Full interview: "Godfather of artificial intelligence" talks impact and potential of AI
Summary
TLDRこのビデオのスクリプトでは、AIと機械学習の現在の重要な時点について語られています。特に、ChatGPTのような大規模言語モデルの可能性と、一般公衆の反応の大きさに注目しています。ニューラルネットワークと後方伝播の概念、ディープラーニングの進化、そしてこれらの技術が人間の脳の理解にどのように貢献するかに焦点を当て、AI技術の将来への展望を提供しています。また、これらの進歩が社会、職場、倫理的問題にどのように影響を与えるかについても考察しています。
Takeaways
- 🔍 AIのこの瞬間は、言語モデルが素晴らしいことができるという事実を一般の人々が突然認識した画期的な瞬間です。
- 🤖 チャットGPTに対する一般の反応は、研究者たちも驚くほど大きなものでした。
- 🧠 AI分野には、論理と推論に基づく主流のAIと、生物学的なニューラルネットに基づく別の学派が存在しています。
- ⚙️ ニューラルネットワークが1980年代にうまく機能しなかったのは、コンピュータの速度とデータセットの大きさが不十分だったからです。
- 💡 ニューラルネットワークによる学習は、脳の働きを模倣することに基づいており、それは人間が読み書きや数学を学ぶ能力にも関連しています。
- 📈 ディープラーニングの進化は、複数の表現層を持つニューラルネットを用いて複雑な学習を可能にする重要な転換点でした。
- 🖥️ バックプロパゲーションとは、予測が間違っていた場合にネットワークの各接続の強度を調整して、より正確な予測を行うプロセスです。
- 🔬 画像認識システムの進化は、物体を正確に認識できるようになったことで、AIの重要な成果の一つとなりました。
- 🤔 AIが言語を理解するプロセスは、単に次の単語を予測すること以上のものであり、実際の理解を必要とします。
- 🚀 AI技術の発展は、産業革命や電気の発明と同じくらいの規模で人類の生活に影響を与える可能性があります。
Q & A
ChatGPTに対する一般の人々の反応について、最初に使用したときの反応はどうでしたか?
-ChatGPTそのものはそれほど驚きませんでしたが、一般の人々の反応の大きさには多少驚きました。
AIとニューラルネットワークにおける2つの主要な学派とは何ですか?
-AIには「メインストリームAI」があり、論理と推論に基づいていました。もう一方の学派は「ニューラルネットワーク」で、生物学的な学習方法、すなわちニューロン間の接続の変化に重点を置いていました。
ニューラルネットワークが1980年代にうまく機能しなかった主な理由は何ですか?
-コンピューターの処理能力が十分ではなく、データセットも十分に大きくなかったためです。
ChatGPTの訓練に用いられる「バックプロパゲーション」について、脳が同じ方法を使用しているとは思いますか?
-いいえ、脳がバックプロパゲーションを使用しているとは思わず、AIと脳の学習方法には根本的な違いがあると考えています。
2006年にAI研究で起きた重要な変化とは何ですか?
-深層学習(ディープラーニング)の研究が始まり、複数のレイヤーを持つニューラルネットワークが複雑な学習を行うことができるようになりました。
画像認識システムにおいて、ニューラルネットワークのアプローチが従来の方法とどのように異なるか?
-ニューラルネットワークはランダムな重みから始めて、エラーを最小限に抑えるように重みを調整することにより、自動的に特徴検出器を学習します。従来の方法では、人が手動で特徴を定義していました。
ニューラルネットワークの研究が加速した理由は何ですか?
-コンピュータの処理能力の向上とデータの量が増加したため、ニューラルネットワークをより大きく、より複雑にすることができるようになりました。
ニューラルネットワークと人間の脳との間の主要な違いは何ですか?
-ニューラルネットワークはデジタルコンピュータ上で動作し、高い計算能力を必要としますが、人間の脳はアナログで、はるかに低いエネルギーで動作します。
将来、コーディングのスキルが必要かどうかについての見解は?
-AIの発展により、コーディングの仕事の性質が変化する可能性がありますが、創造的な側面により重点を置くようになるかもしれません。
AIと脳の研究が異なる道を進むと考える理由は何ですか?
-AIの発展はバックプロパゲーションに依存していますが、人間の脳が同じメカニズムを使用しているとは思わないため、理論的に異なる方向に進んでいると考えています。
Outlines
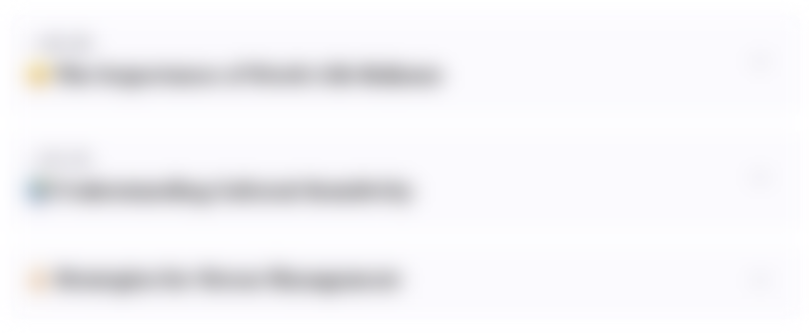
This section is available to paid users only. Please upgrade to access this part.
Upgrade NowMindmap
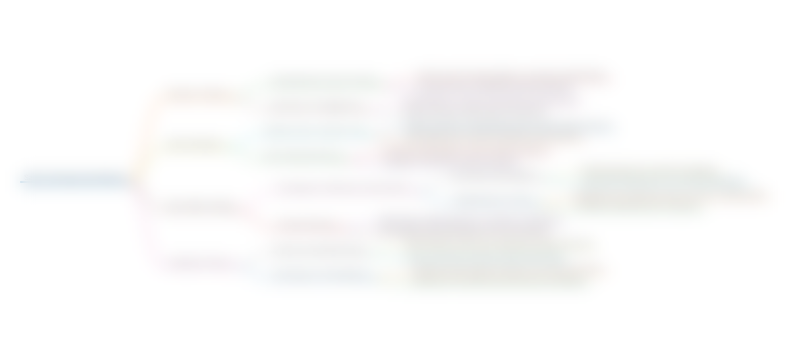
This section is available to paid users only. Please upgrade to access this part.
Upgrade NowKeywords
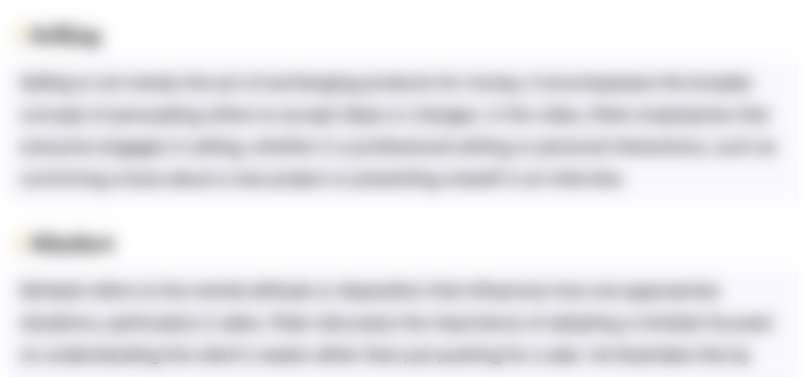
This section is available to paid users only. Please upgrade to access this part.
Upgrade NowHighlights
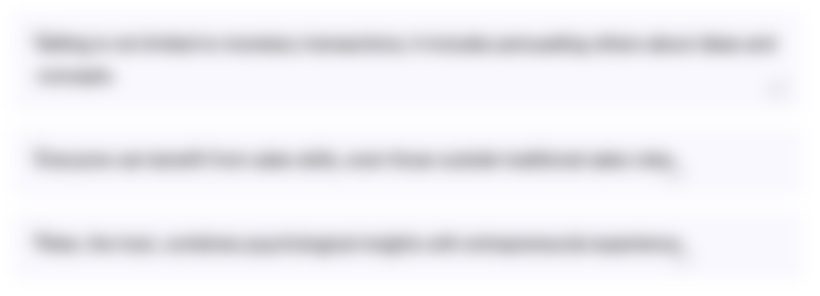
This section is available to paid users only. Please upgrade to access this part.
Upgrade NowTranscripts
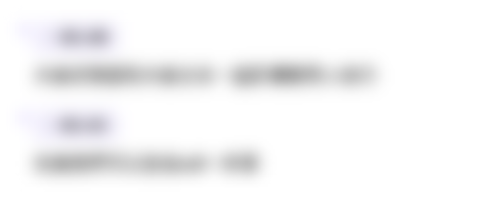
This section is available to paid users only. Please upgrade to access this part.
Upgrade NowBrowse More Related Video
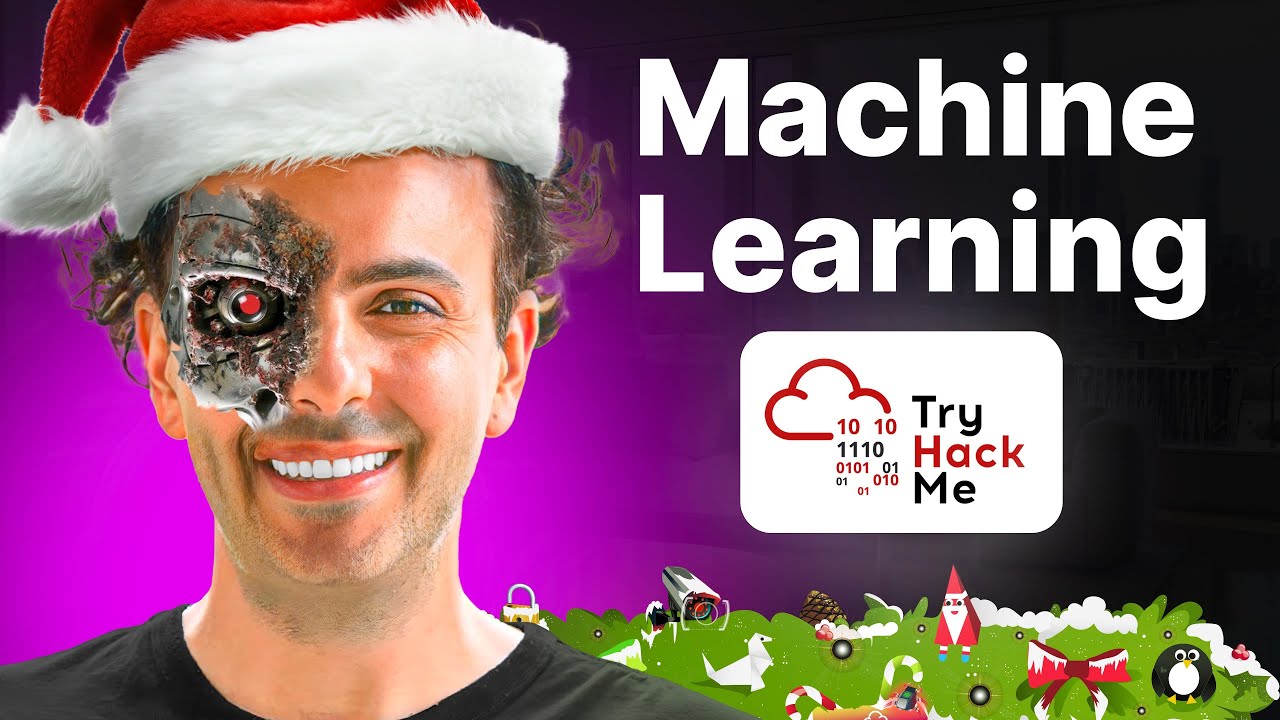
Beginner Machine Learning tutorial (TryHackMe Advent of Cyber Day 14)

【超効率英語学習メソッド】1日90分で英語は伸びる / ビジネスパーソン必見の英語コーチング / 英会話スクールで挫折する理由を分析 【&SKILL SET】
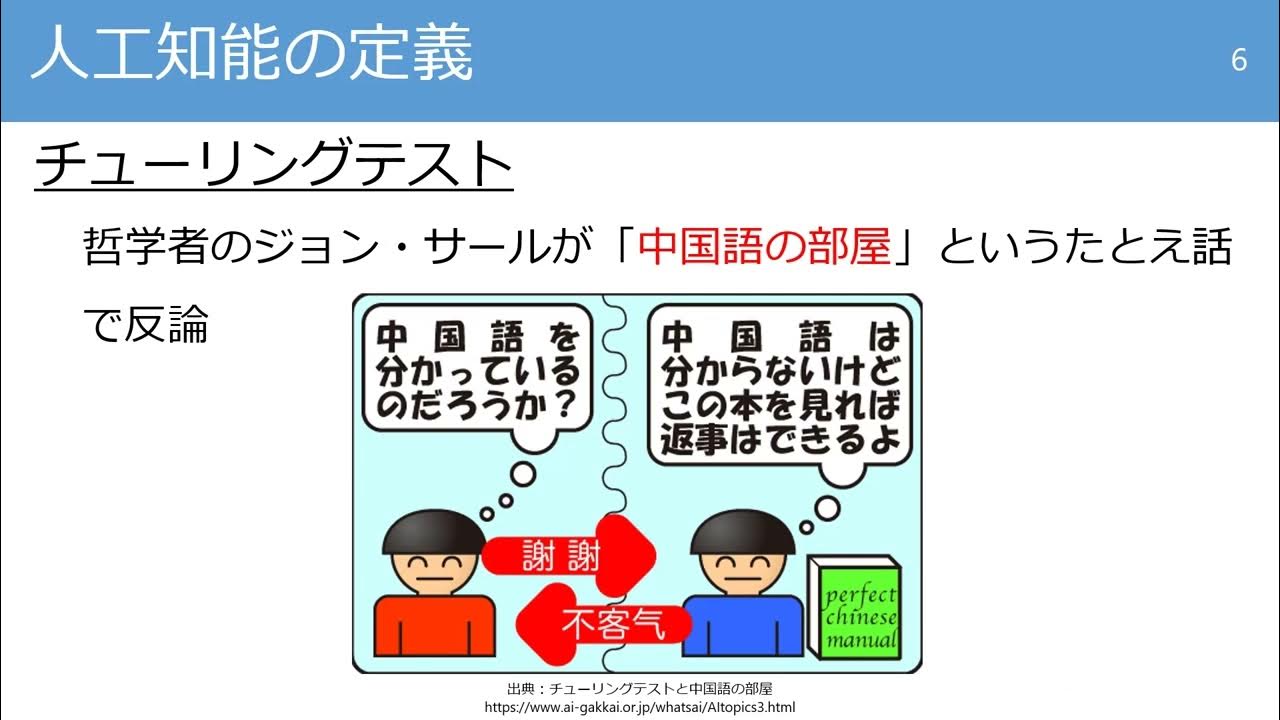
AIの歴史
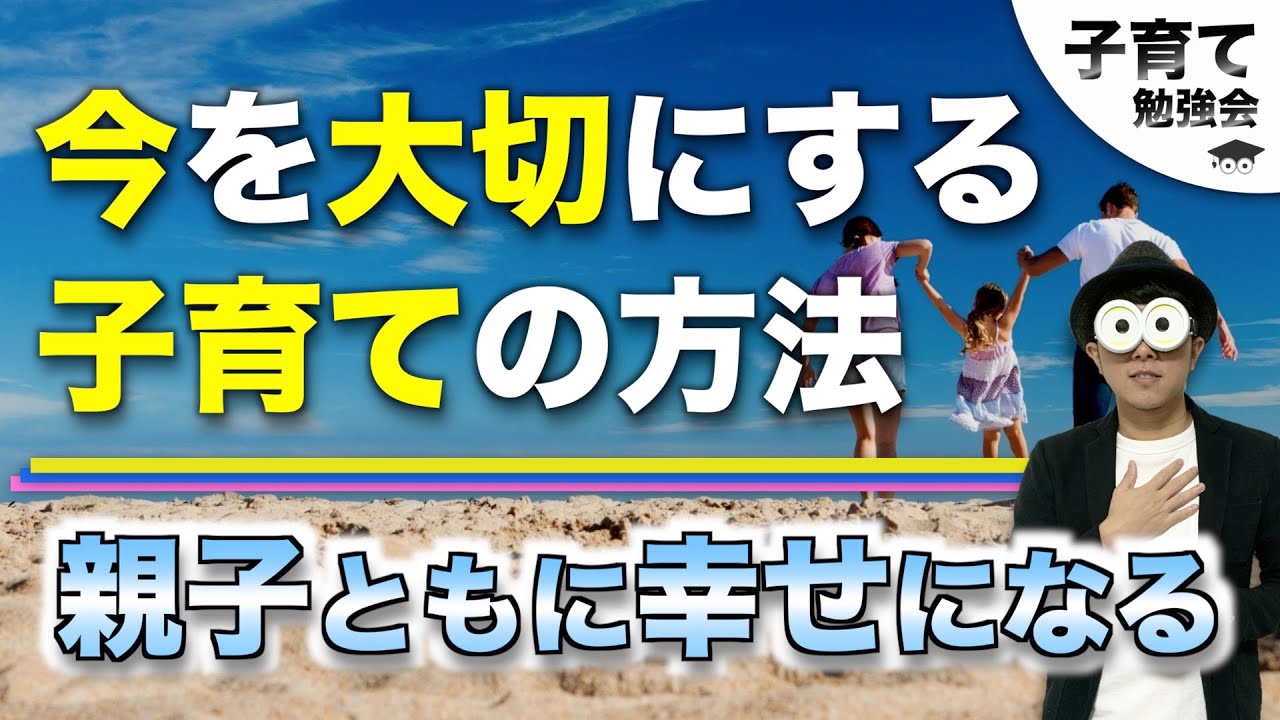
0~12歳【子育ての大切な価値観】今の幸せ・時間を大切にしてほしい/子育て勉強会TERUの育児・知育・幼児教育・子どもの教育講義
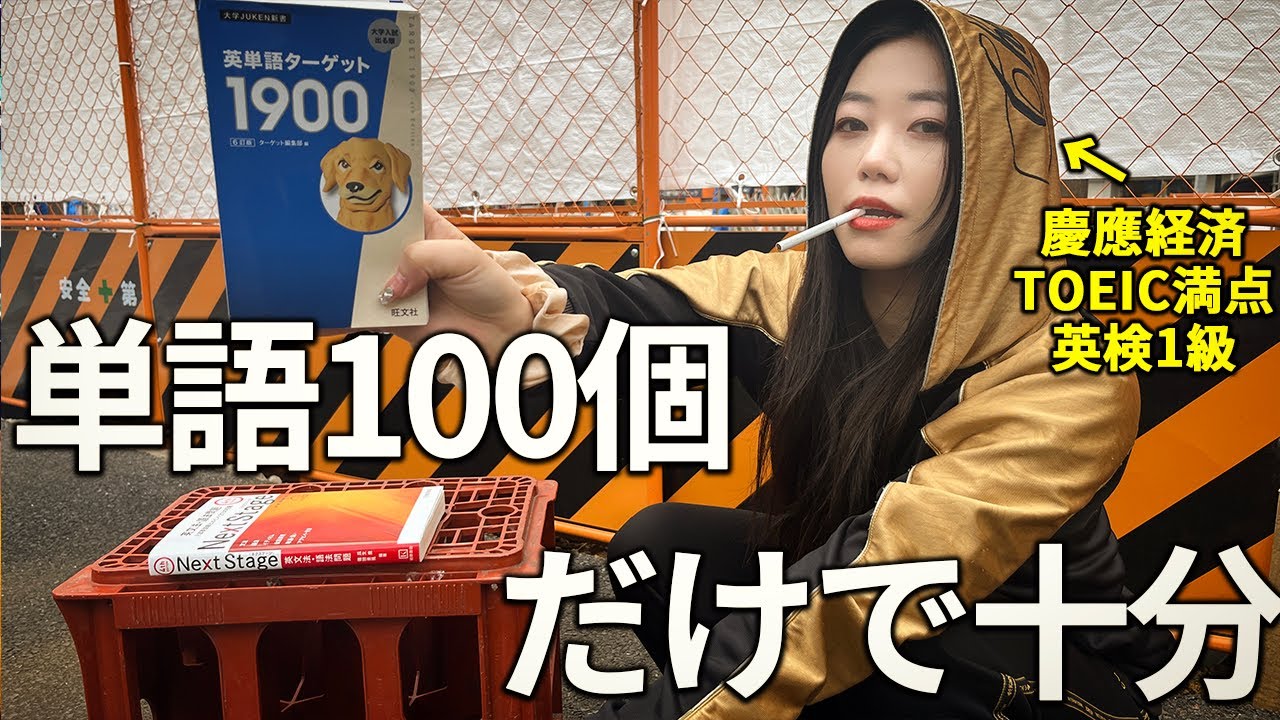
【完全版】この100単語で50%の英会話ができるようになるから、やるしかねーだろ卍
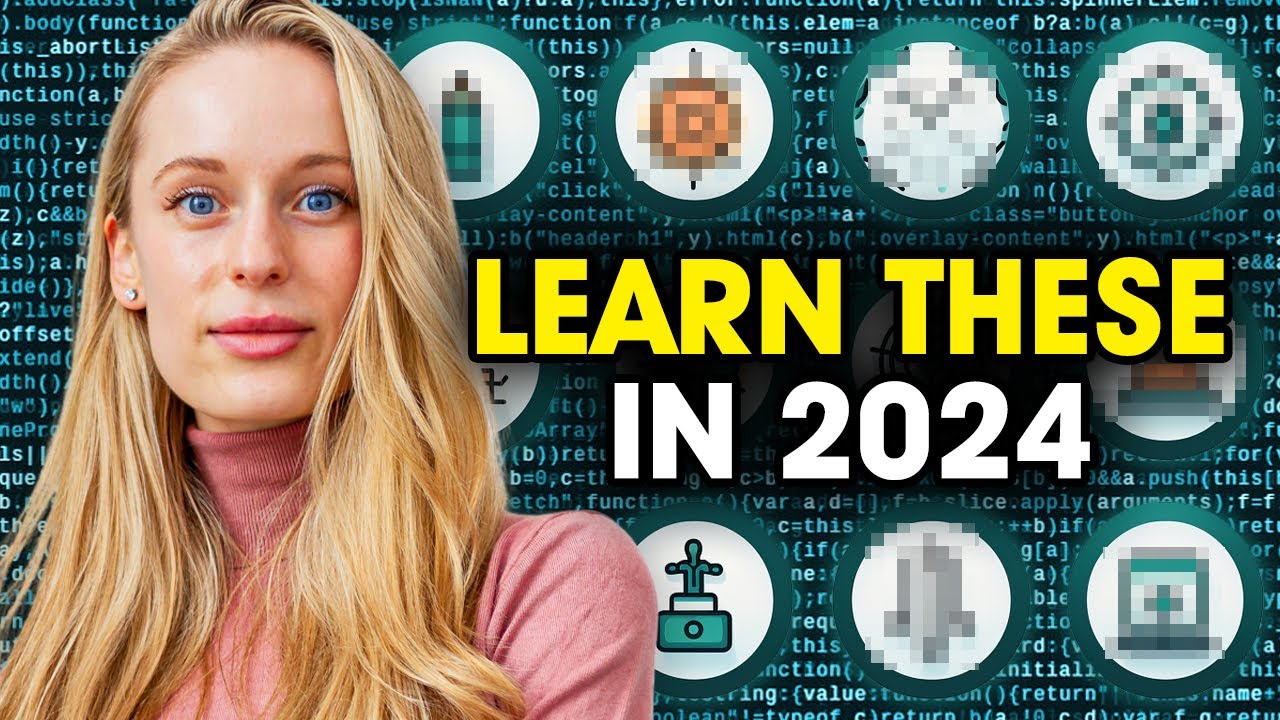
What are the top 10 technologies to learn in 2024?
5.0 / 5 (0 votes)