AI/ML Fundamentals for Product Managers
Summary
TLDR本次演讲由Darris Kumari主讲,讨论了产品经理学习人工智能和机器学习的重要性,以及如何在现有产品和组织中实现AI。演讲涵盖了AI的基本能力、如何作为产品经理理解AI的内部工作原理,并通过实例讲解了监督学习、无监督学习和神经网络在AI领域的作用。强调了AI在提高用户体验、产品差异化和推动收入增长方面的关键作用,同时探讨了实施AI技术的战略方法和潜在风险。
Takeaways
- 🚀 产品管理者应学习AI和ML,以保持竞争力并最大化客户和用户体验。
- 💡 创新的七个关键力量包括流程需求、行业变化、市场变化、新知识、潜在的不一致性和用户期望。
- 🌟 AI和ML可以提供额外的价值,帮助组织获得溢价收入和产品差异化。
- 🛠️ 实施AI技术时,组织可以选择自建或与云供应商合作。
- 🔄 对于AI和ML的采纳,组织结构上可能需要一个集中的平台团队来支持其他产品团队。
- 📈 产品管理者应从用户痛点和旅程出发,思考AI和ML在产品中的应用。
- 🥄 开始AI和ML项目时,建议采用分步方法,包括创意、概念验证和产品化。
- 🔒 在实施AI和ML时,需要考虑法律、数据所有权和用户数据安全等问题。
- 📊 AI和ML的成功需要业务的大力支持,包括领导层的支持和资源分配。
- 🎯 产品管理者应关注AI和ML技术的最新发展,以便在产品中实施创新用例。
Q & A
为什么产品经理需要学习人工智能和机器学习?
-产品经理学习人工智能和机器学习可以帮助他们掌握新技术,最大化客户和用户体验,提供差异化和有吸引力的AI驱动功能,从而使组织保持竞争力,避免被更敏捷的初创公司颠覆,并推动最大的收入回流到组织。
如何将AI集成到现有产品和组织中?
-可以通过与云服务提供商合作,利用他们的API和AI能力来增强自己的产品功能。对于大型组织,可以利用已有的AI技术平台和产品团队。对于早期AI应用,建议从云服务提供商开始,而不是从头构建模型。
人工智能和机器学习的关键能力包括哪些?
-关键能力包括回归、分类、神经网络、强化学习、自然语言处理和计算机视觉等。这些能力可以用于预测分析、用户旅程中的决策支持、文本和图像生成、语音识别和内容提取等。
如何开始AI和ML的创新之旅?
-作为产品经理,可以从识别用户痛点和旅程中的AI应用开始,然后通过概念验证和用户验证来测试和验证想法。最后,将这些功能产品化并推向市场。
在实施AI技术时,产品经理可能会遇到哪些法律风险?
-可能的法律风险包括数据所有权问题、客户数据训练的合法性、第三方云AI服务提供商的数据安全和隐私问题,以及确保遵守相关的数据保护法规。
如何确保AI模型的训练数据质量?
-需要对训练数据进行适当的预处理,去除个人身份信息(PII),确保数据安全,避免使用有偏见或非法获取的数据集,并确保法律要求得到满足。
产品经理如何识别AI在其产品中的应用机会?
-产品经理应该了解自己的产品领域、用户以及他们试图解决的用例。然后,可以根据这些信息思考AI和ML在现有产品和业务流程中可能发挥作用的地方。
什么是神经网络,它如何工作?
-神经网络是一种模仿人类大脑神经元功能的机器学习模型,由多层节点(神经元)组成,通过权重和激活函数处理输入数据,进行决策和分类。
生成性AI技术有哪些应用?
-生成性AI技术可以用于文本生成、图像生成、音乐创作等领域。它们通过预测下一个最高概率的单词或图像来生成新的内容。
产品经理如何使用AI技术提高自己的工作效率?
-产品经理可以使用AI技术来生成产品文档的大纲、测试用例列表、研究问题、路线图项目和功能等。此外,AI还可以用于生成演示数据和进行市场分析。
Outlines
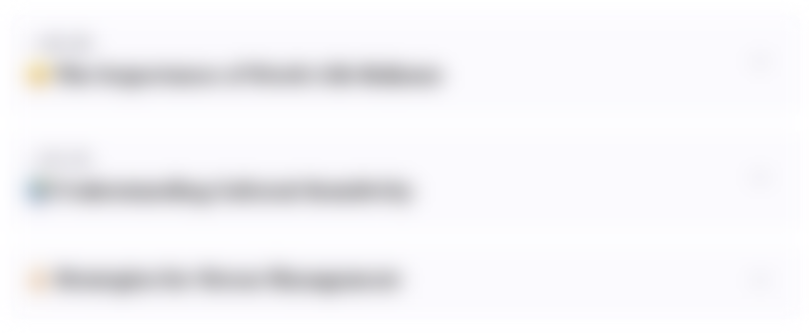
This section is available to paid users only. Please upgrade to access this part.
Upgrade NowMindmap
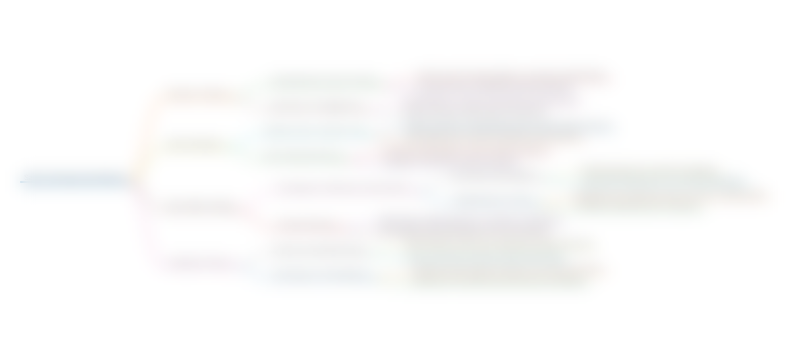
This section is available to paid users only. Please upgrade to access this part.
Upgrade NowKeywords
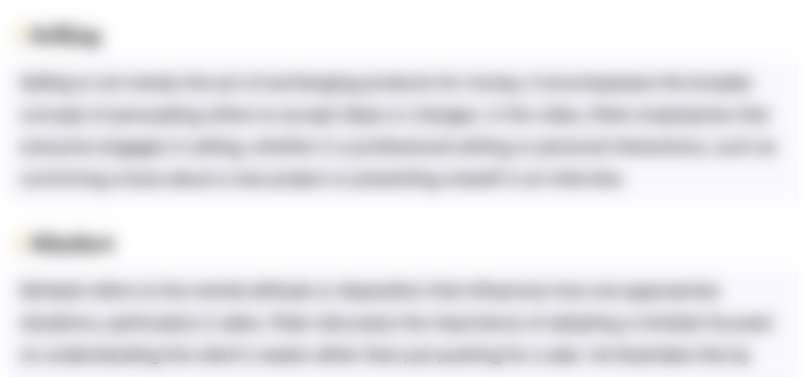
This section is available to paid users only. Please upgrade to access this part.
Upgrade NowHighlights
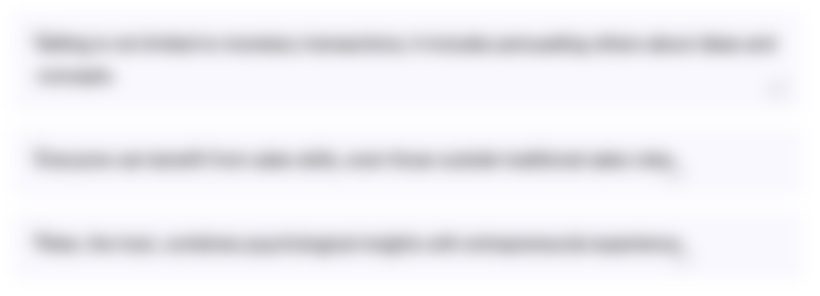
This section is available to paid users only. Please upgrade to access this part.
Upgrade NowTranscripts
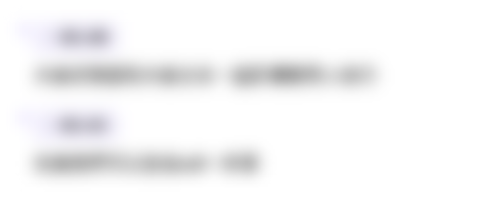
This section is available to paid users only. Please upgrade to access this part.
Upgrade NowBrowse More Related Video
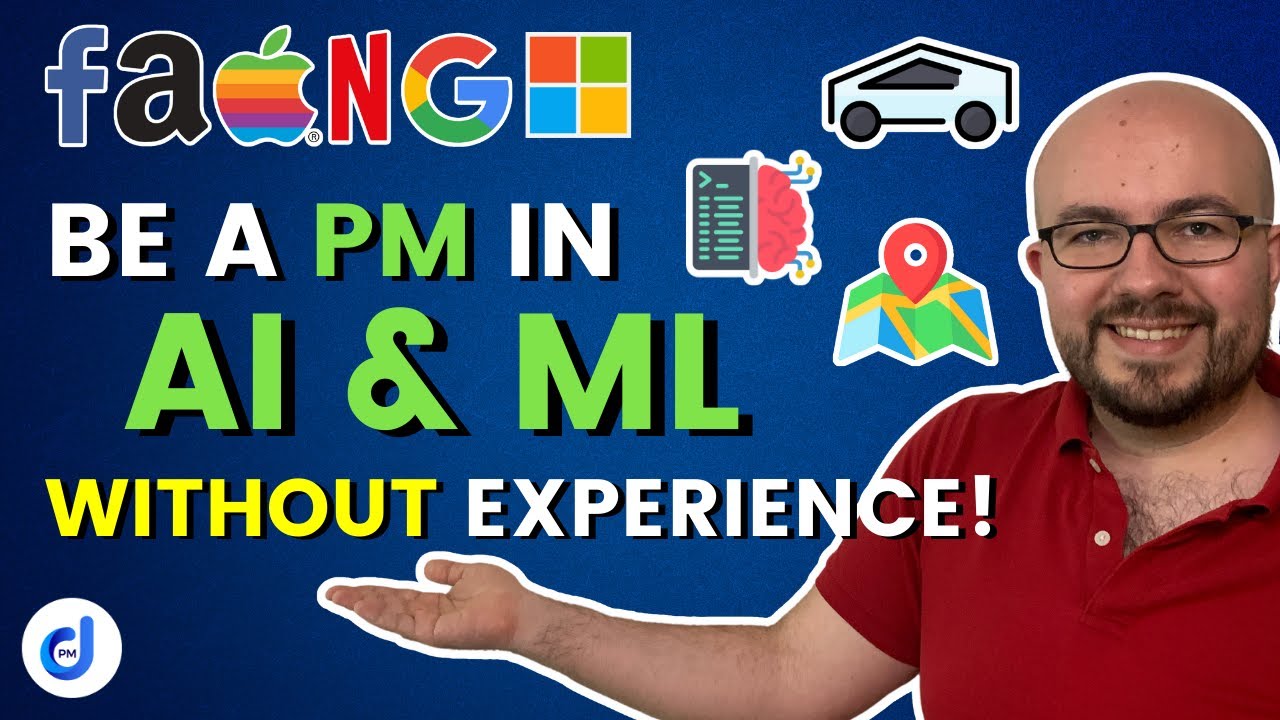
How to Break into AI Product Management without experience
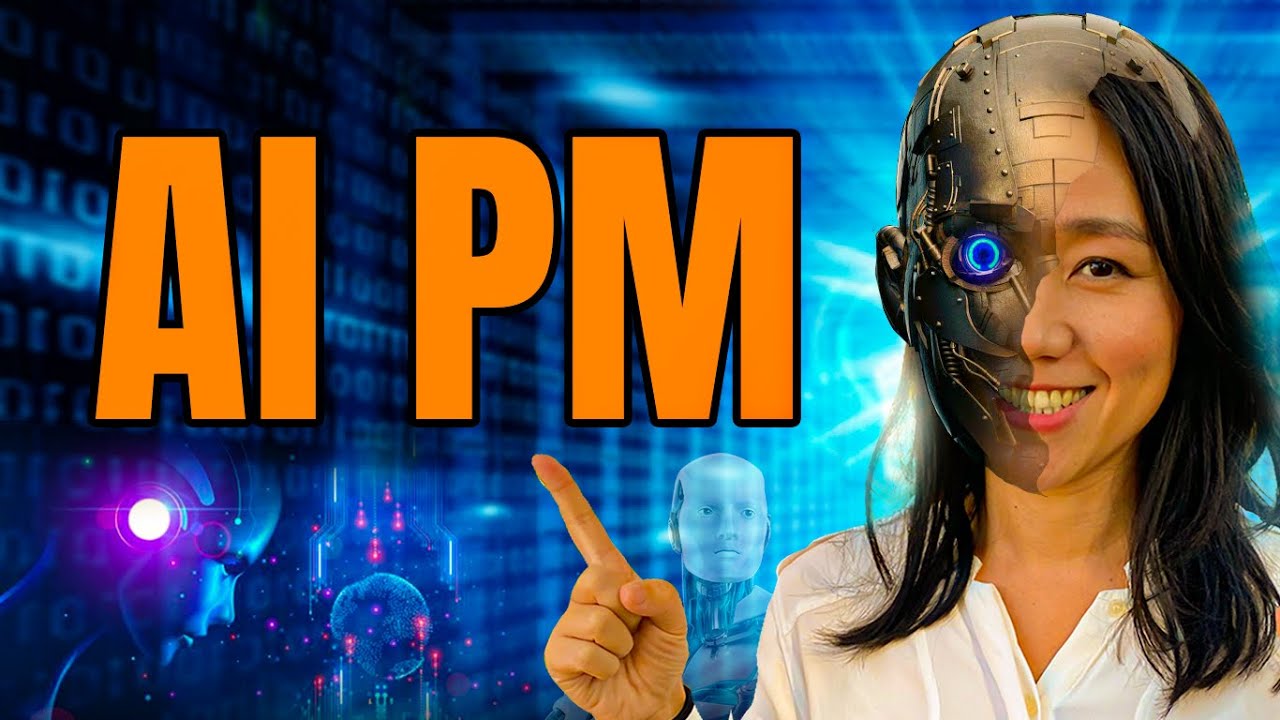
The ONLY 4 Ways to Become an AI Product Manager with No Experience
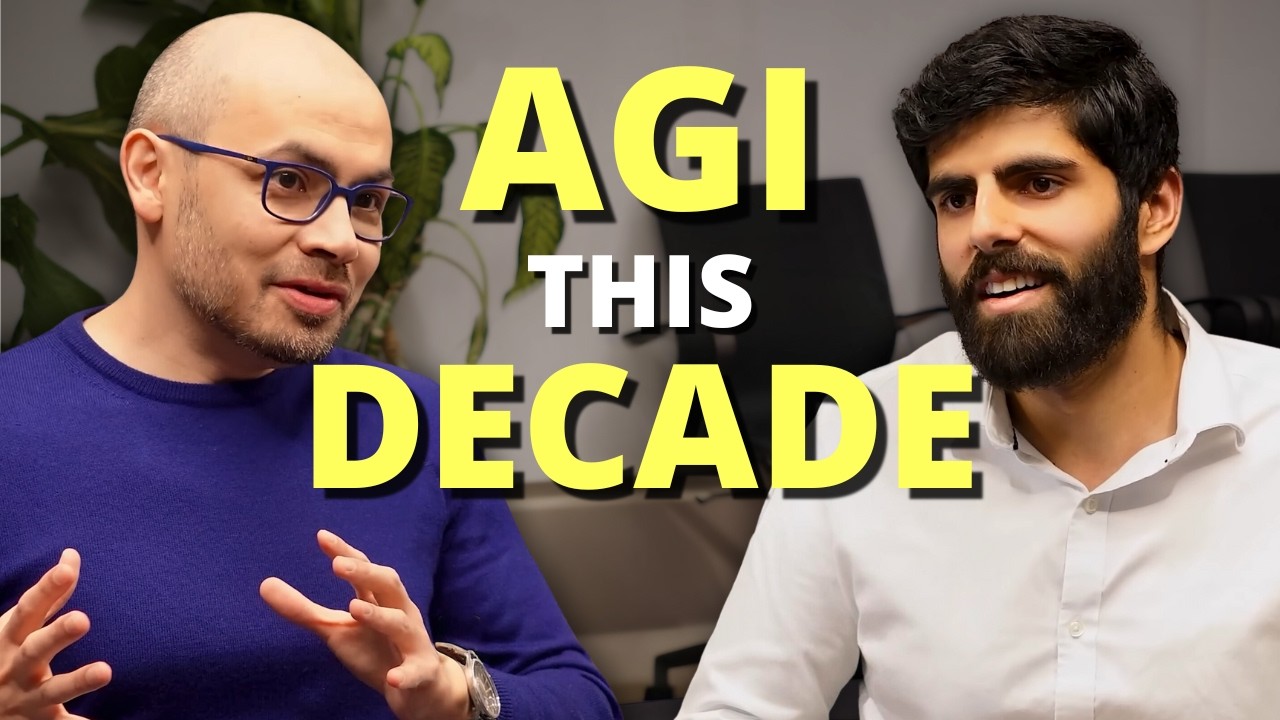
Demis Hassabis - Scaling, Superhuman AIs, AlphaZero atop LLMs, Rogue Nations Threat
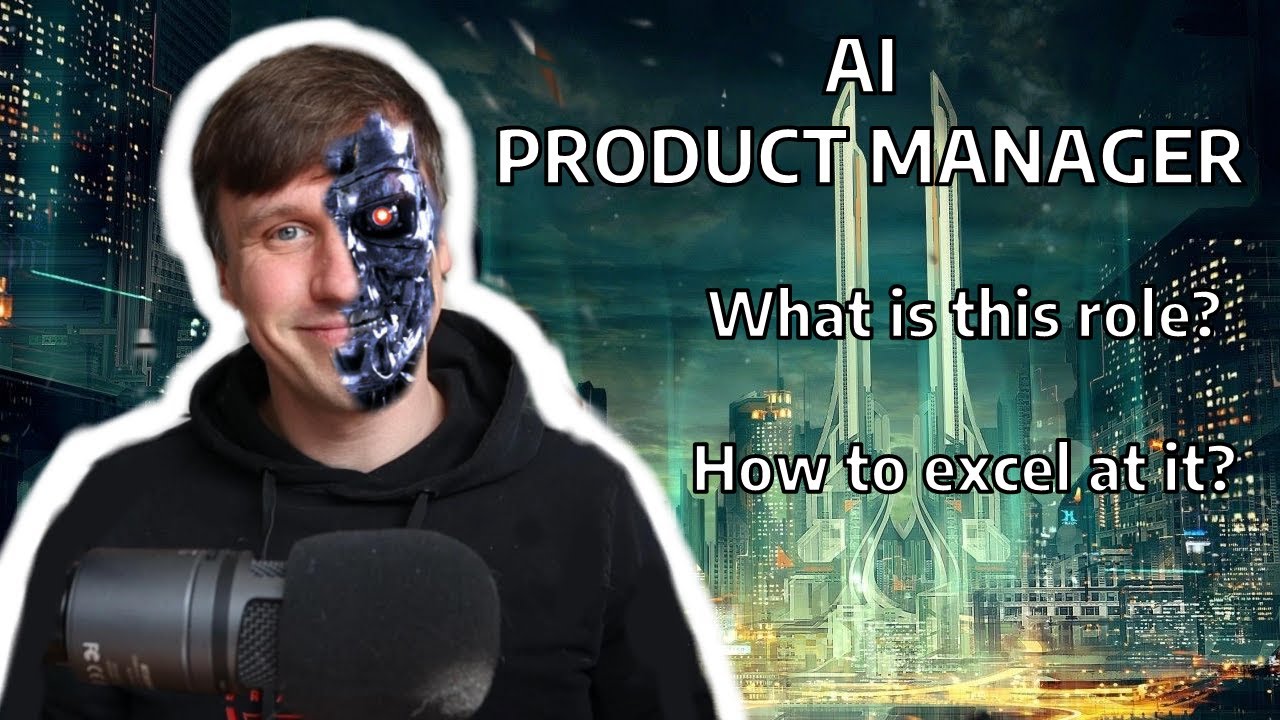
AI Product Manager
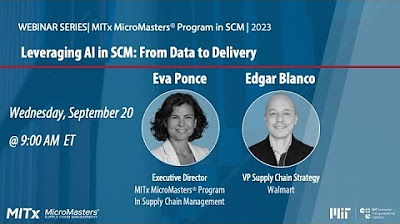
Leveraging AI in Supply Chain Management: From Data to Delivery
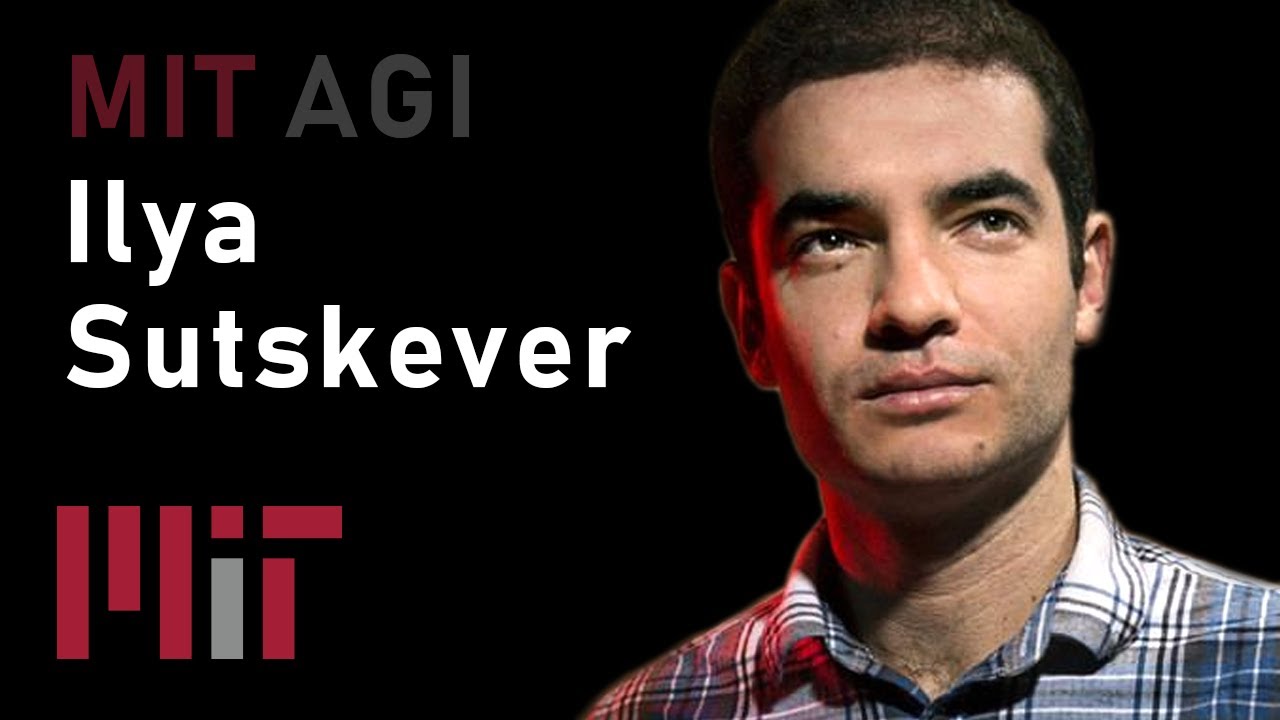
Ilya Sutskever: OpenAI Meta-Learning and Self-Play | MIT Artificial General Intelligence (AGI)
5.0 / 5 (0 votes)