How to Break into AI Product Management without experience
Summary
TLDR本视频由产品经理Diego主讲,探讨了在人工智能和机器学习领域成为产品经理的策略。Diego强调,尽管AI和机器学习产品的团队构成略有不同,但无需深厚的工程或计算机科学背景。他通过简单示例讲解了机器学习模型的创建过程,并建议通过Kaggle等平台学习和实践。Diego还提供了如何通过项目和网络建设进入这一领域的建议,鼓励观众了解AI产品经理的多样性和可能性。
Takeaways
- 🚀 作为AI和机器学习领域的产品经理,与一般产品经理的主要区别在于团队构成和工作重点。
- 🌟 AI和机器学习产品的团队中通常会加入数据科学家这一新角色,负责理解数据和构建、优化机器学习模型。
- 📊 产品经理不需要是工程师或拥有计算机科学学位,但需要了解不同技术概念和所涉及的技术栈。
- 🛠️ 即使没有技术背景,通过学习也能对应用程序的技术细节有所了解,这有助于产品管理工作。
- 🏠 通过简单示例,展示了如何使用机器学习模型预测房价,说明了模型创建的基本过程。
- 🔍 创建机器学习模型不仅仅是编写代码,还涉及到数据清洗、模型选择、结果解释和改进等多个步骤。
- 📈 评估机器学习模型时,需要考虑多种指标,而不仅仅是模型的绝对误差。
- 🔑 产品经理需要理解算法的创建过程和评估方法,以便更好地与团队沟通和规划产品特性。
- 🌐 AI和机器学习已广泛应用于各种应用程序中,如搜索引擎、智能家居、电子邮件过滤等。
- 📚 通过在线课程、参与数据科学竞赛和实际项目,即使没有机器学习背景的人也可以学习并进入AI产品管理领域。
- 💼 建立公共作品集和参与社区活动,可以提升个人在AI和机器学习产品管理领域的专业度和可信度。
Q & A
AI和机器学习领域产品经理与其他产品经理有何不同?
-AI和机器学习领域的产品经理在团队构成上有所不同,他们会与数据科学家合作,关注模型的构建、部署和整合。团队比例也会有所变化,每个产品经理可能会配5到10个数据科学家和2到5个工程师。
成为AI或机器学习产品经理需要哪些技能?
-虽然不需要计算机科学或数据科学背景,但需要理解算法的创建过程和评估方法,以便与团队进行有效沟通,评估权衡,规划产品特性,并为用户创造最佳体验。
产品经理需要了解哪些技术概念?
-产品经理需要了解与其产品相关的技术概念和科技栈,例如数据库、数据处理、跨平台编程工具、前后端组件等。
如何通过简单的代码创建一个机器学习模型?
-可以通过导入决策树回归器库,创建基于该算法的模型,使用模型特征训练模型,然后进行预测和结果验证。
机器学习模型的开发时间是否很短?
-尽管编码模型相对简单,但整个过程比想象中复杂,需要考虑数据质量、模型选择、性能评估等多个方面。
如何验证机器学习模型的结果?
-可以通过选择合适的评估指标,如平均绝对误差,来衡量模型的性能。
AI和机器学习在哪些产品中得到应用?
-AI和机器学习应用于多种产品,如搜索引擎、语音识别设备、电子邮件过滤、推荐系统和导航应用等。
如何进入AI和机器学习产品管理领域?
-可以通过学习如何将机器学习应用于产品,理解模型创建过程,并通过实际项目或参与数据科学竞赛来展示自己的能力。
Kaggle.com对于学习机器学习模型有何帮助?
-Kaggle提供了免费的机器学习模型编码课程,基础的Python编程语言教学,以及数据科学竞赛,有助于实践和建立公共作品集。
如何通过实际项目展示自己的机器学习能力?
-可以创建一个使用机器学习解决问题的产品或站点项目,记录整个过程,包括问题识别、用户交流、原型开发和产品发布等,并将其作为公共作品集的一部分。
如果我想更深入地学习AI和机器学习,有哪些途径?
-可以选择回到学校学习计算机科学或数据科学,专注于AI和机器学习领域,这可能需要投入时间和金钱,但也能开启职业发展的其他可能性。
Outlines
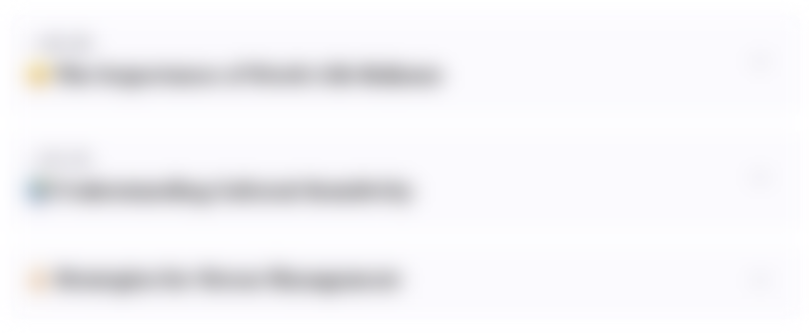
This section is available to paid users only. Please upgrade to access this part.
Upgrade NowMindmap
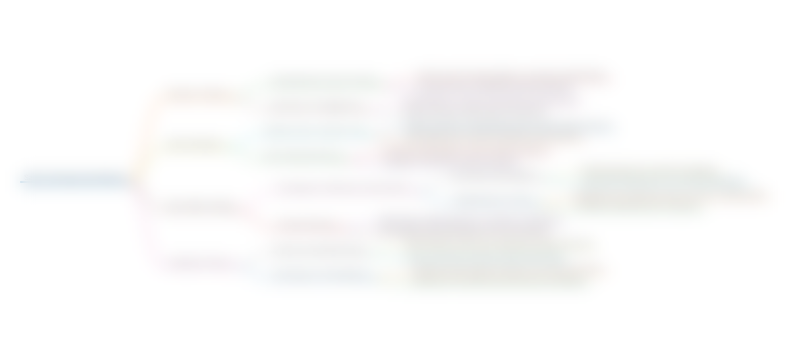
This section is available to paid users only. Please upgrade to access this part.
Upgrade NowKeywords
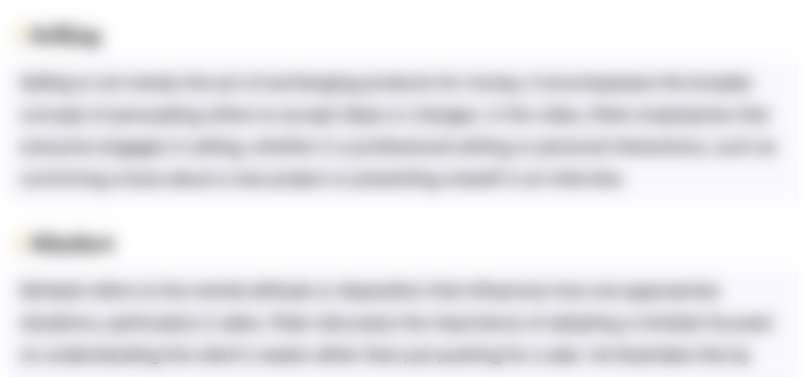
This section is available to paid users only. Please upgrade to access this part.
Upgrade NowHighlights
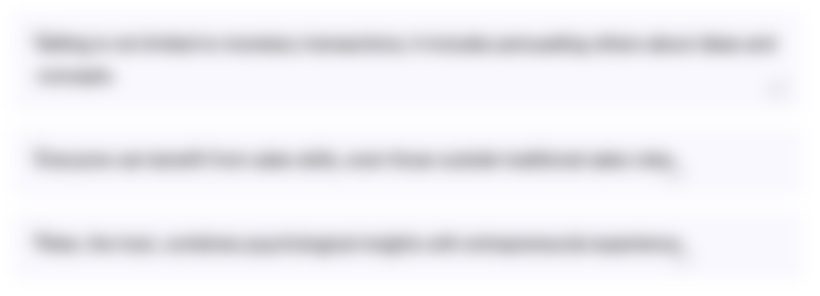
This section is available to paid users only. Please upgrade to access this part.
Upgrade NowTranscripts
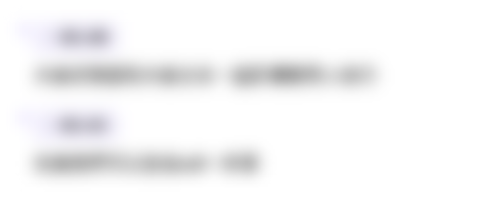
This section is available to paid users only. Please upgrade to access this part.
Upgrade NowBrowse More Related Video
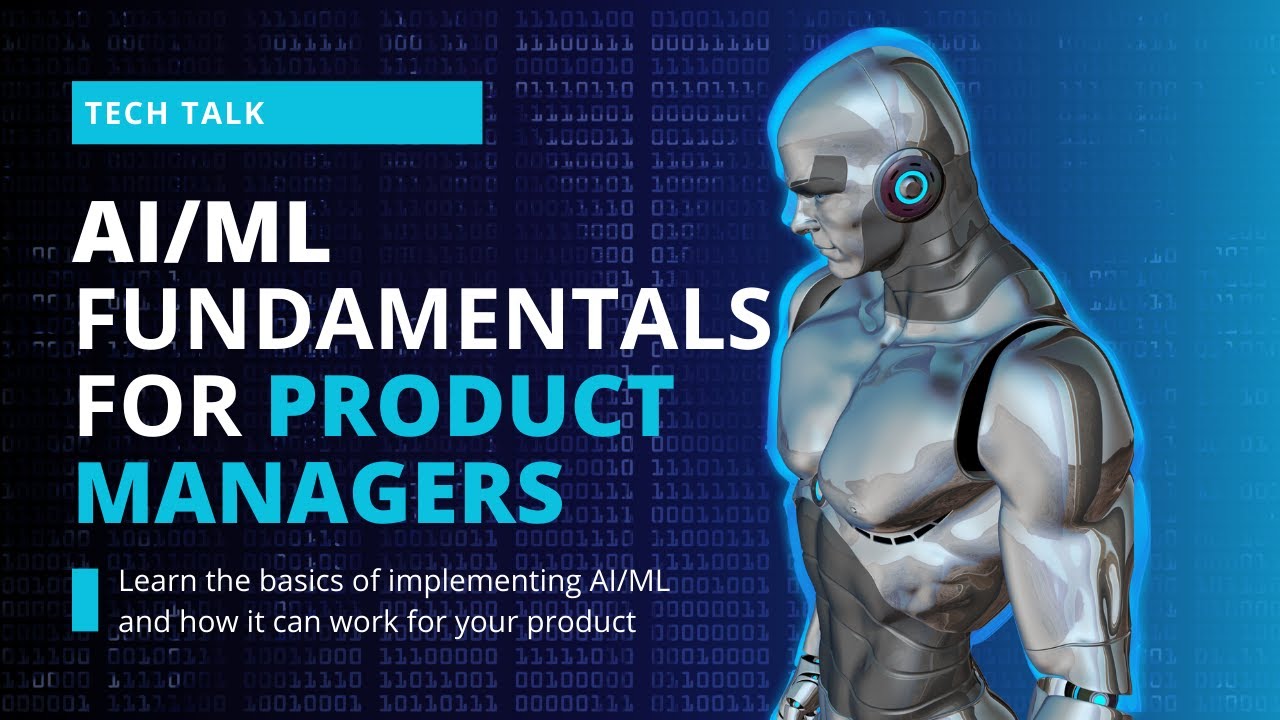
AI/ML Fundamentals for Product Managers
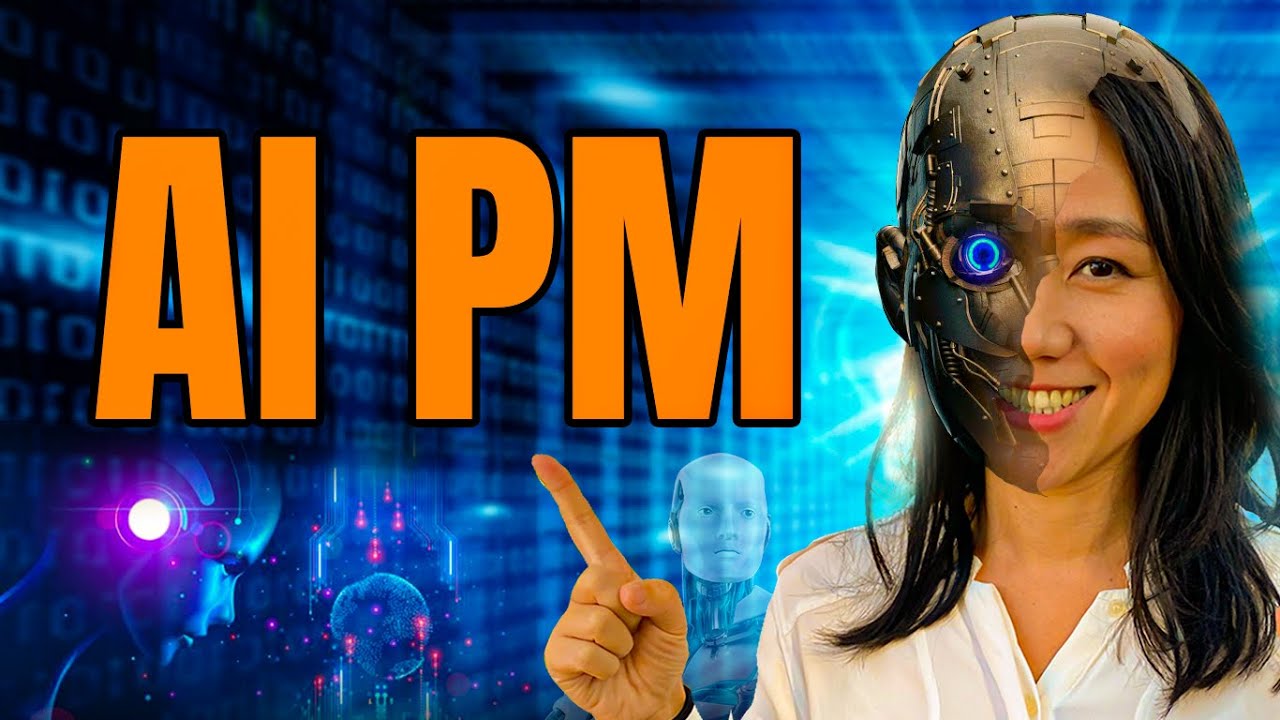
The ONLY 4 Ways to Become an AI Product Manager with No Experience
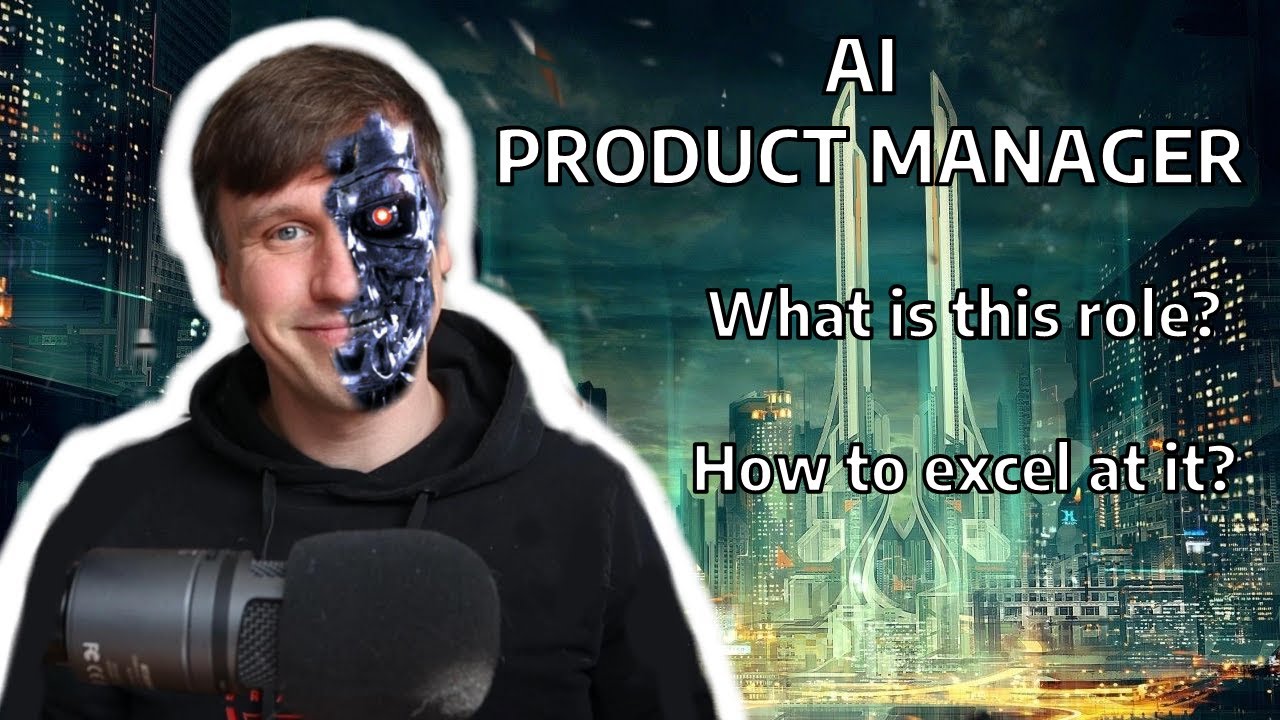
AI Product Manager

Stanford CS25: V2 I Introduction to Transformers w/ Andrej Karpathy
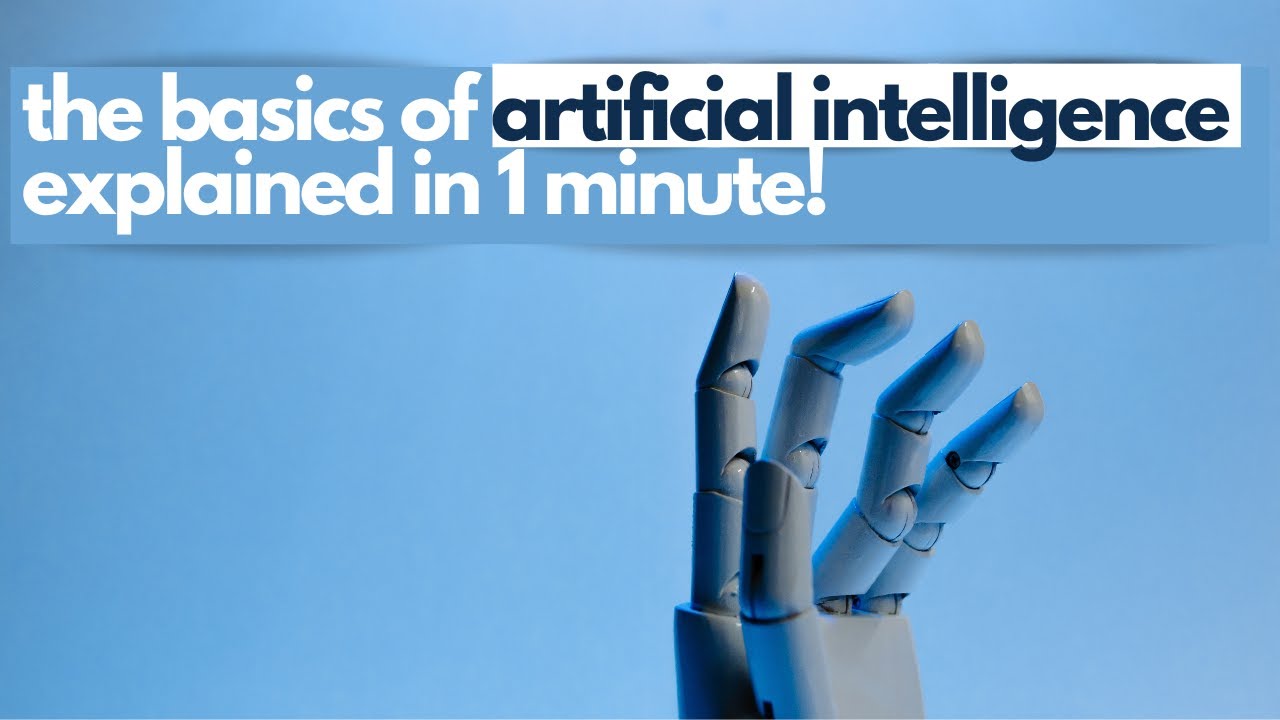
Artificial Intelligence Explained Simply in 1 Minute! ✨
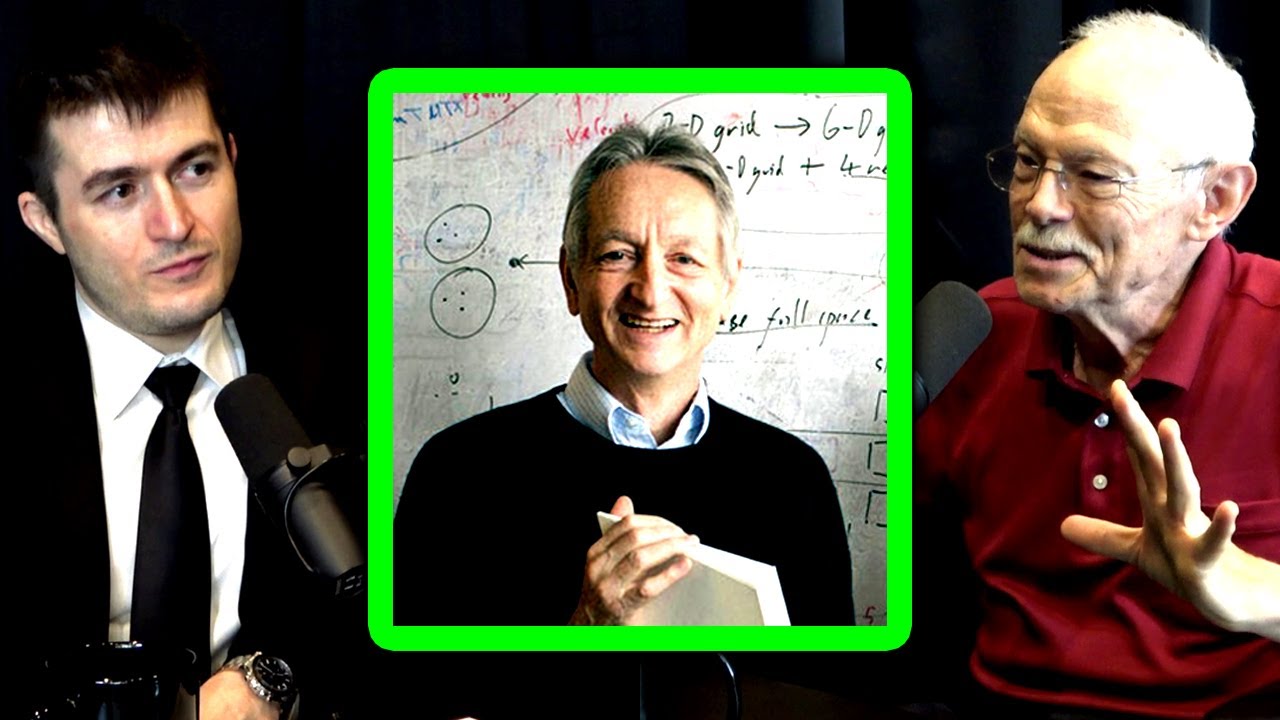
Geoffrey Hinton is a genius | Jay McClelland and Lex Fridman
5.0 / 5 (0 votes)