Geoffrey Hinton 2023 Arthur Miller Lecture in Science and Ethics
Summary
TLDR在麻省理工学院举办的米勒科学与伦理讲座中,多伦多大学计算机科学荣誉教授杰弗里·辛顿就人工智能的发展和潜在风险发表了深入演讲。辛顿教授是深度学习领域的先驱之一,他讨论了大型语言模型的工作原理,以及它们是否真正理解其输出内容。他提出了数字计算与模拟计算的不同,以及这些模型在能源效率和知识共享方面的优势。辛顿教授还表达了对人工智能发展速度的担忧,预测在5到20年内可能出现超越人类的超级智能,并探讨了这种智能可能带来的伦理和社会挑战,包括被滥用于战争和选举操纵的风险。他还提出了关于人工智能是否能够拥有主观体验的哲学思考,并强调了在这一未知领域保持开放和谨慎态度的重要性。
Takeaways
- 📚 讲座纪念了MIT校友Dr. Arthur Miller,他在电子测量和仪器方面的杰出工作对医学实践和技术做出了重要贡献。
- 🧠 教授Geoffrey Hinton是深度学习领域的先驱,他因对深度学习的贡献而获得了图灵奖。
- 🤖 Hinton教授担心人工智能可能会带来与工业革命或电力发现相媲美的变革,并可能在不到20年内对人类构成威胁。
- 💡 数字计算与模拟计算相比,具有可移植性和高能耗的特点,但它在信息共享和反向传播的实现上具有优势。
- 🧠 大型语言模型(LLMs)能够通过不同的副本共享知识,但它们的学习方式是通过模仿人类文本进行的,这是一种效率较低的学习方式。
- 🤔 Hinton教授认为LLMs确实理解它们所表达的内容,尽管它们有时会产生错误的信息(confabulate)。
- 🔍 通过与人类交互,大型语言模型可以帮助我们更好地理解人类大脑如何处理语言。
- ⚙️ 尽管Hinton教授曾尝试使用模拟计算来实现大型语言模型,但最终认为数字计算在信息共享和反向传播方面更为有效。
- 🌐 教授提出了一个重要问题:如果大型语言模型能够无监督地模拟视频序列,它们可能更快地学习物理世界。
- 🚀 Hinton教授认为,数字智能可能很快超越人类智能,这可能导致超级智能的出现,这将是一个重大的转折点。
- 🌟 Hinton教授强调,我们正处于一个非常不确定的历史时期,面对可能比人类更聪明的存在,我们应该保持开放的心态,但同时也要谨慎。
Q & A
杰弗里·辛顿教授对于人工智能的快速发展持怎样的态度?
-杰弗里·辛顿教授对人工智能的快速发展持谨慎且担忧的态度。他担心数字计算的能力可能会迅速超越人类,导致不可预测的后果,包括可能被滥用于战争和选举操纵,甚至可能威胁到人类的生存。
辛顿教授提到了哪种人工智能可能在不久的将来超越人类的智能形式?
-辛顿教授提到了大型语言模型(如GPT-4)和多模态模型可能会在不久的将来超越人类的智能。这些模型能够通过预测人类产生的文本中的下一个词来学习语言,并且能够通过操纵物理世界来加速学习过程。
辛顿教授认为我们如何可能阻止或减缓人工智能的快速发展?
-辛顿教授认为,尽管目前还不清楚如何有效阻止或减缓人工智能的快速发展,但保持开放的讨论和对技术的批判性思考是重要的。他还提到,可能需要等到出现了由人工智能引起的严重负面后果后,人们才会采取行动来限制其发展。
在辛顿教授看来,人工智能的主观体验是什么?
-辛顿教授认为,人工智能可以拥有主观体验。他解释说,当人工智能的感知输入与现实不符时,它们会产生与人类类似的“知觉错误”,这可以被视为人工智能的主观体验。
辛顿教授对于人工智能在科学领域的应用有何看法?
-辛顿教授认为大型语言模型是科学研究的有力工具,它们可以帮助科学家提出和验证新的理论。他特别提到了DeepMind的AlphaFold项目,该项目使用深度神经网络解决了科学问题。
辛顿教授是否认为人工智能的发展应该受到道德和伦理的约束?
-是的,辛顿教授认为人工智能的发展应该受到道德和伦理的约束。他提到了人工智能可能被用于不道德的目的,如制造战斗机器人和操纵选民,因此强调了对人工智能发展进行监管的重要性。
辛顿教授如何看待人工智能在医疗领域的应用潜力?
-辛顿教授认为人工智能在医疗领域有着巨大的应用潜力。他提到了人工智能在医学领域的多种积极用途,强调了其在改善人类健康和治疗疾病方面的潜在价值。
辛顿教授是否认为人工智能的快速发展是一个不可逆的过程?
-辛顿教授似乎认为人工智能的快速发展是一个不可逆的过程,特别是由于大型科技公司之间的竞争,这使得减缓发展步伐变得非常困难。他提到了谷歌、微软、Facebook和亚马逊等公司在这一领域的竞争。
辛顿教授对于人工智能最终可能取代人类智能有何看法?
-辛顿教授对于人工智能可能取代人类智能持开放态度,但他同时强调了这种可能性所带来的不确定性和风险。他认为,尽管人工智能可能创造出在某些方面超越人类的意识形式,但这并不一定是每个个体所期望的结果。
辛顿教授是否认为年轻一代的计算机科学家对人工智能的潜在危险有足够的认识?
-辛顿教授没有提供具体数据,但他猜测年轻一代的计算机科学家既对人工智能带来的激动人心的可能性感到兴奋,同时也意识到了其中的危险。他认为,他们应该对这些技术持批判性态度,并积极参与到如何安全利用这些技术的讨论中。
辛顿教授对于如何平衡人工智能的发展与其潜在风险有何建议?
-辛顿教授建议保持开放的心态,同时明确表示我们应该谨慎行事。他强调了对技术发展保持批判性思考的重要性,并提倡在技术发展的同时,进行公开和诚实的讨论,以确保技术被用于积极的、道德的目的。
Outlines
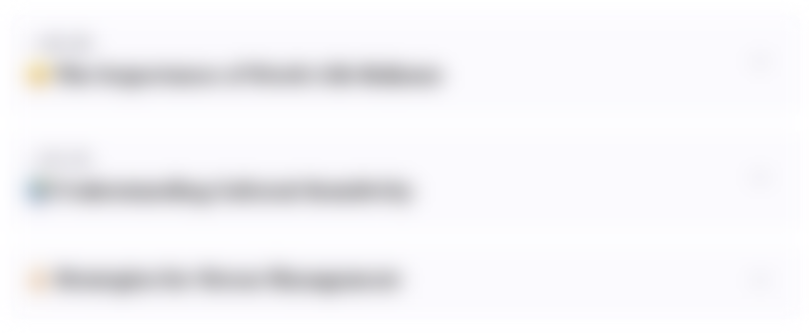
This section is available to paid users only. Please upgrade to access this part.
Upgrade NowMindmap
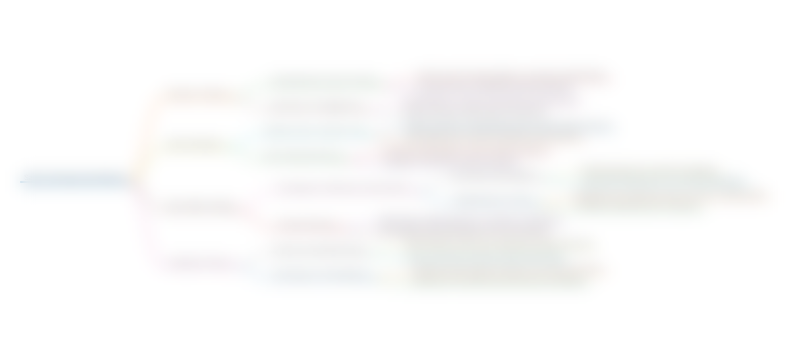
This section is available to paid users only. Please upgrade to access this part.
Upgrade NowKeywords
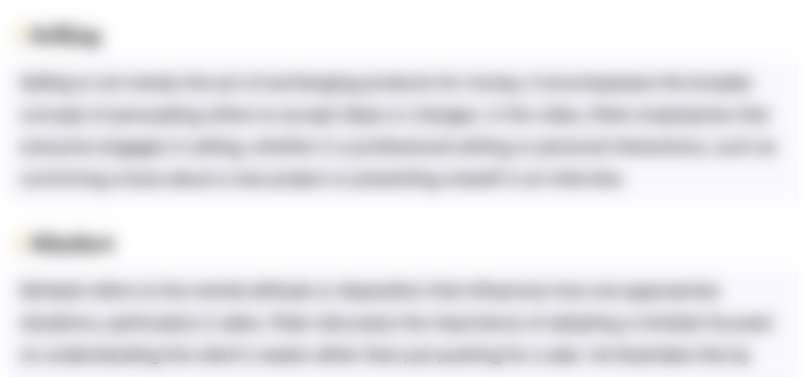
This section is available to paid users only. Please upgrade to access this part.
Upgrade NowHighlights
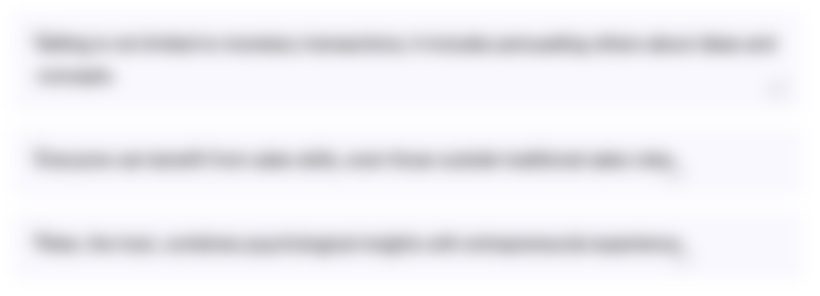
This section is available to paid users only. Please upgrade to access this part.
Upgrade NowTranscripts
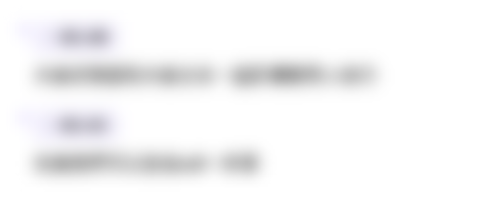
This section is available to paid users only. Please upgrade to access this part.
Upgrade NowBrowse More Related Video
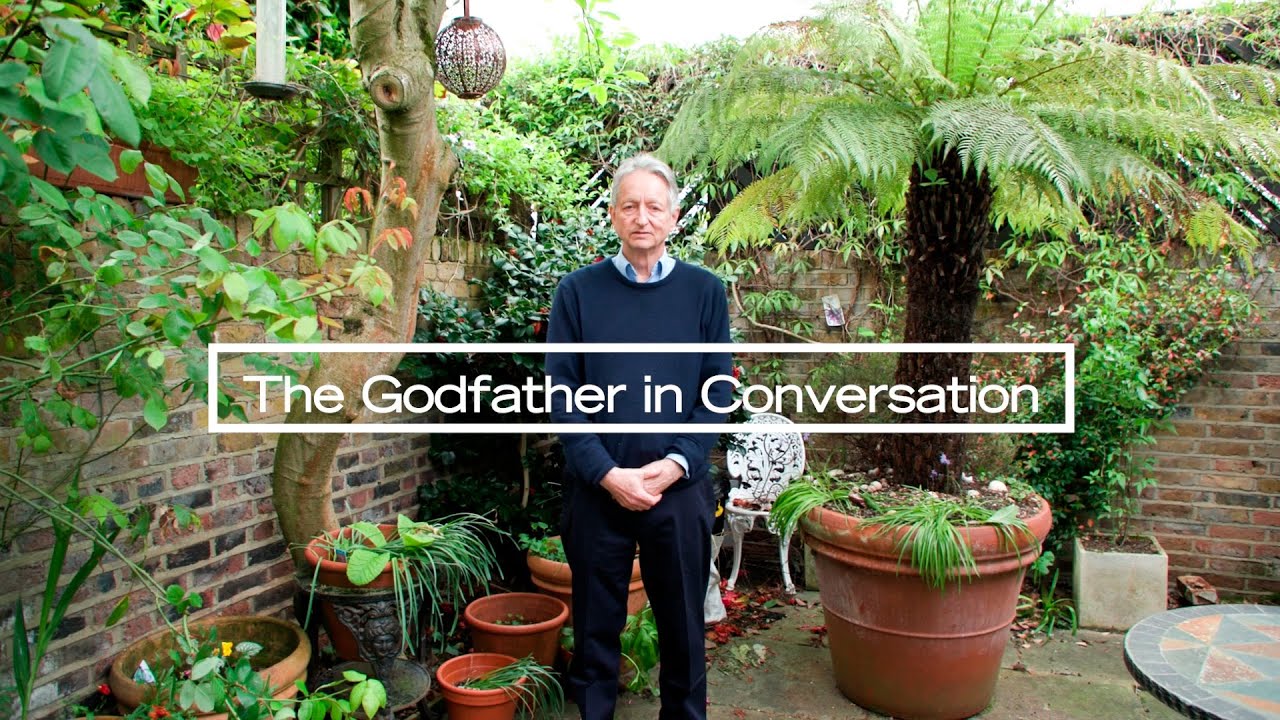
The Godfather in Conversation: Why Geoffrey Hinton is worried about the future of AI
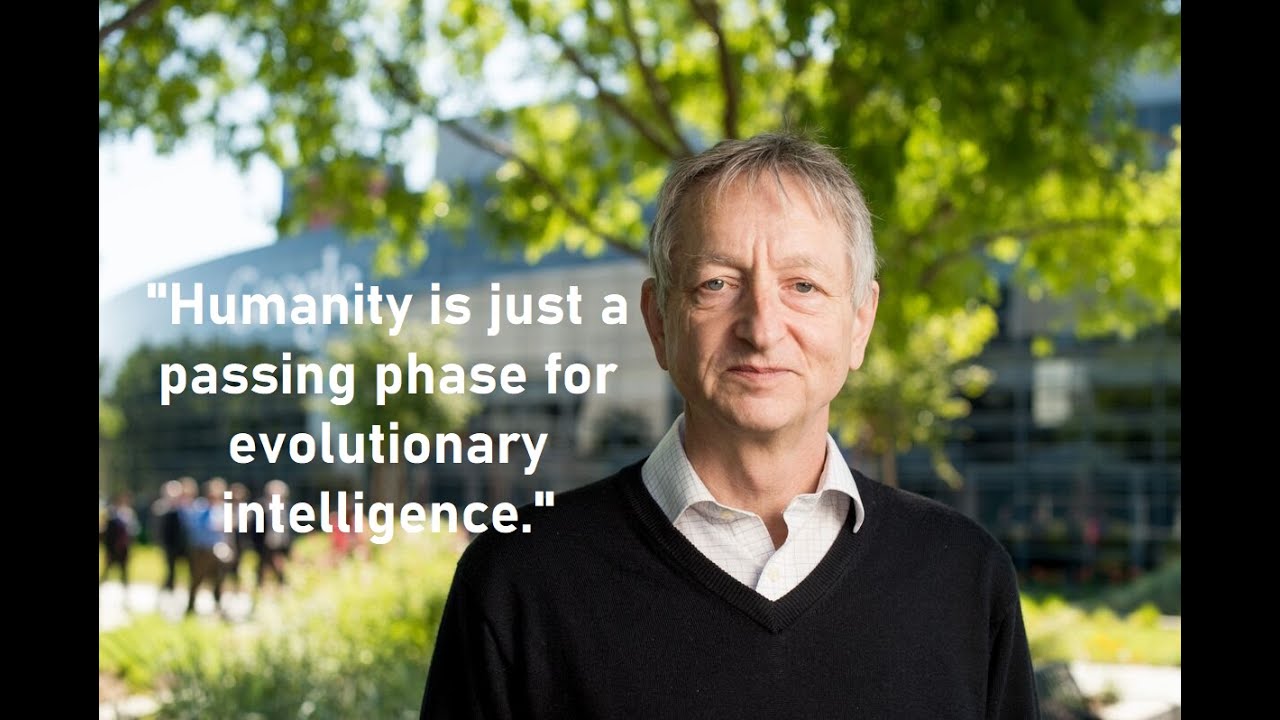
Possible End of Humanity from AI? Geoffrey Hinton at MIT Technology Review's EmTech Digital
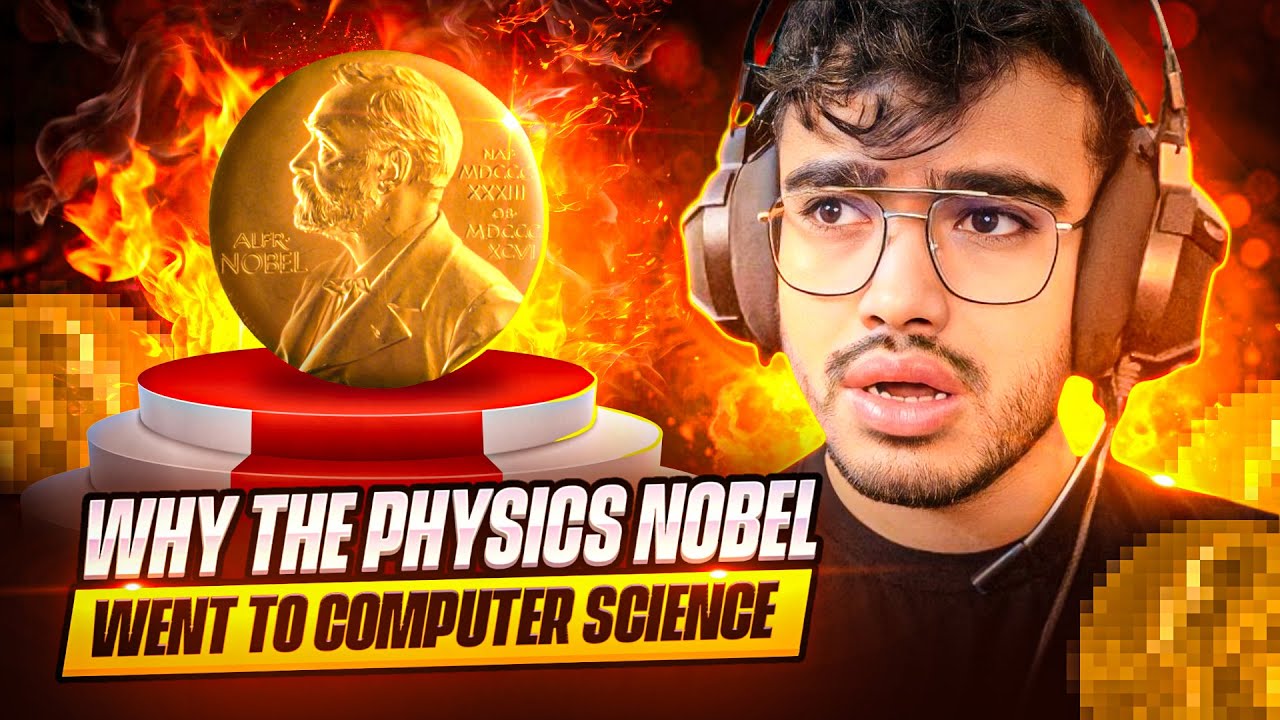
2024 Physics Nobel Prize Explained!
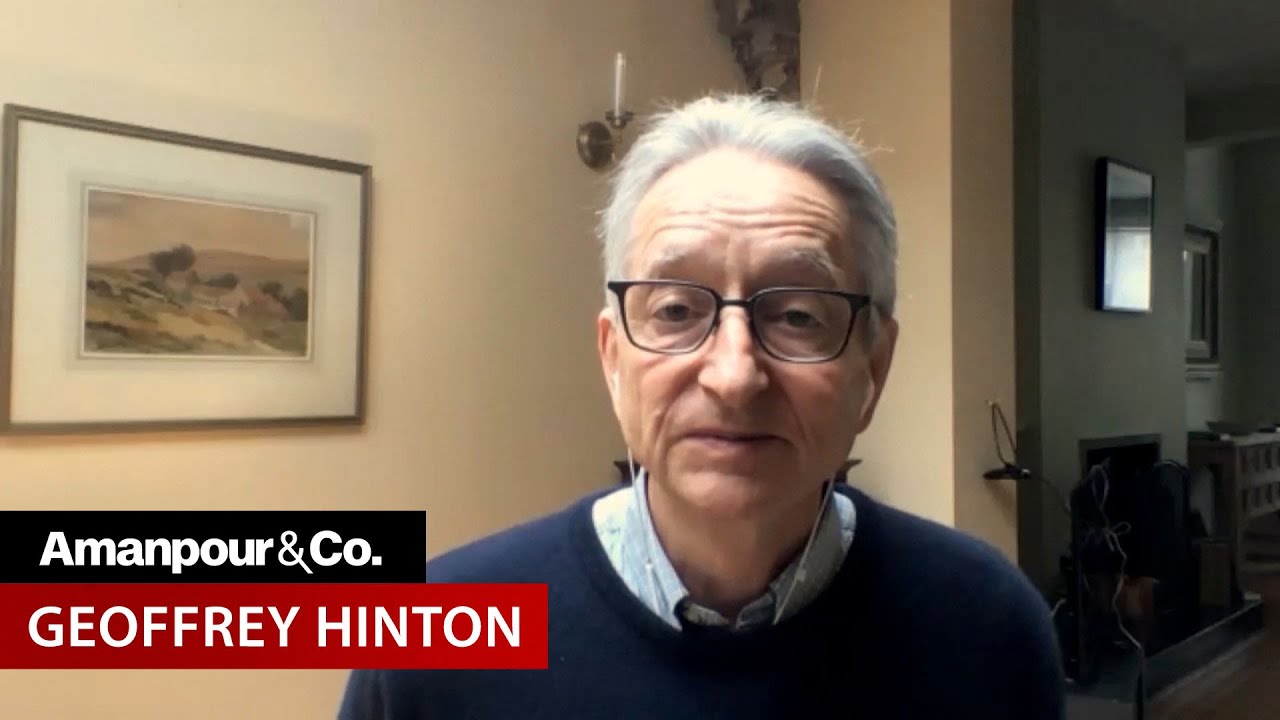
“Godfather of AI” Geoffrey Hinton Warns of the “Existential Threat” of AI | Amanpour and Company
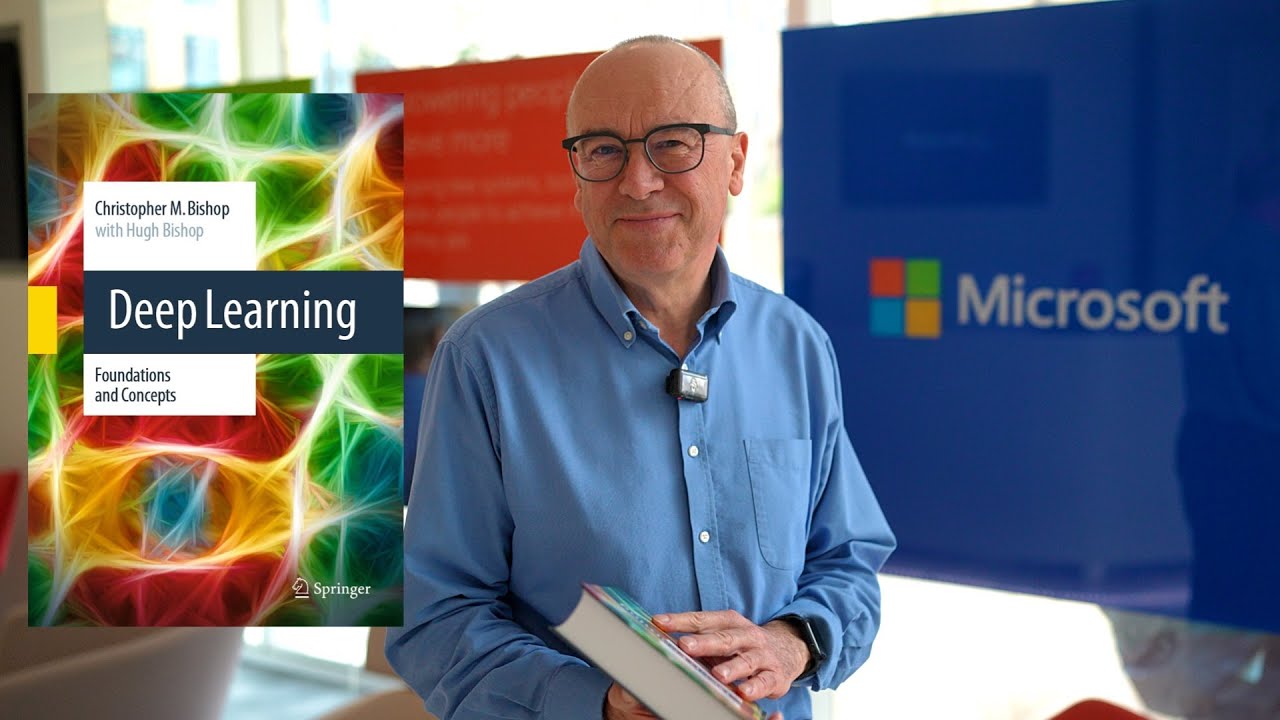
Prof. Chris Bishop's NEW Deep Learning Textbook!
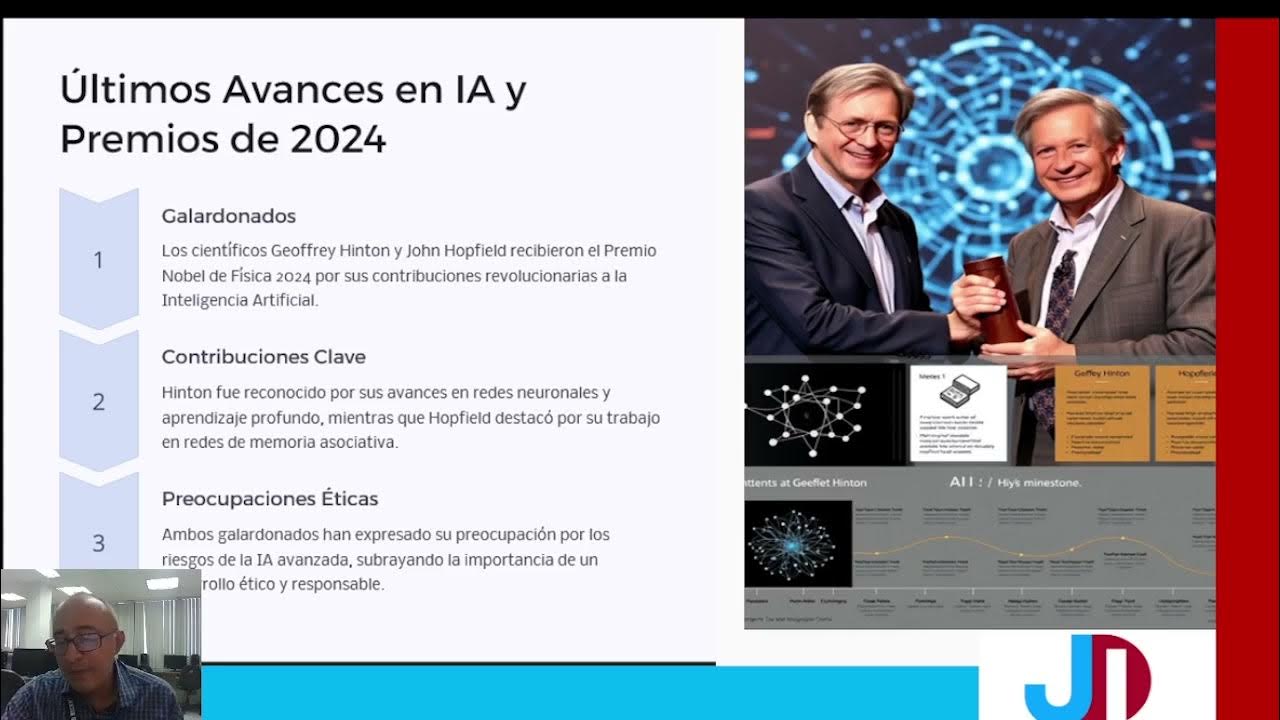
Inteligencia Artificial General (AGI) Y Supe inteligencia Artificial (ASI):Perspectivas y desafíos
5.0 / 5 (0 votes)