Cumulative Distribution Function for Continuous RV
Summary
TLDRThis video explains the cumulative distribution function (CDF) for continuous random variables, highlighting its properties and how to derive it from the probability density function (PDF). It compares the methods for finding probabilities using the CDF and PDF, demonstrating the process with practical examples. The video emphasizes integration for finding CDF from PDF and differentiation for the reverse process. Additionally, it provides examples to illustrate these concepts, including calculations and graphical representations of both CDF and PDF, making the theory accessible and engaging for viewers.
Takeaways
- π The video emphasizes the importance of mental health awareness in educational settings.
- π It discusses the impact of stress and anxiety on student performance and well-being.
- π Practical strategies are offered for managing stress, such as mindfulness and time management techniques.
- π The role of educators in fostering a supportive environment for students is highlighted.
- π The video advocates for integrating mental health education into the curriculum.
- π It addresses the stigma associated with seeking help for mental health issues.
- π The importance of parental involvement in supporting children's mental health is discussed.
- π It encourages open dialogue about mental health among peers and within families.
- π The video outlines resources available for students struggling with mental health challenges.
- π The overall message promotes a holistic approach to education that includes mental and emotional support.
Q & A
What is the cumulative distribution function (CDF) for continuous random variables?
-The CDF for continuous random variables, denoted as F(x), represents the probability that the random variable X takes on a value less than or equal to x. It is obtained by integrating the probability density function (PDF) from a lower limit up to x.
How does the CDF differ between discrete and continuous random variables?
-For discrete random variables, the CDF is calculated by summing the probabilities of the outcomes up to x. In contrast, for continuous random variables, the CDF is found by integrating the PDF over an interval.
What notation is used for the cumulative distribution function?
-The notation used for the cumulative distribution function is F or sometimes capital F.
What is the process to find the probability of a continuous random variable falling between two values a and b?
-To find the probability that a continuous random variable falls between two values a and b, you can use the CDF by calculating F(b) - F(a) or by integrating the PDF from a to b.
How can one derive the PDF from a given CDF?
-To derive the PDF from a given CDF, you differentiate the CDF with respect to x, resulting in the PDF.
What is the relationship between the probability density function and the cumulative distribution function?
-The relationship is that the CDF is the integral of the PDF, while the PDF is the derivative of the CDF.
What example was given in the transcript to find the CDF?
-The example discussed involved finding the CDF for a random variable X given the probability density function for X greater than 0, which was expressed as 3e^(-3t).
How is integration used in finding the CDF from a PDF?
-Integration is used to compute the area under the curve of the PDF from the lower limit (typically 0) to the value x, allowing the calculation of the CDF.
What is the result of differentiating a constant CDF?
-Differentiating a constant CDF results in zero, indicating that there is no probability associated with that interval.
How do you plot the CDF and PDF based on the provided functions?
-To plot the CDF, you sketch the function based on the intervals defined (e.g., F(x) = 0 for x β€ 0, F(x) = x for 0 < x < 1, and F(x) = 1 for x β₯ 1). For the PDF, plot the constant value for the defined range and zero elsewhere.
Outlines
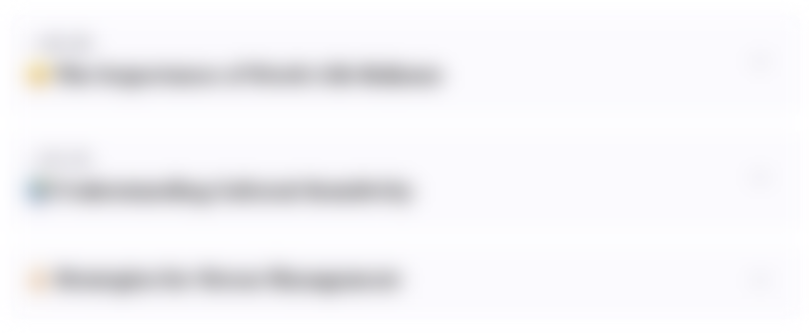
This section is available to paid users only. Please upgrade to access this part.
Upgrade NowMindmap
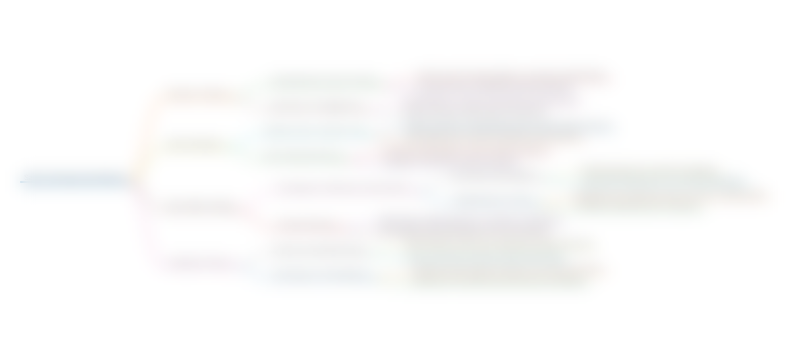
This section is available to paid users only. Please upgrade to access this part.
Upgrade NowKeywords
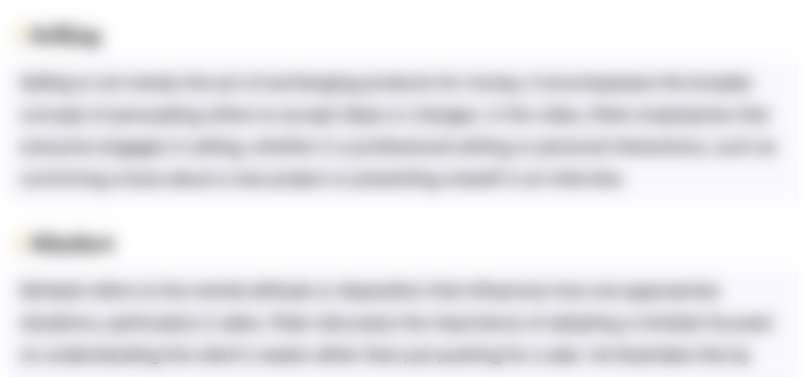
This section is available to paid users only. Please upgrade to access this part.
Upgrade NowHighlights
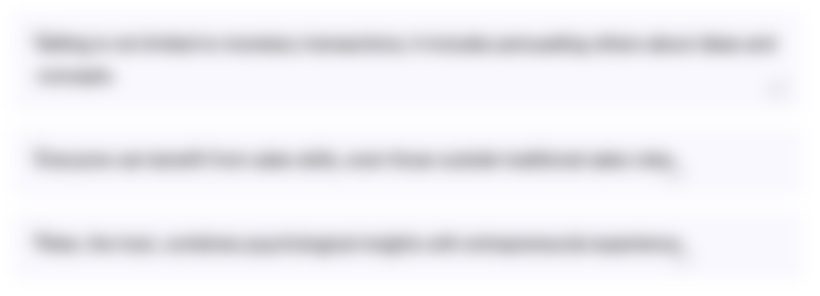
This section is available to paid users only. Please upgrade to access this part.
Upgrade NowTranscripts
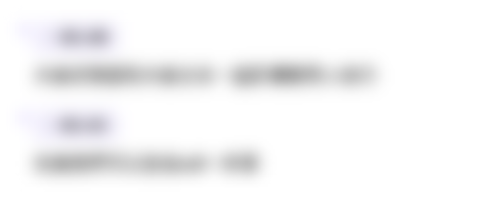
This section is available to paid users only. Please upgrade to access this part.
Upgrade NowBrowse More Related Video
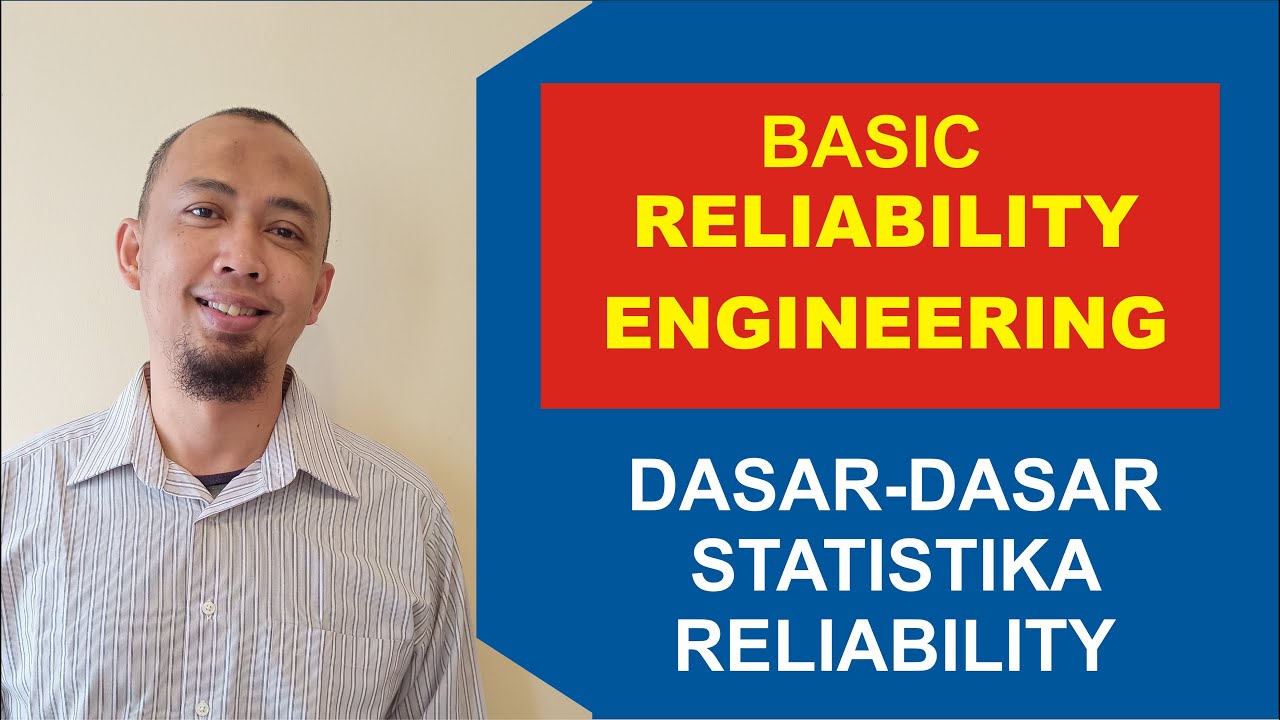
MENGHITUNG KEHANDALAN PRODUK-BASIC RELIABILITY ENGINEERING-Dasar Statistik Reliability-BHS INDONESIA
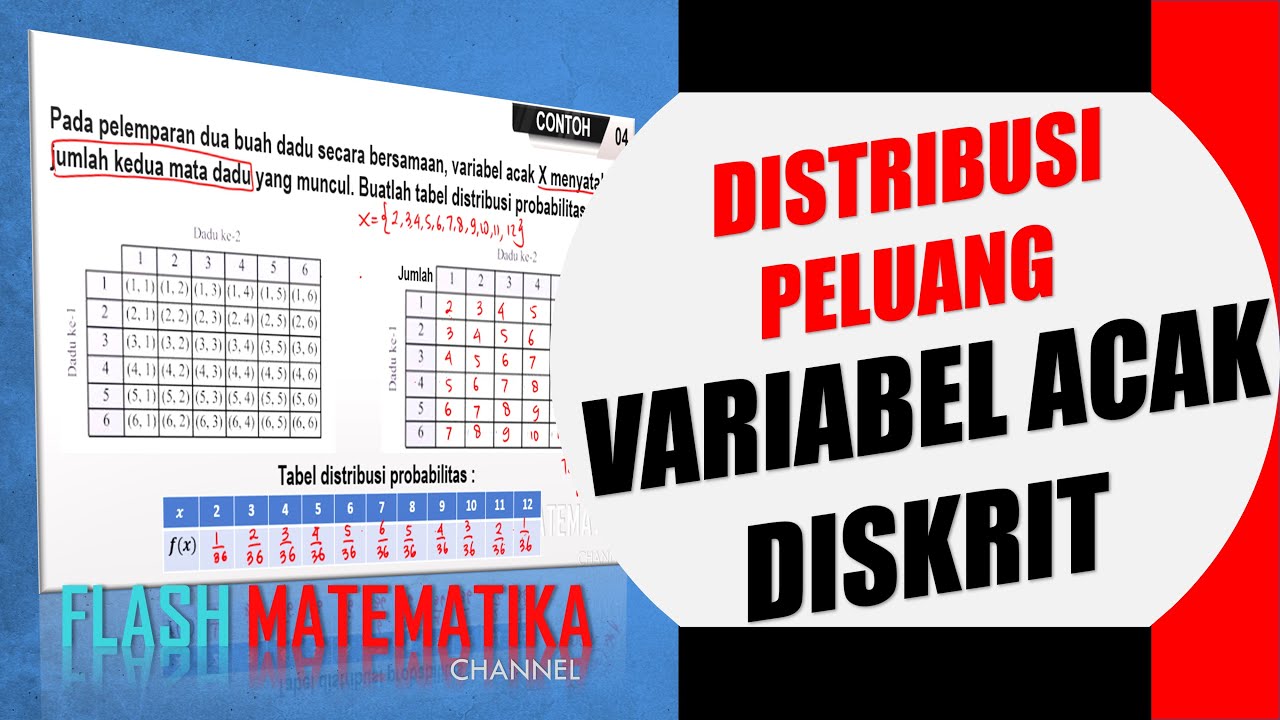
Distribusi Peluang Variabel Acak Diskrit Part 1
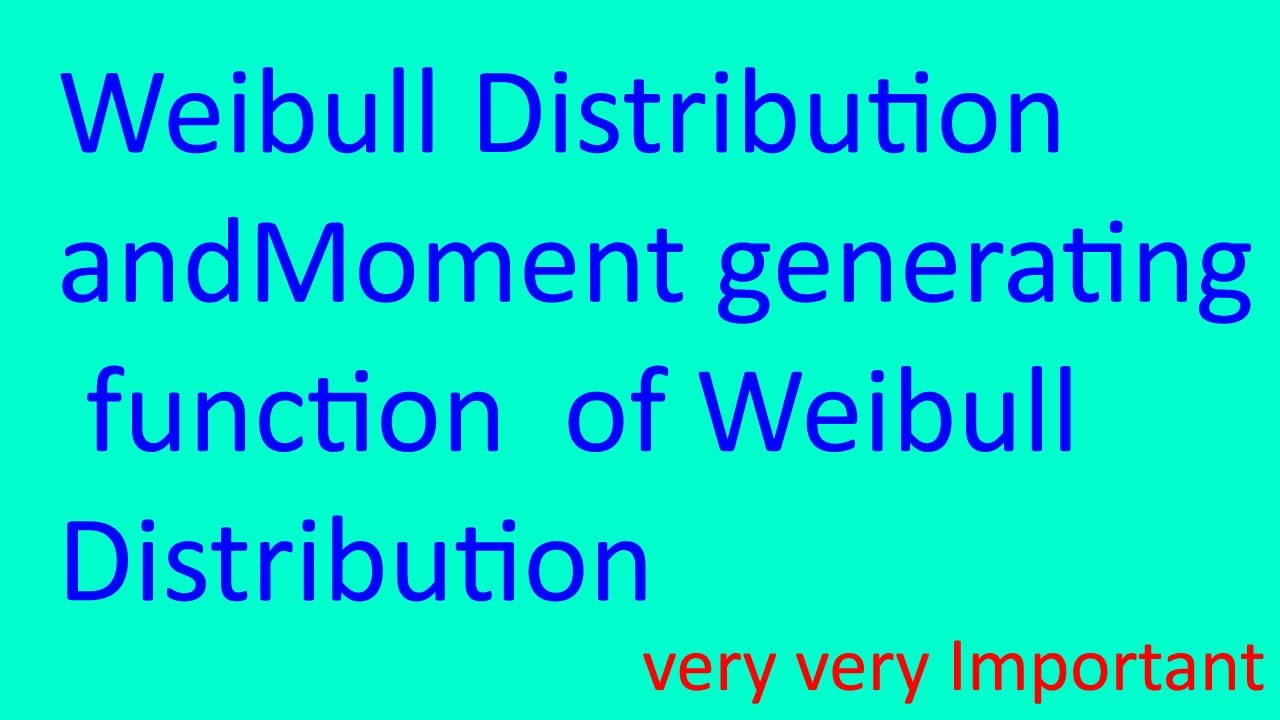
Weibull Distribution and Moment generating function (mgf) of Weibull Distribution in statistics|
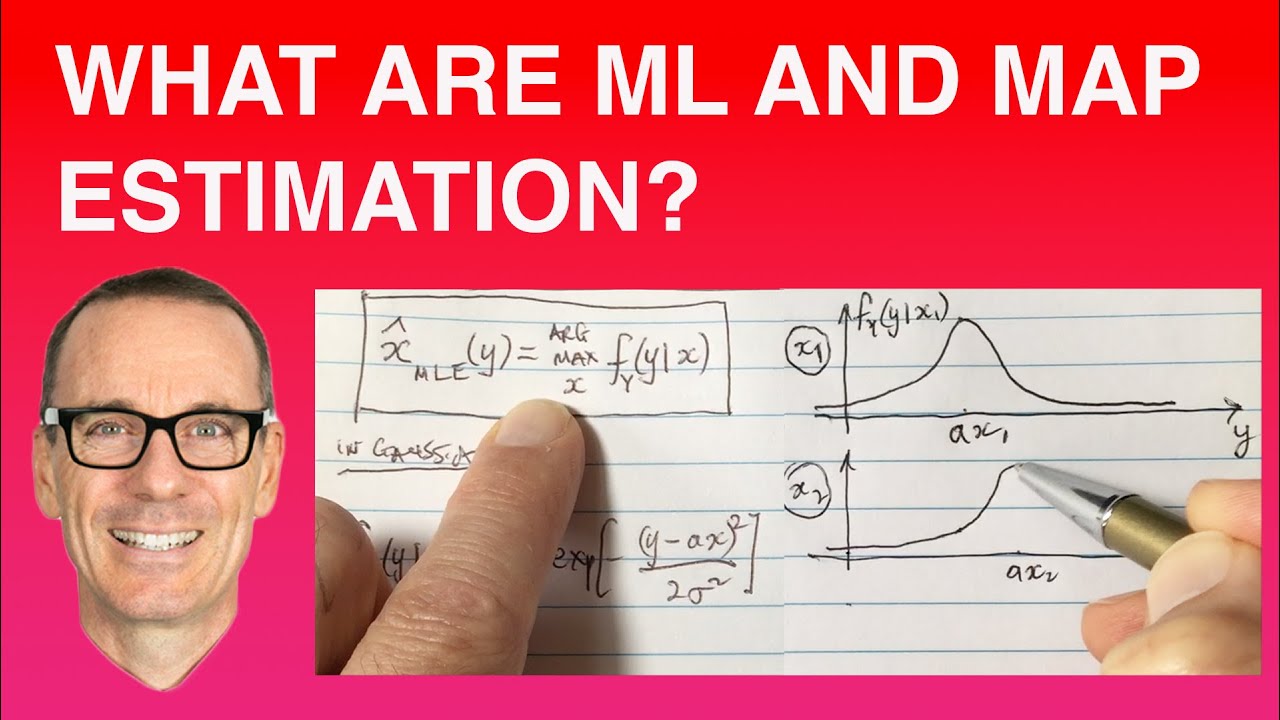
What are Maximum Likelihood (ML) and Maximum a posteriori (MAP)? ("Best explanation on YouTube")

FM2 2 1b 2 Discrete Rand Vars
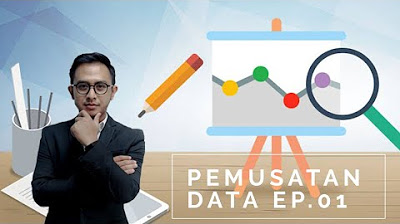
Kuliah Statistika Terapan - Pemusatan Data Sesi 1 Ep.04
5.0 / 5 (0 votes)