Reviewing the AI Battlefront | The Brainstorm EP 38
Summary
TLDRIn episode 38 of the Brainstorm podcast, the hosts discuss the transformative impact of AI on enterprise productivity, using Clara's AI software as a case study. They explore how AI can multiply knowledge worker productivity, leading to significant cost savings and improved customer experiences. The conversation also touches on the challenges faced by tech giants like Google in developing competitive AI models and the strategic implications for companies considering AI adoption. The hosts emphasize the rapid pace of AI innovation and the need for organizations to adapt to stay relevant.
Takeaways
- 🚀 AI software is seen as a productivity multiplier for enterprises, with potential to boost knowledge worker productivity significantly.
- 📈 By 2030, AI software spend is projected to reach $14 trillion, a substantial increase from the current global spend.
- 🤖 Clara's AI implementation in their call center has led to an eightfold increase in productivity, saving the company millions annually.
- 💡 AI adoption in enterprises is not about replacing employees but enhancing their bandwidth and efficiency.
- 📊 The success of AI implementations like Clara's is expected to drive more customer service interactions due to improved efficiency.
- 🔍 Companies that aggressively deploy AI are likely to see better margins, reduced churn, and improved competitiveness.
- 📝 The deployment time for AI models can vary, but the impact on business operations can be rapid and transformative.
- 🌐 Large tech companies like Google and Apple are expected to eventually integrate AI advancements into their consumer-facing services.
- 🔄 The balance between AI model performance and distribution capabilities is crucial for both consumer and enterprise adoption.
- 🔄 Open-source AI models may become a strategic play for companies like Meta, offering a backend infrastructure for enterprises.
Q & A
What is the main theme of the brainstorm episode 38?
-The main theme of the episode is the impact and potential of AI within enterprises, specifically focusing on AI software as a productivity multiplier for knowledge workers.
How much value is AI expected to deliver to enterprises by 2030?
-By 2030, AI software spend is expected to reach $14 trillion, which is a significant increase from the current annual spend of four to five trillion dollars.
What was the press release from Clara about their AI software implementation?
-Clara's press release highlighted that they are saving approximately 40 million dollars annually by using AI software in their call center operations, which has improved customer interaction times and reduced the need for human agents.
How has Clara's AI software improved customer service efficiency?
-Clara's AI software has reduced the time to provide an answer to a customer from 11 minutes to 2 minutes per interaction and decreased the likelihood of needing to follow up with a customer agent by 25%.
What is the estimated productivity improvement for Clara's AI software?
-The AI software has resulted in an estimated eight times productivity improvement in terms of time to provide an answer to a customer.
How does the deployment of AI in customer service impact the overall customer experience?
-The deployment of AI in customer service is expected to make interactions more productive and pleasant, potentially increasing the likelihood of customers choosing to interact with companies for their needs.
What are the potential implications for companies that do not adopt AI technology?
-Companies that do not adopt AI technology may fall behind in competitive landscapes, as AI can significantly improve efficiency, customer service, and overall business performance.
What is the significance of the performance of AI models in the enterprise context?
-In the enterprise context, even marginal performance improvements in AI models can be extremely valuable, as they can lead to significant cost savings and efficiency gains in operations.
How does the distribution of AI models affect their adoption and impact on businesses?
-The distribution of AI models is crucial as it allows for broader adoption and more data collection, which in turn can improve the model's performance and adaptability to various business needs.
What is the current state of AI development at Google compared to other companies?
-Google appears to be lagging behind in AI development, as their recent release of the Gemini Ultra model is not as performant as state-of-the-art models from a year ago, despite their vast resources and data.
Outlines
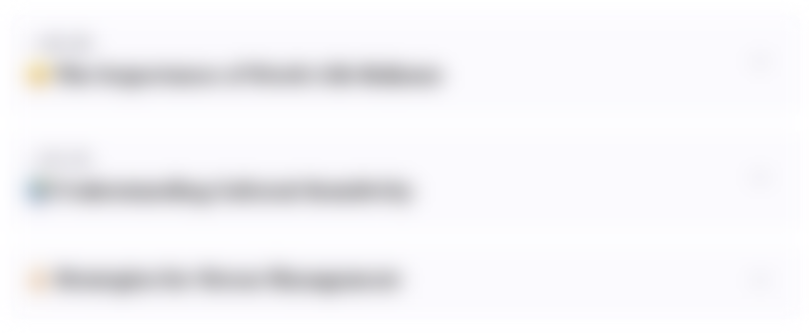
This section is available to paid users only. Please upgrade to access this part.
Upgrade NowMindmap
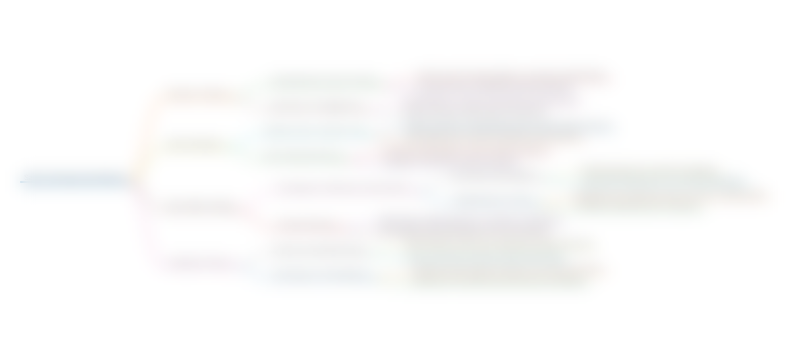
This section is available to paid users only. Please upgrade to access this part.
Upgrade NowKeywords
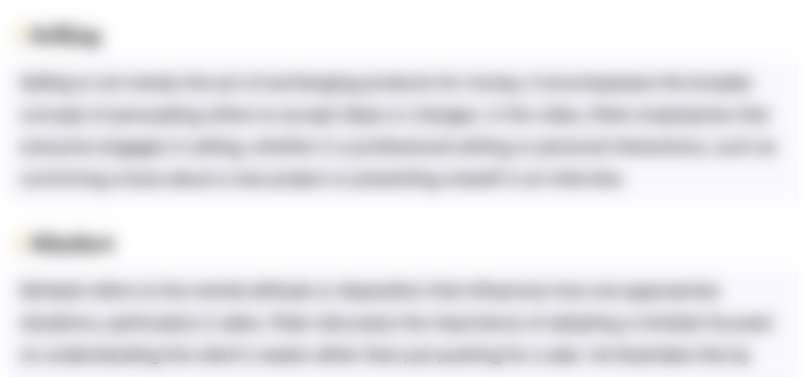
This section is available to paid users only. Please upgrade to access this part.
Upgrade NowHighlights
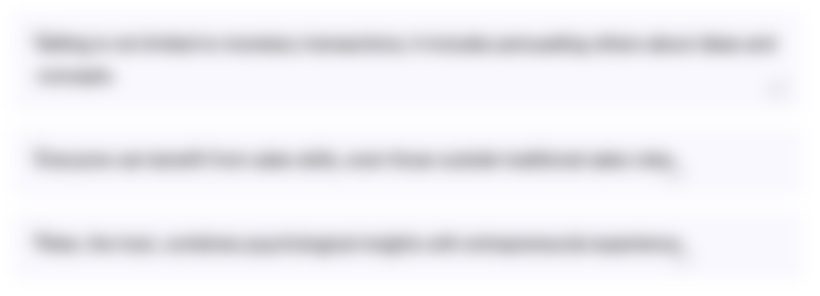
This section is available to paid users only. Please upgrade to access this part.
Upgrade NowTranscripts
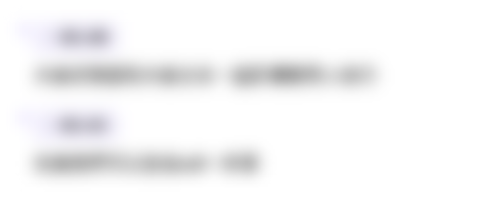
This section is available to paid users only. Please upgrade to access this part.
Upgrade NowBrowse More Related Video
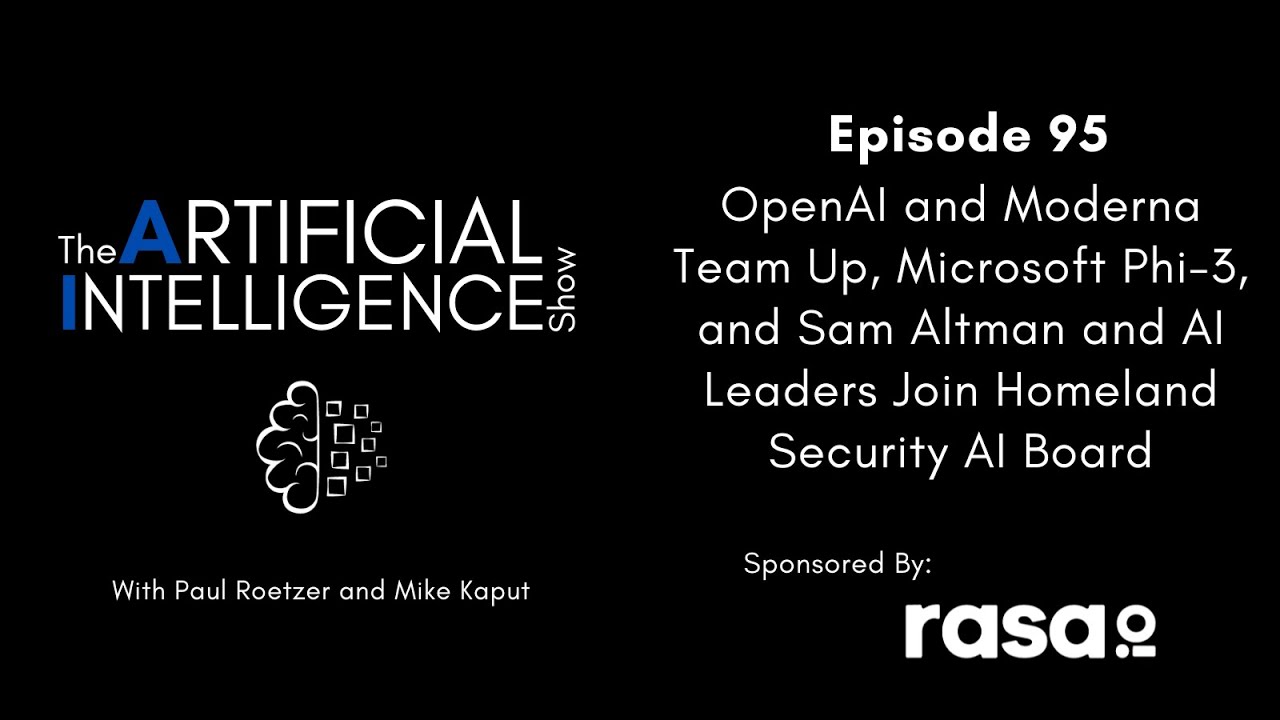
Ep #95: OpenAI and Moderna, Microsoft Phi-3, Sam Altman & AI Leaders Join Homeland Security AI Board

Jeff Clarke, Dell | Dell Technologies World 2024
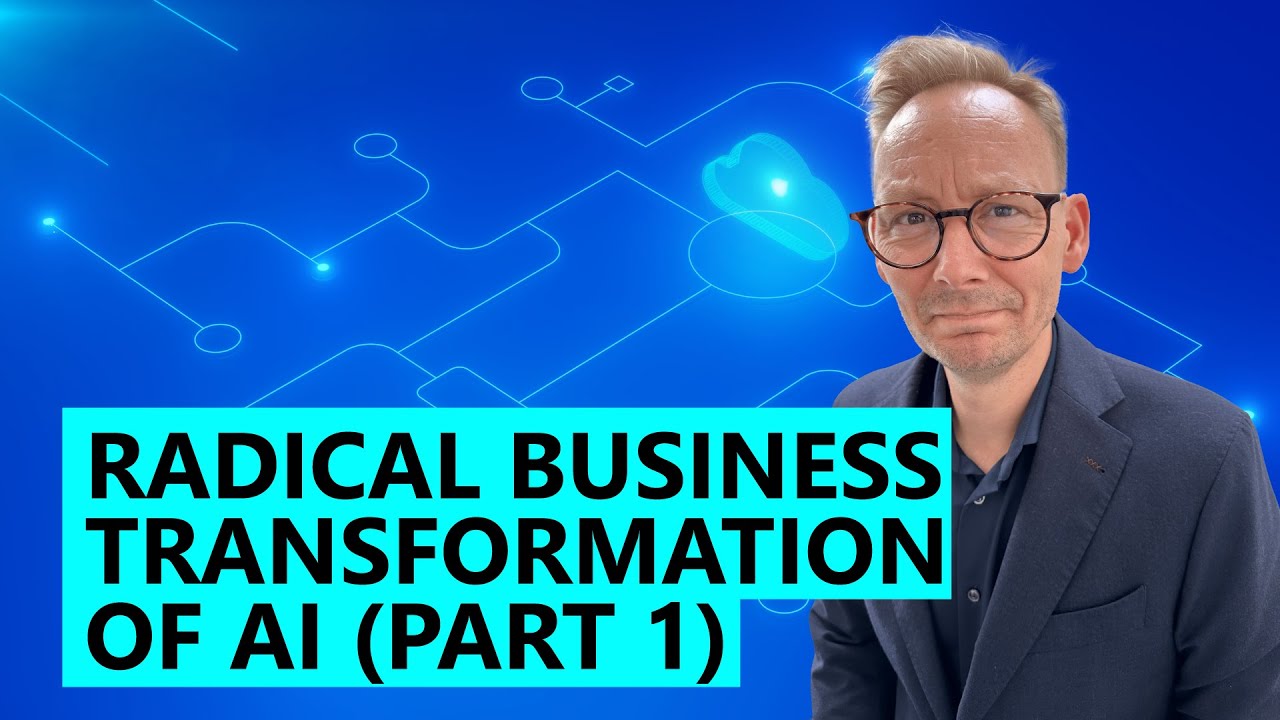
Radical Business Transformation of AI (Part 1)

Creepy or Cool? Exploring Personal AI - PI AI Blew Our Minds!
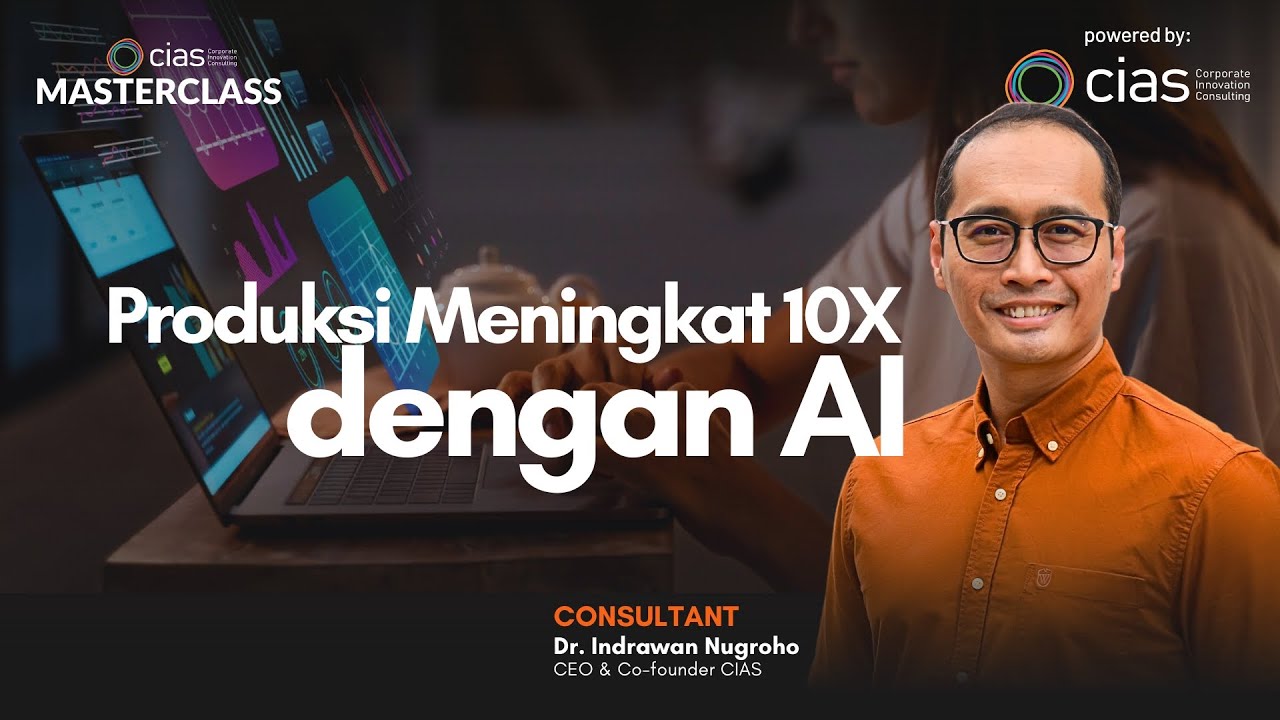
HR WAJIB Tahu! Ini Strategi Adopsi AI agar Karyawan Makin Produktif
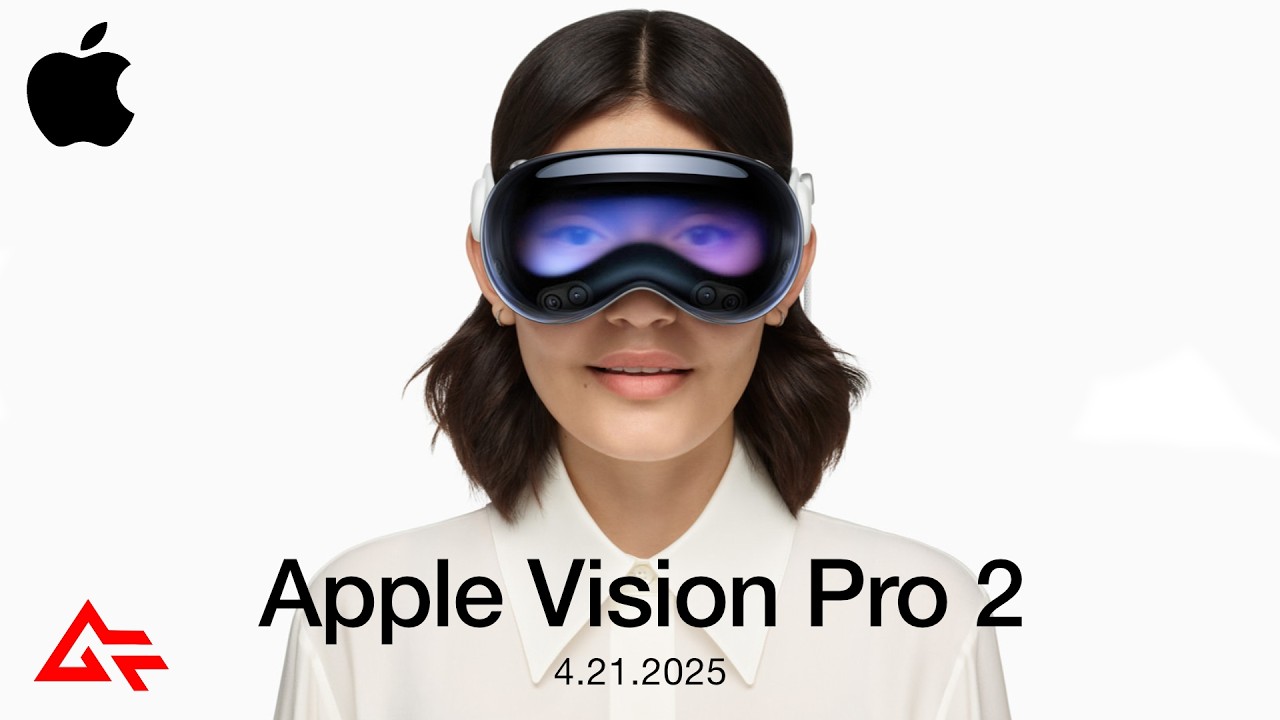
NEW M5 Apple Vision Pro 2 LEAKED | 2025 Launch Date
5.0 / 5 (0 votes)