Principal Component Analysis (PCA) : Mathematical Derivation
Summary
TLDRThis video explores Principal Component Analysis (PCA), a key technique in machine learning for dimensionality reduction using eigenvalues and eigenvectors. It outlines the mathematical foundation of PCA, focusing on projecting high-dimensional data into a lower-dimensional space while maximizing variance. The speaker explains how to compute the covariance matrix and discusses the significance of eigenvectors and eigenvalues in capturing data spread. Additionally, the video addresses computational challenges when features outnumber data points and introduces alternative methods for efficient processing. Viewers are encouraged to implement PCA in Python for deeper understanding, making this a practical and insightful guide.
Takeaways
- 😀 Understanding the importance of a strong personal brand in today's competitive job market.
- 😀 Emphasizing the role of storytelling in conveying one's experiences and values.
- 😀 Highlighting the significance of networking and building relationships for career advancement.
- 😀 Discussing the impact of social media on personal branding and professional opportunities.
- 😀 Encouraging the continuous development of skills to stay relevant in the industry.
- 😀 Stressing the need for authenticity in presenting oneself to potential employers.
- 😀 Offering strategies for effectively communicating one's unique value proposition.
- 😀 Advising on how to create a cohesive online presence across various platforms.
- 😀 Addressing the benefits of mentorship and seeking guidance from industry leaders.
- 😀 Reinforcing the idea that personal branding is an ongoing process that evolves with one's career.
Please replace the link and try again.
Outlines
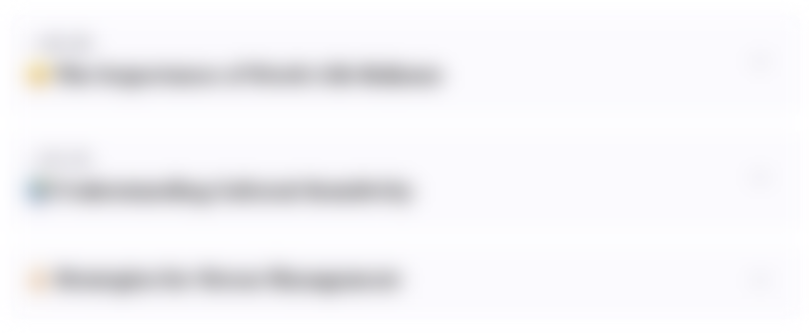
This section is available to paid users only. Please upgrade to access this part.
Upgrade NowMindmap
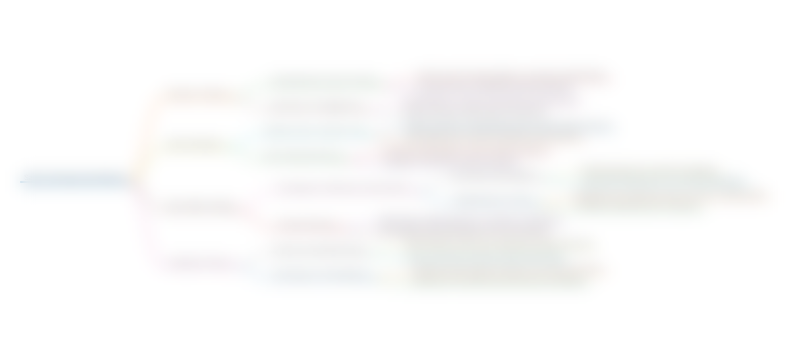
This section is available to paid users only. Please upgrade to access this part.
Upgrade NowKeywords
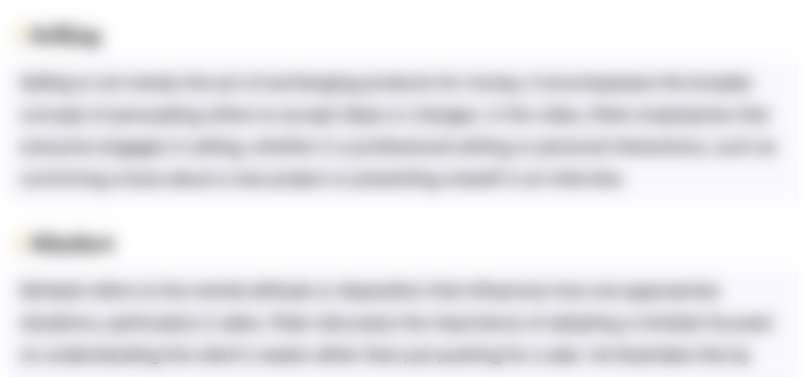
This section is available to paid users only. Please upgrade to access this part.
Upgrade NowHighlights
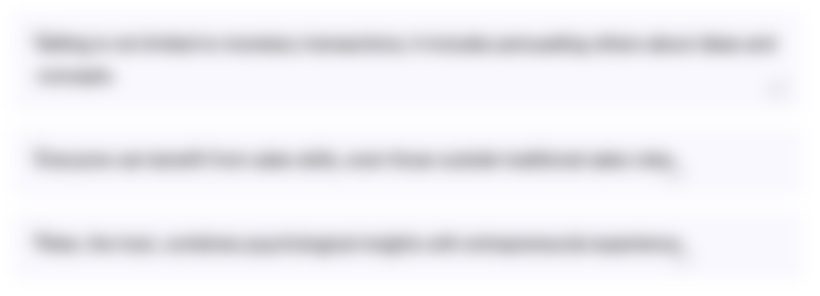
This section is available to paid users only. Please upgrade to access this part.
Upgrade NowTranscripts
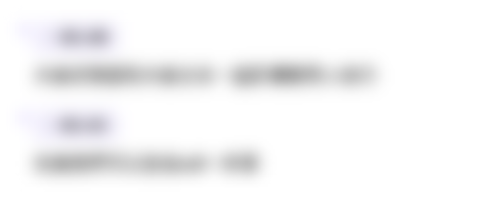
This section is available to paid users only. Please upgrade to access this part.
Upgrade NowBrowse More Related Video
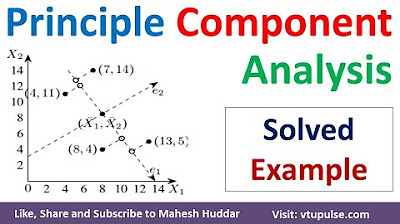
1 Principal Component Analysis | PCA | Dimensionality Reduction in Machine Learning by Mahesh Huddar
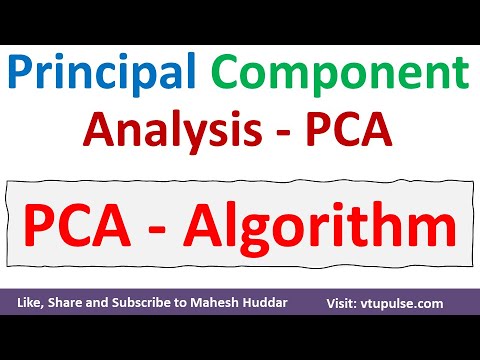
PCA Algorithm | Principal Component Analysis Algorithm | PCA in Machine Learning by Mahesh Huddar
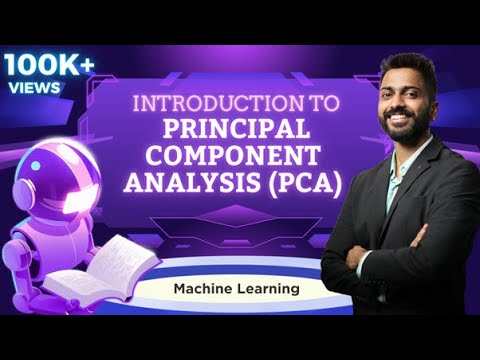
Lec-46: Principal Component Analysis (PCA) Explained | Machine Learning

Linear Algebra - Distance,Hyperplanes and Halfspaces,Eigenvalues,Eigenvectors ( Continued 3 )

Python Exercise on kNN and PCA
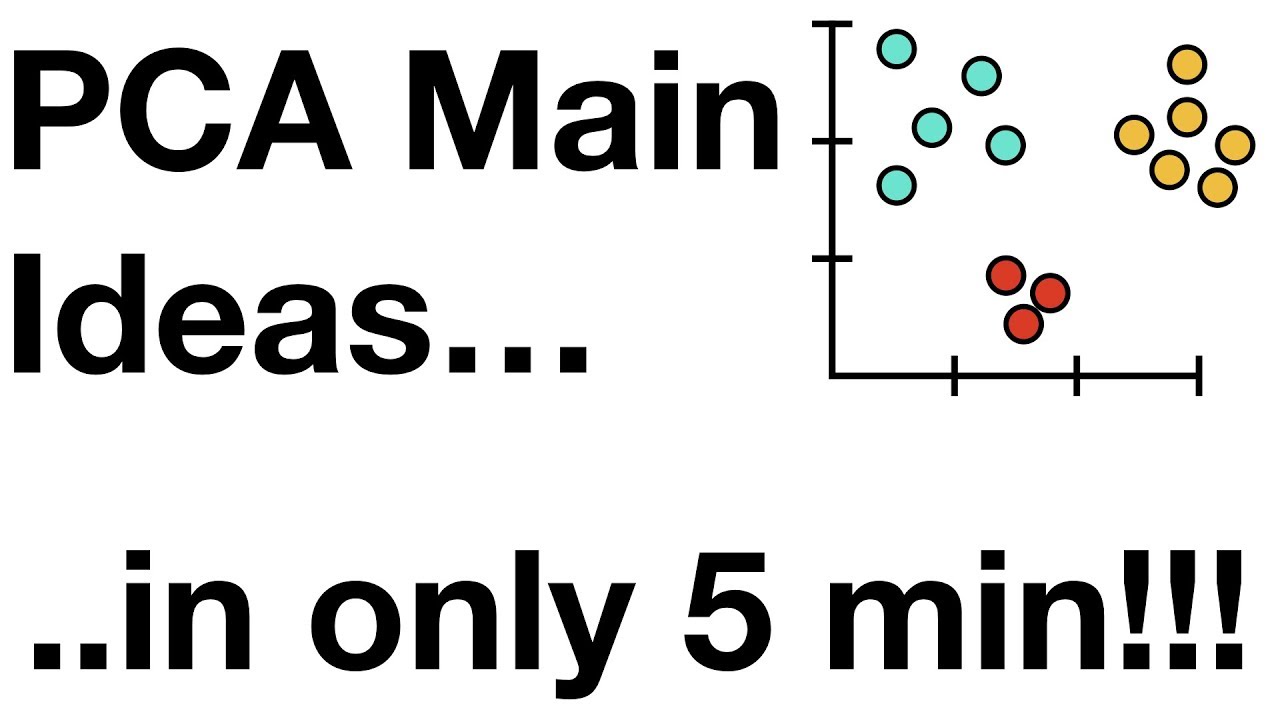
StatQuest: PCA main ideas in only 5 minutes!!!
5.0 / 5 (0 votes)