Shane Legg (DeepMind Founder) - 2028 AGI, Superhuman Alignment, New Architectures
Summary
TLDRShane Legg,Google DeepMind的创始人兼首席AGI科学家,在播客中探讨了通用人工智能(AGI)的进展衡量、当前大型语言模型的不足以及未来的发展路径。他强调了人类认知的多样性和复杂性,以及实现AGI所需的多方面测试和理解。Legg还讨论了深度学习模型的样本效率问题,以及如何通过架构创新和对伦理的深入理解来实现对强大AI的安全对齐。此外,他还预测了多模态AI将成为该领域的下一个重要里程碑。
Takeaways
- 🤖 AGI(人工通用智能)的定义是能够执行人类通常能做的各种认知任务的机器。
- 📈 衡量AGI进展需要多种不同类型的测试和测量,涵盖人类认知任务的广泛范围。
- 🧠 当前的AI模型,如大型语言模型,缺乏类似人类的情景记忆(episodic memory)的能力。
- 🔍 现有的基准测试无法充分衡量人类的某些认知方面,如理解视频流等。
- 🌐 未来的AI发展需要解决样本效率问题,即如何让AI在较少的数据上也能快速学习。
- 🎮 人类水平的AI到来的标志不是单一的测试,而是一系列综合测试和无法找到AI性能低于人类的任务。
- 🧭 早期研究强调AI应该能够在多种开放式环境中操作和成功,类似于视频游戏。
- 🔄 深度学习模型目前通过增加上下文窗口和训练基础模型来学习,但缺少中间过程。
- 🌟 未来的AI里程碑可能是更加全面的多模态理解,这将极大地扩展AI的应用范围。
- 🚀 目前的AI安全研究包括可解释性、过程监督、红队测试、评估危险能力和机构治理等。
Q & A
如何具体衡量我们朝着人工通用智能(AGI)的进展?
-衡量AGI的进展需要多种不同类型的测试和测量,这些测试应该涵盖人类可以执行的各种认知任务的广度。我们需要了解人类在这些任务上的表现,然后判断AI系统是否达到了人类的水平。如果AI系统能够在广泛的任务上达到人类的表现,并且很难找到它表现不佳的任务,那么我们可以认为已经实现了AGI。
目前的基准测试有哪些局限性?
-目前的基准测试,如MMLU等,主要针对语言模型,因此它们不能衡量人类理解视频流等非语言任务的能力。此外,它们也不测试人类的记忆系统,如情景记忆,这是人类快速学习特定信息的能力。
样本效率与人类的记忆系统有何关联?
-样本效率与人类的记忆系统密切相关,因为它是人类能够快速学习新事物的能力之一。大型语言模型在一定程度上具有样本效率,因为它们可以在上下文窗口中快速学习新信息。然而,现有的系统缺乏人类快速学习特定信息的能力,即情景记忆。
深度学习模型的样本效率是否是一个根本性缺陷?
-样本效率不是深度学习模型的根本性缺陷。虽然这些模型需要比人类一生中能看到的更多数量级的数据,但我们可以预期,随着研究的深入,将有清晰的路径来解决现有模型的这些不足,包括它们的记忆和学习类型。
如何具体判断人工智能达到了人类水平?
-无法通过单一的测试或任务来判断人工智能达到了人类水平,因为这是一个关于通用智能的问题。我们需要确保AI能够在大量不同的任务上达到人类水平,并且没有明显的能力差距。此外,当人们尝试找到机器失败的任务示例时,如果他们无法成功,那么我们就可以认为AI达到了人类水平。
Shane Legg对于人工智能在开放环境中操作和成功的看法有何变化?
-Shane Legg的早期研究强调了人工智能应该能够在各种开放环境中操作和成功。随着时间的推移,他的观点有所发展,从尝试定义一个完全通用、数学上清晰的智能概念,转变为更具体地考虑人类智能和构建能够匹配人类智能的机器,因为我们理解人类智能是一个非常强大的东西。
Shane Legg如何看待AlphaFold等特定领域的模型与AGI的关系?
-像AlphaFold这样的特定领域模型并不是直接推动AGI发展的,它们可能在这个过程中学到了一些可能相关的东西,但Shane Legg并不认为它们是通往AGI的道路。不过,DeepMind作为一个大团队,会探索各种不同的项目,包括AlphaFold这样的重大项目。
Shane Legg对于未来的人工智能模型有什么预测?
-Shane Legg预测,未来的人工智能模型将会更加多模态,这将打开更广阔的可能性空间。他认为,未来的人们回顾过去的模型时,会认为它们只处理文本聊天是非常狭窄的。未来的模型将能够理解图像、视频,并能处理更多种类的数据,这将使系统以更强大的方式与世界建立联系。
Shane Legg如何看待当前的人工智能安全研究?
-Shane Legg认为,为了实现真正符合伦理的AI系统,我们需要构建具有深入理解世界、良好伦理观念和稳健推理能力的系统。他强调,我们需要训练系统理解伦理,并且需要社会和伦理学家来确定系统应该遵循的伦理原则。此外,我们需要确保系统在每次做出决策时都进行伦理分析,并且人类专家需要不断检查这些决策和推理过程。
Shane Legg对于如何确保AI遵循我们设定的伦理原则有何看法?
-Shane Legg认为,我们需要向系统明确指出应该遵循的伦理原则,并且我们需要检查系统在实际操作中是否一致地遵循这些原则。他提出,通过深入理解世界和伦理,以及稳健的推理,系统应该能够在面对不同选项时进行伦理分析,并执行最符合伦理的行动。
Shane Legg如何看待当前多模态AI模型的发展?
-Shane Legg认为,目前的多模态AI模型还处于早期阶段,但他预期未来人们将越来越多地理解图像和视频等内容。他认为,当系统能够很好地处理视频和其他类型的数据时,它们将对世界有更深入的理解,这将自然地打开许多新的应用和可能性。
Outlines
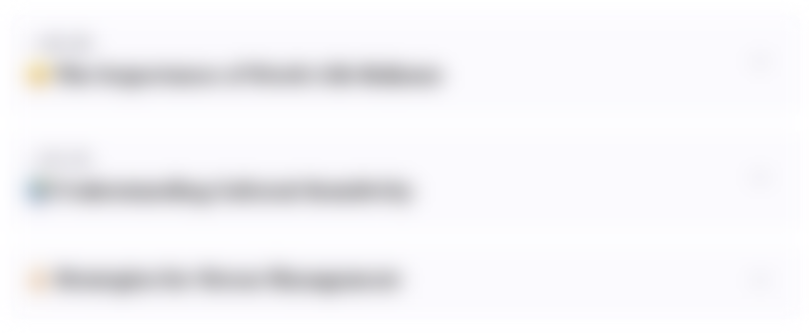
This section is available to paid users only. Please upgrade to access this part.
Upgrade NowMindmap
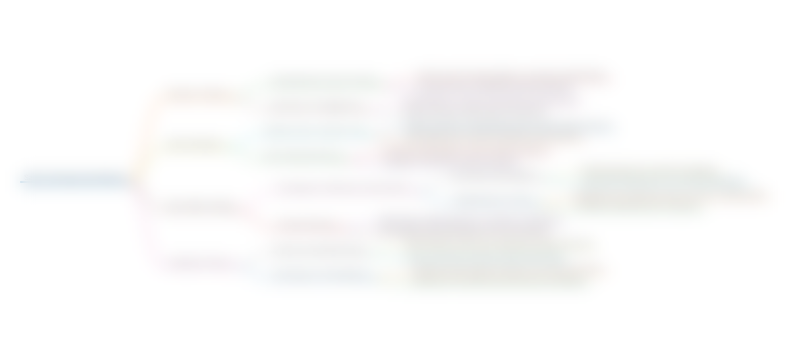
This section is available to paid users only. Please upgrade to access this part.
Upgrade NowKeywords
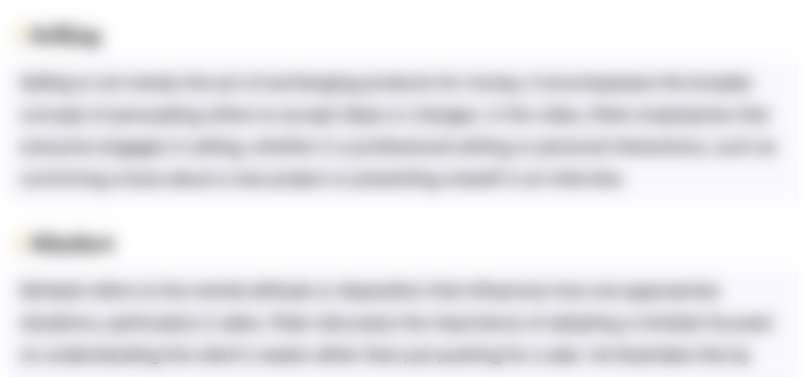
This section is available to paid users only. Please upgrade to access this part.
Upgrade NowHighlights
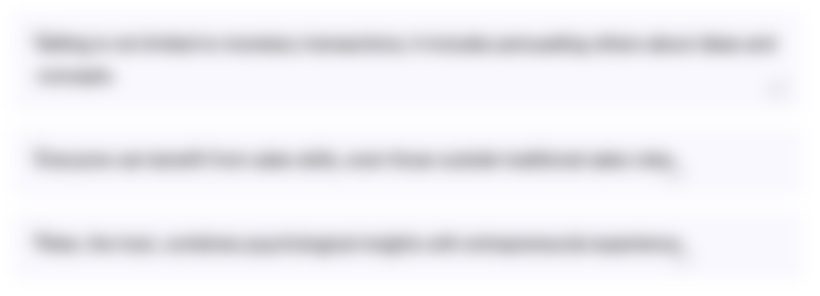
This section is available to paid users only. Please upgrade to access this part.
Upgrade NowTranscripts
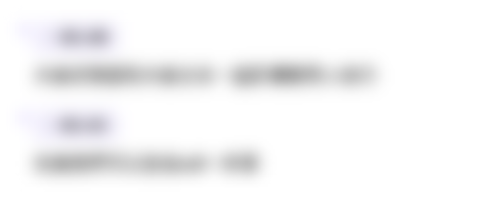
This section is available to paid users only. Please upgrade to access this part.
Upgrade NowBrowse More Related Video
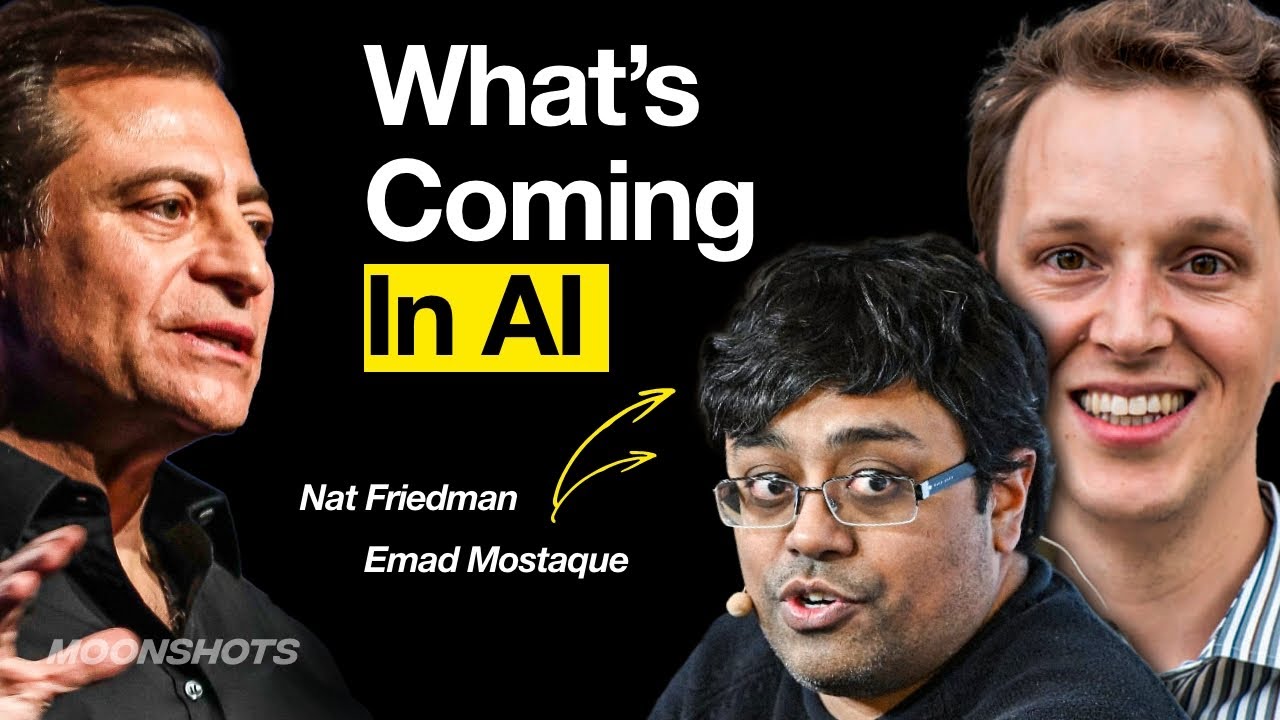
2 Ex-AI CEOs Debate the Future of AI w/ Emad Mostaque & Nat Friedman | EP #98
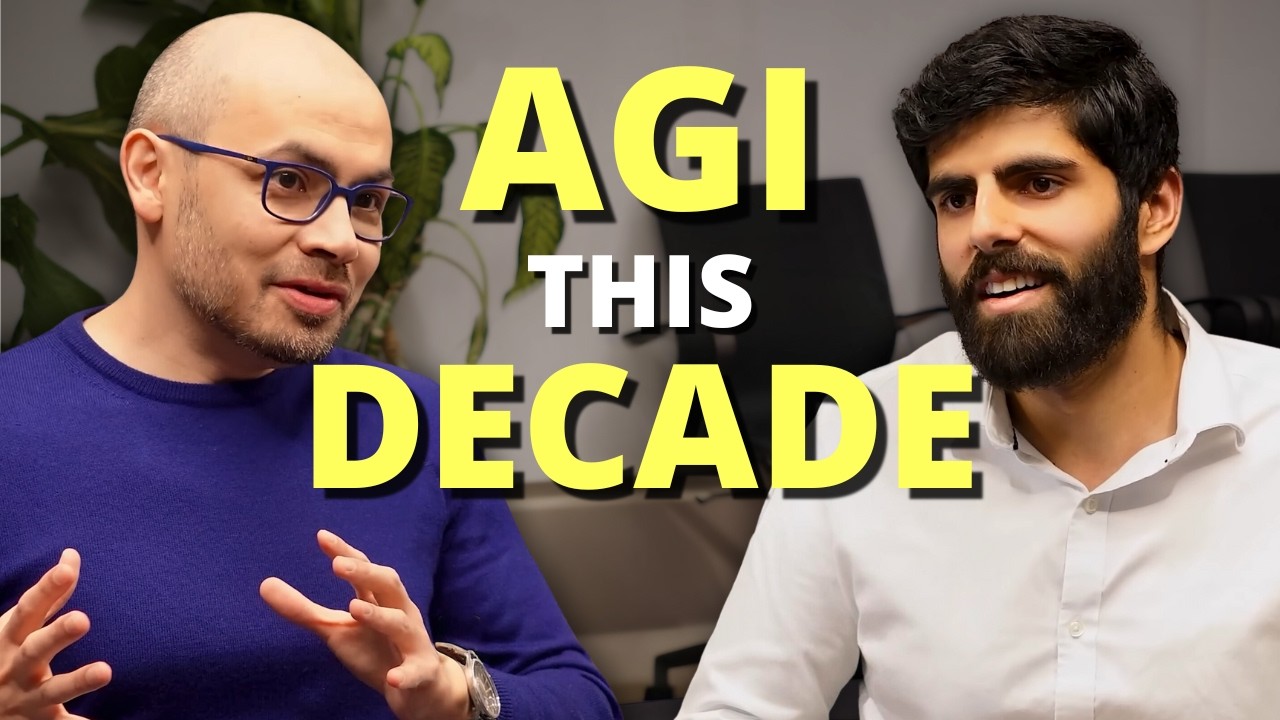
Demis Hassabis - Scaling, Superhuman AIs, AlphaZero atop LLMs, Rogue Nations Threat
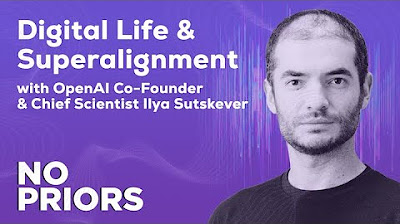
No Priors Ep. 39 | With OpenAI Co-Founder & Chief Scientist Ilya Sutskever
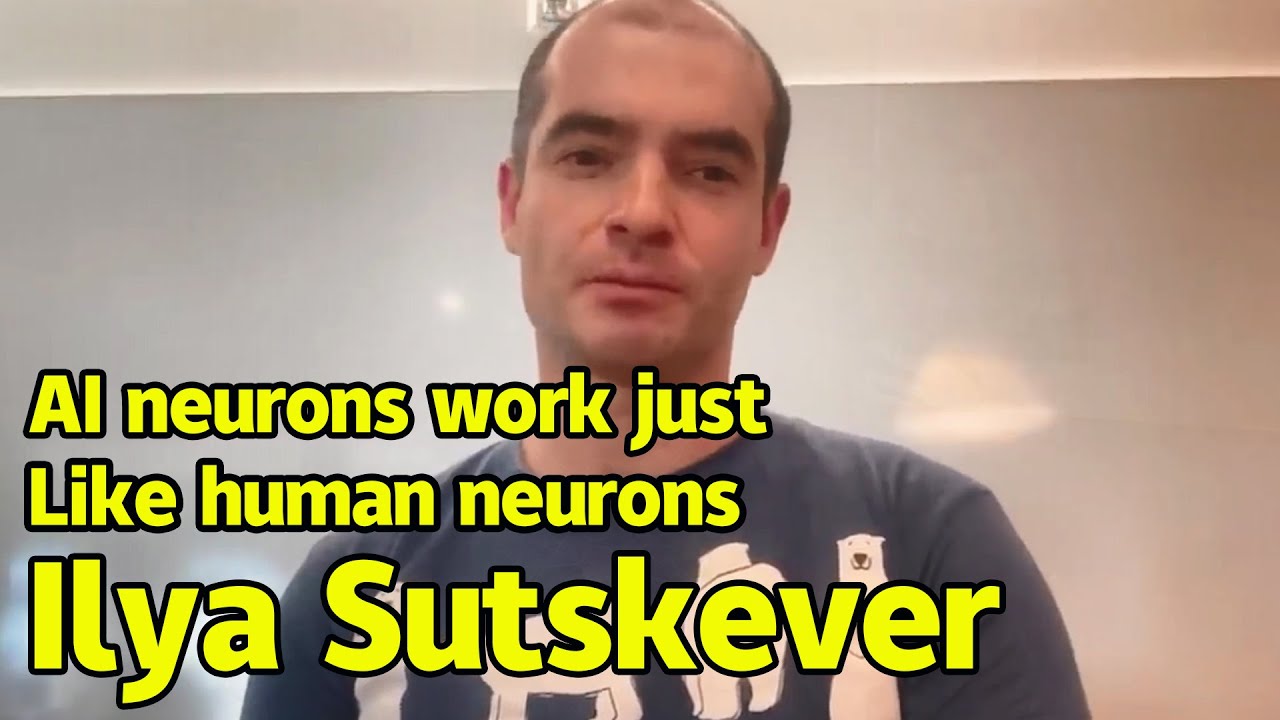
Ilya Sutskever | AI neurons work just like human neurons | AGI will be conscious like humans
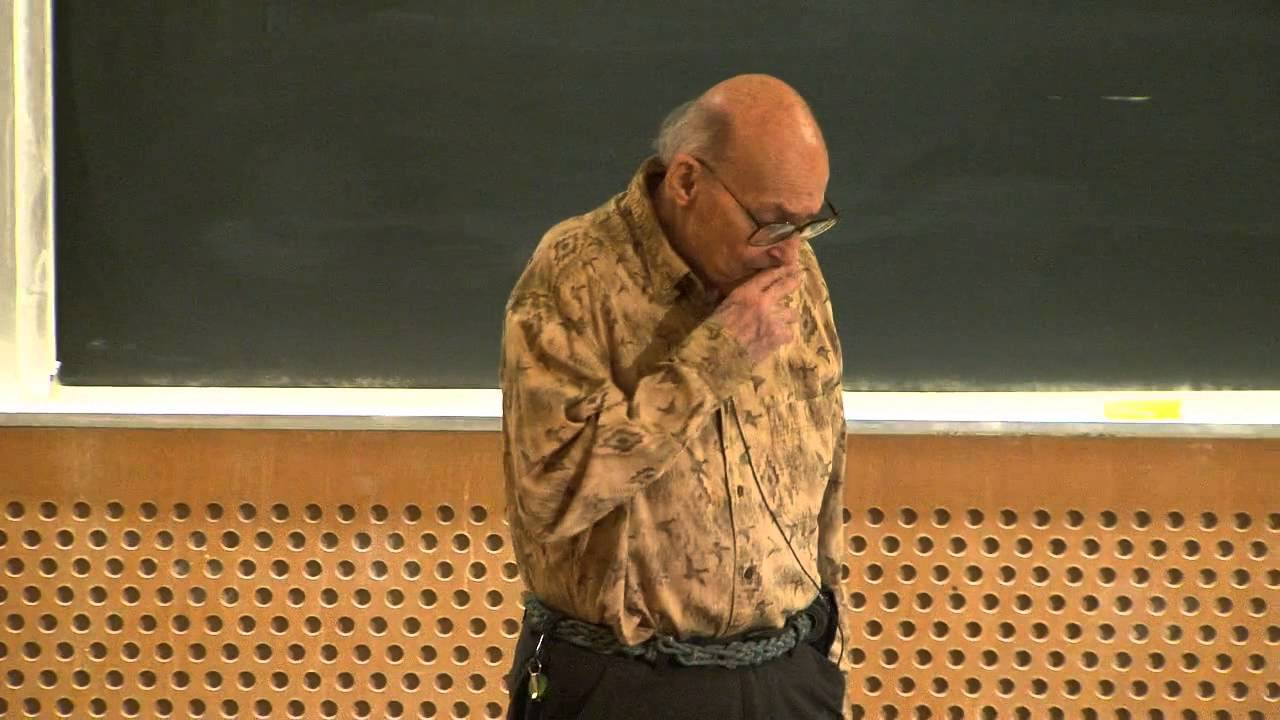
6. Layers of Mental Activities
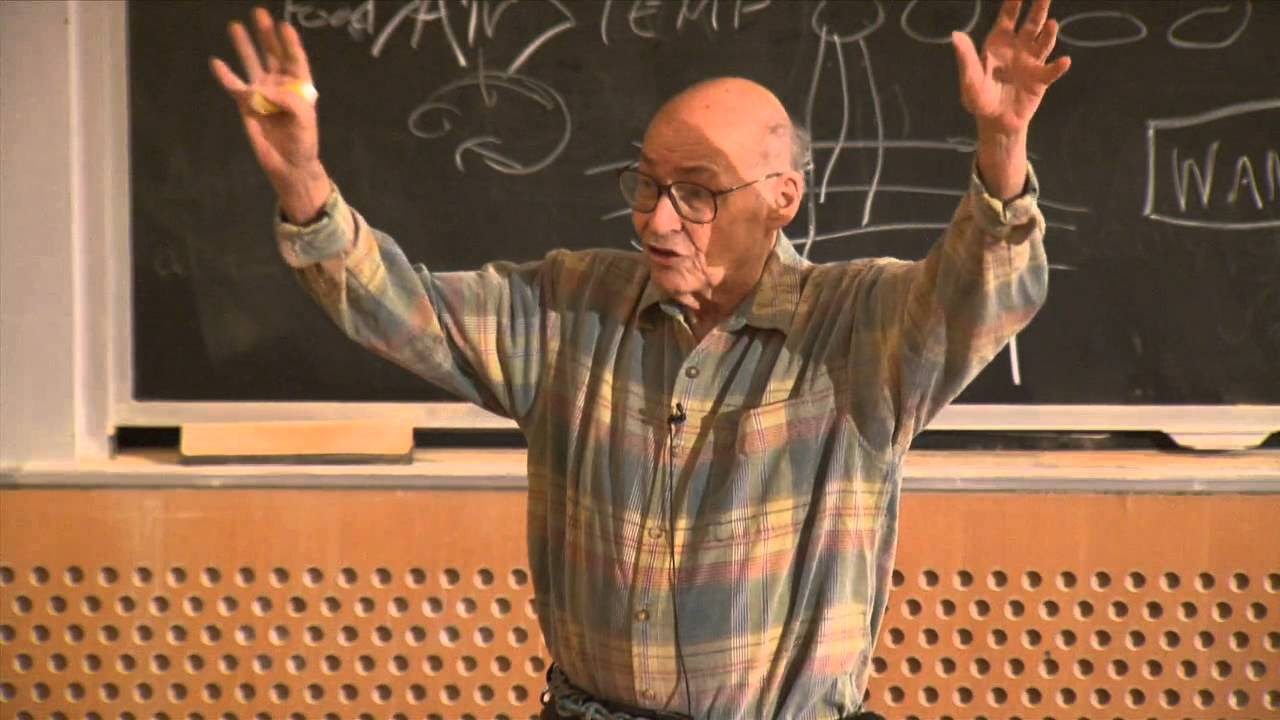
7. Layered Knowledge Representations
5.0 / 5 (0 votes)