DataVis Colour Basics
Summary
TLDRThis video script offers a comprehensive guide on color usage in data visualization, emphasizing the importance of color scales for representing numerical data and categories. It discusses the psychological impact of color, suggesting dark colors for higher values and light for lower ones. The script covers various color scales, including sequential and diverging, and provides tips for choosing categorical colors that are both aesthetically pleasing and functionally distinct. It also addresses accessibility, such as color blindness considerations, and stresses the need for high contrast and intuitive color choices to ensure clarity and comprehension in data visualization.
Takeaways
- 🎨 **Use Color Scales Wisely**: When representing numerical data with color, create a scale that maps larger numbers to darker colors and smaller numbers to lighter ones for better visual comprehension.
- 🌈 **Sequential Color Palettes**: Utilize sequential color palettes that transition from one hue to another, such as a gradient from blue to green, to effectively represent changes in data.
- 🔄 **Divergent Color Scales**: For comparing two opposing categories, use divergent color scales that meet at a midpoint, ensuring that hues are distinct to emphasize the difference.
- 📊 **Categorical Color Choices**: When mapping data categories, choose colors that are distinct from each other to ensure readability, especially for audiences with varying visual capabilities.
- 🚫 **Avoid Adjacent Hues**: For categorical data, avoid using colors that are too close on the color wheel; instead, maximize differences by selecting hues with at least 35° of separation.
- 🔢 **Limit Color Palette**: Restrict the use of colors in data visualization to five or six to maintain ease of understanding and memorability for the audience.
- 📈 **Use Colors to Highlight Important Data**: Employ colors to emphasize key data points, using gradients for patterns and bars or positions for specific values to enhance readability.
- 🔗 **Consistency in Color Usage**: Maintain consistency by using the same color for the same variables across different charts to avoid confusion.
- 👀 **Ensure High Contrast**: Pay attention to color contrast for accessibility and readability, especially important for text and small elements in a visualization.
- 🌐 **Consider Cultural Color Meanings**: Be aware of the cultural significance of colors and use intuitive colors that resonate with the audience's preconceived associations.
- 👓 **Check for Color Blindness Compatibility**: Utilize tools to verify that color choices are distinguishable by individuals with color vision deficiencies to ensure inclusivity.
Q & A
What is the primary purpose of using color in data visualization?
-The primary purpose of using color in data visualization is to make shapes visible, encode data or categories, and enhance the visual representation of numerical data.
Why is it important to create a scale when using color to represent numerical data?
-Creating a scale is important because it helps in mapping numerical data effectively. It uses a gradient that starts with a larger number on one end and a smaller number on the other, leveraging visual psychology to guide color choices.
How does the association of darker colors with density influence color choices in data visualization?
-Darker colors are perceived as having higher value or representing more of something due to their association with density. Therefore, they are typically mapped to the larger end of a scale, while lighter colors represent less or smaller values.
What is a sequential color scale and how is it developed?
-A sequential color scale is a gradient that progresses from one color to another, usually from a darker color representing higher values to a lighter color for lower values. It is developed by selecting hues that are adjacent on the color wheel and maintaining a consistent lightness.
What is the significance of using cool and warm colors in data visualization?
-Using cool colors like blue or purple for high contrast and warm colors for low contrast on the opposite end helps to accentuate the transition from dark to light, making it easier for viewers to understand the data.
How does the choice of colors affect the differentiation between categories in a data visualization?
-The choice of colors should maximize differences between categories to ensure they are easily distinguishable. Colors that are too similar or close on the color wheel can be confusing, especially for those with impaired vision or on low-quality screens.
What is a diverging color scale and how should it be used effectively?
-A diverging color scale uses two different scales to represent two opposing ends of a spectrum, such as comparing Democrats and Republicans. It should be used effectively by ensuring that the hues of each scale are distinct and do not get too close to each other, accentuating the distance from the midpoint.
Why is it recommended to limit the color palette to five or six colors in data visualization?
-Limiting the color palette helps to maintain readability and ease of understanding. More than six colors can overwhelm the viewer, making it difficult to remember the meaning behind each color and potentially confusing the visualization.
What is the role of gray in data visualization and how should it be used?
-Gray is an important color in data visualization as it can be used for less important parts of the chart, allowing color to be used as a highlight for important data points. It's also useful for general context data and less important annotations.
Why is high color contrast important in data visualization, and what are the recommended contrast ratios for text?
-High color contrast is important for accessibility and readability, especially on screens in low light conditions. The recommended contrast ratio is 2.5 for large text and at least 4.5 for small text.
How can color choices be improved when considering the target audience's cultural associations with colors?
-Color choices can be improved by using intuitive colors that the target audience will associate with the data. This includes considering cultural meanings, avoiding stereotypes, and using colors that are naturally or learnedly associated with certain concepts, like red for alert or green for go.
What is the impact of using gradient color palettes for categories instead of values?
-Using gradient color palettes for categories can lead to confusion as viewers may misinterpret the gradient as representing different amounts or values of the categories, rather than distinct categories.
How can color blindness be considered when choosing color palettes for data visualization?
-Color blindness can be considered by using online tools to check color combinations for visibility to those with color vision deficiencies. Ensuring that colors used can be distinguished by all viewers is crucial for inclusive data visualization.
Outlines
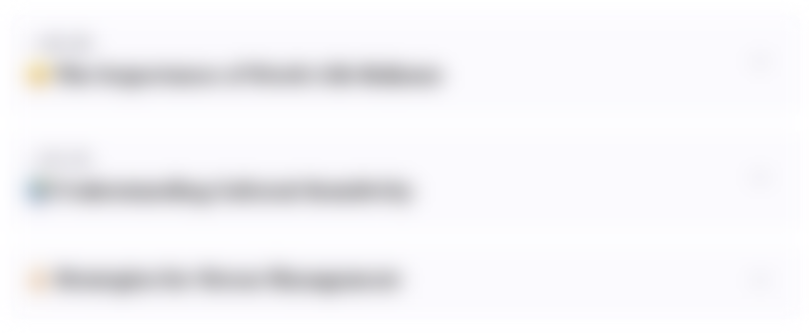
This section is available to paid users only. Please upgrade to access this part.
Upgrade NowMindmap
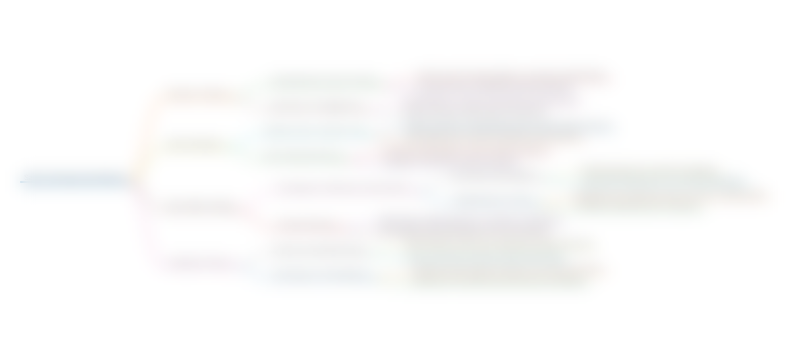
This section is available to paid users only. Please upgrade to access this part.
Upgrade NowKeywords
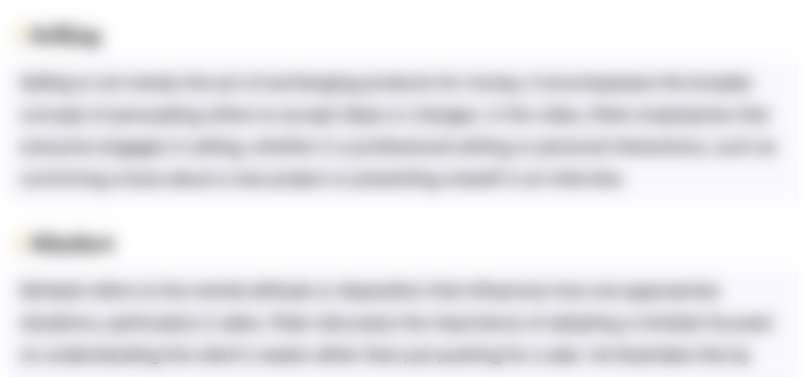
This section is available to paid users only. Please upgrade to access this part.
Upgrade NowHighlights
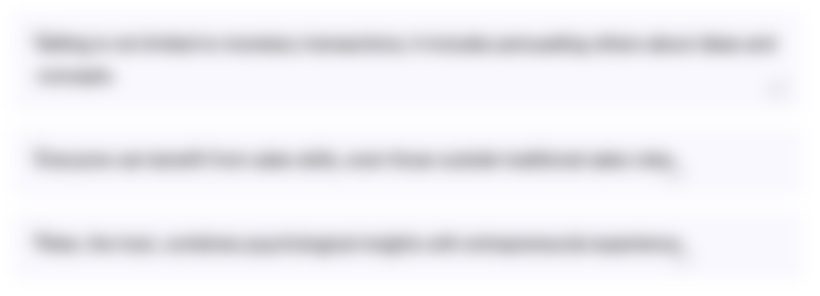
This section is available to paid users only. Please upgrade to access this part.
Upgrade NowTranscripts
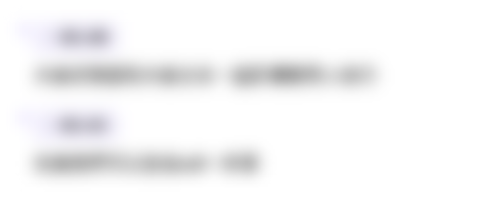
This section is available to paid users only. Please upgrade to access this part.
Upgrade NowBrowse More Related Video

How to use color in your data visualization
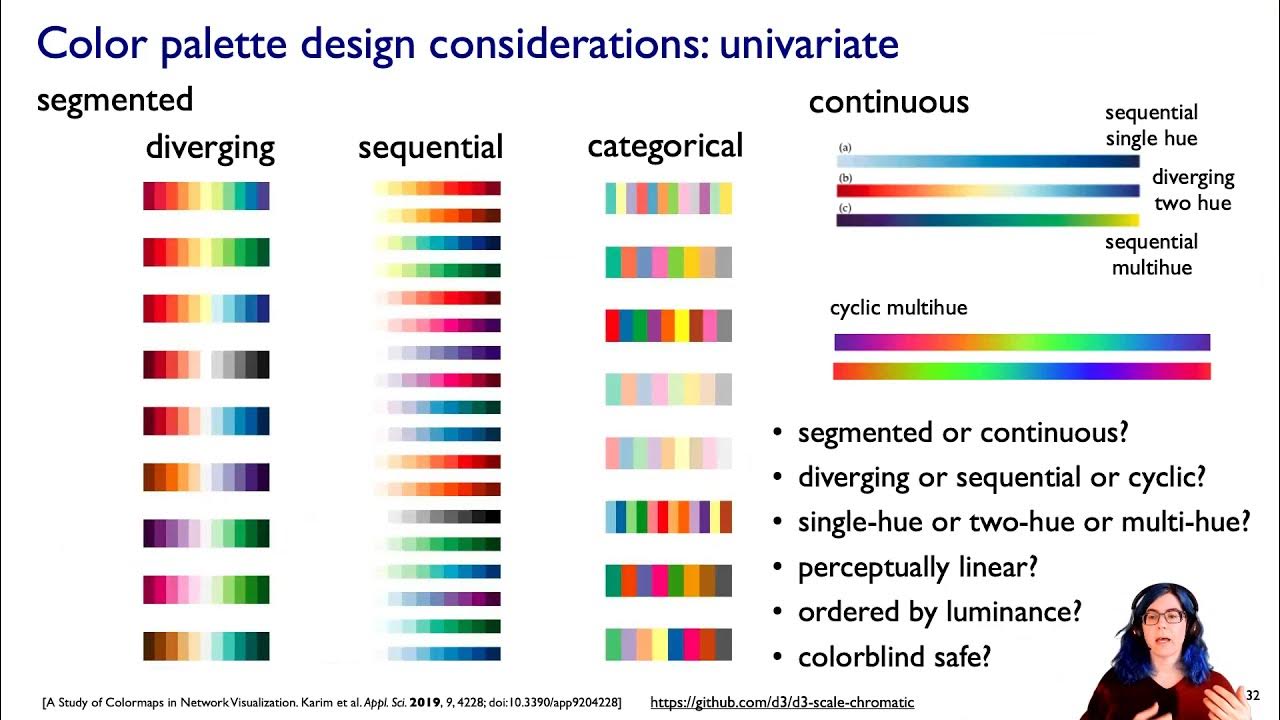
Color (Ch 10) I, Visualization Analysis & Design, 2021
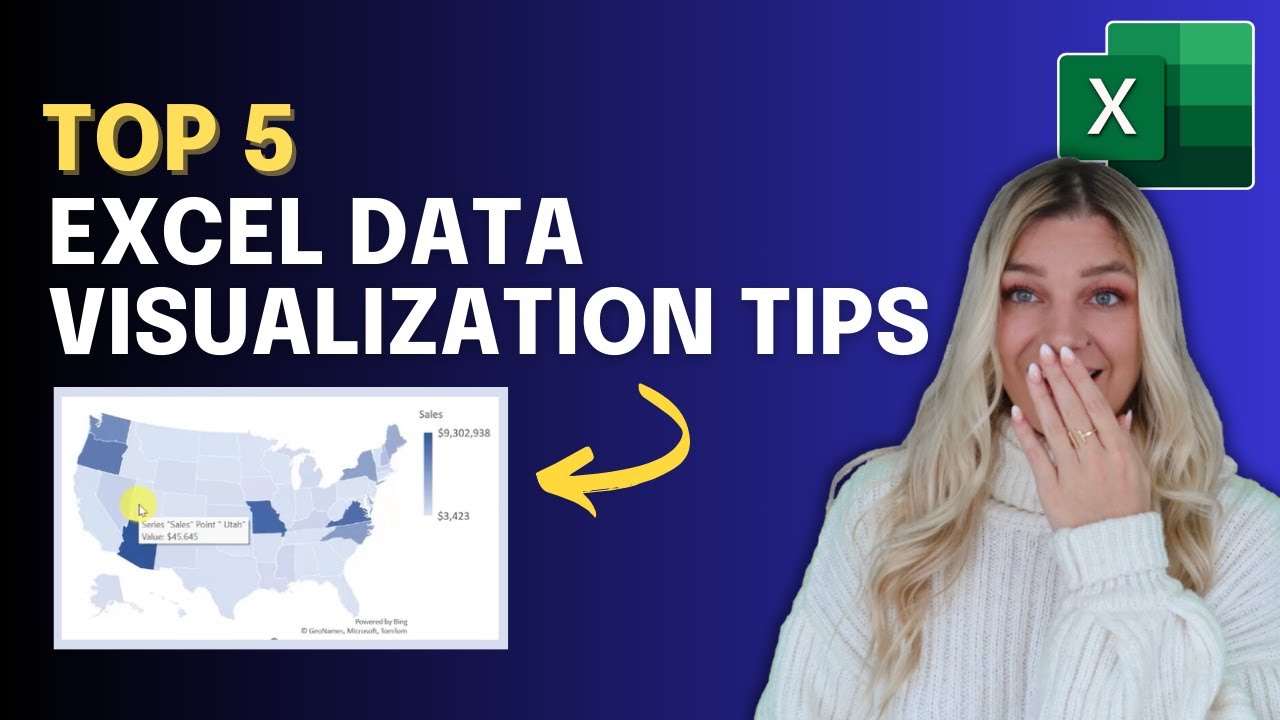
TOP 5 Excel Data Visualization Tips (Become a Data Visualization PRO)

7 Effective Tips for Presenting Data at Work!
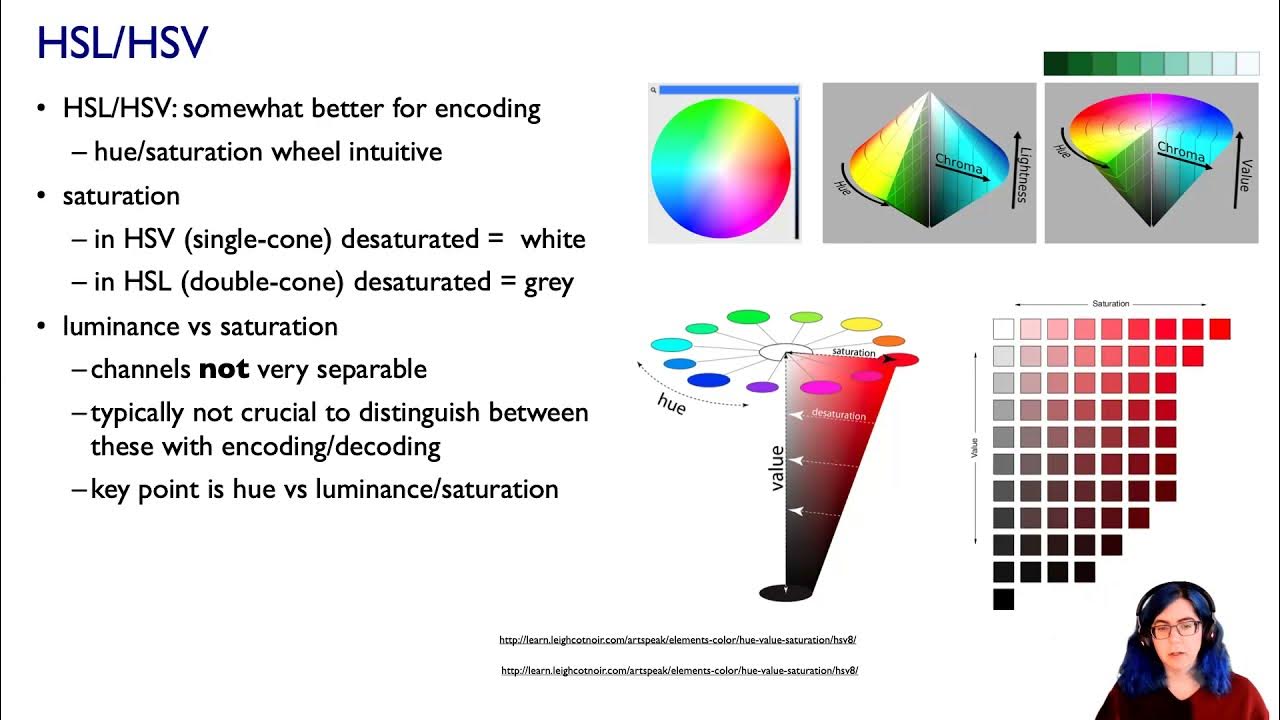
Color (Ch 10) III, Visualization Analysis & Design, 2021
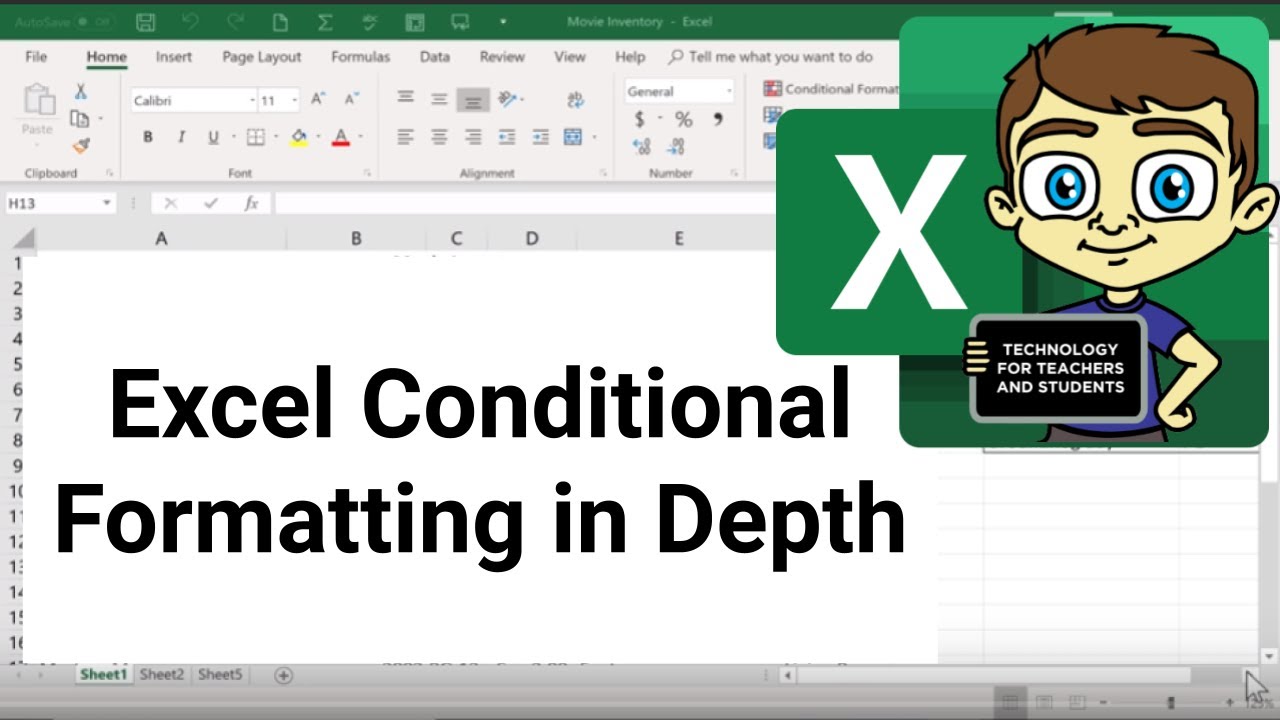
Excel Conditional Formatting in Depth
5.0 / 5 (0 votes)