrade NowHighlights
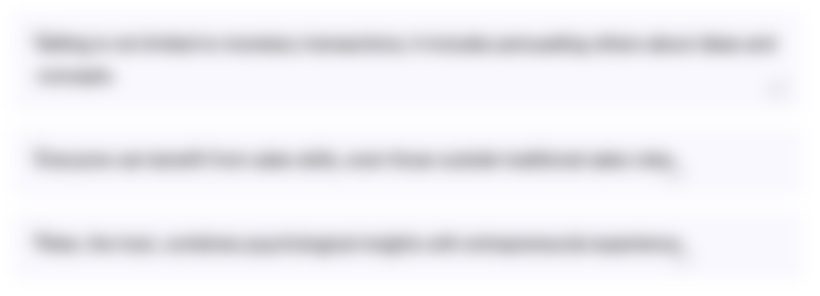
This section is available to paid users only. Please upgrade to access this part.
Upgrade NowTranscripts
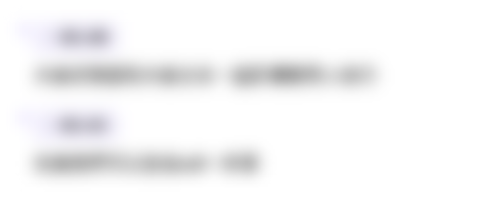
This section is available to paid users only. Please upgrade to access this part.
Upgrade NowBrowse More Related Video
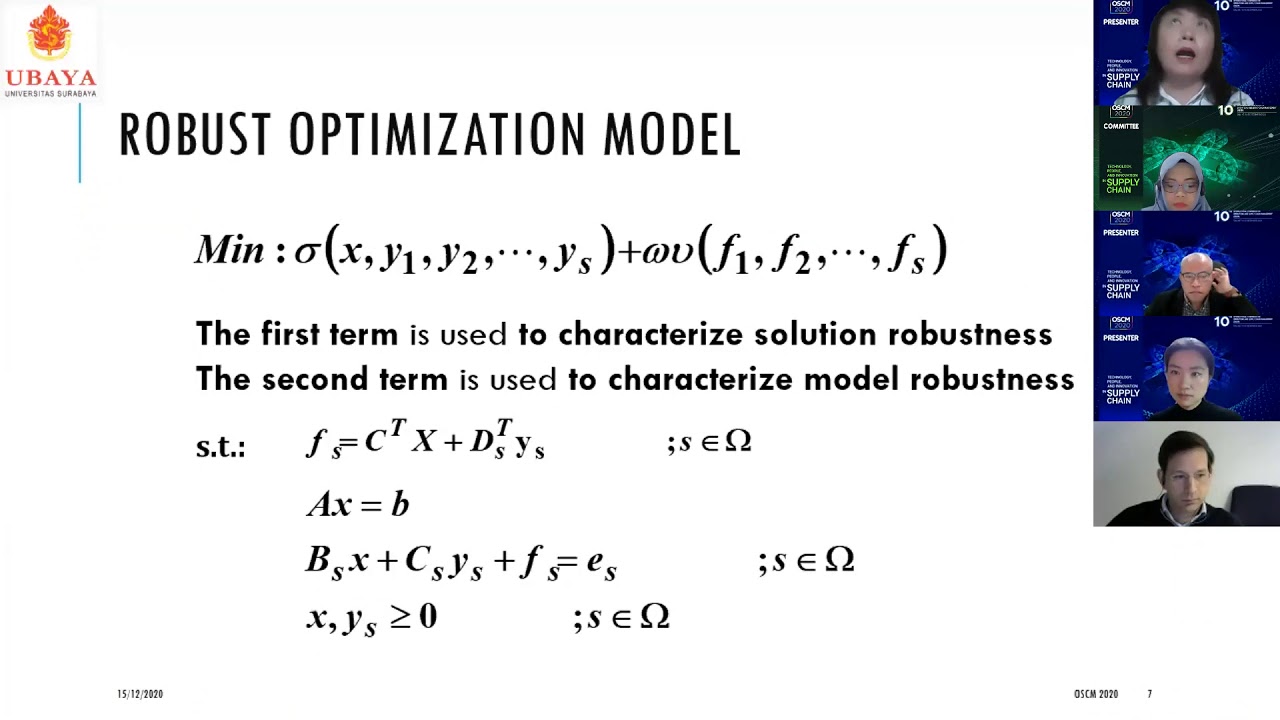
Supply Chain Modelling: Multi Objective Robust Optimization Model for Facility Layout Design
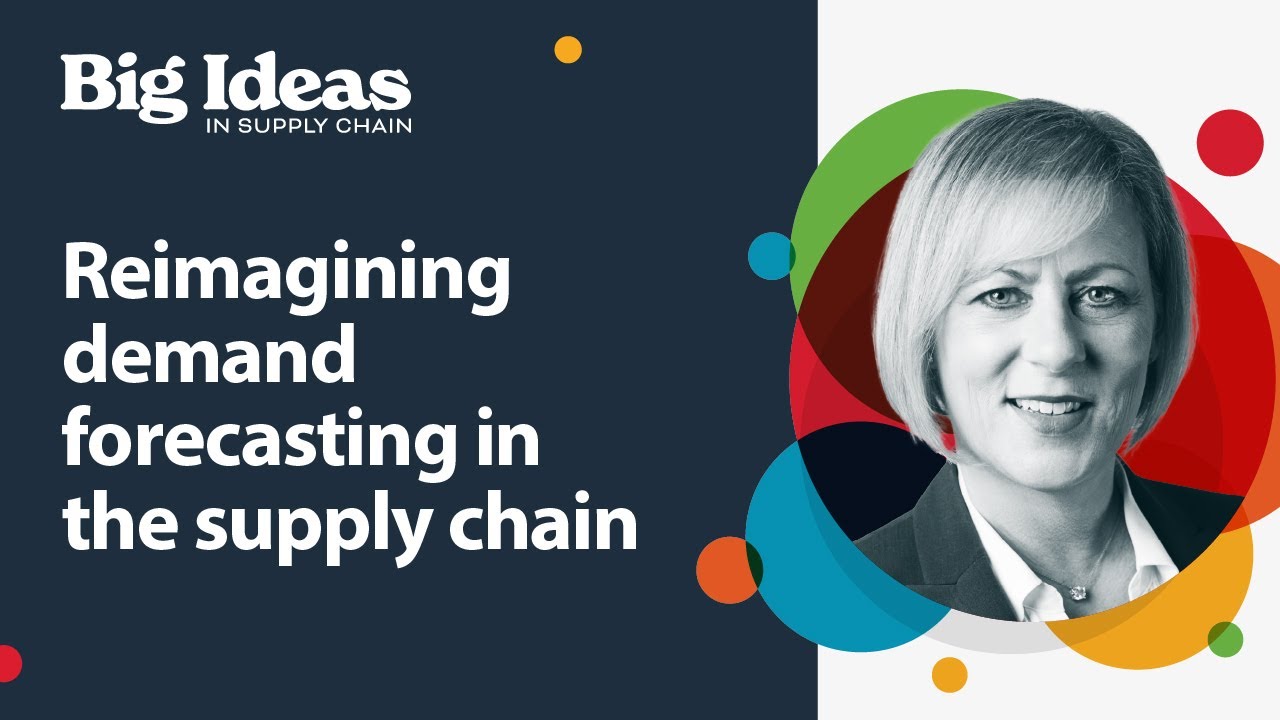
Reimagining demand forecasting in the supply chain
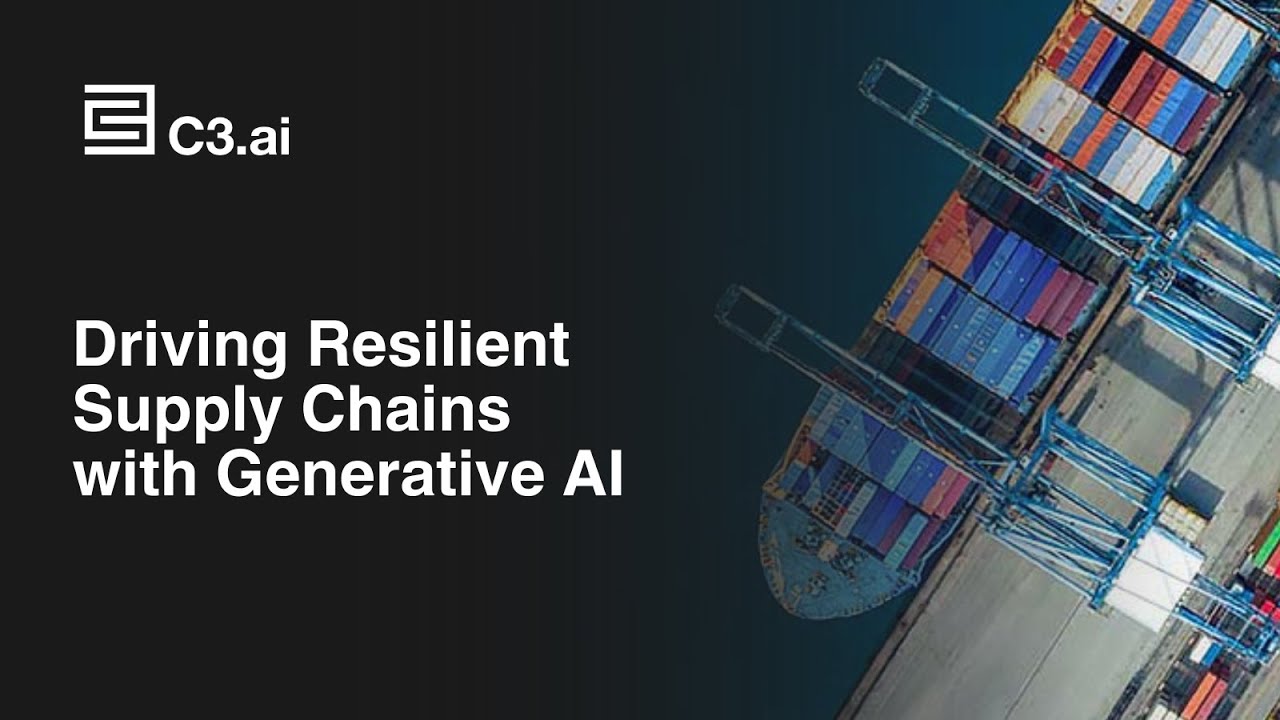
Generative AI - Driving Resilient Supply Chains
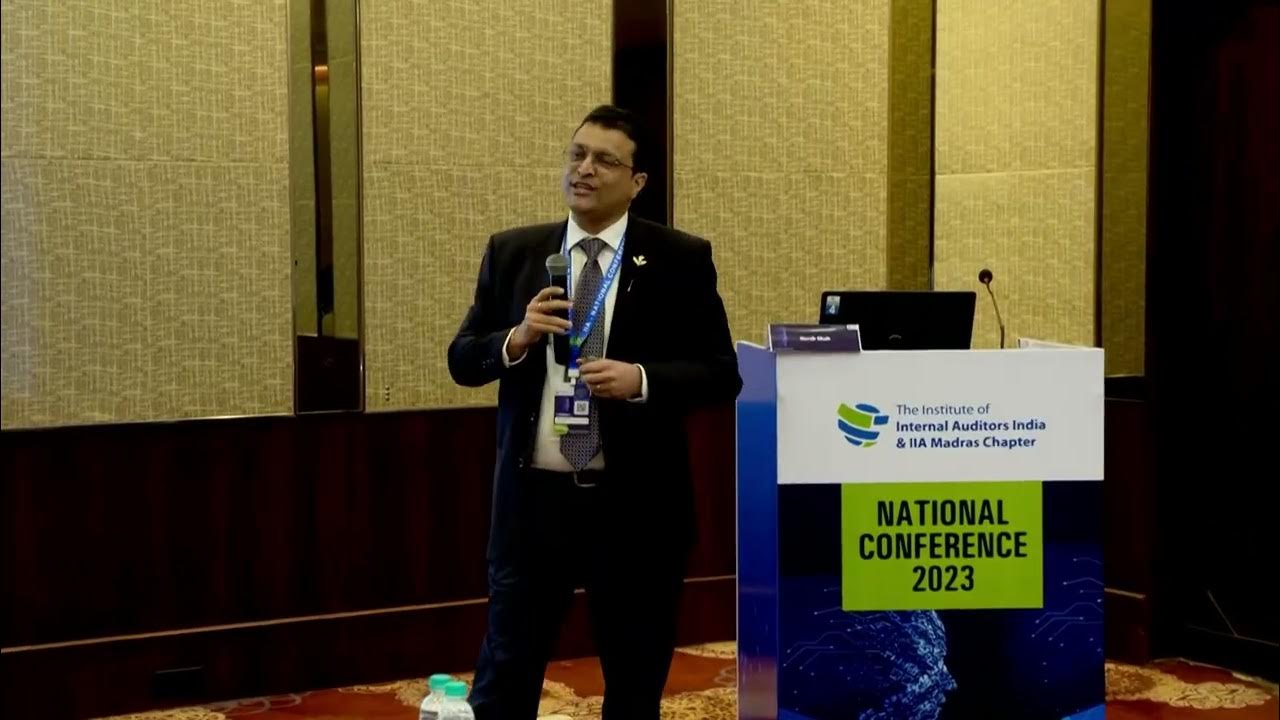
Track B - Evaluating Supply Chain Strains in their Race to Robustness, Flexibility & Resilience
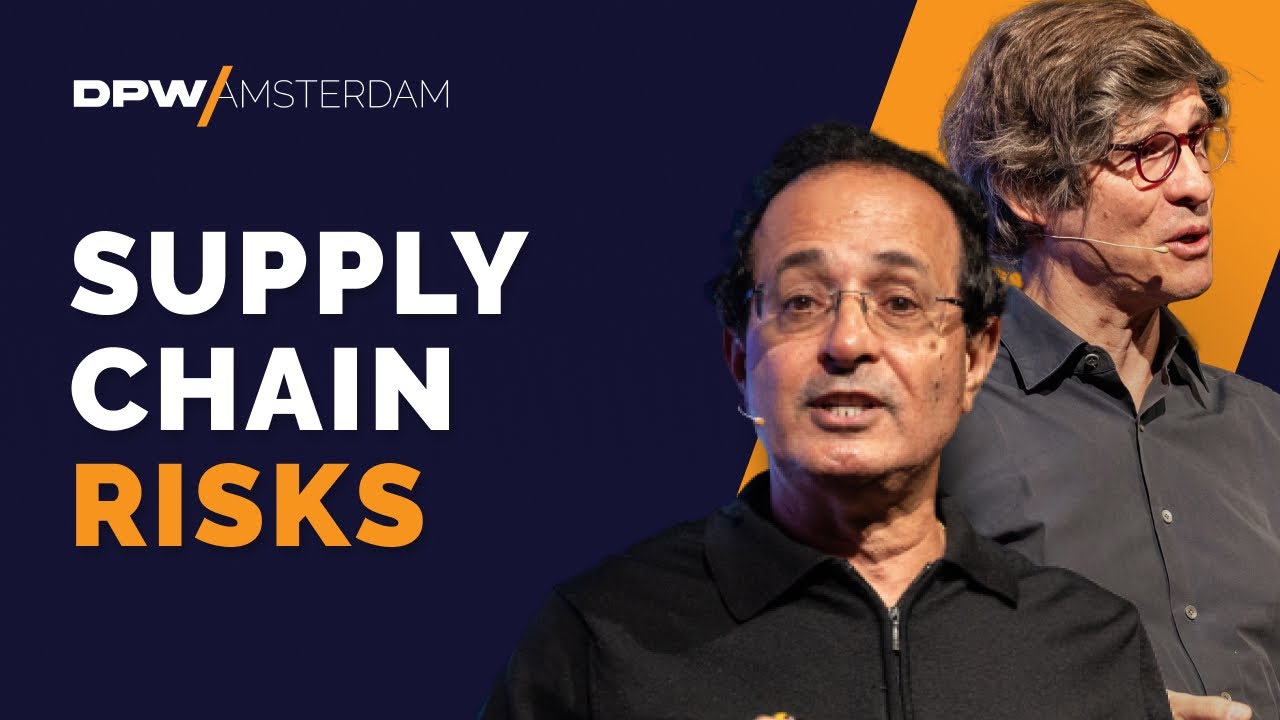
Proactive Strategies for Resilient Supply Chains: Mitigating Hidden Risks

AI-Driven Supply Chains: 3 Cases | MIT SCALE Webinar | English
5.0 / 5 (0 votes)