特斯拉自动驾驶的“通用世界模型”和视频生成技术|Ashok23年CVPR主题演讲
Summary
TLDR特斯拉Autopilot团队的Asha Kisami介绍了他们的自动驾驶技术进展。目前,全自动驾驶Beta软件已在美国和加拿大的约400,000辆车上运行,行驶里程超过5亿英里。他们的自动驾驶堆栈主要基于八个摄像头,提供360度全方位覆盖,利用现代机器学习技术,尤其是神经网络,来处理转弯、交通灯和与其他物体的互动。他们还开发了一种基于占用网络的3D空间预测技术,以及预测未来行人和车辆流动的模型。特斯拉正在构建一个通用的世界模型,通过大量视频剪辑和先进的生成模型来训练,以实现更准确的未来预测。此外,特斯拉还在开发Dojo,一种定制的训练硬件,以支持这些基础模型的大量计算需求。
Takeaways
- 🚗 特斯拉全自动驾驶(FSD)软件已向美国和加拿大购买此服务的约400,000辆车辆推出,这些车辆已累计行驶约2.5亿英里。
- 📸 特斯拉FSD的核心是一个基于现代机器学习的系统,主要依赖车辆上的8个摄像头提供的360度全景视图,不同于传统的依赖本地化地图和雷达的自动驾驶技术。
- 🧠 特斯拉的自动驾驶技术将多个自驾驶组件集成到神经网络中,包括使用大型变压器模型进行空间和时间注意力的计算。
- 🛣️ 特斯拉开发了一种状态-of-the-art的生成模型,用于实时预测道路线和移动物体,这些预测不仅仅基于摄像头视频流,还包括车辆自身的运动信息和导航指令。
- 🔮 特斯拉正在开发一种更通用的世界模型,这个模型能够基于过去的数据和条件预测未来的状态,这可能会对自动驾驶技术产生重大影响。
- 🎓 该技术的成功依赖于特斯拉强大的自动标记系统,该系统可以处理来自全球范围内数百万视频片段的数据,以构建精确的3D场景重建和标签。
- 🚦 特斯拉的自动标记技术能够无需人工干预地准确标记交通灯、道路线等关键信息,极大地提高了数据处理效率和精度。
- 🌍 特斯拉的技术不仅限于汽车,还旨在跨越不同的机器人平台,展现了强大的通用性和适应性。
- 💻 为了支持这些先进模型的训练,特斯拉正在成为全球计算能力领先者,开发了名为Dojo的自定义训练硬件。
- 🤖 特斯拉强调其技术的核心是建立一套基础模型,这套模型能够理解世界上的各种复杂情况,并且这些模型将在接下来的12到18个月内进一步发展。
Q & A
特斯拉自动驾驶团队的核心研究方向是什么?
-特斯拉自动驾驶团队的核心研究方向是构建能够实现自动驾驶和机器人自主性的基础模型,这包括通过摄像头实现360度全方位覆盖的现代机器学习堆栈。
特斯拉FSD Beta软件已经覆盖了多少辆车辆?
-特斯拉FSD Beta软件已经覆盖了大约400,000辆车辆。
特斯拉自动驾驶技术与传统自动驾驶技术有什么不同?
-特斯拉自动驾驶技术主要依赖摄像头,而不是传统的定位、地图和雷达超声波等传感器,通过现代机器学习技术实现自动驾驶功能。
什么是占用网络,它在自动驾驶中扮演什么角色?
-占用网络是一种预测3D空间中某个体素是否被占用的模型,它可以代表任意场景,无需特定的标签或本体论设计,是特斯拉自动驾驶技术中的一个关键部分。
特斯拉如何处理车道的预测和表示?
-特斯拉使用最新的生成模型技术,如自回归变换器,以GPT类似的方式模型化车道,并将它们表示为向量,如多边形线、样条线或多项式,以便在实时中容易使用。
特斯拉是如何实现对移动对象的理解和预测的?
-特斯拉通过综合考虑摄像头视频流和其他输入(如自我运动学和导航指令)来实现对移动对象的全面理解和预测,包括对象的形状、未来运动等信息。
特斯拉的自动标记管道是如何工作的?
-特斯拉的自动标记管道通过汇总多辆特斯拉车辆上传的视频片段和其他数据,重建完整的3D场景,并在此基础上使用更多神经网络自动生成标签。
特斯拉如何处理紧急刹车情况?
-特斯拉系统可以自动检测潜在的撞车风险,如忽略停车标志的车辆或横穿车道的车辆,并自动刹车以避免碰撞。
特斯拉的未来世界模型将如何帮助自动驾驶技术?
-特斯拉正在开发一个能够基于过去的情况预测未来并模拟不同未来情景的神经网络世界模型,这将大大增强自动驾驶系统处理复杂场景和未知变量的能力。
特斯拉如何确保有足够的计算资源来训练其基础模型?
-特斯拉正在生产自定义的训练硬件Dojo,并计划成为全球计算平台的领导者,以确保有足够的计算资源来训练和实验其基础模型。
Outlines
🚗 自动驾驶的创新之路
Asho Kisami在视频中介绍了自己作为特斯拉自动驾驶团队的一员,展示了该团队在自动驾驶和机器人技术方面的最新工作。他们已经向美国和加拿大购买全自动驾驶Beta版的用户推出了软件,覆盖了大约400,000辆车,这些车已经驾驶了约2.5亿英里。这个系统主要依赖于车上的八个摄像头提供的360度视角,与传统的自动驾驶技术不同,这一系统更多地依赖于现代机器学习技术,而不是传统的定位、雷达或超声波传感器。他还介绍了占用网络(Occupancy Networks)作为系统中的一个核心部分,强调了它的通用性和对3D空间的理解能力。
🌐 处理道路不确定性的先进模型
在第二段中,Asho Kisami深入讨论了预测道路线(lanes)的挑战,特别是考虑到它们的不确定性和复杂性。他提到了使用最先进的生成模型技术,如自回归变换器,类似于GPT的方法来处理这些问题。这种方法能够以向量形式准确预测道路线,这对实时操作至关重要。此外,他也提到了如何处理移动对象,比如车辆和行人,并强调了整个系统采用现代机器学习堆栈的重要性,这允许端到端的感知处理,大大提高了效率和准确性。
🔮 构建未来的基础模型
第三段讲述了通过重建准确的3D场景并自动生成标签来提高自动驾驶系统精度的方法。Asho Kisami介绍了使用神经网络离线生成道路、交通灯等要素的向量表示,这些都是基于从特斯拉车队收集的大量视频片段。这种方法提供了对世界的深刻理解,并作为基础模型应用于自动驾驶和手动驾驶辅助中,如紧急刹车系统。他还强调了跨物体预测其将来动作的重要性,并分享了Tesla在自动紧急刹车领域的领先成就。
🌟 跨越新技术的边界
在最后一段中,Asho Kisami谈到了通过预测视频序列中的未来发展来构建一个更广泛应用的世界模型的可能性。他介绍了一个能够基于过去的行为预测未来的神经网络模型,这个模型能够理解和预测复杂场景的动态变化。这种模型不仅可以应用于自动驾驶汽车,还可以扩展到其他领域,如机器人技术。他强调了为了训练这些先进的模型,特斯拉正在成为世界领先的计算力提供者,特别提到了Dojo训练硬件的引入。Asho Kisami概述了这项技术如何帮助实现跨汽车和机器人平台的知识共享,预示了一个充满创新的未来。
Mindmap
Keywords
💡自动驾驶
💡基础模型
💡机器学习
💡神经网络
💡实时处理
💡自动驾驶堆栈
💡自动标记
💡轨迹校准
💡运动规划
💡计算平台
Highlights
特斯拉Autopilot团队的演讲,介绍了他们对于自动驾驶和机器人技术的基础模型研究。
特斯拉已经向美国和加拿大的购买者全面推送了全自动驾驶Beta软件。
大约有40万辆车辆在FSD Beta上行驶了高达5亿英里。
特斯拉的自动驾驶堆栈是可扩展的,可以在美国任何地方使用。
特斯拉的自动驾驶主要依赖车上的八个摄像头,提供360度全方位覆盖。
特斯拉的自动驾驶技术与传统的自动驾驶方法不同,主要基于机器学习和神经网络。
特斯拉使用占据网络(occupancy networks)作为其自动驾驶堆栈的重要组成部分。
占据网络能够预测3D空间中的体积是否被占据以及占据的概率。
特斯拉的自动驾驶技术能够实时预测车道流和未来行走路径。
特斯拉的自动驾驶架构虽然看起来复杂,但实际上并不那么复杂。
特斯拉使用最先进的生成建模技术来预测车道,类似于GPT。
特斯拉的自动驾驶系统能够实时预测移动物体的完整运动状态。
特斯拉通过整个车队的视频剪辑建立了复杂的自动标记流水线。
特斯拉的多视角重建技术能够精确对齐不同车辆的数据,重建整个3D场景。
特斯拉正在学习一个更通用的世界模型,能够代表任意事物。
特斯拉的神经网络能够基于过去的视频预测未来,并且可以行动条件化。
特斯拉的自动驾驶技术不仅适用于汽车,也适用于机器人。
特斯拉的Dojo训练硬件将开始生产,旨在成为世界领先的计算平台。
特斯拉的自动驾驶技术将在未来12到18个月内取得重大进展。
Transcripts
great thank you so much for the
introduction hi everyone my name is asho
kisami um I a cesla on the autopilot
team hopefully you're able to hear my
voice and see my screen and video and
things please let me know if that's not
the
case yeah today I would like to present
uh our work on what we think is going to
be the foundation model for uh autonomy
and
Robotics this is not just a work of
myself I'm representing a large team of
talented engineers in our
team let's get
started um our team has shipped the full
self-driving bet software to everyone
who has purchased it in the United
States and Canada um there roughly
400,000 vehicles and today they have
driven uh up to 250 m 50 million miles
uh on FSD
beta
uh I think the cool thing about this is
that this is a scalable s driving stack
that you can take the car anywhere to
the US turn it on put in a destination
and the car would attempt to navigate to
the destination you know handling all of
the uh turns shopping at traffic lights
interacting with other objects and all
of this is driven primarily by the eight
cameras that are on the car that are uh
giving a full 360 degree coverage around
the
car the reason that works well is
because our stack is based on you know
really modern machine learning based
stack where uh a lot of the components
of the self driving stack are just
folded into neur neural networks and I
would say this is different than the
more traditional approach to
self-driving which uses localization
Maps lot of Radar Radar Ultrasonics Etc
to fuse together instead this is
primarily being driven by just cameras
and you can if you have a test yourself
you can obviously by the car and
experience it otherwise just to take my
word or look at some videos but it works
uh quite well and we are in the process
of making it even
better I shared about these occupancy
networks that are one of the more
important pieces in our stack um I would
consider this as one of the foundational
model uh task because this is very
general this is a very general task and
doesn't have any specific onology
um or like at least robust to ontology
errors uh it really just predicts
whether some voel in 3D space is
occupied or not and the probability of
that and um and cans represent you know
arbitrary scenes there's no um labeling
or onology design required and it's
quite General and can apply like
anywhere in addition to just the
occupancy we also predict the flow of
walks in the future that kind of like
gives arbitrary motion as well um and
everything runs in real time this is
quite similar to Nerf in general uh but
unlike Nerf or like say multiv view
reconstruction which which is usually
done for a single scene uh we predict
the occupancy based on the eight cameras
in real time so the video streaming and
then we just predict for all space
around the car on whether this walk is
occupied or not as opposed to like doing
this post like offline post procing
step so architecture looks um you know
with a lot of it looks very complicated
but then it's actually not that
complicated in the end um videos from
multiple cameras streaming and you can
choose whatever backbone you want you
know R Nets uh whatever the latest
widths uh you can throw anything in
there and then everything comes together
in uh large Transformer block that does
sort of a spatial attention to build up
features and also does temporal
attention um with some like geometry
thrown in there to form some features
that that can then be upsampled uh into
the actual predictions it's it's quite
straightforward even though the diagram
looks a bit
complicated
in the same architecture and the
modeling can be used not just for
occupancy but for other tasks that are
needed for driving um obviously lanes
and roads are very important for driving
task but I'd say lanes are quite
obnoxious um to predict the reason is um
you know first of all L are higher
dimensional objects unlike um you know
definitely not like 1D or 2D like you
know High dimensional and then they have
like a graph structure um like objects
for the most part like say Vehicles
there self-contained they're just you
know local whereas Lanes can span the
entire road you can see multiple miles
of lanes in your view um and they can
fork and merge and cause all kinds of
trouble in the
modeling um they also have large
uncertainty sometimes you don't you
might not be able to like um view the
lanes because they're uded or it's
nighttime only part of the lane is
visible and it's not just that sometimes
even if everything is visible even
humans cannot agree on whether some
thing that you're looking at is two
lanes or one lane for instance so
there's a ton of uncertainty in um like
what are
lanes and then it's not sufficient to
just predict them as some kind of raster
it's very hard to use Downstream then so
it's better to predict them as some kind
of vector representation you know like
poly lines plines polinomial Etc to help
with use usee of use and all of this
needs to happen within tens of
milliseconds in real
time like I said it's like a very
difficult problem to predict lenses in
real time
in the real
world nonetheless we use
state-of-the-art generative modeling
techniques um in this case we use you
know Auto regressive Transformers quite
similar to I would say GPT uh in terms
of how we model the lanes so we can
predict the uh you know you can tokenize
the lenses and predict them one token at
a time um unlike language which is
mostly linear we have to you know
predict the full graph structure hence
we come back uh predict you know what
are the forking Point what is the
merging point Point Etc and everything
is done end to end using neural networks
with like little to no postprocessing
required after
this another important task for driving
is obviously moving objects you know
Vehicles drugs pedestrians what have you
and it's not sufficient to just detect
them you need to have their full
kinematic
State um and also predict their shape
information their Futures Etc all of
these models the models that I described
earlier even the lanes one and objects
one or in some ways multimodel models in
the sense that they take in not just
camera um video streams they also take
in other inputs such as um in this case
ego's own kinematics so os's um velocity
acceleration jerk Etc all goes in uh we
also provide in um the navigation
instructions to the lanes to kind of
guide us where to like which lane to use
Etc so everything is done within the
network that's why I say it's like a
modern machine learning stack where
instead of doing this in post proc in we
just try to combine everything and then
do perception sort of end to end so to
speak so here you can see predictions of
these models um the lanes that you see
here the vehicles that you see here are
all just predicted again the by these
networks without a lot of postprocessing
there's no tracking or anything like
that uh in in the things that you're
seeing here so overall you know I would
say that's quite stable the green um
spines that are coming out of these
vehicles are just their for C future
it's kind of like a standard task at
this point I would say uh but you know
it all works quite nicely and in real
time in the
car doesn't have to stop with just
perception to once we have all of these
percepts um you know like Lanes
occupancy objects and even few more like
traffic controls and other things you
can do the entire motion planning um
also using just a network um I hav't go
into too many details on like how we do
that but essentially you know it can
just be thought of as like one more task
instead of it being a separate
thing so how is all of this possible and
I think it's because we have built the
sophisticated Auto labeling pipeline
that gives us data from the entire fleet
uh you know millions of video clips
across the entire world uh can be tapped
on the left side what you're seeing is
um an example of multi Rec construction
where we choose some
location multiple Tesla vehicles driving
through the location upload their video
clips and other other like you know
vehle kinematic data to us we bring
everything together and reconstruct the
entire 3D scene um so the um SPL the
poly lines that you look you know there
the San colored one there's also a few
other colored ones those are all
different cars doing different trips uh
to the world and it's actually all well
very well aligned let me see if I can
play again yeah the pink line and the S
line that you see there those are like
different trips of different cars uh
driving around and everything is just
aligned very nicely um and this
multi-rip reconstruction has enabled us
to you know get all the lanes Road lines
um everything directly from the fleet in
the millions um anywhere on Earth
essentially once you have this like base
um structure of trajectories calibration
from all these cameras you can really do
a lot of cool things to reconstruct the
entire scene not sure the video plays
quite nicely but in my on on screen it
looks very smooth where you can see the
ground surface it's reconstructed quite
nicely um there's like no artifact such
as double vision or blurring things are
a crisp um look like geometric they are
correct um this is a hybrid approach to
Nerf and um General 3D
reconstruction sometimes in Nerf even
though the um rendered visuals might
look very nice the underlying geometry
might be very fuzzy and cloudy um so we
have a hybrid approach which works quite
nicely you can see here you know all the
barriers the vehicles even trucks Etc
are reconstructed pretty
accurately once we have these uh
reconstructions we then run even more
neural NWS just offline to produce the
labels that we want like I had men
mentioned earlier for lenses we need uh
some kind of vector representation to
make it very easy to use um so instead
of just using a raster directly take
rasters we have offer neural networks
that run on top of it and then produce
the vector representation that can be
then use as labels for the online
stack similar to the lanes like once you
have the lanes and the roads
reconstructed you can also Auto label
traffic lights here you're seeing
traffic lights autol labeled by our
system without any human inputs um and
these are all like multiv view
consistent me try to play again yeah U
we we can predict their shape color
relevancy uh you can see this white
traffic lights on the side they also
reproject correctly into all the camera
views and it's because we have this
really good autoing system that
calibrates everything jointly and it's
like Pixel Perfect in 3D
space so yeah all of these predictions
together know give us super SL
understanding um of the world from
cameras and um I would already try to
call this you know sort of a foundation
model that can be used in a lot of
different places um and these
predictions really help FSD um you know
drive in any place um like you don't
have to Geor restricted you can you know
even sometimes construct a new road turn
it on and it would work quite nicely
there in addition they also help manual
driving um obviously because you know uh
humans are not perfect
drivers so they need some help every now
and then in this case on the left side
the ego driver for some reason blew past
the stop sign and was almost about to
crash into this red car uh and our
system um you know DED this and then
break
automatically and similar on the right
side driving straight and then someone
just comes in then Cuts us off it's
quite dangerous but then the system app
the break quite early uh the reason why
this is different than you know what you
think that AB systems have been there
for since 1980s what is new about this I
think Tesla is the first company to ship
uh this emergency braking for crossing
vehicles to the best of my knowledge uh
and the reason that that Crossing
objects are harder unlike say vehicles
that are in your own lane is because um
for crossing objects you need to know
whether they're going to stop in time or
not um what is the stop line do they
have traffic lights uh and if they were
to turn which Lanes they turn into Etc
there's a ton of work that needs to
happen to understand where the in
objects would go and like likely to go
do they have you know room to stop Etc
it's it's not as simple as just
directing a vehicle and having their
velocities and things like
those yeah so like I said I think I I
believe Tesla is the first company to
ship Crossing ab and it's already in
customers hands in the last several
months but is the foundation model
really just you know a bunch of these
stats concatenated together or can there
be more to it we think there can be more
to it you know um these STS like in the
occupancy for example white while it's
quite General um some some things are
harder to represent even in that space
I'll probably go to more details
shortly so that's why we are working on
um learning a more General World model
that can really just represent uh
arbitrary things so in this case what we
do is we have a neural network that can
be conditioned on the past or other
things to predict the future and I mean
obviously everyone has wanted to work on
this forever and and I think with the
recent um rise in generative models like
you know Transformers diffusion Etc we
finally have a shorted it what you're
seeing here is purely generated video
sequences given the past videos the
network predicts some sample from the
future hopefully the most likely sample
and you can see that it is being
predicted not just for one camera but it
predicts the all the eight cameras
around the car uh jointly and see how
you know the car colors are consistent
across the cameras the motion of objects
is consistent in 3D even though we have
not explicitely asked it to do anything
in 3D or not even baked in any 3D priors
this is just the network understanding
depth and motion on its own without us
uh informing it of
so and since this is all just predicting
future you know RGB values the ontology
is quite General you can like throw any
video clip from driving or you know from
YouTube or from your own phone anything
can be used to uh train this General
Dynamics model of the
world additionally it can also be action
conditioned um show a few examples so
here on the left side the car is driving
in the lane and we're asking it to okay
just keep in this Lane and keep driving
and then you know like I said earlier
the car is able to or the model is able
to predict all of the geometry Flow by
uh very nicely and understands 3
on the right here we're asking to change
lenses to the right
side uh maybe we'll go back and fate
again so on the left it's just going
straight and we ask it to go straight
the model goes straight and then on the
right side we ask it to make a lane
change and it makes a lane change and
the past context is the same for both of
these um outputs so given the same past
and when we ask it for different Futures
then model is able to produce or like
imagine different futures
this is super powerful because you know
now you have essentially a neural
network simulator uh that can U simulate
different Futures based on different
actions and unlike a traditional game
simulator this is way more powerful
because uh it can you know
represent things that are very hard to
describe in an expit system um I'll show
a few more examples but then um is super
powerful and also the mo the intention
and then the natural behavior of other
objects such as Vehicles is very hard to
represent explicitly but in this world
it's very easy to
represent it doesn't have to stop with
just RGB you can obviously do this kind
of future prediction task not just RGB
but also in optic segmentation or you
can extend it to also 3D spaces where
you can imagine future 3D uh scenes
entirely based on just the past and then
your action prompting or even without
prompting You can predict different
Futures uh this is I personally I you
know I'm Amazed by how uh well this
works uh and it's very exciting future
uh that we are working on
here yeah here are some examples where I
think you know something like this is
going to be needed to represent um like
what's happening in the scene like
there's a lot of smoke coming in one of
the uh pictures like there's paper
flying everywhere you know that's going
to be tough for you know even um
occupancy where okay you have paper
flying everywhere there's occupancy
there's occupancy flow but then how do
you know it's paper what do you know the
material properties of it um there's
like smoke obviously you can drive
through it but you know it is occupying
space and light does not transmit
through um you know there's a lot of
nuances to driving and we have to really
solve all of these problems to build a
general driving stack that can drive
anywhere in the world and be humanlike
might be fast efficient uh at all speeds
yet very safe uh and I think you know we
are working on the right recipe for um
building this
obviously training all these models
takes a ton of compute and that's why
Tesla is aiming to become a world leader
uh in compute Dojo is our training
Hardware that we have custom built at
Tesla that is starting production next
month essentially uh and with that we
you know we we think uh we are on the
way to become one of the top um computer
Platforms in the entire world and we
also think that in order to train these
Foundation for vision we need a lot of
compute and not just train compute for
training this one model but compute to
try a lot of different experiments to
see which models actually work well uh
and that's why it's super exciting for
us to you know uh be in this spot where
computer is going to be abundant and
it's just going to be bed by ideas as of
Engineers and T is this is not just
being built for the car but also for the
robot um we already have the occupancy
networks for example and other few of
networks all shared between the car and
the robot and it actually works quite
well and generalizes across these
platforms and we want to extend it to
all the T all the tasks that we have um
even like lanes and vehicles for example
should not be specific to cars if the
robot say happens to walk to the road
and looks around it should understand
roads and vehicles and you know how
Vehicles move Etc all of this are just
be built for both the platforms and you
know any other future robotics platform
that would also need
this
that's basically it um we to summarize
again we're building this super cool
foundational models for vision that
really understands everything um and it
should generalize across cars and robots
it's going to be trained on tons of
diverse data from the fleet on tons of
compute um yeah so I'm really excited
for the next um you know 12 to 18 months
of what's going to happen uh here thank
you
Browse More Related Video
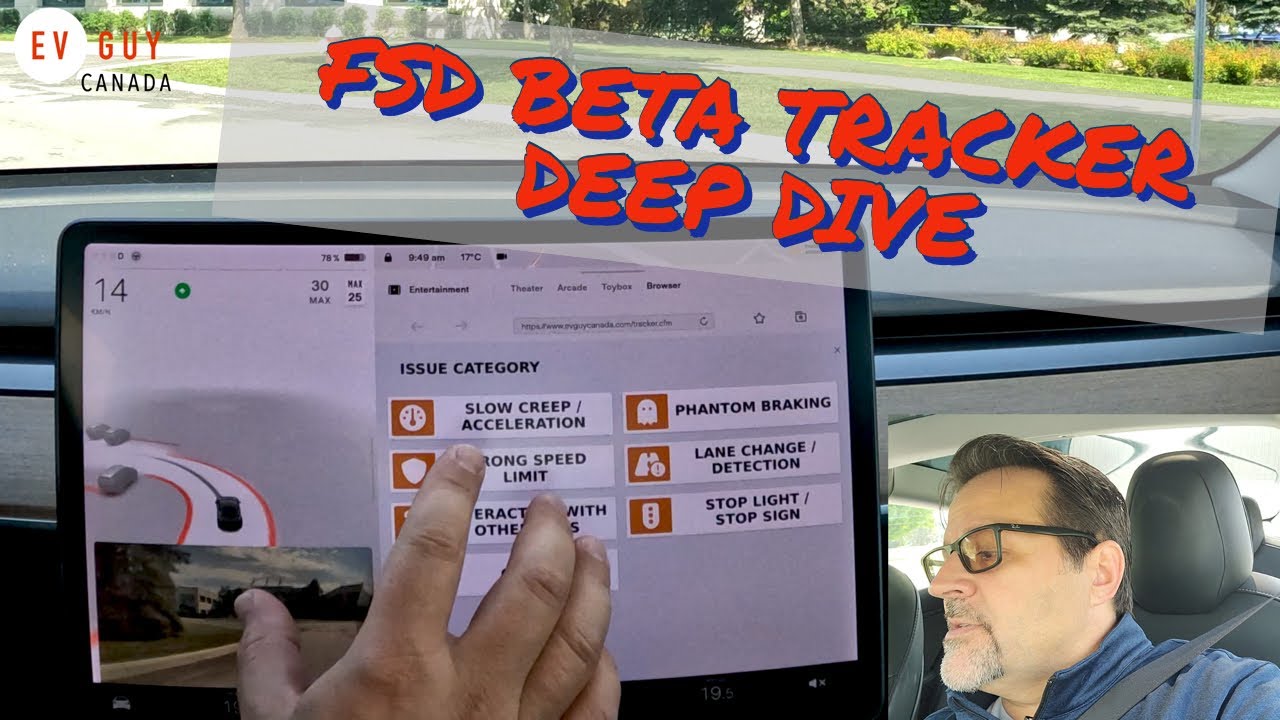
Channel Overview and FSD Tracker Deep Dive
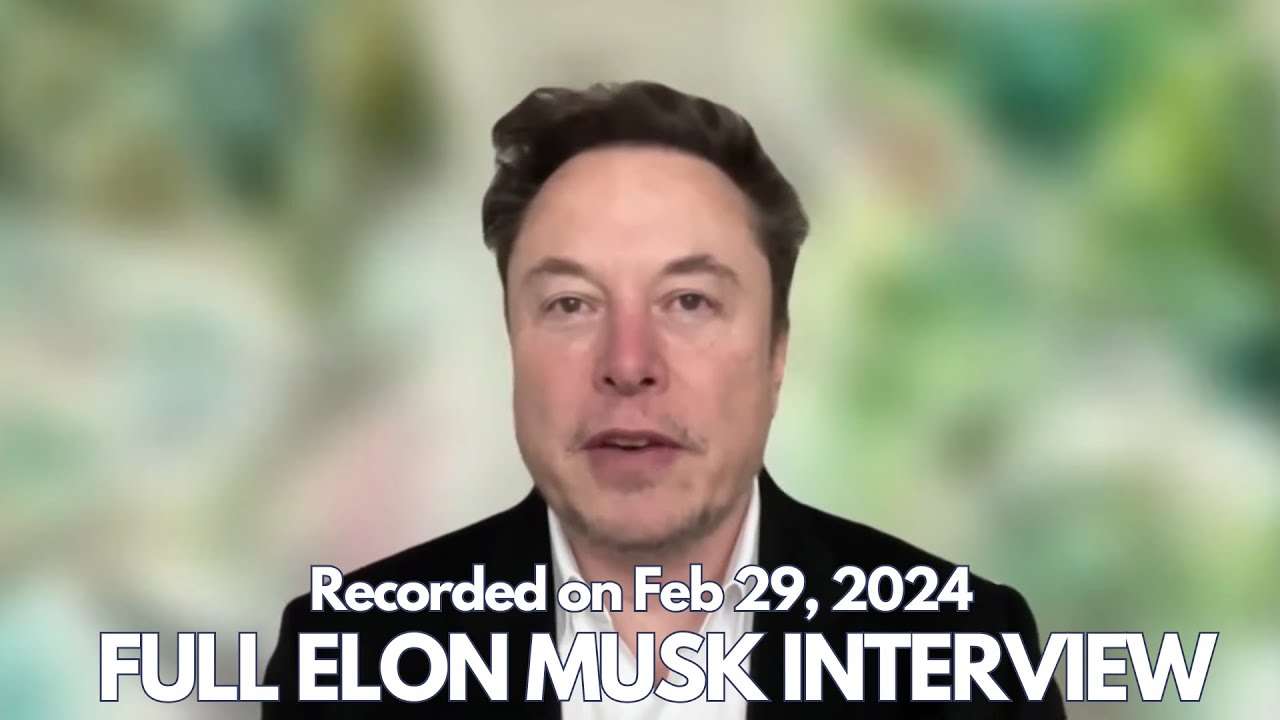
“I’ve NEVER seen anything like this” - Elon Musk drops a bombshell in Interview (Feb 29, 2024)
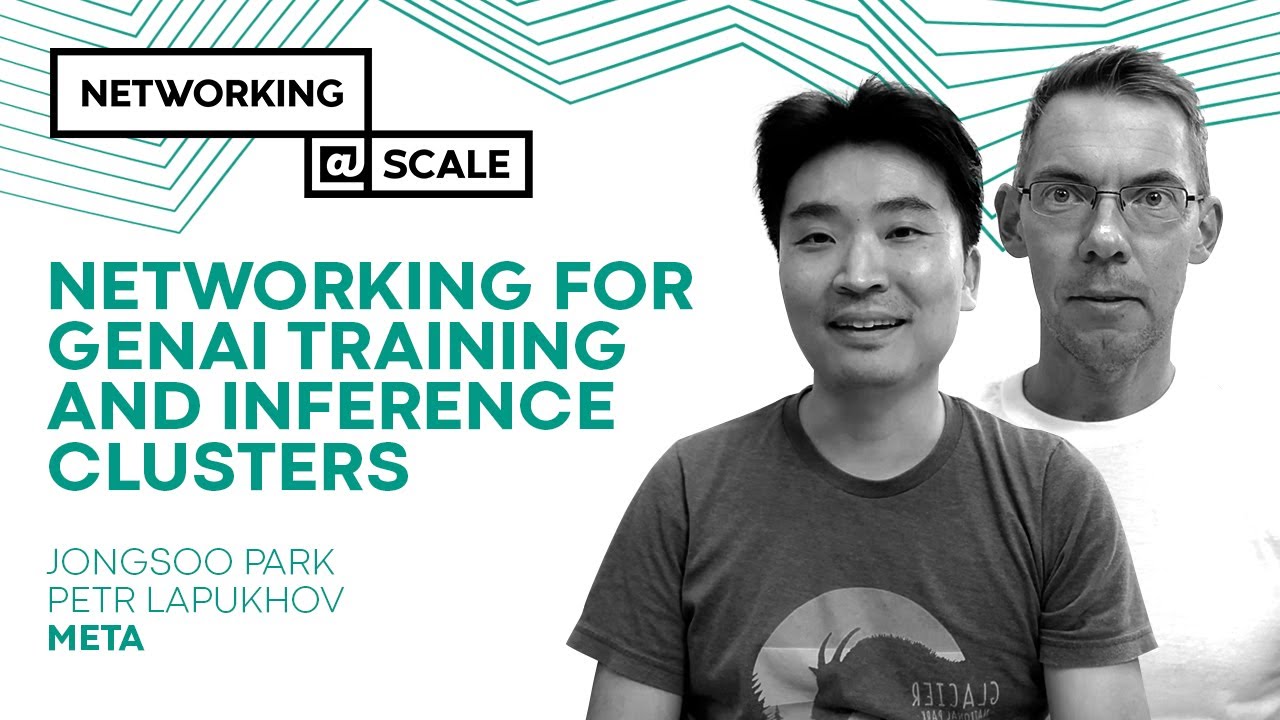
Networking for GenAI Training and Inference Clusters | Jongsoo Park & Petr Lapukhov
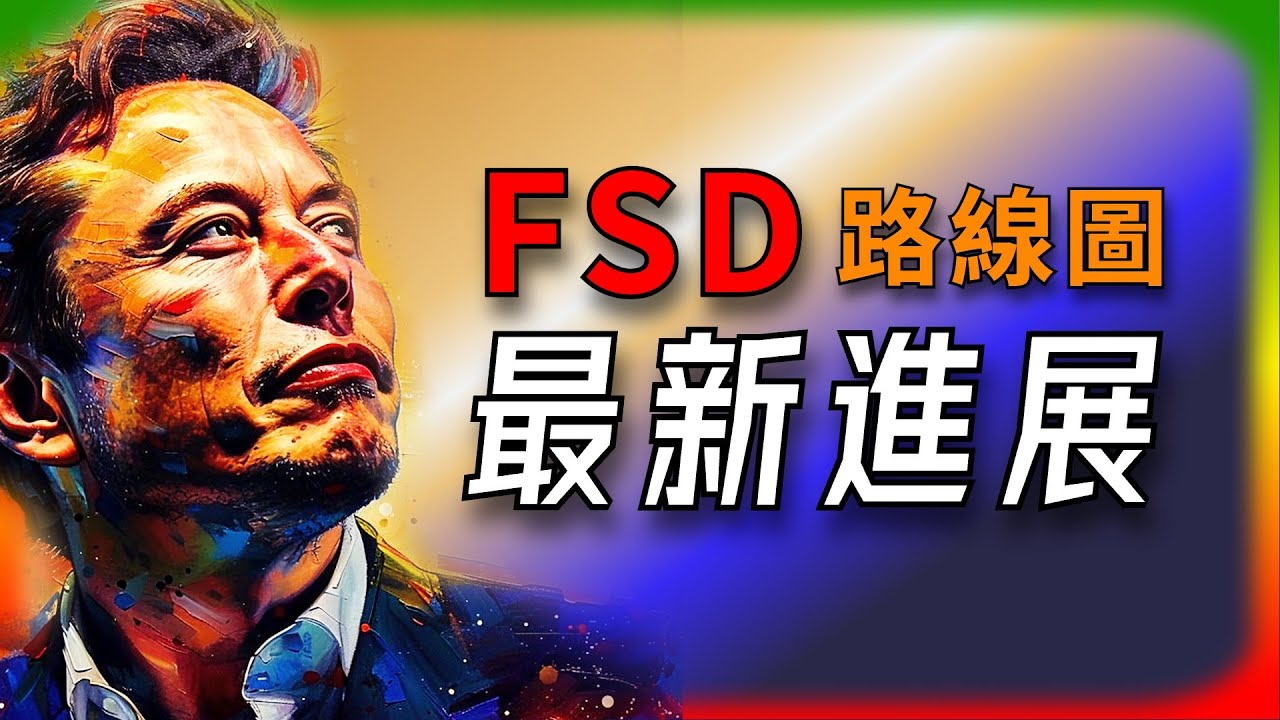
【Tesla每日快訊】 FSD 12.5.4重磅更新:自動駕駛路線圖再下一城! 🔥Semi卡車即將席捲全球(2024/09/23-2)

DeepMind’s New AI Beats Billion Dollar Systems - For Free!

A1 TOURING 2024 NUEVO CIRCUITO OFICIAL RUTA B [EXPLICADO AL DETALLE]
5.0 / 5 (0 votes)