Open sourcing the AI ecosystem ft. Arthur Mensch of Mistral AI and Matt Miller
Summary
TLDRArthur, founder and CEO of mistal AI, discusses the company's rapid growth and focus on open-source AI models. Despite being a young company, they've made significant strides by releasing high-quality models comparable to GPT-4 and forming strategic partnerships. Arthur emphasizes the importance of balancing open-source contributions with commercial interests and shares insights on the future of AI, including multilingual and multimodal models. He also highlights the advantages of building a business in Europe and the company's vision for AI's role in the next five years.
Takeaways
- ๐ Arthur, the founder and CEO of mistol AI, has led the company to release high-quality models rivaling GPT-4 in just nine months since its inception.
- ๐ The company's success is attributed to its open-source approach and the ability to efficiently develop models with a lean team of experienced individuals.
- ๐ค Mistol AI has established strategic partnerships with major companies like Microsoft and Snowflake, indicating a strong go-to-market strategy.
- ๐ง The decision to start mistol AI was driven by the founders' desire to see AI progress through open exchanges, which they felt was lacking in the field since 2022.
- ๐ The company aims to bring AI technology to every developer, promoting a more open platform than competitors, and accelerating adoption through this strategy.
- ๐ The balance between open-source and commercial models is managed by offering two families of models, ensuring leadership in open source while driving commercial adoption.
- ๐ก Mistol AI's rapid development is attributed to hands-on work with data and a team that is willing to engage in the less glamorous aspects of machine learning.
- ๐ The company is focused on improving its large models and developing open source models for specific vertical domains.
- ๐ Being a European company provides Mistol AI with unique advantages, such as access to a strong talent pool and linguistic capabilities, as well as geographical opportunities.
- ๐ฎ Looking ahead, Mistol AI envisions a future where AI infrastructure is open, enabling the creation of assistance and autonomous agents accessible to all users.
- ๐ผ For founders in the AI space, the advice is to maintain an ambitious mindset and be prepared to build and reinvent from scratch every day, as the AI landscape is ever-evolving.
Q & A
What motivated Arthur and his co-founder to start mistol AI?
-Arthur and his co-founder were inspired by the open exchanges between academic and industrial labs that contributed to the progress of AI. They were disappointed that this openness stopped early in the AI journey and wanted to push the field back towards more open source contributions, especially given the rapid advancements in AI technology.
How does mistol AI balance open source contributions with commercial interests?
-Mistol AI maintains two families of models - one focused on open source to lead in that domain, and another for commercial purposes. They aim to stay relevant by continuously producing open source models while also developing better commercial models available on various cloud providers.
What is the advantage of being a European AI company like mistol AI?
-Being a European company allows mistol AI to tap into a strong pool of junior talent from countries like France, Poland, and the UK. Additionally, they benefit from support at the state level and have a geographical advantage in serving the European market, including having a strong French language model.
What are some of the challenges mistol AI faces in maintaining its position in the AI field?
-Mistol AI faces the challenge of staying ahead in the rapidly evolving AI field. They need to balance contributing to the open source community while also securing commercial adoption and enterprise deals to sustain their business model.
What is mistol AI's strategy for the future in terms of model development?
-Mistol AI is working on improving their existing models and developing open source models for various vertical domains. They are also focusing on multilingual and multimodal models and plan to make customization and fine-tuning part of their platform.
How does Arthur view the future of AI technology?
-Arthur envisions a future where AI technology becomes more autonomous, with the ability to create assistants and autonomous agents that can perform a wider range of tasks. He expects AI to become so controllable through human language that creating such agents will be a common skill learned at school.
What are some of the most exciting applications that mistol AI has seen built on their models?
-Mistol AI has seen startups in the Bay Area using their models for fine-tuning and fast application development. They have also seen web search companies and enterprises using their models for knowledge management, marketing, and other standard enterprise applications.
How does mistol AI support its community of developers?
-Mistol AI invests in developer relations, creating guides and gathering use cases to showcase what can be built with their models. They encourage developers to engage with them to discuss use cases, advertise their applications, and provide insights for future evaluations and improvements to their models.
What is mistol AI's approach to partnerships with companies like Snowflake and Databricks?
-Mistol AI believes that AI models become stronger when connected to data. They have formed partnerships to run natively in the clouds of these companies, allowing customers to deploy mistol AI's technology where their data resides, which they see as important for the future of AI deployment.
How does mistol AI decide on the size of the models they develop?
-The decision on model size is based on scaling laws and depends on the compute resources available, the desired training and inference costs, and the balance between latency and reasoning capabilities. Mistol AI aims to have a family of models ranging from small to very large ones.
What advice does Arthur have for founders in the AI space?
-Arthur advises founders to always act as if it's day one, to be ambitious, and to dream big. He emphasizes the importance of continuous exploration and innovation while also leveraging existing achievements to stay relevant in the rapidly evolving AI field.
Outlines
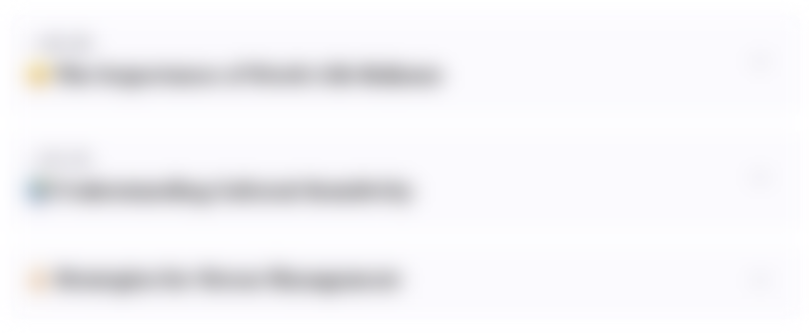
This section is available to paid users only. Please upgrade to access this part.
Upgrade NowMindmap
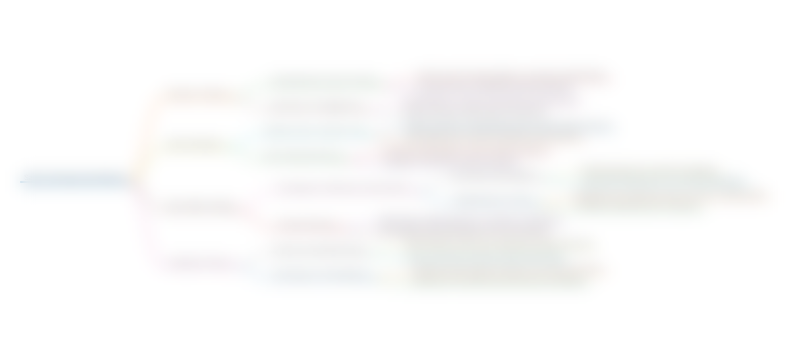
This section is available to paid users only. Please upgrade to access this part.
Upgrade NowKeywords
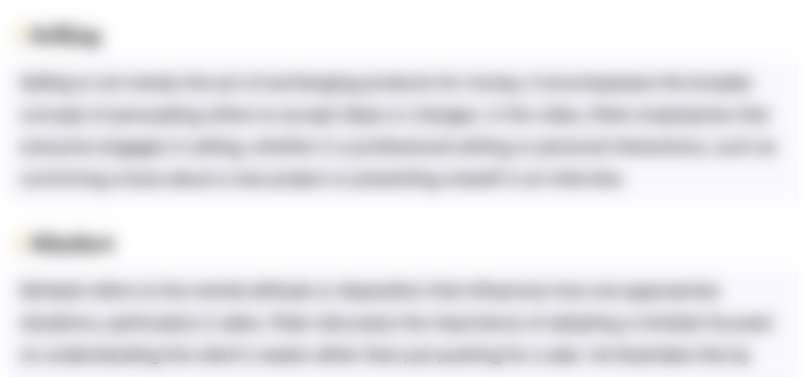
This section is available to paid users only. Please upgrade to access this part.
Upgrade NowHighlights
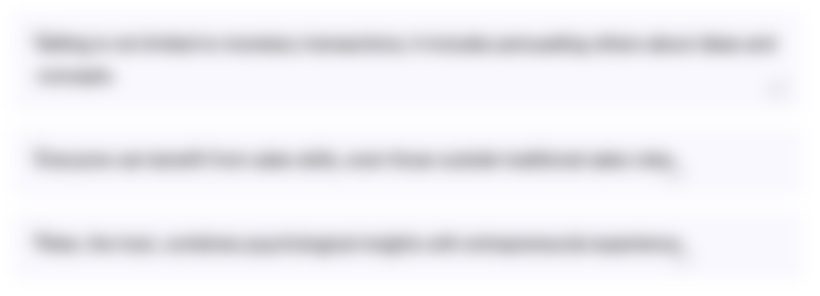
This section is available to paid users only. Please upgrade to access this part.
Upgrade NowTranscripts
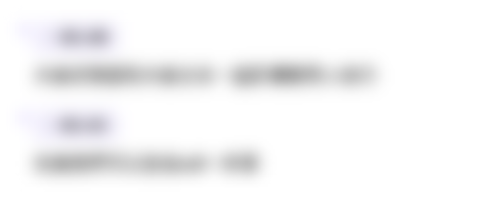
This section is available to paid users only. Please upgrade to access this part.
Upgrade NowBrowse More Related Video
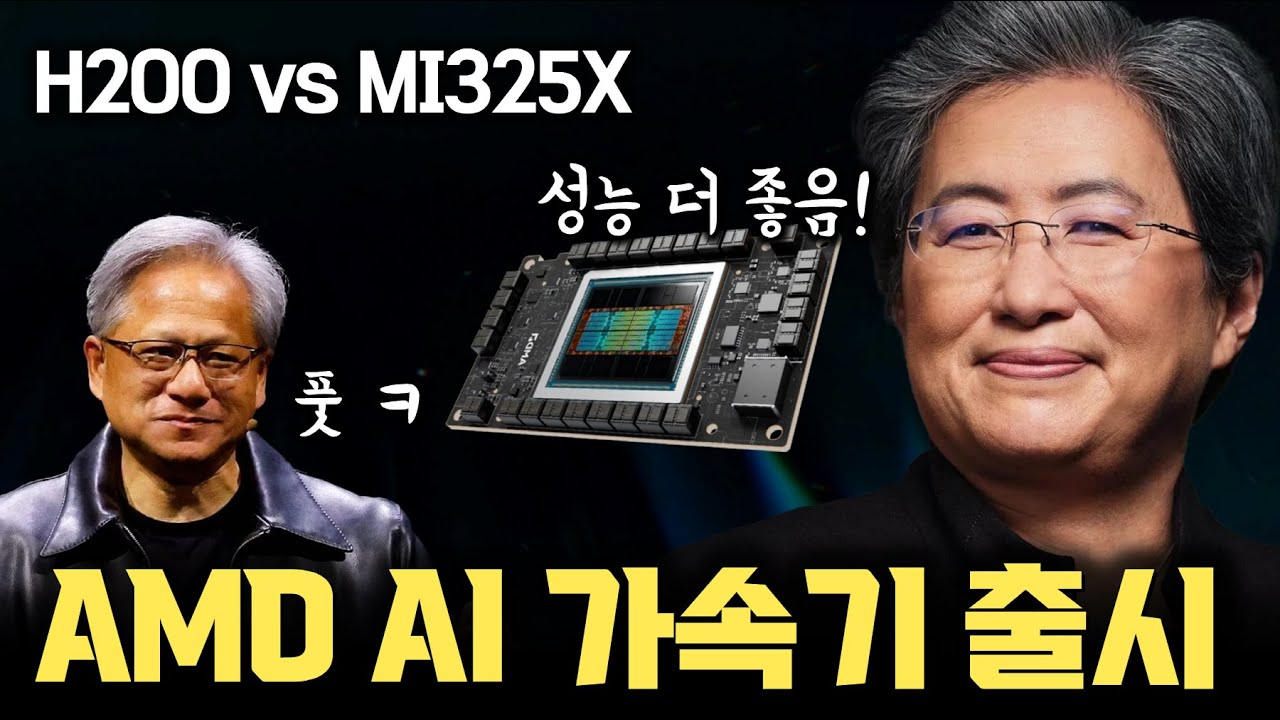
AMD ๋ฆฌ์ฌ์, NVIDIA GPU ๋์ HBM3E ๊ธฐ๋ฐ Instinct MI325X ๋ฐํ | CUDA, infiniband, NVLink ๋์ SW ๋ฐ ๋คํธ์ํน ํ๋ซํผ ์ ๊ทธ๋ ์ด๋
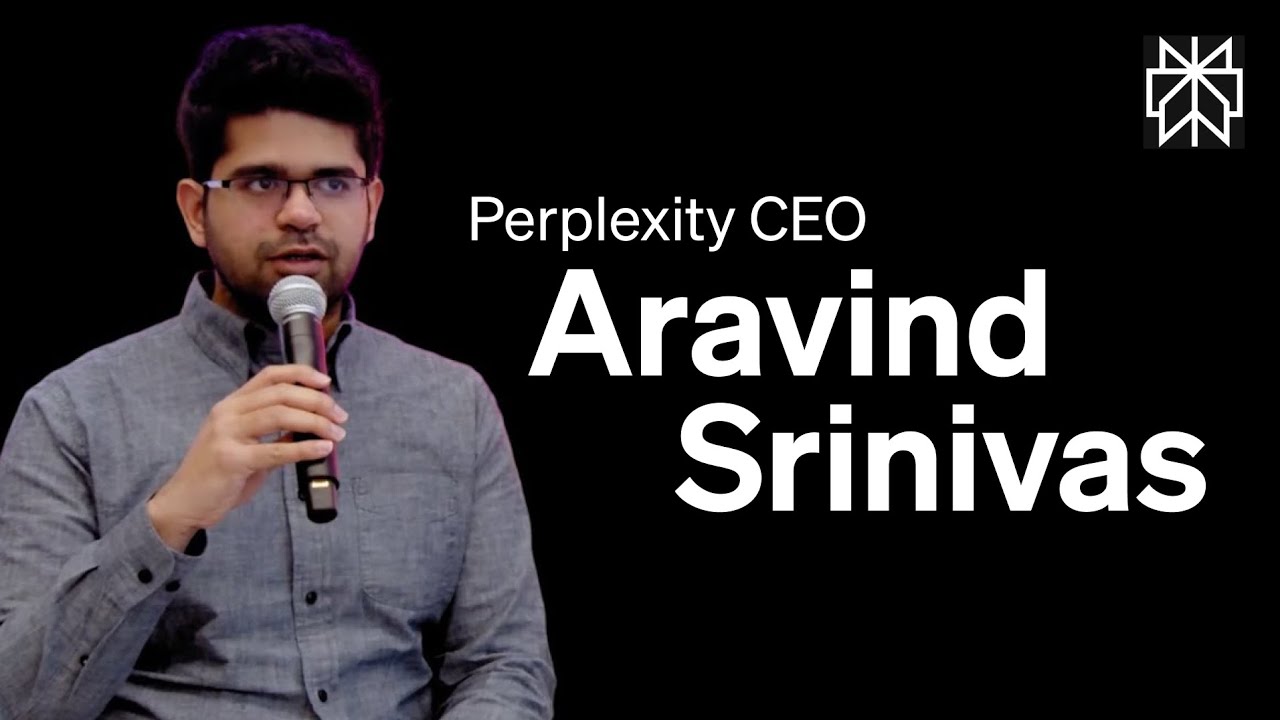
Aravind Srinivas (Perplexity) and David Singleton (Stripe) fireside chat
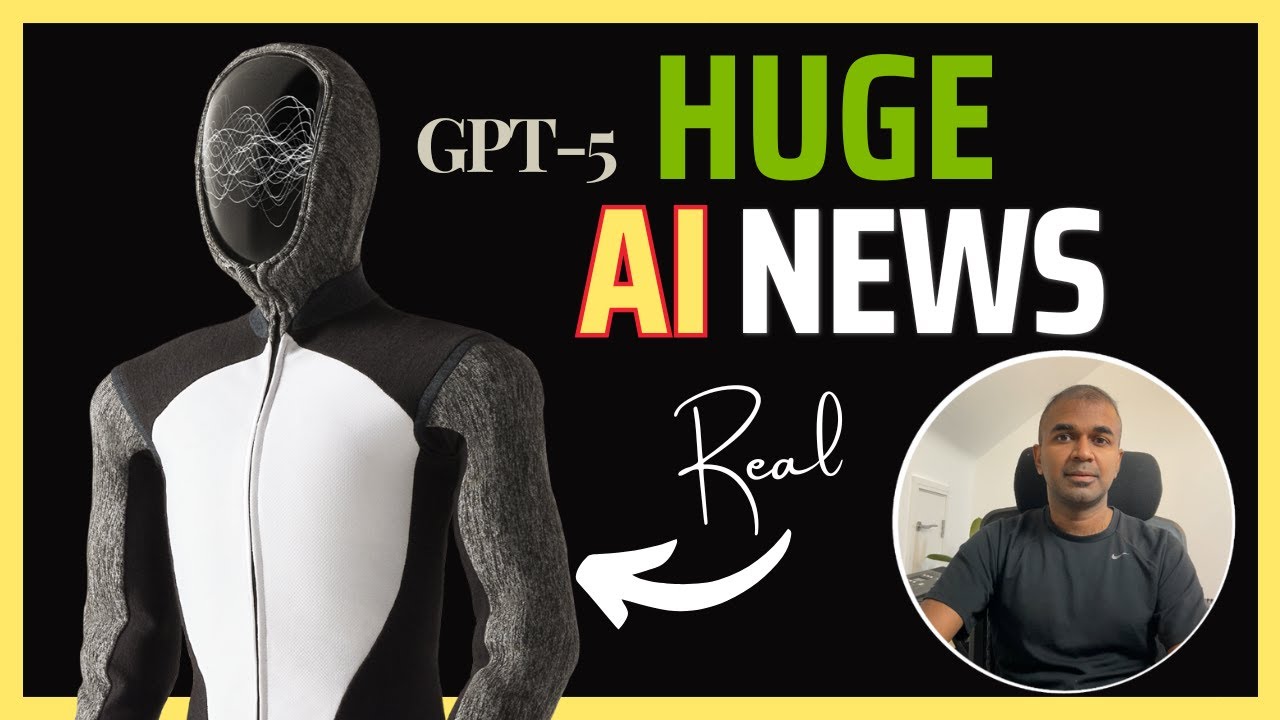
AI News: GPT 5, Cerebras Voice, Claude 500K Context, Home Robot
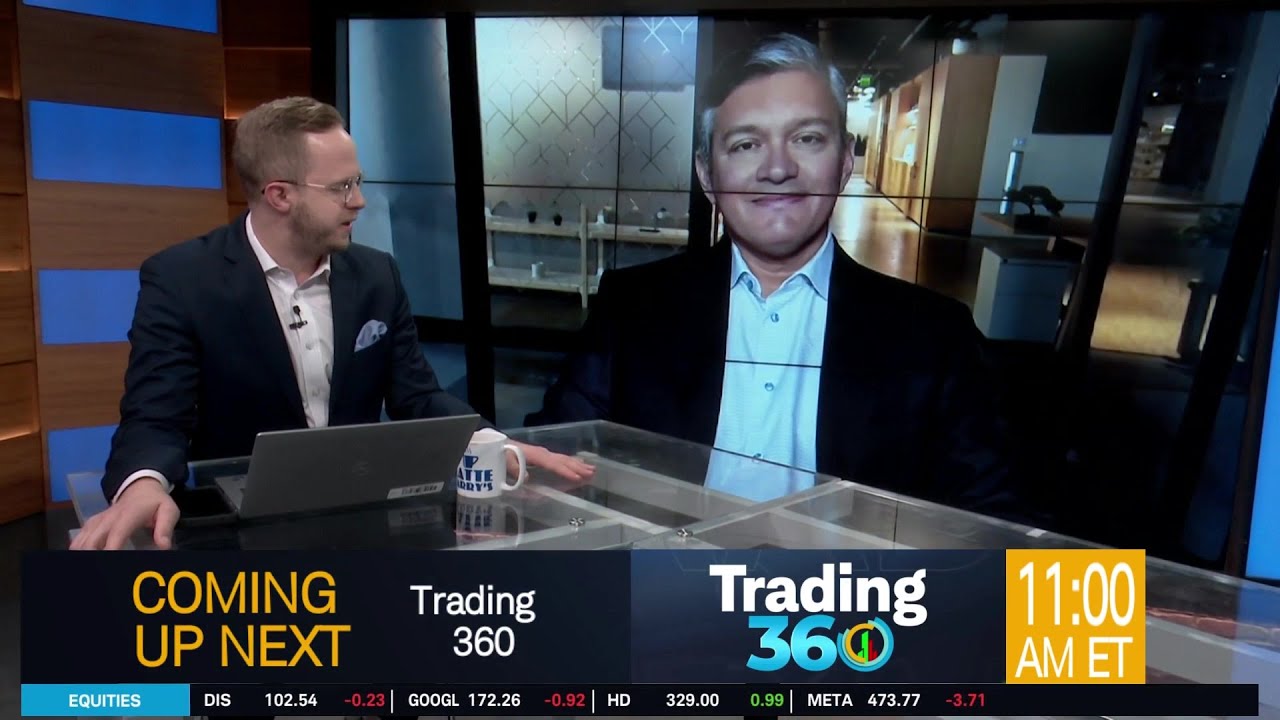
Elastic (ESTC) CEO on How the Company Uses A.I.
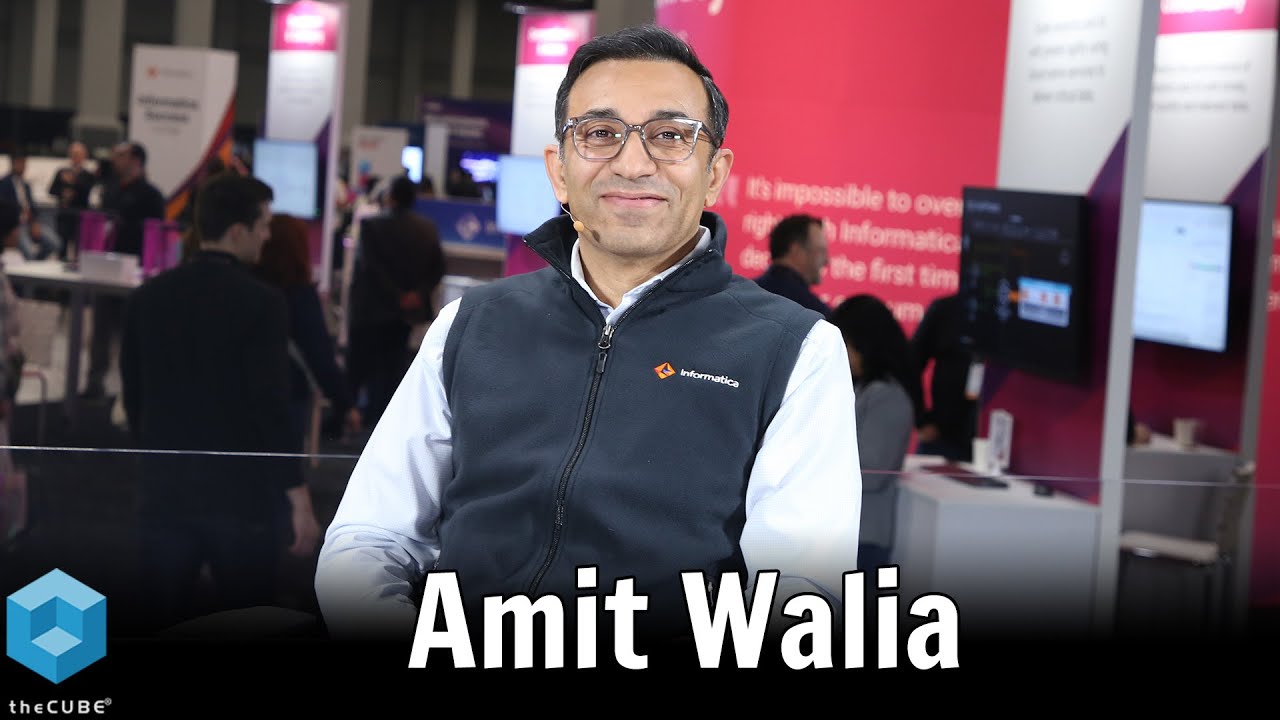
Amit Walia, Informatica | Informatica World 2024
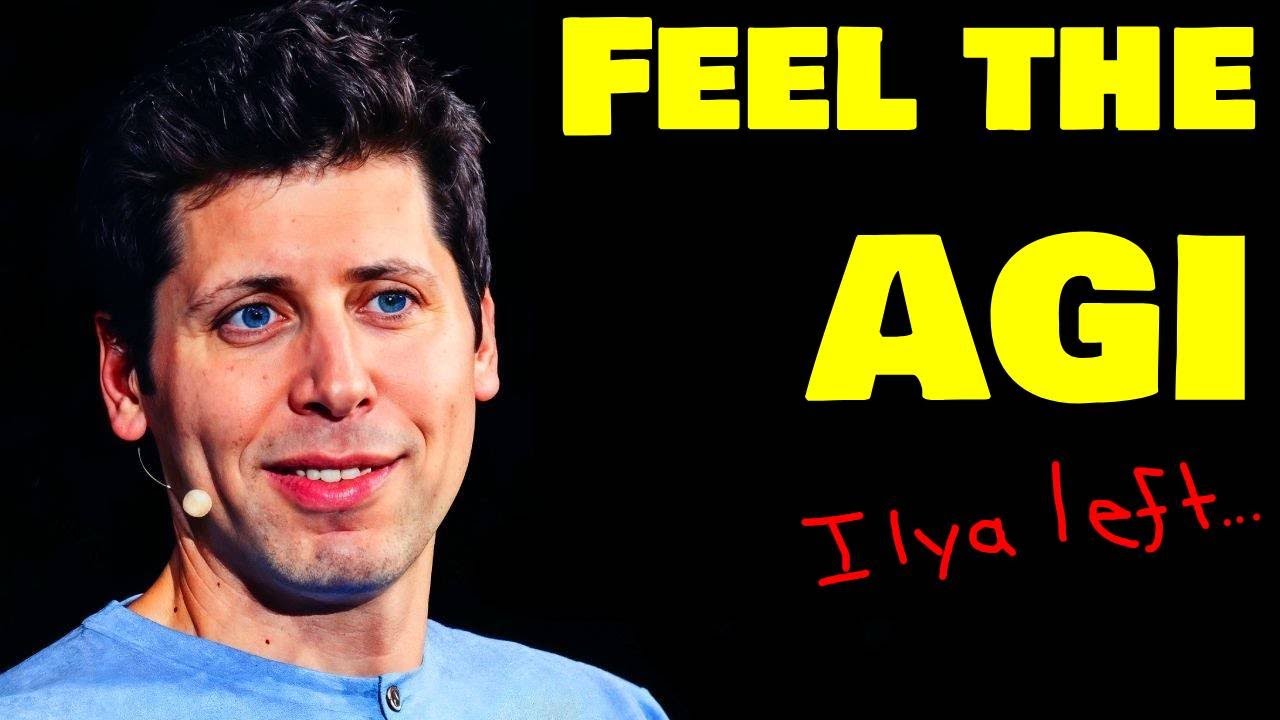
AI News: The AI Arms Race is Getting INSANE
5.0 / 5 (0 votes)