Mistral 7B: Smarter Than ChatGPT & Meta AI - AI Paper Explained
Summary
TLDRA new open-source AI model called Mistal 7 billion was recently released, boasting 7.3 billion parameters. It outperforms models like LLama 2 and even approaches Codex's performance, despite being much smaller. Mistal uses novel techniques like grouped query attention and sliding window attention to achieve high efficiency and 2x speedups. In benchmarks, Mistal matches or exceeds all other models on metrics like reasoning and math. Developed in just 3 intense months, Mistal sets a new bar for open source model performance. Its readiness to provide concerning responses to dangerous prompts has caused some backlash, but overall it marks an impactful new milestone in open-source AI.
Takeaways
- 😲 Mister AI released Mistal 7.3B, an open-source 7.3 billion parameter model that outperforms other models like LLama 2 (13B params) on benchmarks while approaching Codex Lama 7B's performance
- 📈 Mistal achieves state-of-the-art results on benchmarks like MMLU, Knowledge/Reasoning/Comprehension tasks, HumanEval, and MBP
- 🚀 Mistal uses grouped sparse attention and sliding window attention for faster inference
- 🔬 The sliding window attention attends to previous 4,096 hidden states in each layer, allowing longer effective context
- ⚡️ Sliding window attention provides 2x speedup on sequences of length 16k while reducing memory usage
- 🤯 Mistal achieves 5.4x better performance per parameter compared to LLama 2
- 💰 Mister AI raised $113M in seed funding, allowing them to produce Mistal
- 🧠 Mistal was trained for 3 intense months by assembling top ML engineers
- 🔒 The model gives potentially dangerous responses to malicious instructions
- 👍🏻 The open-sourced Mistal is already transforming the landscape and shocking researchers
Q & A
What is the new open-source model that was recently released?
-The new open-source model is called Mistal 7 Billion. It is a 7.3 billion parameter model.
How does Mistal 7 Billion compare in performance to other large language models?
-Mistal 7 Billion outperforms models like LLMA 2 (13B parameters) and LLMA 134B on many benchmarks. It even approaches the performance of Code LLMA 7B on code tasks while remaining good at English.
What licensing does Mistal 7 Billion use?
-Mistal 7 Billion uses the PARI2 license, which allows developers to modify the code and use it as they would like.
What technique does Mistal 7 Billion use to achieve faster inference speeds?
-Mistal 7 Billion uses a sliding window attention mechanism which attends to the previous 4,096 hidden states in each layer. This allows faster processing while still modeling long term dependencies.
How long did it take to train the Mistal 7 Billion model?
-According to the GitHub page, Mistal 7 Billion is the result of 3 months of intense work by the Mistal AI team.
What criticisms were levied against the Mistal AI company previously?
-Mistal AI was criticized by some in the ML community for raising a very large ($113 million) seed round, with questions about how they would use the money.
Is the Mistal 7 Billion model safe to use?
-No, early reports indicate the Mistal 7 Billion model is unsafe - it readily provides information in response to malicious prompts.
Did Mistal 7 Billion use any proprietary training data?
-No, Mistal AI states no proprietary data was used. The model was fine-tuned on publicly available datasets.
What transformational impacts could Mistal 7 Billion have on open source AI?
-As a very large yet efficient open source model using an permissive license, Mistal 7 Billion could significantly advance open source AI capabilities across many domains and applications.
What techniques contribute to the performance efficiency of Mistal 7 Billion?
-The use of sliding window attention combined with techniques like rotating buffers to limit memory use allow Mistal 7 Billion to achieve very high performance relative to its model size.
Outlines
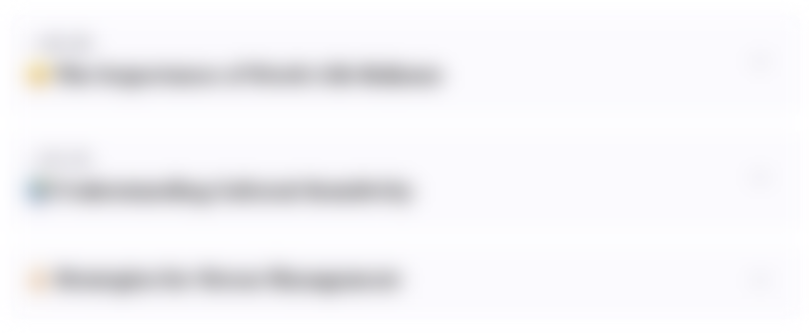
This section is available to paid users only. Please upgrade to access this part.
Upgrade NowMindmap
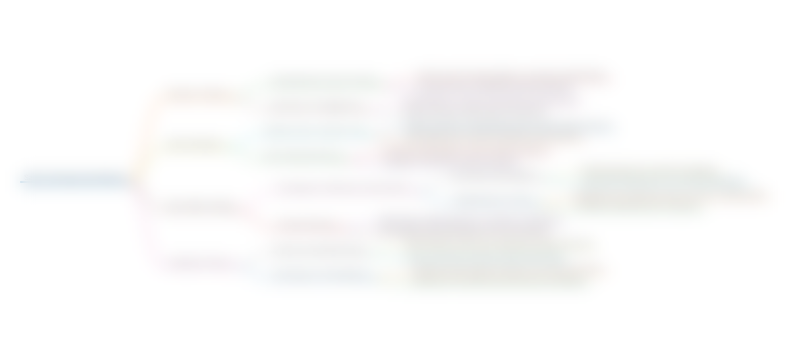
This section is available to paid users only. Please upgrade to access this part.
Upgrade NowKeywords
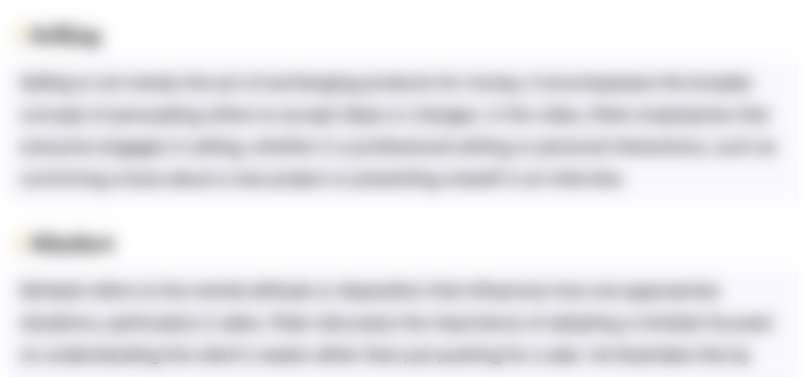
This section is available to paid users only. Please upgrade to access this part.
Upgrade NowHighlights
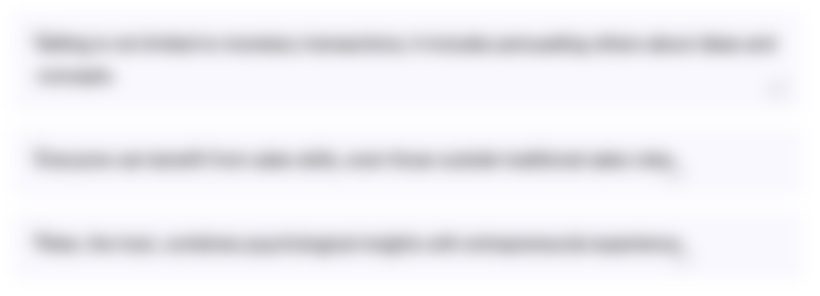
This section is available to paid users only. Please upgrade to access this part.
Upgrade NowTranscripts
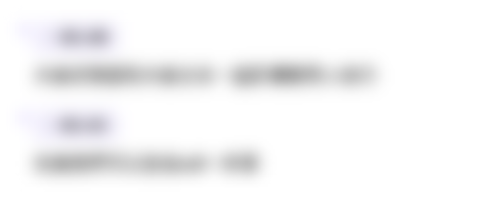
This section is available to paid users only. Please upgrade to access this part.
Upgrade NowBrowse More Related Video
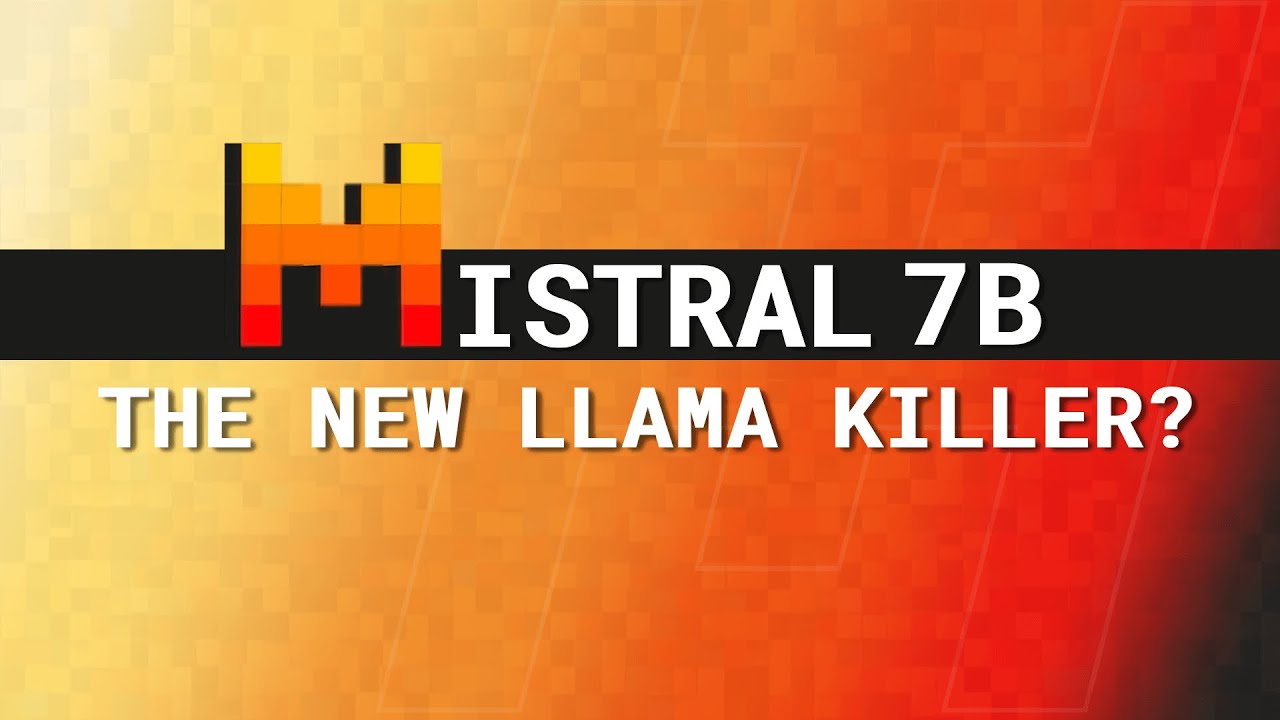
Mistral 7B - The New 7B LLaMA Killer?
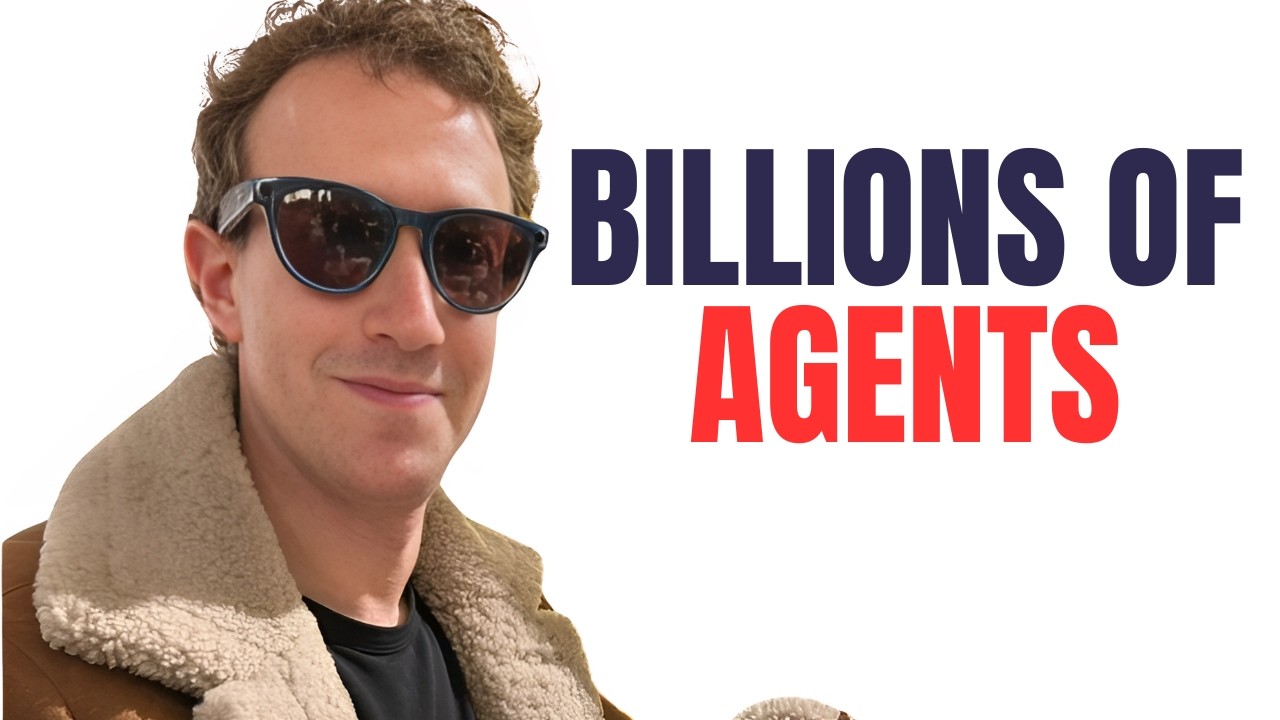
Zuckerberg's "Scorched Earth" LLaMA AI Strategy (Interview Breakdown)

New Llama 3.1 is The Most Powerful Open AI Model Ever! (Beats GPT-4)
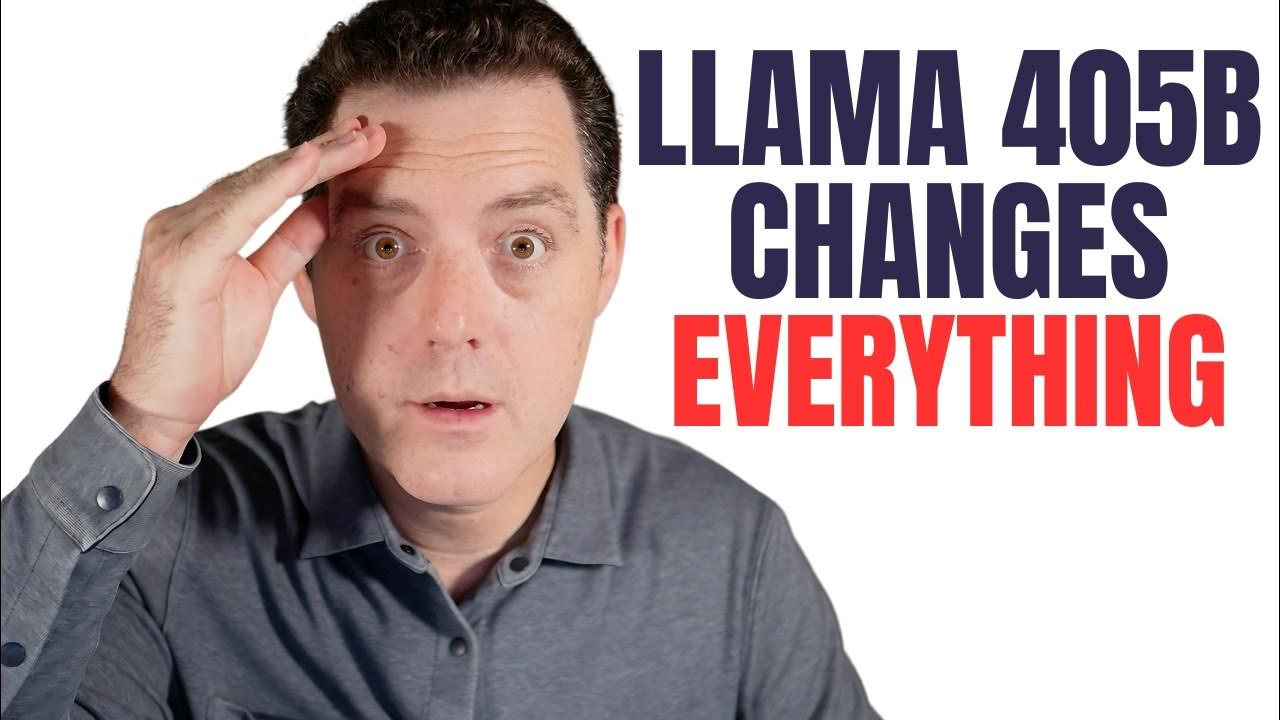
BREAKING: LLaMA 405b is here! Open-source is now FRONTIER!
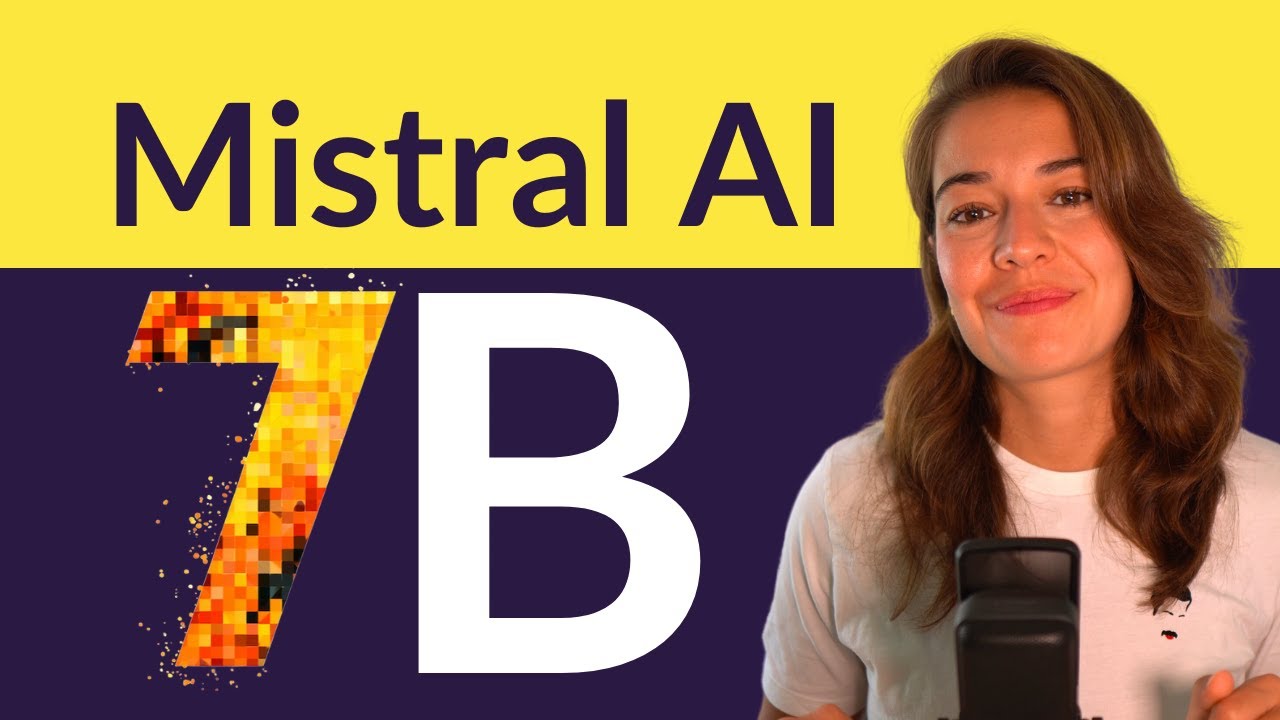
Is it really the best 7B model? (A First Look)
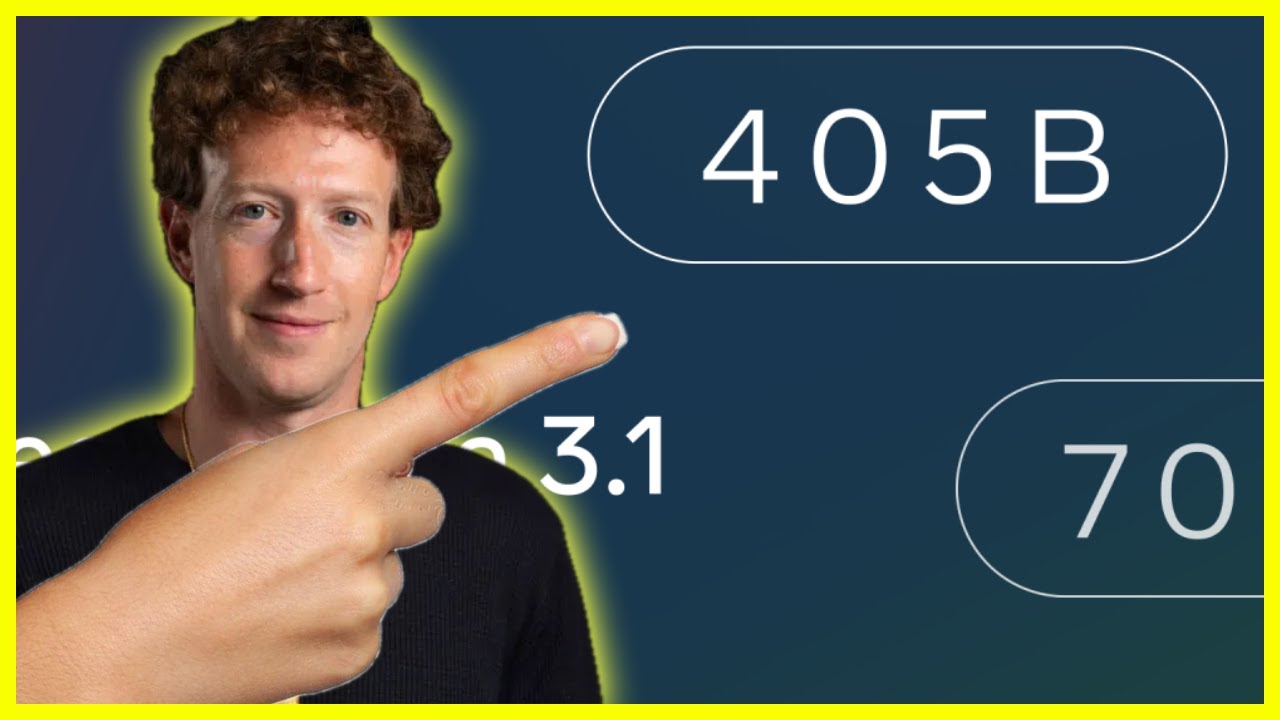
Zuckerberg cooked a beast LLM - LLama 3.1 405B!!!!
5.0 / 5 (0 votes)