Business Intelligence & AI
Summary
TLDRLe script présente une nouvelle méthode d'analyse des entreprises, développée par Alis, qui utilise l'intelligence artificielle neuro-symbolique pour la prise de décision. Après cinq années de recherche et développement, cette approche permet de détecter des risques et des opportunités de manière automatisée, en identifiant des signaux d'alerte précurseurs. L'IA spot sept fois plus d'insights que l'exploration humaine, aidant ainsi les analystes à comprendre les causes profondes des problèmes et à élaborer des plans d'action correctifs. L'objectif principal est de repérer des opportunités même dans les unités commerciales en difficulté et des points d'amélioration chez les unités réussies. La solution d'Alis vise à résoudre les problèmes des outils de reporting actuels, en offrant une vision consolidée des données et en mettant en avant l'importance de l'analyse déconsolidée et de la dynamique des données pour les entreprises.
Takeaways
- 🚀 Une nouvelle méthode d'analyse des entreprises est présentée, basée sur une intelligence artificielle neuro-symbolique pour la prise de décision.
- 🔍 L'intelligence artificielle permet d'automatiser la détection des risques et des opportunités, ainsi que la découverte de signaux d'alerte précurseurs.
- 🌟 L'objectif principal est de repérer des opportunités même dans les unités commerciales en difficulté et des points d'amélioration dans les unités réussies.
- 📊 Les outils actuels de reporting sont critiqués pour ne pas offrir d'analyse multidimensionnelle ou de déconsolidation des données.
- 🤖 L'intelligence artificielle générative est combinée avec l'IA symbolique et les graphes de connaissances (KGE) pour identifier la cause profonde des problèmes.
- 🔑 L'algorithme de priorisation Mark of Shame est utilisé pour classer les problèmes business en fonction de leur impact et d'autres critères.
- 🧠 Une solution basée sur l'IA est proposée pour aider les analystes à construire des plans d'action, plutôt que de simplement générer des rapports.
- 📈 Des trackers spécialisés sont utilisés pour analyser les données financières d'entreprise de manière systématique et multidimensionnelle.
- 🗣️ Une interface de speech-to-text est envisagée pour simplifier l'interaction avec l'application et aider à identifier les problèmes non résolus dans les rapports.
- 🔄 L'IA est utilisée pour upskill les analystes et les aider à améliorer leur expertise en matière d'analyse financière.
- 🎯 L'objectif de la solution est de devenir un outil d'analyse complémentaire aux méthodes de reporting traditionnelles, offrant une vision plus large et une aide à la prise de décision.
Q & A
Quel est le nouveau modèle d'analyse des entreprises présenté dans le script ?
-Le nouveau modèle d'analyse des entreprises présenté dans le script est la méthode d'analyse des risques et des opportunités. Elle utilise une intelligence artificielle neuro-symbolique pour la prise de décision, permettant de découvrir automatiquement les signaux d'alerte précoce dans les données de l'entreprise.
Quelle est la durée de recherche et développement pour cette nouvelle méthode d'analyse ?
-La durée de recherche et développement pour cette nouvelle méthode d'analyse est de cinq ans.
Quelles sont les antécédents de l'orateur en matière de science des données ?
-L'orateur a travaillé dans le domaine de la science des données pendant cinq ans avant de rejoindre Alis. Avant cela, il était Directeur Financier (CFO) dans le secteur public en France. Il a également publié beaucoup de contenu lié à la science des données sur LinkedIn et Medium.
Comment l'intelligence artificielle peut-elle aider dans l'analyse des performances d'entreprise ?
-L'intelligence artificielle permet aux organisations d'automatiser la détection et la découverte des risques et des opportunités, ce que l'on appelle les signaux d'alerte précoce. En utilisant cette méthode, on peut identifier sept fois plus d'informations pertinentes que par l'exploration humaine.
Quel est le rôle d'un analyste dans le cadre de cette nouvelle méthode d'analyse ?
-Le rôle d'un analyste est de comprendre la cause fondamentale du problème. Grâce à l'intelligence artificielle, les analystes peuvent automatiquement détecter les anomalies dans les données de l'entreprise, puis trouver la cause racine et bâtir un plan d'action correctif.
Quelles sont les principales limitations des outils de reporting actuels ?
-Les outils de reporting actuels sont principalement basés sur une analyse consolidée des données, ce qui masque les anomalies et les insights pertinents. Ils ne permettent pas une analyse multi-dimensionnelle systématique et ne répondent pas aux questions sur la conversion d'insights en plans d'action.
Pourquoi les entreprises ne détectent-elles pas proactiquement les anomalies avec les logiciels de business intelligence actuels ?
-Les logiciels de business intelligence actuels ne sont pas conçus pour détecter proactiquement les anomalies. Ils laissent aux analystes la responsabilité de faire une analyse multi-dimensionnelle manuelle, ce qui est impossible pour l'homme lorsqu'il s'agit de données à plusieurs dimensions.
Quelle est la solution proposée par Alis pour améliorer la détection d'insights ?
-Alis propose une solution qui utilise de l'intelligence artificielle, combinant l'IA générative et l'IA symbolique sous forme de graphes KGE, pour trouver la cause racine et bâtir un plan d'action correctif. Cette méthode permet de repérer jusqu'à sept fois plus d'insights qu'avec les méthodes traditionnelles de reporting manuel.
Comment la solution d'Alis aide-t-elle les entreprises à réagir plus rapidement que leurs concurrents ?
-La solution d'Alis permet de détecter les anomalies et les risques plus tôt que les concurrents, grâce à une analyse multi-dimensionnelle systématique et automatisée. Elle offre également des insights sur les actions correctives à prendre, permettant aux entreprises de réagir rapidement et de s'adapter à la situation.
Quelle est la vision globale de la solution proposée par Alis pour l'analyse des entreprises ?
-La vision globale de la solution proposée par Alis est de trouver des opportunités même dans les unités d'affaires en difficulté et des points d'amélioration dans les unités prospères. Elle vise à aider les entreprises à répondre aux questions critiques et à suivre les KPIs pertinents grâce à une analyse plus approfondie et à une prise de décision plus éclairée.
Quelle est la méthode proposée par Alis pour améliorer la qualité des données et la pertinence des insights ?
-Alis utilise une approche de traitement par lots pour détecter les insights chaque mois en utilisant des données financières de qualité supérieure. Ils ont également développé un algorithme de priorisation pour gérer le flot d'alertes et pour identifier les insights les plus importants en fonction de la stratégie de l'entreprise et de la situation urgente.
Outlines
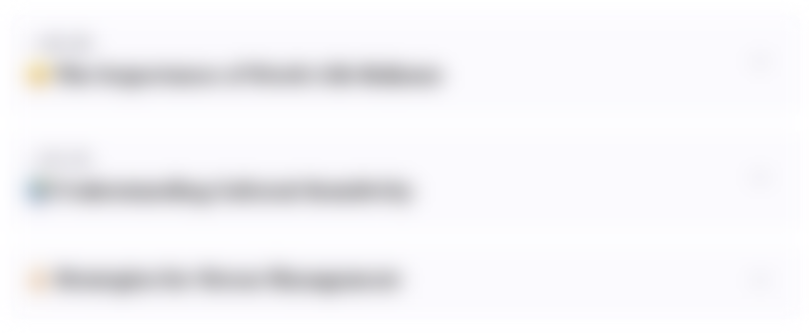
This section is available to paid users only. Please upgrade to access this part.
Upgrade NowMindmap
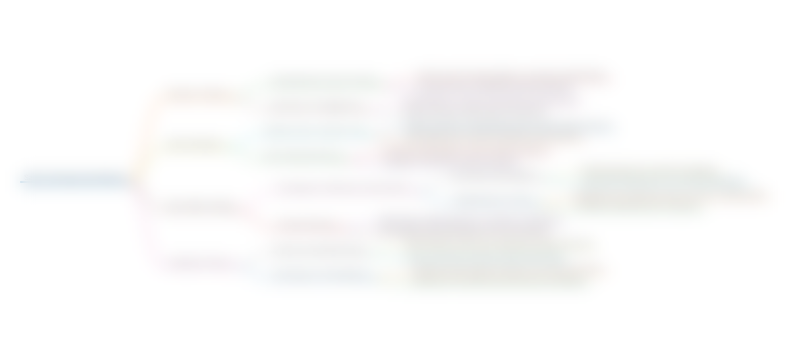
This section is available to paid users only. Please upgrade to access this part.
Upgrade NowKeywords
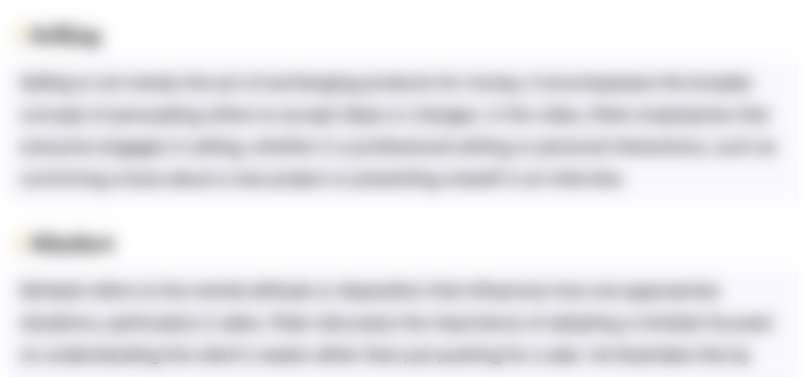
This section is available to paid users only. Please upgrade to access this part.
Upgrade NowHighlights
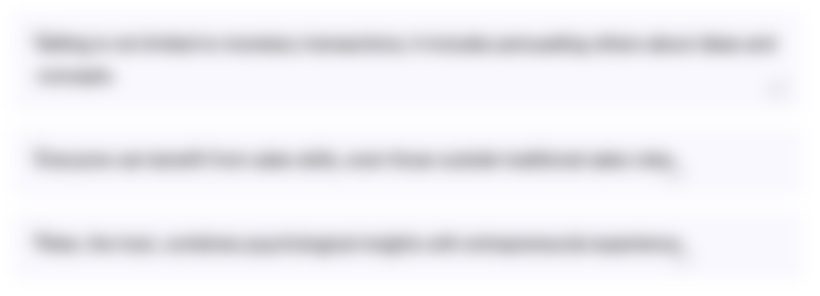
This section is available to paid users only. Please upgrade to access this part.
Upgrade NowTranscripts
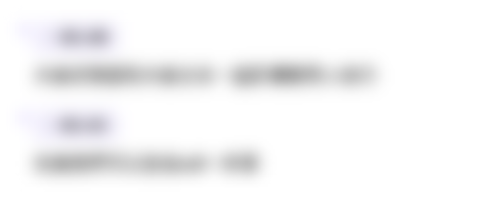
This section is available to paid users only. Please upgrade to access this part.
Upgrade NowBrowse More Related Video

Cartographie des environnements : un outil indispensable pour les analyses stratégiques • FRANCE 24

IA : faisons le point sur l'intelligence artificielle

On von Neumann's Theory of Games and Economic Behavior
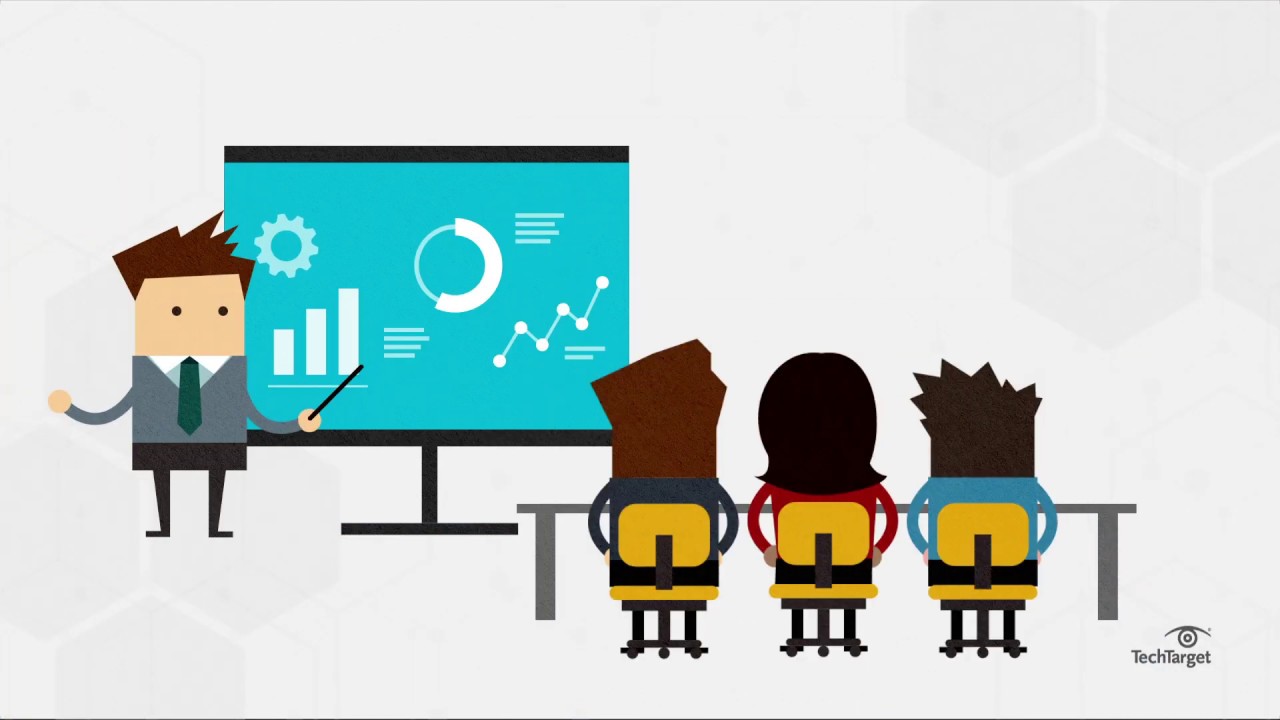
What is Business Intelligence (BI) (2020)
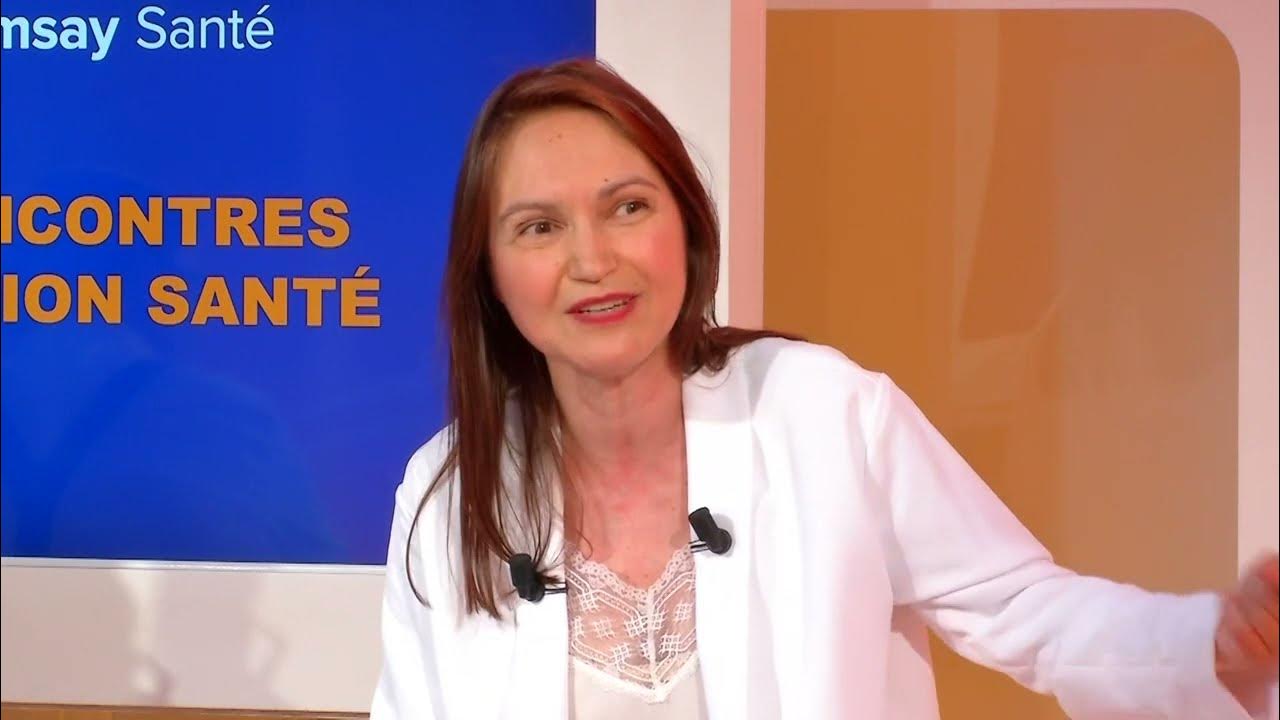
Intervention Marie Quartier, fondation Ramsay: la méthode de la préoccupation partagée
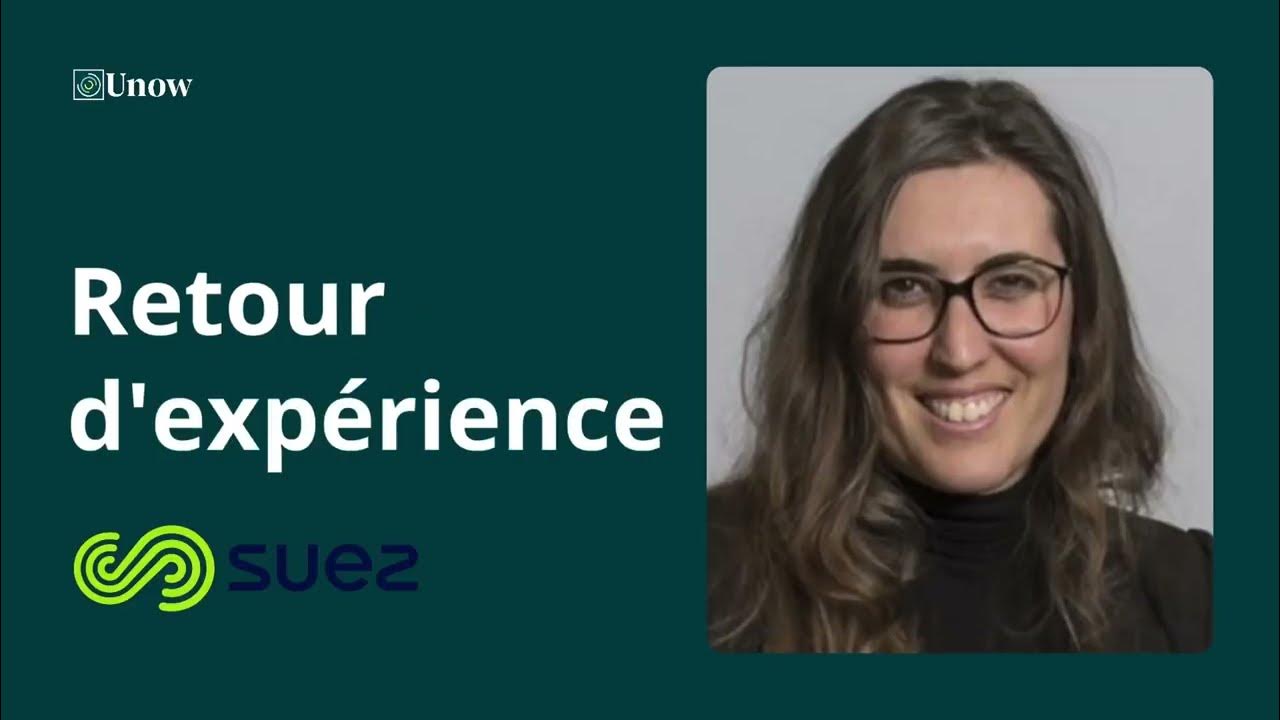
Interview client - Perrine Renevey, Suez
5.0 / 5 (0 votes)