Elastic (ESTC) CEO on How the Company Uses A.I.
Summary
TLDRIn a recent interview, Ashutosh Kulkarni, CEO of Elastic Data Analytics, discusses the company's growth and innovative approach to AI and large language models (LLMs). He emphasizes the importance of maintaining privacy by keeping proprietary data secure while leveraging AI to extract insights from unstructured data. Kulkarni highlights the significance of their retrieval augmented generation model, which enhances the accuracy of AI-generated responses without exposing sensitive information. With a focus on organic growth and potential acquisitions, he shares optimism about Elastic's future in the AI landscape, underscoring their strategic plans for expansion and profitability.
Takeaways
- 🚀 Elastic Data Analytics is positioned as a search AI company, helping businesses extract insights from messy and unstructured data.
- 📈 The company reported a 20% growth in Q4 and a 32% growth in its cloud business, reflecting consistent performance.
- 🔍 Their platform allows businesses to use their private data securely, enhancing AI applications without compromising proprietary information.
- 🛡️ Retrieval augmented generation is a key mechanism that provides context to large language models while maintaining data privacy.
- 🤖 Large language models are trained on publicly available data, making it essential for businesses to protect their proprietary information.
- 👥 Elastic has a strong internal talent pool in machine learning and continues to hire experts in the field to enhance its capabilities.
- 💼 The company has over $1 billion in cash, which may be used for acquisitions or to support organic growth.
- 🌱 Elastic aims to build a generational company, focusing on long-term growth and profitability while capturing market share.
- 📊 Operating margins have improved, with non-GAAP operating margins projected to increase from 11% to over 12% in the current year.
- 🔮 The CEO emphasizes the massive opportunity within the AI space and the importance of leveraging business strengths for future success.
Q & A
What is the core business model of Elastic Data Analytics?
-Elastic Data Analytics positions itself as a search AI company, focusing on helping businesses extract insights from unstructured and semi-structured data.
How does Elastic integrate AI into its search tools?
-Elastic utilizes a mechanism called retrieval augmented generation to provide private data context to large language models, allowing businesses to build generative AI applications securely.
What recent growth metrics did Elastic report for Q4 of fiscal 2024?
-In Q4 of fiscal 2024, Elastic reported a 20% growth for the quarter, with its cloud business experiencing a growth of 32%.
What concerns might clients have regarding data privacy when using AI models?
-Clients are concerned about the potential risk of handing over proprietary data to large language models, which have only been trained on publicly available information.
How does Elastic ensure data security for its clients?
-Elastic allows clients to keep their private data within their environment, ensuring that privacy controls are defined by the clients themselves.
What is the significance of the term 'retrieval augmented generation' in Elastic's services?
-Retrieval augmented generation refers to Elastic's method of providing real-time context from private data to large language models, allowing for precise and relevant answers without compromising data privacy.
How does Elastic plan to build its workforce to support AI integration?
-Elastic aims to grow its workforce by hiring new talent with machine learning expertise and leveraging existing talent within the company, along with considering technology tuck-in acquisitions.
What strategies does Elastic have for utilizing its significant cash reserves?
-Elastic plans to use its cash reserves for both organic growth and potential acquisitions to capture more market share and support long-term growth.
What are the expected operating margins for Elastic in the current fiscal year?
-Elastic anticipates its non-GAAP operating margin to exceed 12% in the current fiscal year.
Why is Elastic's search platform considered essential for businesses?
-The search platform is crucial for businesses because it connects their proprietary data with large language models, enabling them to extract valuable insights without exposing sensitive information.
Outlines
🤖 Harnessing AI for Business Growth
In this segment, the discussion revolves around the integration of AI and Language Model Systems (LMS) at Elastic Data Analytics, led by CEO Ashutosh Kulkarni. The company has experienced a notable growth of 40% in the past year, showcasing its strength in AI-driven search solutions. Kulkarni describes Elastic as a search AI company, emphasizing its role in helping businesses, both large and small, derive insights from unstructured and semi-structured data. The company focuses on privacy and security by allowing clients to maintain control over their private data while leveraging generative AI applications. Kulkarni also highlights a 20% growth in Q4 and a 32% increase in the cloud business, asserting that their innovative approach positions them well in a rapidly evolving market.
🔍 The Future of Search and Data Privacy
This paragraph addresses the challenges and opportunities presented by the rapid development of language models and AI technologies. Kulkarni underscores the importance of proprietary data for businesses, arguing that many organizations are hesitant to share sensitive information with external language models. He explains that Elastic's platform enables real-time connections between private data and large language models, ensuring that responses are accurate and contextual without exposing proprietary information. This capability reduces the risk of data leakage while enhancing the precision of the responses generated, positioning Elastic's technology as essential for companies looking to harness AI responsibly.
Mindmap
Keywords
💡AI (Artificial Intelligence)
💡LMS (Large Language Models)
💡Data Privacy
💡Search AI
💡Retrieval-Augmented Generation
💡Generative Applications
💡Market Share
💡Operating Margins
💡Tuck-in Acquisitions
💡Proprietary Data
Highlights
Elastic Data Analytics has experienced a 40% increase in business over the past year.
The company positions itself as a search AI firm, combining the precision of search with AI intelligence.
Elastic works with Fortune 500 companies and government organizations to extract insights from unstructured data.
AI enables businesses to derive value from messy data in unprecedented ways.
Elastic provides a mechanism called Retrieval Augmented Generation to integrate private data into LLMs.
The company ensures that sensitive data remains within the client’s environment for security.
Businesses prefer not to expose proprietary data to external LLMs trained on public information.
Retrieval Augmented Generation helps LLMs provide precise answers without needing access to proprietary information.
Kulkarni highlights the importance of a company's private data in differentiating and competing in the market.
Elastic has invested in machine learning techniques for years, building native models on Elasticsearch.
The company is hiring new talent with machine learning expertise while also considering acquisitions.
Kulkarni mentions the significant cash reserves of about $1 billion for potential acquisitions and organic growth.
Elastic aims to capture market share while maintaining a focus on long-term growth and profitability.
The company has demonstrated consistent revenue growth exceeding expense growth, improving operating margins.
Elastic's strategy includes leveraging both organic and inorganic growth opportunities to expand in the AI space.
Kulkarni expresses optimism about the future potential for growth through market expansion and acquisitions.
Transcripts
tech. Let's talk about
harnessing AI and LMS with the
CEO of Elastic Data Analytics
and Business. That's up about
40% in the past year. Just had
earnings to joining us. Ashutosh
Kulkarni is a CEO at Elastic
Ticker STC. Ash, thanks for
being here on the show with us
this morning. Welcome to the
Schwab Network. Thank you very
much. It's great to be here.
Tell us about your business and
how you guys are dealing with
the avant garde of AI and LMS,
because it seems like you're
kind of right in the middle of
it. Yeah. So, you know, we
describe ourselves as a search
AI company. We bring the
precision of search and the
intelligence of AI and help you
help businesses, companies, big
and small. We work with the
largest fortune 500 companies as
well as government organizations
all around the world to get
insights out of their really
messy, unstructured,
semi-structured data. And as you
know, there's a lot of it. And
AI is providing an amazing
mechanism to really discover and
get value out of that. That
information in ways that were
never possible. And we bring
your private data context to
these large language models. And
through that, we allow you to
bring and build a generative AI
applications for your business.
And that probably is the most
exciting part of our, our,
business today, we just as you
mentioned, announced earnings
for Q4, and our fiscal 24, we
delivered, 20% growth in the
quarter, our cloud business grew
by 32. So a very strong, sort of
beat across all metrics. Your,
growth, too, has been very
consistent, basically at 20,
give or take a couple percent
for about the past year. And it
seems like analysts believe
you're going to be hitting that
going forward. So when you talk
about applying the new
technology into your search
tools and the products that you
provide to big companies, a lot
of big banks, big retailers, is
that coming from in-house
development, how are you putting
that on top of your offerings?
Is it a layer that you're
building, or can you just source
it from what's available
publicly through these language
models? So our core strength is
our search platform and what it
does is it takes all of your
private data. So you know,
businesses, our customers will
bring their private data onto
elastic where it stays within
their environment. So it's
protected. It's safe. You know,
they're able to define all the
privacy controls and so on. And
then through this mechanism
called retrieval augmented
generation, we're able to
provide that internal private
context to these large language
models that could be running,
you know, through the Azure
OpenAI service or through
Google's Gemini models, or
through Amazon Bedrock. You
know, there are so many out
there now, this entire space is
just growing, and you're seeing
models even open up in in open
source. But fundamentally, the
way our customers use us is to
provide that private context
without shipping all your
private proprietary data. And so
they're able to build these
generative applications in a
very privacy first security
forward model. And that's really
the future. You know, that's how
these applications are going to
get built. Is there any risk
that your clients or customers
would say, okay, we used to pay
elastic to do these search
functions for us. Now we can
just plug our data into one of
these models and have them
program it for us. We don't need
to have specialty programmers.
Do you have to stay one step
ahead of that or what do you see
as any potential risk that this
can be done in house? Well,
that's, you know, if you think
about any business out there,
whether you are a retail store,
whether you are a manufacturing
company, a financial services
company, your data is your
lifeblood, your information
about your customers, your
proprietary, you know,
processes. That's what you
differentiate on. That's what
you compete on. Nobody wants to
hand off all of that private
data to these large language
models. And that's the reason
why these large language models,
whether it's from OpenAI or
Google or whoever, they've all
been trained on publicly
available non proprietary
information that's out there on
the internet that you can just
scour and learn from and so on.
They have no concept, they have
no understanding of a
business's, you know, private
proprietary information. And
that's exactly where retrieval
augmented generation and our
search AI platform comes in. We
connect the dots in real time.
So when a question gets asked we
are able to in real time tell
the large language model only
answer this question based on
these few documents. Because of
that, the large language model
does not hallucinate, it knows
exactly how to construct the
answer. It's able to give very
precise answers, and it does not
need to have access to all of
your proprietary information,
which is what all of these
businesses care about. So this
model is the only way businesses
truly expect to be able to do
things going forward. And that's
what's so exciting about the
space that we're in. So do you
have to hire new people that
know how to do this? But then
House or these, programmers that
are are adapting to this because
if we are to believe that this
technology is completely novel
in the last 18 months or year,
how do you build a workforce
around that? Have you needed to
go out and find, kind of new
ways to think about it or is it
able to just kind of adapt and
tweak, with then, the workers
and the expertise you already
have? That's a great question.
And, you know, look, large
language models, foundation
models, as they often refer to,
you know, this is body of work
that's been going on in academia
and in a lot of companies for a
very long time now. ChatGPT
really came onto the stage about
a year and a half ago, and this
was the first real attempt by
any company to get this out,
democratize the space. And since
then, this is, you know, it's
become part of our daily
conversations. But the reality
is that there's been a group of
people who have been working on
these machine learning
techniques for some time now,
you know, within elastic, much
like, you know, with other
companies out there, with a few
other companies out there, we've
been investing in this space for
the last eight, nine years, you
know, we've built ways to make
machine learning models work
natively on data that's stored
in Elasticsearch, which is our
core platform. And we've evolved
that over the years. So we both
have a big pool of organic
talent within the company. We
are growing and we are hiring
people that bring that
additional ML expertise to us.
But we are also a company that
has done, you know, technology
tuck in acquisitions in the
past. So if we find a group of
people that have the same
mission that we are on, that
have that expertise, you know,
we've always looked at, being
acquisitive where it makes sense
, bringing that technology to
help us accelerate our future
forward, but I expect that we'll
do all of those, but feel really
good about our ability to
attract that kind of talent. The
question I was going to ask
maybe just got answered in some
ways, but you had about $1
billion in cash or equivalents
on your balance sheet as of the
last earnings. So I guess if you
do more tuck ins or acquisitions
, there's one use of it. But,
that seems like a pretty chunky
amount of cash when you're,
doing about 300 million a
quarter in revenues. So what do
you want to do with it? Is there
any low hanging fruit, apart
from acquisitions, or is that a
way to put some of that money to
work? Yeah. Fundamentally, what
our investors keep telling us
is, you know, the opportunity is
so large and we believe this in
our hearts. The opportunity is
so massive. We are right in the
sweet spot of AI. And it's
really important that as we look
at the long term, we believe
that we have the opportunity to
build a generational company.
That means we stay focused on
the right way to keep growing,
capture as much market share as
we can. And that means, you
know, deploying this capital
towards not just inorganic, what
we just talked about, but even
our organic growth to make sure
that we can continue to grow
that business and capture more
and more share. We believe that
there's a lot of leverage in our
business, inherent leverage in
our business. So even as we are
growing revenue, what we've
demonstrated in the last many
years is we can grow our revenue
more than we grow our expenses
in a consistent manner. And that
means our operating margins are
non-GAAP. Operating margins have
continued to grow last fiscal
year, we delivered, 11% non-GAAP
operating margin. And the guide
that we've been given for the
current year that we've just
gotten into, is, over 12. So we
believe that, you know, we can
keep doing that in the right way
and keep growing it, in a way
that is going to be right for
long term growth as well as
profitability. Okay ash, thanks
for the explanation, sounds like
we should keep an eye on, some
potential growth via your
market, but also, potential
acquisitions. So appreciate the
insight. Thank you very much.
Absolutely. Ash Kulkarni, CEO at
elastic. All right. Wh
Browse More Related Video
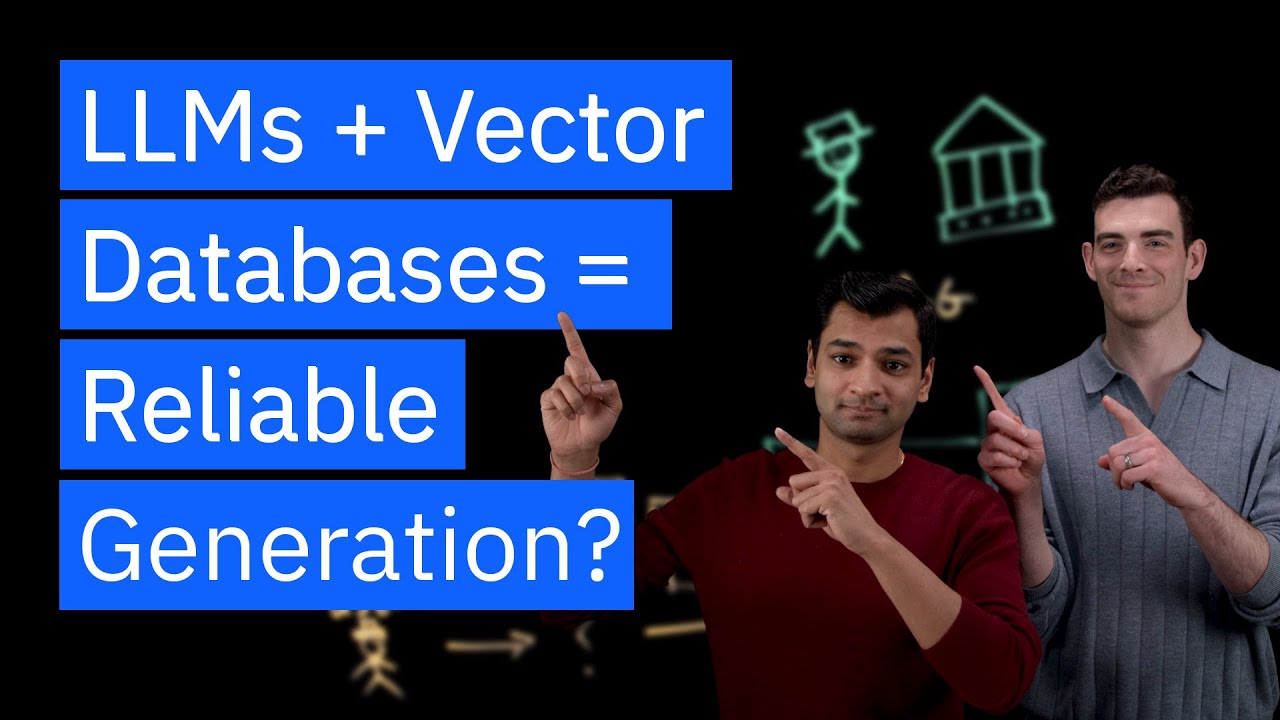
RAG Explained
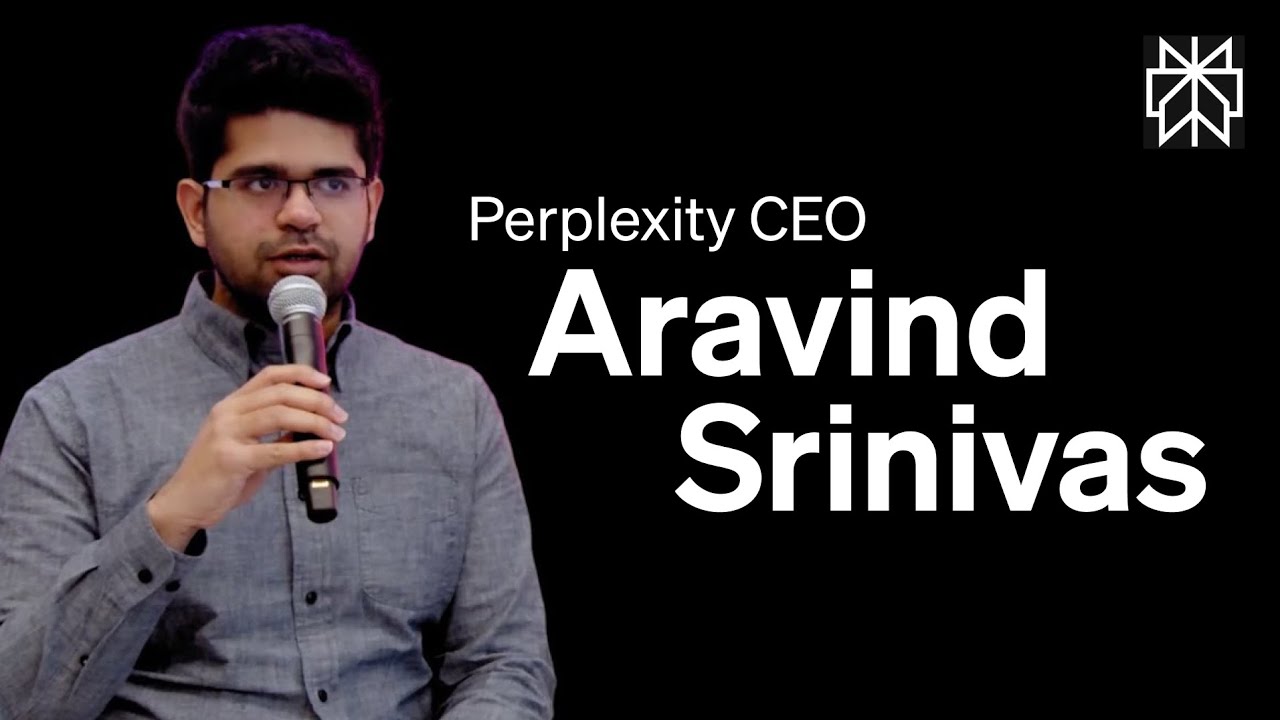
Aravind Srinivas (Perplexity) and David Singleton (Stripe) fireside chat
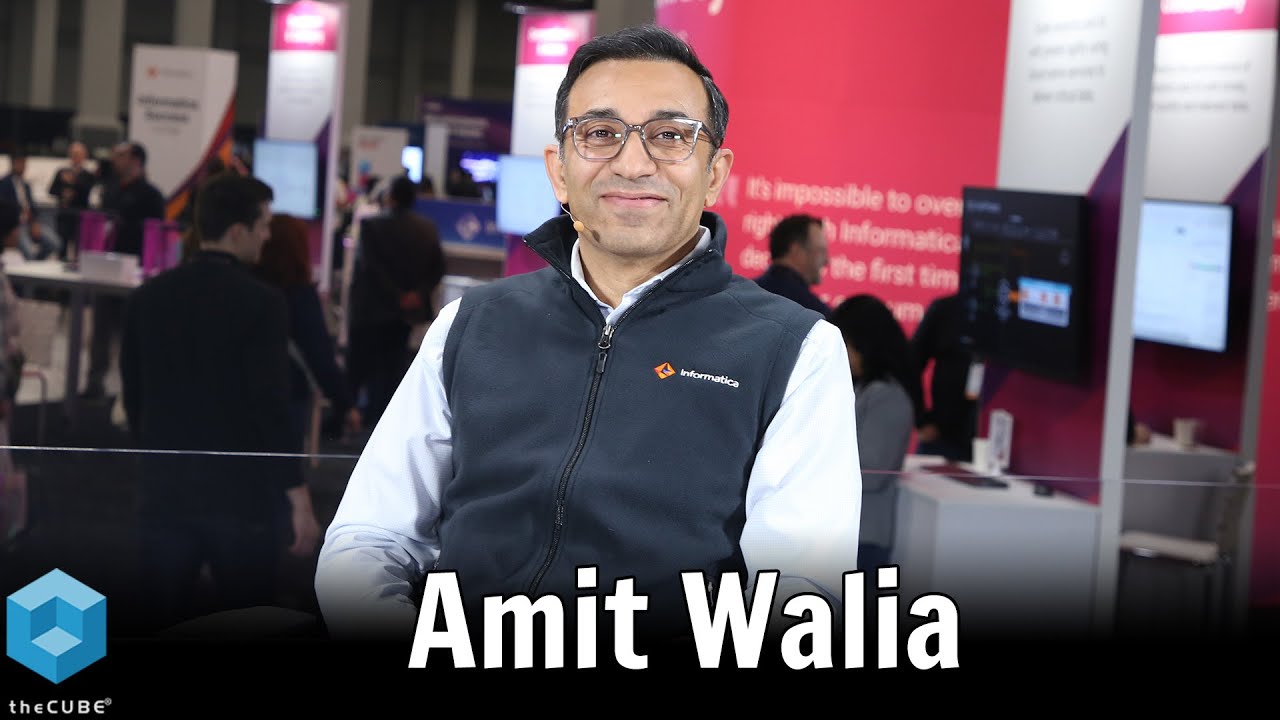
Amit Walia, Informatica | Informatica World 2024
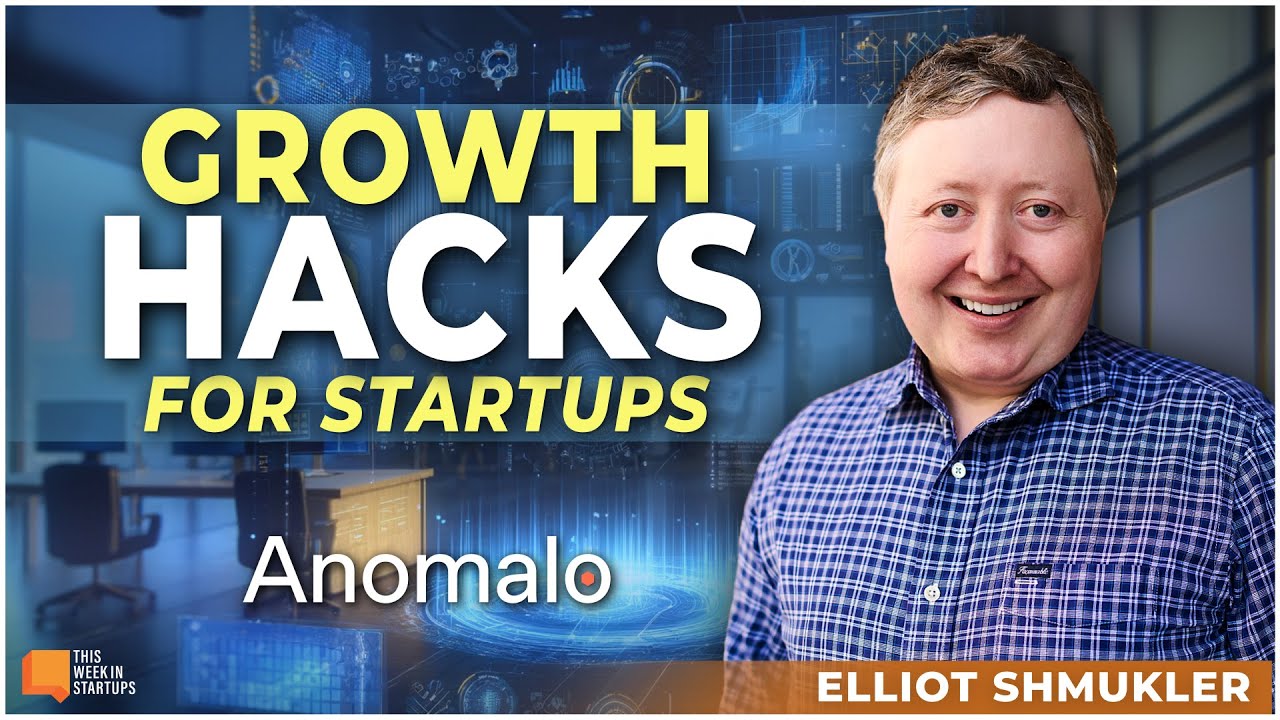
Growth Hacks for Startups from Elliot Shmukler of InstaCart, LinkedIn and now Anomalo | E1926

RCM Revenue Cycle Management Medical AI LLM Case Studies
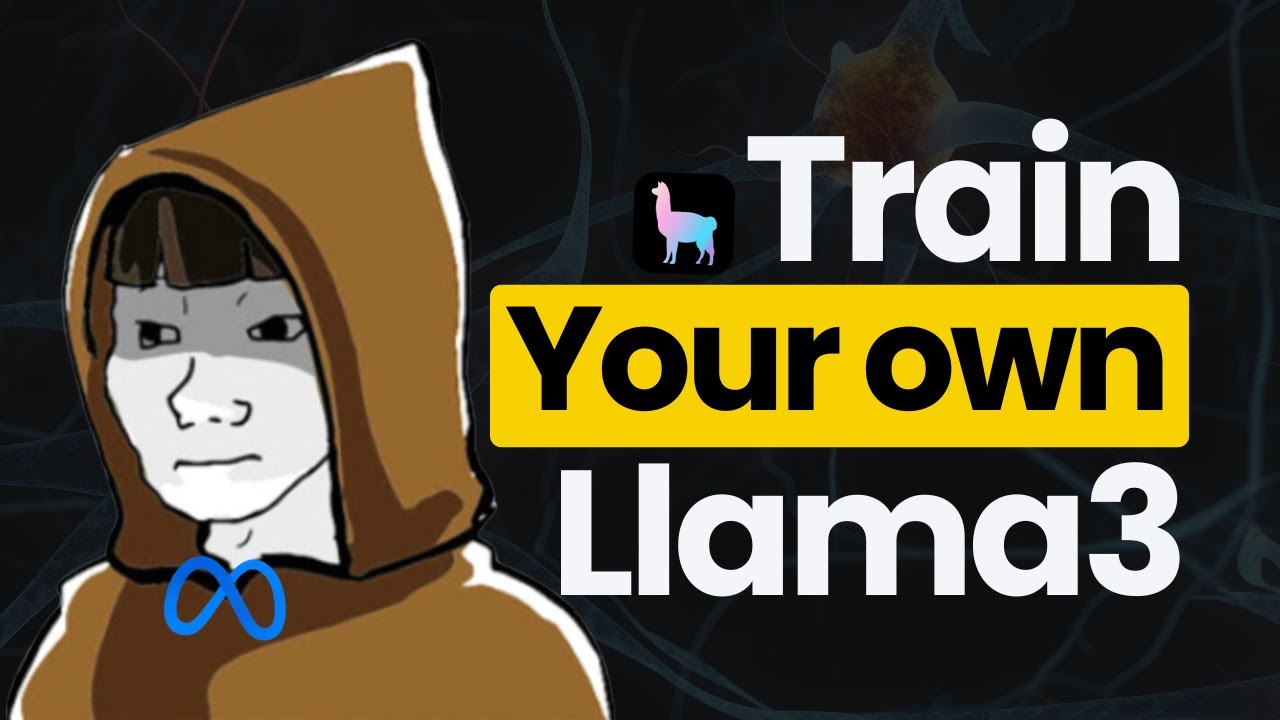
"I want Llama3 to perform 10x with my private knowledge" - Local Agentic RAG w/ llama3
5.0 / 5 (0 votes)