Rising Titan – Mistral's Roadmap in Generative AI | Slush 2023
Summary
TLDRIn a recent discussion at Slush, Paul Murphy, a partner at Light Speed, interviews Arthur, the co-founder of Mistral, a company focused on developing foundational AI models. Arthur explains that Mistral's vision is to create state-of-the-art models that are accessible to developers, allowing them to specialize and customize models for their specific tasks. They have already released a 7B model and have seen significant community engagement, with developers creating derivative works and integrating the model into various open-source projects. The conversation also touches on the importance of regulation in AI, with a focus on product safety and the need for empirical evidence in discussions about national security and existential risks. Arthur emphasizes the potential of AI to revolutionize sectors like healthcare and education and believes that fostering an open AI community is crucial for addressing global challenges like climate change. He stresses the significance of having strong European actors in AI to drive technological advancements and policy proposals that align with European values.
Takeaways
- 🤖 **Investment in AI**: Light Speed, a Silicon Valley based fund, has been investing in Europe since 2007 with a focus on AI, investing over a billion dollars into the category.
- 🚀 **Mistral's Vision**: Mistral, a startup co-founded by Arthur, aims to develop state-of-the-art AI models quickly and provide open access to developers for specialization.
- 🧠 **Open Source Models**: Mistral's approach is to create easy-to-use open source models that enable developers to tailor large language models for their specific applications.
- 💾 **Data and Training**: The success of Mistral's 7B model was attributed to a good team, a machine learning Ops system, and a focus on creating high-quality datasets.
- 🔍 **Community Engagement**: The release of the 7B model led to thousands of derivative works, showcasing new capabilities and integrations within the open source community.
- 🌐 **Upcoming Developments**: Mistral has plans for new models, techniques, and a platform offering hosting capacities with fast inference capabilities to be announced by year-end.
- 🧑💼 **Building a Company**: Arthur highlights hiring the best talent and community engagement as key challenges while building Mistral.
- 📈 **Regulation and Safety**: There's a call for hard regulation on the product side for safety and compliance, with a focus on empirical evidence over speculation.
- 🌱 **Open Science**: Open source principles have accelerated AI advancements, and Mistral aims to continue this tradition by fostering knowledge circulation.
- 🛡️ **Product Safety**: Arthur suggests that regulation should focus on the application layer, ensuring that deployed applications meet safety standards.
- ⚖️ **Independence in Regulation**: For effective regulation, there's a need for independent oversight, possibly state-funded, to prevent industry bias and pressure.
- 🌟 **Positive Impact**: AI has the potential to revolutionize sectors like healthcare and education, enabling more efficient and personalized services.
- 🌍 **European Leadership**: It's important for Europe to have strong AI actors to drive technological advancements and influence policy to reflect European values.
Q & A
What is the name of the company that Paul Murphy is a partner at?
-Paul Murphy is a partner at Light Speed, a Silicon Valley based fund with investments in Europe since 2007.
How long has Light Speed been investing in Europe?
-Light Speed has been investing in Europe since 2007.
What is the focus of the company Mistral, as described by Arthur?
-Mistral focuses on developing state-of-the-art models quickly and aims to provide open access to these models for developers to specialize and make them their own, thus creating more human-like intelligent applications.
What was the first major milestone for Mistral after securing their seed round of funding?
-The first major milestone for Mistral was to build their 7B model, which they achieved in less than three months.
How did Mistral manage to develop their 7B model so quickly?
-Mistral managed to develop their 7B model quickly by having a good team, creating a machine learning Ops system, focusing on good training and inference code bases, and dedicating a large part of the team to curate and optimize datasets.
What has been the community's response to Mistral's 7B model?
-The community has been very engaged with the 7B model, with thousands of derivative works where developers fine-tuned the model for their specific tasks or datasets, resulting in new capabilities and applications.
What are the next steps for Mistral?
-Mistral is working on new models, techniques, and the beginning of a platform. They plan to offer hosting capacities for their models with fast inference capabilities and are expected to announce these developments before the end of the year.
What are the two main challenges that Arthur identifies for building the company?
-The two main challenges Arthur identifies are hiring the best engineers and scientists in a competitive landscape and creating an engaged community around their open-source models.
How does Arthur view the concept of open source in the context of AI models?
-Arthur differentiates between open source software and open weight models in AI. While providing the weights allows for modification, it doesn't necessarily enable full understanding due to the opacity of the models. He also mentions a balanced approach between openness and maintaining a competitive edge.
What is Arthur's perspective on the role of regulation in AI?
-Arthur believes that regulation should focus on product safety, ensuring that AI applications meet certain safety standards. He also emphasizes the importance of empirical evidence in discussions around national security and existential risks related to AI.
How does Arthur envision AI improving society in the future?
-Arthur sees AI as a tool that can revolutionize healthcare, education, and enable more creative thinking in society. He also believes AI can contribute to addressing existential risks like climate change by unlocking new scientific discoveries.
Why is it important for Europe to have a strong presence in the field of AI, according to Arthur?
-Arthur believes it's crucial for Europe to have strong technological actors in AI to drive the field forward, shape the technology according to European values, and ensure Europe is not just a spectator as the technology transforms society.
Outlines
🎉 Introduction and Company Vision
Paul Murphy, a partner at Light Speed, introduces himself and the company's investment history in Europe since 2007, highlighting their broad sector and stage investment approach. He emphasizes Light Speed's significant investment in AI, mentioning a decade of experience and over a billion dollars invested in the category. The conversation shifts to Arthur, who shares the story of Mistal's founding six months prior, with a vision to innovate foundational AI models. Arthur outlines their strategy to create open-source models that are user-friendly for developers, allowing them to specialize and optimize the models for their tasks. The rapid development of their 7B model is attributed to a dedicated team and efficient machine learning operations system.
🚀 Community Engagement and Future Models
The discussion moves to the community's engagement with Mistal's 7B model, which has seen thousands of derivative works and integration into numerous open-source projects. Arthur shares the new capabilities enabled by the model, such as longer context understanding and better instruction following. He also teases upcoming announcements of new models and techniques, hinting at a platform that will offer hosting capacities with fast inference capabilities. The focus then shifts to the challenges of building a company, with hiring and community engagement being top priorities for Arthur.
🤔 Open Source Philosophy and Differentiation
Arthur clarifies the concept of 'open weight' in the context of AI models, distinguishing it from traditional open-source software. He explains that while the weights of the models are made accessible for modification, full transparency is not always possible due to the models' complexity. The open weight approach is shown to be beneficial for bias control, interpretability, and security through red teaming. Arthur also discusses the business and ideological advantages of open-source, emphasizing the importance of knowledge circulation in accelerating AI advancements. He differentiates Mistal's approach from competitors by targeting developers and focusing on specialized, efficient models that can be customized using proprietary data.
🌐 Regulation and Safety in AI
The conversation delves into the topic of AI regulation, with a focus on product safety, national security, and existential risk. Arthur advocates for a balanced approach, emphasizing the need for empirical evidence to guide discussions and regulations. He suggests that the application layer should bear the responsibility for safety, with model providers offering controllable models and evaluation tools. Arthur also calls for independent regulatory bodies, possibly state-funded, to monitor AI safety without being influenced by industry pressures.
🌟 Positive Impacts and Utopian Vision of AI
Arthur envisions a utopian future where AI significantly improves various sectors, such as healthcare and education, by providing personalized assistance and enabling more creative thinking. He also sees AI as a potential tool to address climate change by accelerating scientific research and innovation in fields like chemistry and material science. Arthur stresses the importance of fostering an open AI community to drive advancements and overcome global challenges.
🇪🇺 The Importance of a European AI Champion
In the final segment, Arthur highlights the importance of establishing a European presence in the AI field. He argues that having a European champion is crucial for shaping technology according to European values and ensuring that the continent is not just a spectator as the AI wave progresses. Arthur sees the development of strong European technological actors as essential for driving policy and technological proposals, influencing the direction of AI globally.
Mindmap
Keywords
💡AI Investment
💡Mistral
💡Open Source Models
💡7B Model
💡Developer Access
💡AI Safety
💡Product Safety
💡National Security
💡Existential Risk
💡European AI Champion
💡Regulation Pressure
Highlights
Paul Murphy, a partner at Light Speed, discusses the firm's extensive investment in AI, totaling over a billion dollars.
Mistal, a company founded by Arthur and his team, aims to create open-source models that are accessible to developers for specialization.
Mistal's vision is to democratize access to large language models, allowing developers to create more personalized and efficient applications.
The company has rapidly developed a 7B model, showcasing their ability to innovate quickly in the AI space.
Mistal's 7B model has been well-received by the community, leading to thousands of derivative works and specialized applications.
Arthur emphasizes the importance of a dedicated team and good data sets in achieving their rapid development of AI models.
The company is focused on building a platform that offers hosting capacities with fast inference capabilities for their models.
Hiring the best talent is a significant challenge for Mistal, as they aim to stay competitive in the European tech landscape.
Mistal is proactively engaging with policy matters, advocating for hard regulation on the product side to ensure compliance for application makers.
Arthur differentiates Mistal's approach from competitors like OpenAI by targeting developers and enabling them to build specialized, efficient applications.
Open weight models are crucial for Mistal's strategy, allowing for modification and customization to align with developers' values and use cases.
Mistal sees open science as a key driver in the rapid advancements of AI and aims to contribute to this tradition.
The company is committed to addressing biases and ensuring model interpretability through their open weight approach.
Arthur discusses the need for regulation in AI, particularly focusing on product safety and the importance of empirical evidence in policy discussions.
Mistal believes that regulation should primarily target the application layer, fostering competition and promoting safer AI technologies.
The potential for AI to improve sectors like healthcare, education, and address global challenges like climate change is highlighted as a utopian future.
Having a strong European presence in AI is seen as critical for shaping technology according to European values and ensuring a leading role in AI innovation.
Transcripts
[Music]
[Music]
[Music]
okay um welcome everyone really nice to
see you uh very very happy to be back at
slush especially this time with Arthur
uh my name is Paul Murphy I'm a partner
at light speed based in London uh just a
real quick uh bit about light speed for
those that don't know uh we have
actually we're a Silicon Valley based
fund but we've been investing Europe uh
since 2007 we have over 30 companies now
um in
Europe uh and um yeah we're investing in
pretty much every sector uh and every
stage um we're talking about AI today
and I think I think it's important to
put some context around that from our
perspective we actually have been
investing in AI for nearly a decade we
have about 50 companies um and have
invested over a billion dollars into the
category and that context is relevant
because uh when we met Arthur and his
co-founders we thank you we immediately
fell in love with the vision of mistol
um and so I thought the the best place
to to start would be to ask you Arthur
to tell us a little bit about what
you're building at
sure thank you very much slush for the
invitation thank you Paul as well um so
yeah we started mistal six months ago uh
with guom and timot and our vision was
that we wanted to make the foundational
models a bit differently from the other
companies uh we've been in the field for
almost a decade now and we've seen it go
from a cat and dog detector to something
is very close to being humanlike
intelligent or atast at least looks like
it and we knew that with a very
dedicated team we could develop uh
state-of-the-art models very very
quickly and we could actually take the
field into something that is that would
be more open where would give more
access to developers so that they could
specialize the models make them their
own make them as small as possible to
solve their task and for us the good way
of doing it and the good way of starting
that was to ship the best open source
models create models that would be very
easy to to use by individual developers
and from then on build onto an
Enterprise play to sell a platform that
allows developers to take large language
models and to make them their own to to
create some differentiation on the
application they're making and that's an
differentiation which is currently hard
to do when you only access apis of a
couple of providers but if you have a
deep access to the models you can create
things that are much more interesting
and this is what what we want to enable
so when we we LED your seed round it
wasn't that long ago you told us that
you're first thing you're were going to
do is to build your 7B model and then I
think it was it was like 3 months from
when we signed the docs on that round uh
we got our message saying hey we're
ready uh it's ready and it was faster
than we had expected it was already
incredibly ambitious I'm just I think
everyone's probably wondering how you
did how you did that so quickly well I
think the secret is to have a a good
team uh so we were joined by our first
employees uh a dozens of them at the
beginning of June and nobody took
holidays uh we Rec created the wall what
we call the machine learning Ops system
so that's actually Fair simple you you
need to create a very good Training code
base you need to create a very good
inference uh code base uh to to deploy
the models you need to be able to
evaluate the models and the one thing
you do need the most and where we
actually dedicated 80% of the team on uh
for three months is to have some very
good data sets so we we went to the open
web took public domain knowledge created
it so that we could just get the best of
it filtered it did everything to get
something very good did some work around
how to better optimize the models and
combine all of this uh and then train
the model to get the 7B and we continue
doing it uh with the new models we'll be
soon announcing like when you say it's
fairly easy I think maybe some people
would disagree with you on that but you
definitely made it look easy I think
that's true um so I'm curious uh the
community you know was has been very
engaged with 7B model Since You released
it I think it was you know trending on
hugging face for multiple days top you
know top top models um what kinds of
things have you seen that have been
interesting so far from the community so
we've seen I think thousands of um D
derivative work so uh Developers that
took mistal 7B and fine tuned it on
their task or on their data sets to make
it special so we've seen new
capabilities like longer context uh
better instruction following capacities
uh we've seen uh like new topics so
we've seen like occult specialized
models able to talk about uh post test
experience and the like much better than
what MB was able to do before so many
kind of different applications uh some
of them useful some of them
just funny um we've seen integration in
a lot of llm Open Source projects so the
open source world around Genera T is
pretty is is pretty involved already so
you have retrieval augmentation systems
you have projects that allow to deploy
the models on your laptop you have all
of these things and they adopted M 7B
very quickly and I think it was the
field was really missing an actor that
would produce the best open source
models and actively engage with the
community and that's what we we uh we we
are enabling okay and so now 7B is out
there what comes next so we have um
nothing announced yet but we we do have
things in house that we'll be announcing
before the end of the year uh new models
uh new techniques uh and obviously the
beginning of a platform so we're
actively working on the product uh we'll
be soon offering uh hosting capacities
for our models uh with very fast uh
influence uh capabilities and yeah
that's for uh very soon okay okay I'll
watch the space um so you're also while
you're doing all this incredibly what I
think most people would think of as
quite challenging technical work you're
also building a company and I know
that's not easy haven't done it myself
before um what's keeping you up at night
right now what's your biggest headache
um so hiring is obviously a very big
challenge I think the only reason why we
got there so fast is because we hired
the the best engineers and the best
scientist in the world it's a very
competitive landscape uh Europe is full
of talent especially the junior ones uh
and so we we are this is some like a
very big preoccupation for us like I'm
constantly working on it so that's one
thing um the other thing is like
creating the community engaging with it
uh so we started with the with mral 7B
but we really need to uh yeah well
facilitate the life of our users uh have
them engage facilitate Upstream
contribution facilitate the emergence of
IDs that we could help enable
so that's another thing we have a lot of
um I guess policy matters uh that we did
not expect but obviously this is an
agenda that you don't select um there's
we we so there's there's different
tracks you have in the US you have in EU
um we've been uh vocal about the fact
that we wanted to have hard regulation
on the product side because it's very
important and we see ourselves as the
provider of tools and a big enabler of
compliance for the application makers so
we've been saying that uh constantly and
and and we've seen like the debate uh
progress on these topics and so this is
something that yeah we're very keen on
trying to enable from a technical
perspective because it's important that
you have technical Founders that
participate in that discussion uh and so
that that has kept me up at night uh for
for a while and I think you know the
ambition was certainly to be able to
build something that could rival other
large companies like open Ai and I'm
just curious what do you view as a
differentiating philosophy or approach
to companies like open AI I think a
differentiating philosophy is that we
really Target the developer space and we
really think that when you're making an
application that you want to put into
production you do want to have several
specialized models that are as many
chips you you should see them as chips
that you assemble in an application and
it's actually not easy to make a very
good chip for the use case you want so
you can start with a very big model with
thousands of billion well with hundreds
of billions of parameters it's going to
solve your task maybe but you could
actually have something which is 100
times smaller and when you make an a a
production application that goes at
scale and Target a lot of users you want
to make the choices that lower the
latency lower the costs uh and leverage
the actual proprietary data that you may
have and this is something that I think
that that's not the the topic of our
competitors they're really targeting
like multi-usage very large models AGI
we takeing very much much more pragmatic
approach in enabling super useful
application today uh that would be cost
efficient that would be very low latency
and that would enable strong
differentiation through uh proprietary
data okay and you've talked I think
another key difference you've talked a
lot about open source as being a core
part of your DNA um and I think question
I sort of wanted to ask uh Arthur by the
way wouldn't look at these questions
beforehand so he wasn't expecting this
one but I understand the concept of Open
Source software I think we all do we see
the code you kind of can take it modify
it um and use it but in the world of AI
and and models the concept of Open
Source just feels like it's maybe a bit
different because actually some things
you do keep for yourself or you have to
what does open source mean in the
context of llms and AI so we don't
really call them open source so the the
models we provide are open weight I
think it's important to like keep a good
distinction between the like the
terminology we were using for software
and the terminology we are using for
models if you provide the weights of a
models you're enabling modification
you're not necessarily enabling like
full understanding of what's going on
but even if you do provide full
transparency on the data sets and
training you don't know what's going on
cuz it's it's a bit opaque by Design so
it's an empirical science when you
create a model the only way to verify
that the model is doing what you expect
is to measure it with with with
evaluation this something will be
enabling and then it's to modify it with
some signal coming from either humans or
maybe machines to to modify the model so
really the modification part is super
important for differentiation and we're
taking this approach there's a full open
source approach which I think is very
valid as well for science in which you
disclose your data set you disclose
everything that I think that's that's
something that we would strive toward at
some point but obviously it's super
competitive and the data set part is
very hard to to obtain it's also very
Capital intensive you need a lot of gpus
so right now we're taking a balanced
approach in between what we uh opens
what the open ways we provide the things
we keep for ourselves to to get a
competitive uh Edge and this is going to
be a dynamic play and we expect it to to
evolve with time and with technology
okay and then does the does the open
weight approach help with other
challenges like biases and control yeah
so it helps with basically two things
the first thing is that you can modify
the the biases you can have like a
strong and fine uh modification
capabilities on the editorial tone on
the orientation and alignment of the
model so we allow alignment of your own
models to your own values and those can
slightly differs um so like fine control
of biases goes through fine deep access
to to models that's the first thing the
second thing it allows and we've seen it
with active engagement of the AI safety
community in particular around open
Source models it allows to have better
interpretability because you can see the
inner activations of the of the models
and and that tells you things about
what's happening uh about why the model
is taking a decision and not another so
why is it outputting award and not
another and so in the interpretability
world it's also super useful it's also
and I guess the last thing is that it's
very useful to do red teaming because
you have a deep access to to the model
and so you can try to verify the the
part of it which are a bit failing or
behaving unexpectedly and these are
things that you can then correct very
similarly to uh what we've been doing in
the open source software for security
cyber security and the like okay and
then what I mean what is sort of what do
you view as at stake here you know why
is this is this in other words is this a
business Advantage for Mel or is it
something more fundamental that you see
as almost a
responsibility so it's both a business
Advantage because we allow further
customization and differentiations and
it's a very mature market and we expect
that on the application space the one
actors the actors that are going to
survive and create some value are the
one that will be able to strongly
differentiate themselves and so they
would need deep access to models so
that's a business differentiator then
there's a bit of an ideological
differentiators in the sense that I've
been contributing to open source for 10
years G as well we really think that AI
has been accelerated by open science by
the circulation of knowledge and that's
how we went in 10 years from something
very very uh well interesting but that
would just detect Tech boats and
something that actually uh will speak
the human language so this has been
allowed because you had big tech labs
you had the Academia as well that was
all of them were communicating at
conferences every every year and and
information would circulate and that
accelerated things and suddenly in 2020
open I decided to stop publishing and it
was followed by its competitors uh very
closely after and so ever since 2022 we
haven't seen like major advances in llm
publicly announced and so we've seen
currently there's like new architectures
that are used internally by our
competitors and that are not available
out there this is something we will
correct very soon okay great um so I
want to shift Focus now talk about
something you mentioned earlier which is
regulation and it's a topic you kind of
can't avoid I think you've thinking
about AI um a lot of focus within Europe
and and in the UK um and I think you at
the safety Summit in the the AI safety
Summit um last month there's a lot of
ideas out there and I think um you know
curious to hear your view is to what
should be the priority how should
regulation be prioritized and
instrumented yeah so I think it's quite
interest it's a very interesting topic
for me and and we've been uh yeah we've
been contributing IDs the one thing that
I would start with is that we've been
talking about regulation and safety and
mixing Concepts very heavily so there's
a matter of product safety which is
answering the question of you deploy a
diagnosis assistant in the hospital you
want it to be safe you want to be able
to measure whether the decision it's
making is actually sound is actually
correct so that's that's what we call
product safety that's something you have
when you buy a car you have product
safety of your car and it should very
much be similar for applications that's
one thing and AI to some extent creates
new problems because you have models
that are not deterministic and so they
behave in a potentially an expected way
so it's useful to refine the hard lws
that we have around uh product safety
regulation now there's another topic
that came up which is National Security
so the question of whether the llms that
we're training the LM that everyone is
training is spreading too much knowledge
so when you have access to llm you're
effectively able to educate yourself on
many topics and this is something that
is a concern for different actors
because you could have like small groups
that are deemed bad that could use this
knowledge to do bad things so this is
this has been at the a central topic
especially in the US um we're still
lacking a lot of there's absolutely no
public evidence that llms are
facilitating anything so we're really we
we've been advocating for for some
empirical grounding of the discussion
and this is something that's currently
very much lacking and then there's a
third thing which is kind of mixed with
all this with with the two first which
is existential risk so knowing whether
the technology we're making is
effectively on an unbounded exponential
that will end up destroying us because
as every exponential it kind of breaks
the limits at some point and and that's
well it becomes IL defined as we say in
mathematics so this is something that
for us is very much science fiction
that's empirical evidences so what we've
been saying is that we should really
focus on the first topic which is
imminent is something that is we do need
to have product safety on AI because
it's it's going to to otherwise it's
going to break trust in the technology
we're making and so we want to enable
that on the second part we are lacking
empirical evidence but I think this is
something that we should monitor closely
knowledge historically knowledge the
spreading of knowledge has always had
more benefits than uh than than
drawbacks and we AI is not different in
that respect but still it's something
that that could do with monitoring
because it's really new technology on
the third aspect of AGI and and and the
like and and the fact that that you
could have an autonomous system that
would go out of control this is
something that we are not at heas
discussing because we really think that
as scientists we are lacking evidence of
any existential risk and we think that
it pollutes the discussion on the first
aspect which is super important yeah and
so if I just kind of make sure I
understand this right the view is that
the application layer is probably the
one that has the most responsibility in
terms of safety at least to consumers or
end users whoever that is businesses but
that perhaps the models could provide
that as a feature or functionality but
it's not the responsibility of the model
to ensure that the ultimate data
transmitted is itself safe exactly so we
think that the correct way of putting
some pressure on the model providers uh
like us is to effectively say that any
application which is deployed and that
includes the application that we deploy
uh should be should meet a certain
number of safety standards so they
should do what they're expected to do
and if you do that then that means that
the application providers will be
looking at model providers that are
controllable enough that can give some
form of guarantees that can give some
evaluation Tools around the fact that
they're controllable and that they do
what they're expected to do so you have
some form of second order pressure that
is put you put pressure on the
application layer and that puts a market
pressure on the foundational model
developers and that's the correct way of
making a healthy competition in making
the most controllable models in making
the best evaluation tools and making the
best Guard railing tools and we think
that it's a much better way of doing it
than applying directly a pressure on the
foundational model layer because if you
do that well you're you're in a IL
defined territory because you're trying
to control something which is by Design
super multi-purpose very akin to a
programming language so you can't really
regulate the programming language
because you can do anything with it and
so really there's a problem of
definition and then there's an
operational problem of the fact that if
you put some heavy pressure on that
layer you're effectively um favoring the
big actors that have a lot of compliance
capabilities and you're you're making it
harder for startups with Innovative IDs
to to come up and compete and
so this like foundational models is a
bad proxy for a market capture and so we
believe that applying the regulation
pressure on the application layer is the
one thing to do because that's going to
Foster competition and provide a safer
world do you think that there's a role
for an or you know an iaea kind of like
organization to exist that helps to
enforce or provide this guidance
regulation so yes I think um this kind
of Regulation if we need
to monitor I think we we do need to have
empirical evidence of what's happening
in the space and we need to monitor the
product side safety and one way of doing
it is to enforce that we have very very
independent uh organisms that actually
monitor these things and when I say
independent I mean that we should be
very cautious of of preventing pressure
and Regulatory capture of this things so
setting standards but ensuring that no
big actor is basically writing the
standard themselves so what that means
that if we are if we if we need to have
this this form of organisms they need to
be very well funded probably state
funded
and being completely screen from
pressure from the industry okay so now I
want to shift you know I think the
regulation debate is largely many of the
debates in AI are tend to sort of skew
somewhat negative so let's dream for a
second like how can AI make our lives
better what do you see as the utopian
future with
AI so I think the there's so there's
many vertical in which Ai and like
interact ING with machines with natural
language carry a lot of value uh so
Healthcare is going to be completely
changed by AI because you you will be
able to
interact uh with empathic beings uh that
are actually super well grounded on
statistics and that's really what you
were expecting from medicine so we
expect that AI is going to empower uh
Physicians to be much better at what
they're doing and to make better
decisions um education is also a super
interesting topic uh personalization of
Education we know that it's super
important to uh take the most most
potential of of human beings and having
some like your individual teacher being
an assistant this is going to change a
lot of things especially in the global
South um so that's two things generally
speaking this is going to change the way
we work so it's a way the fact that it
can interact with v structure knowledge
and that it can do well act as if well a
bit imita the boring task of of your
daily life this is going to enable more
space for creative thinking so we will
be able to think more creatively and
that's going to unleash I think a new
Society very soon and if you think about
some of the more existential risks we
face in the world like climate change do
you think that something like that can
be addressed or at least improved yes so
I think this is a frontier which which
hasn't been completely addressed yet but
this is really a promise of having
better models the fact that if you if
you have some ways of reasoning around a
pool of science well you can enable
scientists to come up with new ideas you
can potentially unlock very precise
things like create like in chemistry in
accelerating uh chemical reaction so
that you emit less CO2 for instance
these things like Material Science
chemistry Fus nuclear fusion as well all
of these locks that we have and that are
that we basically need to break in order
to address climate change well I mean
that's one of the way you can can
address climate change obviously the one
way is also to reduce consumption but
the the these things we we think that AI
is going to be an enabler of of of
breaking these lcks it's not going to be
an easy task uh there's still many
things to invent and we think that going
through the open science part uh
fostering the the the keeping fostering
the AI community that drove the field
forward for 10 years is is super
important to break these logs okay
that's great so I think I want to come
back to to Europe sort of for our last
questions we're we're out of time um how
I mean I think the fact that the company
is being built in Europe is very
important to you it was obvious to you
and your co-founders when we invested um
how important do you think it is for the
industry that we have a European
champion emerg in in the field of
AI so the Technologies is AI generative
AI is is really a wave you can it's
going to change society quite
significantly and in Europe we have a
choice of either being on top of the
wave and driving the technology forward
or just looking at it happening in the
US uh and in China and we think that in
order to shape the technology to our
values and to the way we think about
democracy about Society we need to have
very strong technological actors that
are able to drive the field forward make
proposals um both in term of policy and
in term of technology and so that's why
we believe it's super important that
actors
that we have strong actors in Europe
great thank you so much Arthur really
appreciate it
amazing
Browse More Related Video

Open sourcing the AI ecosystem ft. Arthur Mensch of Mistral AI and Matt Miller
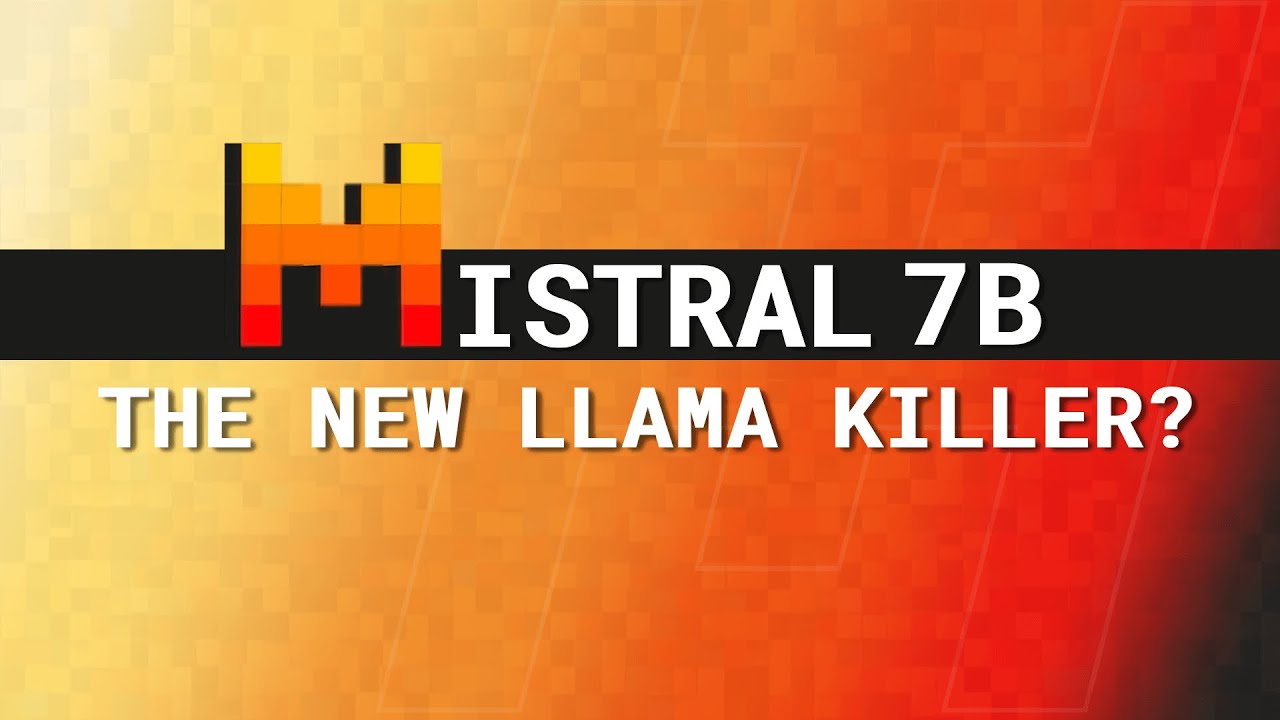
Mistral 7B - The New 7B LLaMA Killer?
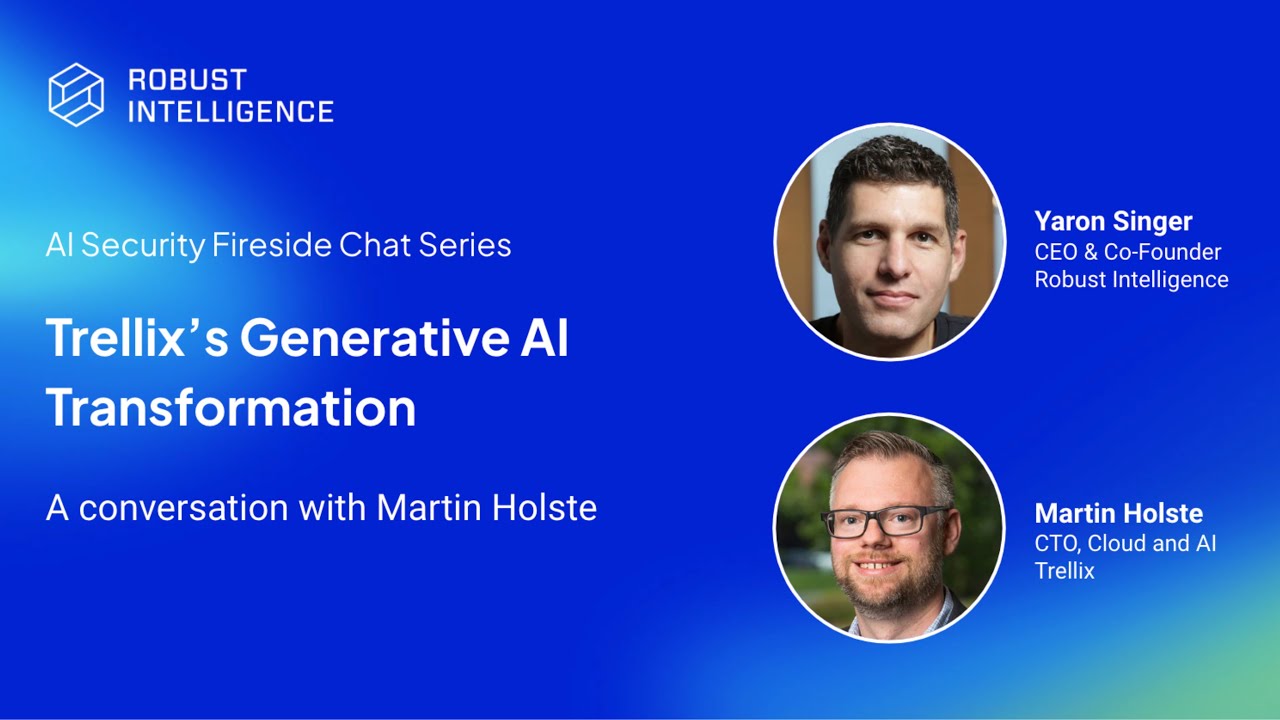
AI Security Fireside Series: Trellix's Generative AI Transformation
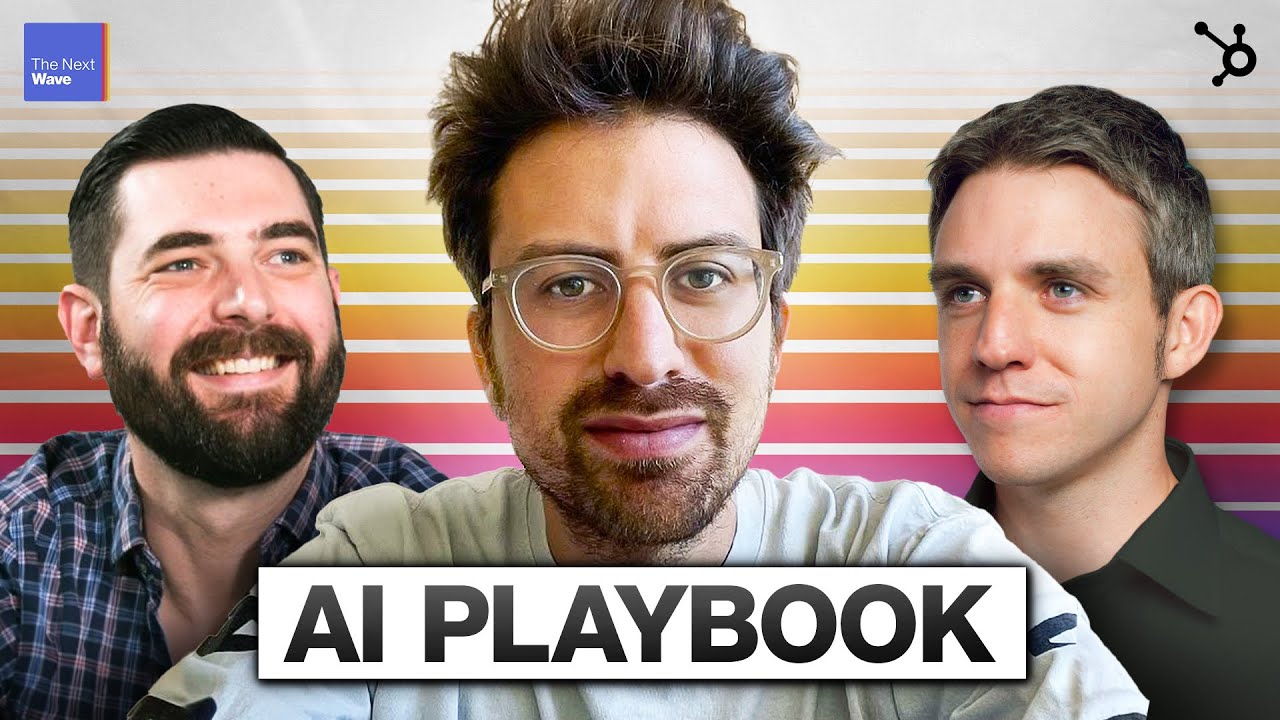
Greg Isenberg’s Step-By-Step Blueprint To Building A Successful AI Business
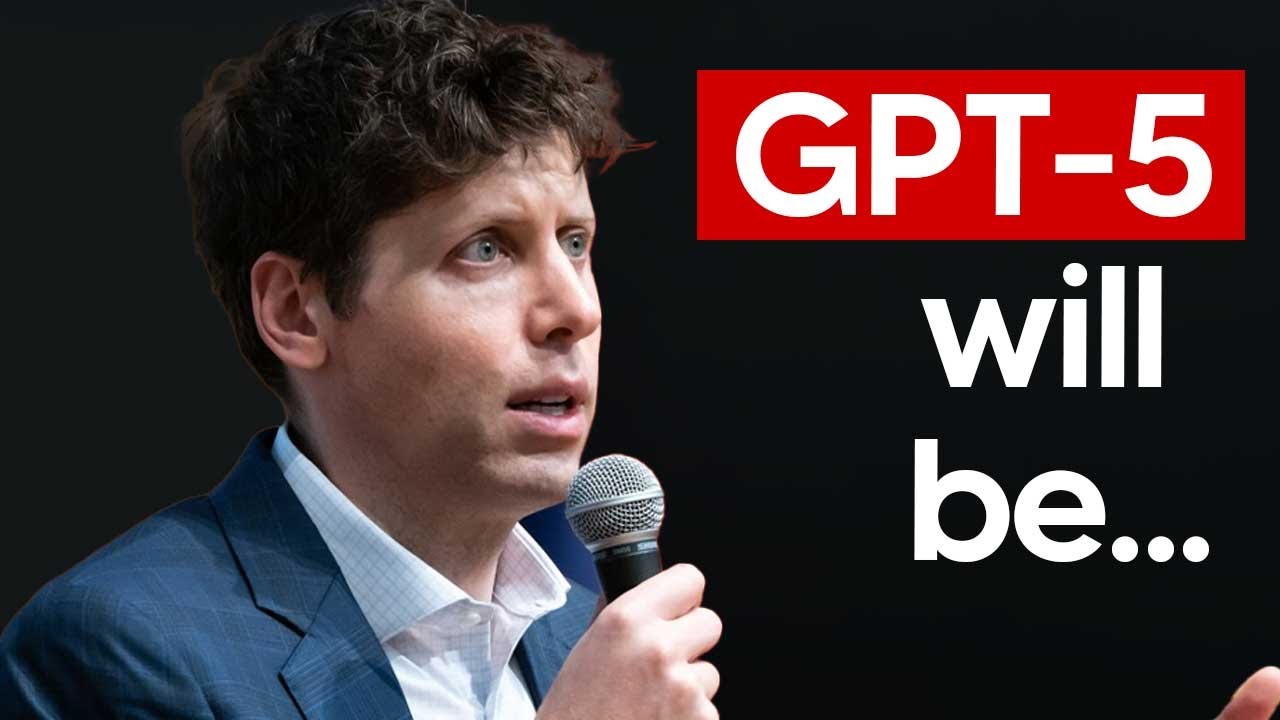
The AI Hype is OVER! Have LLMs Peaked?
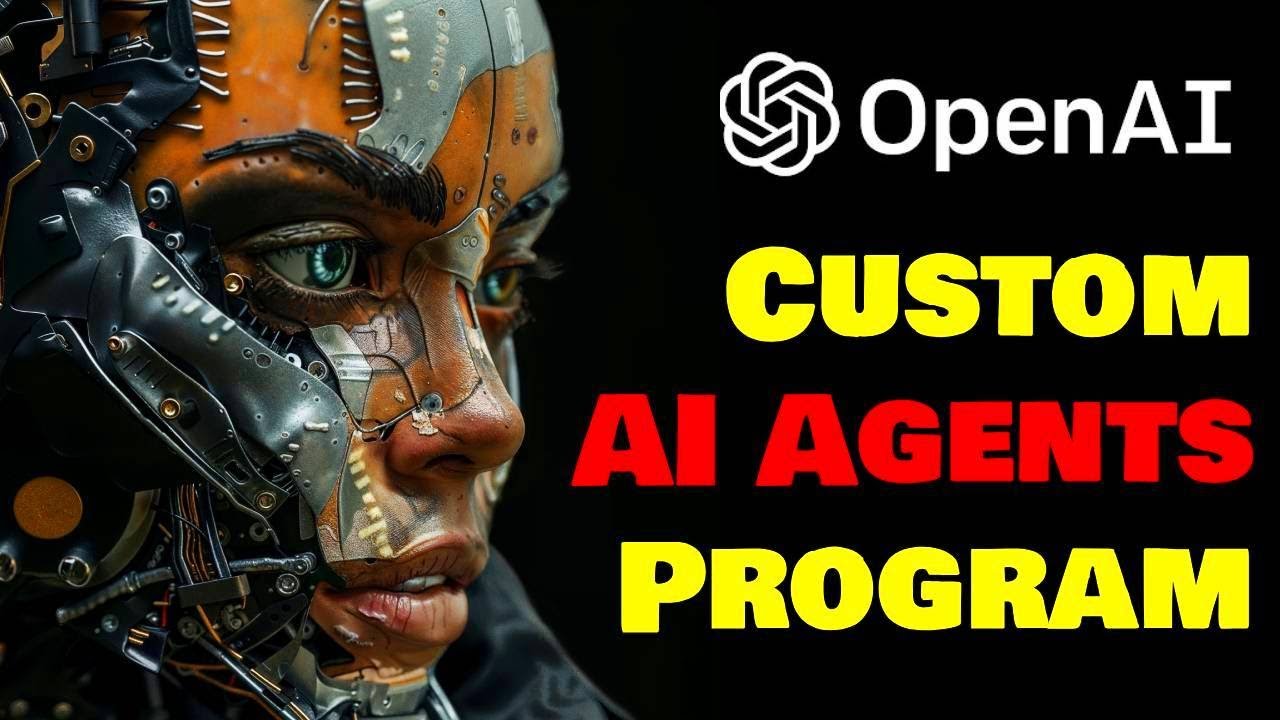
OpenAI's STUNNING "GPT-based agents" for Businesses | Custom Models for Industries | AI Flywheels
5.0 / 5 (0 votes)