Moral Models: Crucial Decisions in the Age of Computer Simulation
Summary
TLDRThe video discusses the role of computer models in decision-making, particularly during the COVID-19 pandemic. It highlights how models, like those from Neil Ferguson's team, influenced unprecedented interventions. The script explores the limitations and moral implications of relying on models that fail to account for societal impacts, such as economic inequality and mental health. The speaker emphasizes the need for transparency, public involvement, and careful consideration of uncertainties to avoid eroding trust in science and using it as a tool to silence differing perspectives.
Takeaways
- π People want certainty from science, especially during crises, but models provide predictions, not absolute truths.
- π» Computer models significantly influenced global decisions during the COVID-19 pandemic, particularly through Neil Fergusonβs model from Imperial College in March 2020.
- π COVID models guided major decisions, such as lockdowns, school closures, and business shutdowns, using detailed projections about life-and-death outcomes.
- π‘ These models often failed to account for indirect effects, like the impact on economies, healthcare systems, mental health, and developing countries.
- π¨βπ» The 'laptop class' benefitted more from pandemic policies compared to those working in essential jobs, exacerbating social inequalities.
- π Models are not morally neutral; they reflect the values and priorities of those who design them, leading to outcomes that favor certain groups over others.
- π€ Early pandemic models underestimated the uncertainties involved, and policymakers often did not revisit or adjust their forecasts as more data became available.
- βοΈ Science and models should inform decisions, but they cannot substitute for moral judgment or societal debate about values and priorities.
- π¬ Trust in science is a valuable resource, and misuse of models as rhetorical tools can erode public confidence in scientific decision-making.
- π£οΈ Claims like 'following the science' can silence legitimate debates over values and uncertainties, making it crucial to consider broader perspectives when using models for public decisions.
Q & A
What is the primary reason people tend to trust scientific models, especially during crises?
-People often trust scientific models during crises because they seek certainty. They believe that advanced technological tools can predict the future with precision, offering virtuous, scientific, and morally neutral guidance.
How did computer models influence decision-making during the COVID-19 pandemic?
-During the COVID-19 pandemic, computer models, such as those developed by Neil Ferguson's team at Imperial College London, played a significant role in decision-making. These models predicted the impact of various interventions, guiding policies that affected millions of lives globally.
What was unprecedented about the use of models in the COVID-19 pandemic?
-The unprecedented aspect was how central computer models became in guiding interventions that affected nearly everyone. These models provided detailed predictions, down to the number of lives that could be saved or lost, influencing policies in the UK, US, and globally.
What important factors did early COVID-19 models fail to consider?
-Early COVID-19 models largely ignored the broader social and economic costs, such as the impact on healthcare systems, the economy, developing countries, social isolation effects, and exacerbating inequalities.
Why did the pandemic response benefit certain groups over others?
-The pandemic response benefited people similar to the modellers (those in 'laptop class' jobs) because the interventions they recommended (e.g., remote work) suited their circumstances, while people in roles like meatpacking or grocery delivery faced greater hardships.
What moral challenges arise when creating models for public decision-making?
-Creating models for public decision-making involves making choices about what to represent, and these choices are never morally neutral. They can favor certain values, often reflecting the interests of the modellers rather than those affected by the policies.
Why should modellers consider the broader social impact of their recommendations?
-Modellers should consider the broader social impact to ensure fairness, especially in how their recommendations might affect people unlike them. For example, school closures may disproportionately harm children from disadvantaged backgrounds.
How did the phrase 'following the science' influence public debate during the pandemic?
-The phrase 'following the science' was often used to shut down alternative viewpoints, suggesting that certain policies were scientifically justified without considering differing values or uncertainties. This silenced legitimate debate.
What is the role of uncertainty in scientific models, and how should it be handled?
-Uncertainty is a fundamental aspect of scientific models. Good models account for various possible outcomes by exploring different parameters. During the pandemic, some models did not fully address the uncertainties, leading to overconfidence in their predictions.
What is the long-term risk of using scientific models as a rhetorical tool in policymaking?
-The long-term risk is eroding public trust in science. If models are used to justify pre-existing decisions or as tools to silence debate, it can undermine the credibility of science and diminish its role in guiding future decisions.
Outlines
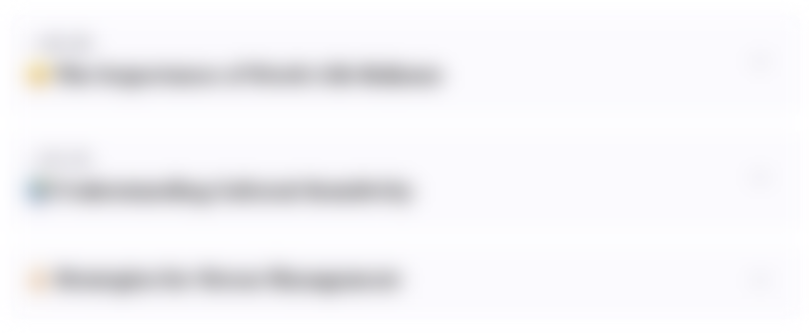
This section is available to paid users only. Please upgrade to access this part.
Upgrade NowMindmap
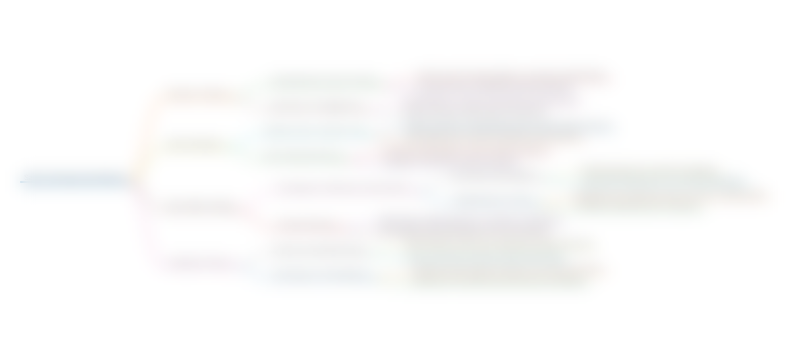
This section is available to paid users only. Please upgrade to access this part.
Upgrade NowKeywords
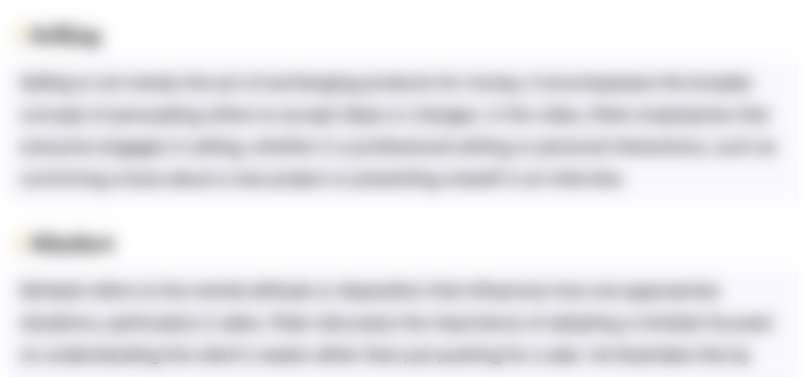
This section is available to paid users only. Please upgrade to access this part.
Upgrade NowHighlights
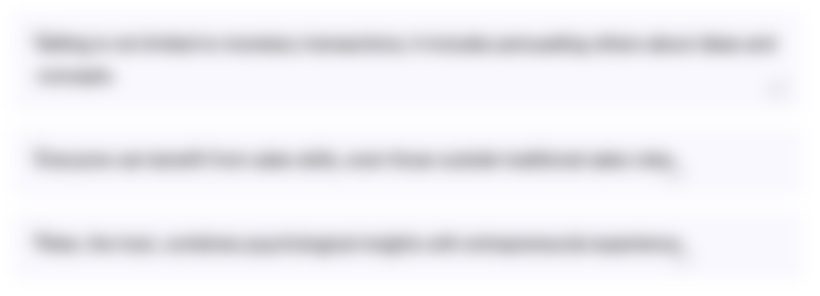
This section is available to paid users only. Please upgrade to access this part.
Upgrade NowTranscripts
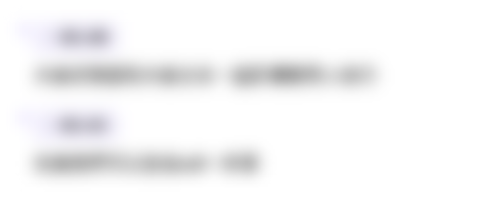
This section is available to paid users only. Please upgrade to access this part.
Upgrade Now5.0 / 5 (0 votes)