Predictive Analytics Process & Tools
Summary
TLDRThis video explores the predictive analytics process, detailing its seven-step approach: defining objectives, understanding and preparing data, developing models, deploying them, monitoring performance, and analyzing results. It highlights the growing demand across industries, particularly in marketing and finance, for enhancing customer understanding and operational efficiency. While predictive analytics offers significant opportunities, such as improving customer service and identifying patterns, it also presents challenges, including high costs and skills gaps. The video concludes by introducing various tools available for predictive analytics, emphasizing the importance of business knowledge and critical thinking in the field.
Takeaways
- 😀 Predictive Analytics is increasingly utilized across various industries, especially in marketing and finance, to enhance decision-making processes.
- 📊 The Predictive Analytics process consists of seven steps: defining objectives, understanding data, preparing data, developing models, deploying models, monitoring performance, and analyzing results.
- 🔍 Defining clear business objectives is crucial for successful Predictive Analytics and requires deep domain knowledge.
- 📈 Data preparation is essential; cleansing and structuring data can significantly impact the accuracy of analyses and outcomes.
- 🧠 Experienced data scientists thrive in the model development phase, where they select and create the appropriate predictive models.
- 💡 Common methods for Predictive Analytics include linear models, decision trees, neural networks, and genetic algorithms, among others.
- 📉 Monitoring model performance ensures accuracy and allows for adjustments based on observed results.
- 🚀 Predictive Analytics offers numerous opportunities, such as improving customer service, predicting employee performance, and managing risks effectively.
- ⚠️ Challenges include high costs for acquiring skilled analysts, potential over-reliance on software outputs, and gaps in understanding business needs.
- 🛠️ Key skills for success in Predictive Analytics include business knowledge, critical thinking, and familiarity with data sources, rather than just software expertise.
Q & A
What are the main consumer segments for Predictive Analytics?
-The main consumer segments include marketing professionals (about a third), banks and financial institutions (another third), and various industries using Predictive Analytics for diverse purposes like sales forecasting and sentiment analysis.
Why is Predictive Analytics in high demand?
-Predictive Analytics is in high demand due to its ability to enhance customer understanding, improve operational efficiencies, and drive product development and innovation.
What are the seven steps of the Predictive Analytics process?
-The seven steps are: 1) Define objectives, 2) Understand the data, 3) Prepare the data, 4) Develop models, 5) Deploy models, 6) Monitor performance, and 7) Analyze results.
What is the importance of defining objectives in Predictive Analytics?
-Defining objectives is crucial as it sets the business goals that the analysis aims to achieve, requiring deep domain knowledge to ensure relevance and effectiveness.
What challenges does Predictive Analytics face?
-Challenges include high costs of acquiring data analytics expertise, reliance on software outputs without understanding their foundations, and a lack of skills and knowledge about the business's potential.
What skills are most important for performing Predictive Analytics effectively?
-The most important skills are knowledge of the business, critical thinking, and understanding of source data, supplemented by training in Predictive Analytics and quantitative disciplines.
What types of predictive models are commonly used in Predictive Analytics?
-Common predictive models include linear models, logistic regression, decision trees, neural networks, and survival analysis, among others.
How does one prepare data for Predictive Analytics?
-Preparing data involves cleansing, inspecting, and transforming it to structure it properly for analysis, ensuring accuracy and removing any duplicates or outdated information.
What are some opportunities that Predictive Analytics can provide organizations?
-Opportunities include enhancing customer service, acquiring profitable customers, predicting employee performance, increasing sales, managing risks, and optimizing resources.
What tools are recommended for Predictive Analytics, and what are their functions?
-Recommended tools include R for statistical analysis, RapidMiner for machine learning, Tableau Public for data visualization, Anaconda for Python-based analysis, and Weka for machine learning and visualization.
Outlines
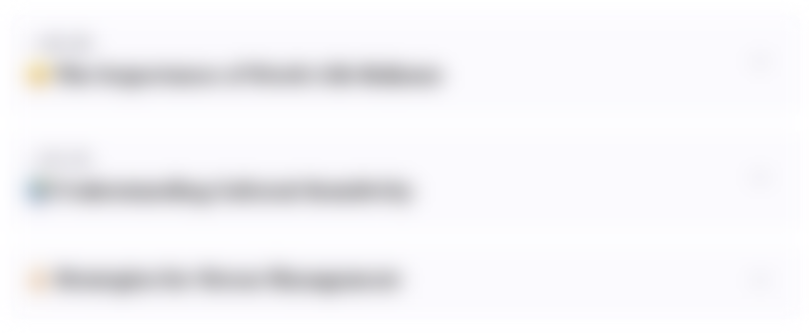
Этот раздел доступен только подписчикам платных тарифов. Пожалуйста, перейдите на платный тариф для доступа.
Перейти на платный тарифMindmap
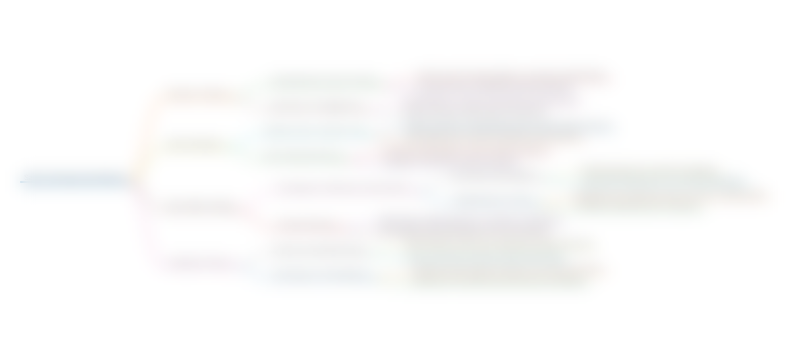
Этот раздел доступен только подписчикам платных тарифов. Пожалуйста, перейдите на платный тариф для доступа.
Перейти на платный тарифKeywords
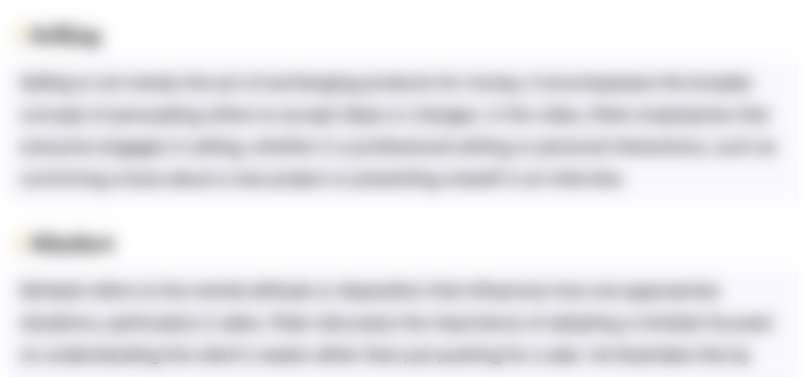
Этот раздел доступен только подписчикам платных тарифов. Пожалуйста, перейдите на платный тариф для доступа.
Перейти на платный тарифHighlights
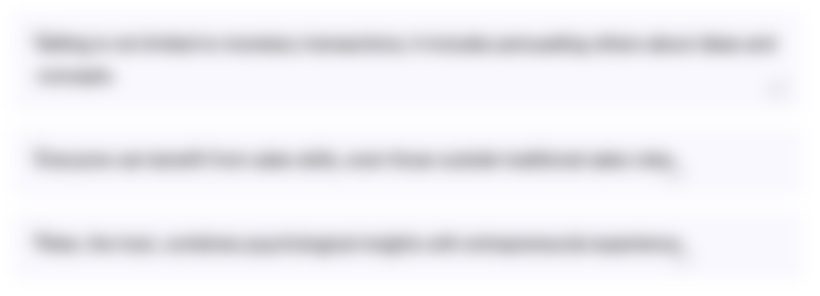
Этот раздел доступен только подписчикам платных тарифов. Пожалуйста, перейдите на платный тариф для доступа.
Перейти на платный тарифTranscripts
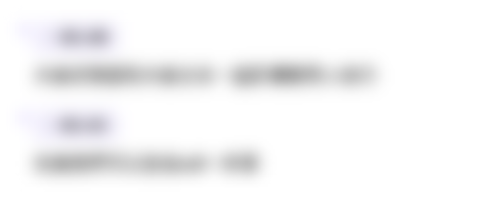
Этот раздел доступен только подписчикам платных тарифов. Пожалуйста, перейдите на платный тариф для доступа.
Перейти на платный тарифПосмотреть больше похожих видео
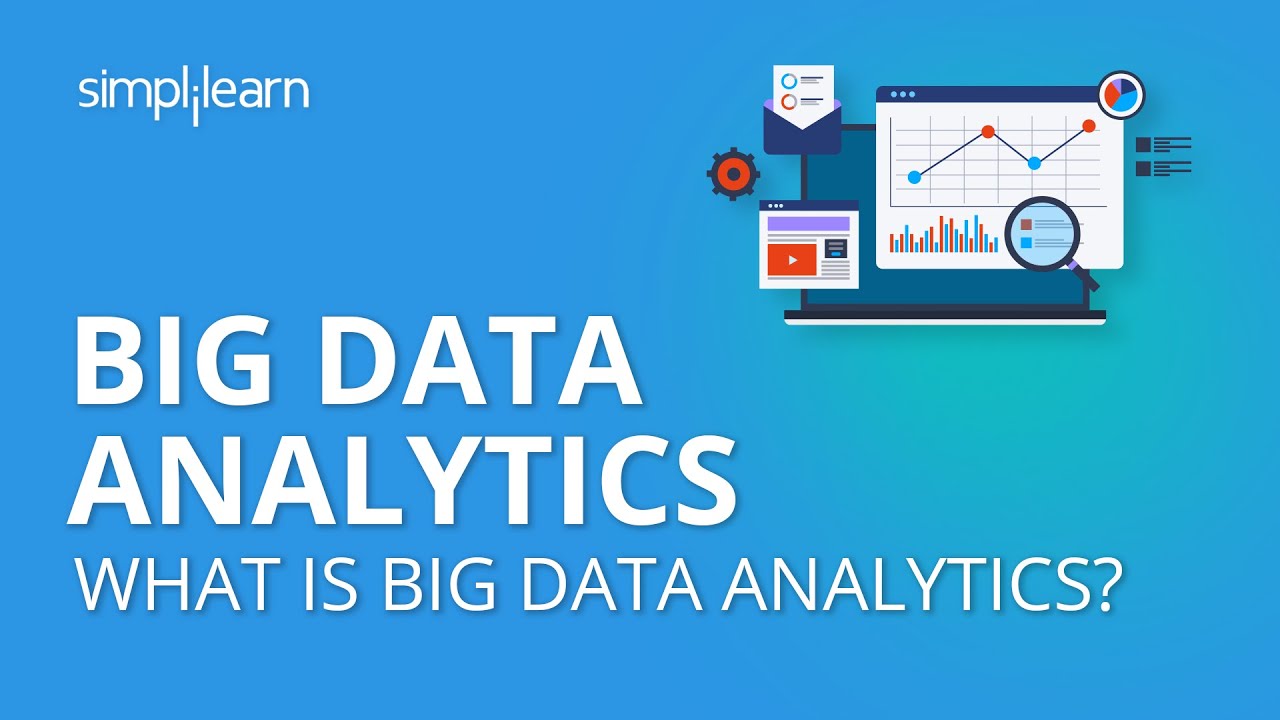
Big Data Analytics | What Is Big Data Analytics? | Big Data Analytics For Beginners | Simplilearn
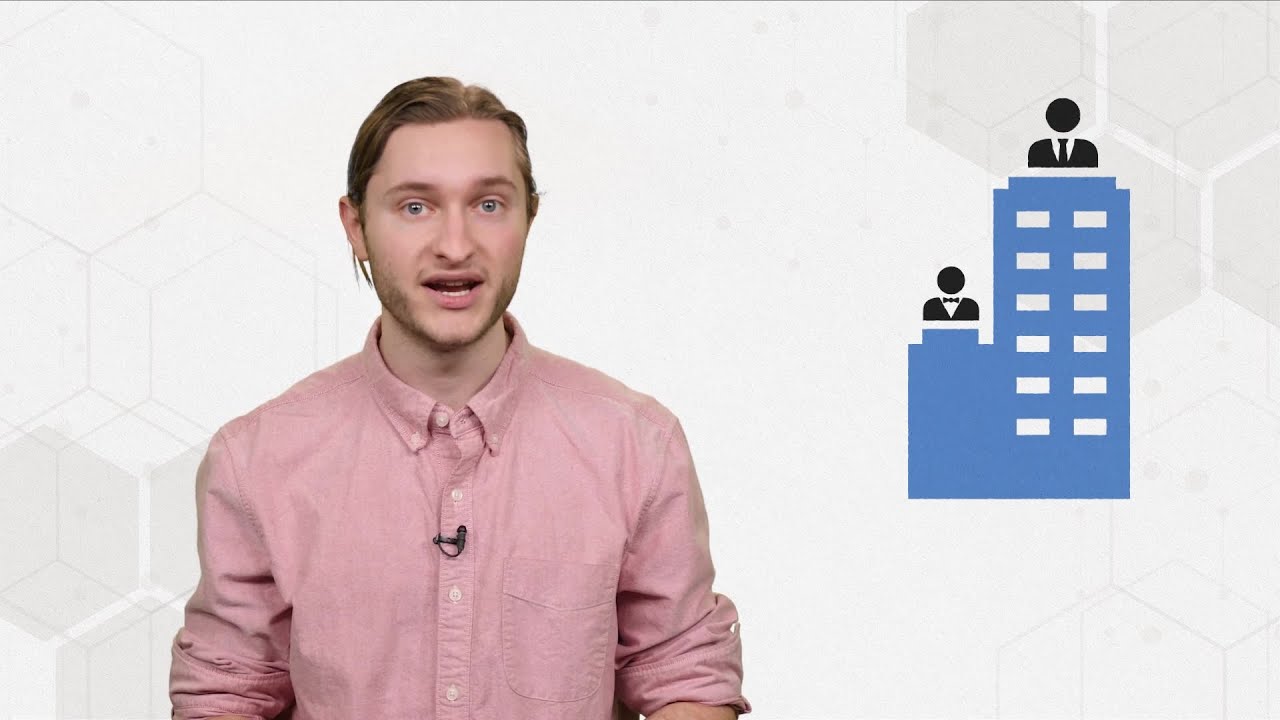
What is Business Intelligence (BI) and Why is it Important? Updated for 2024
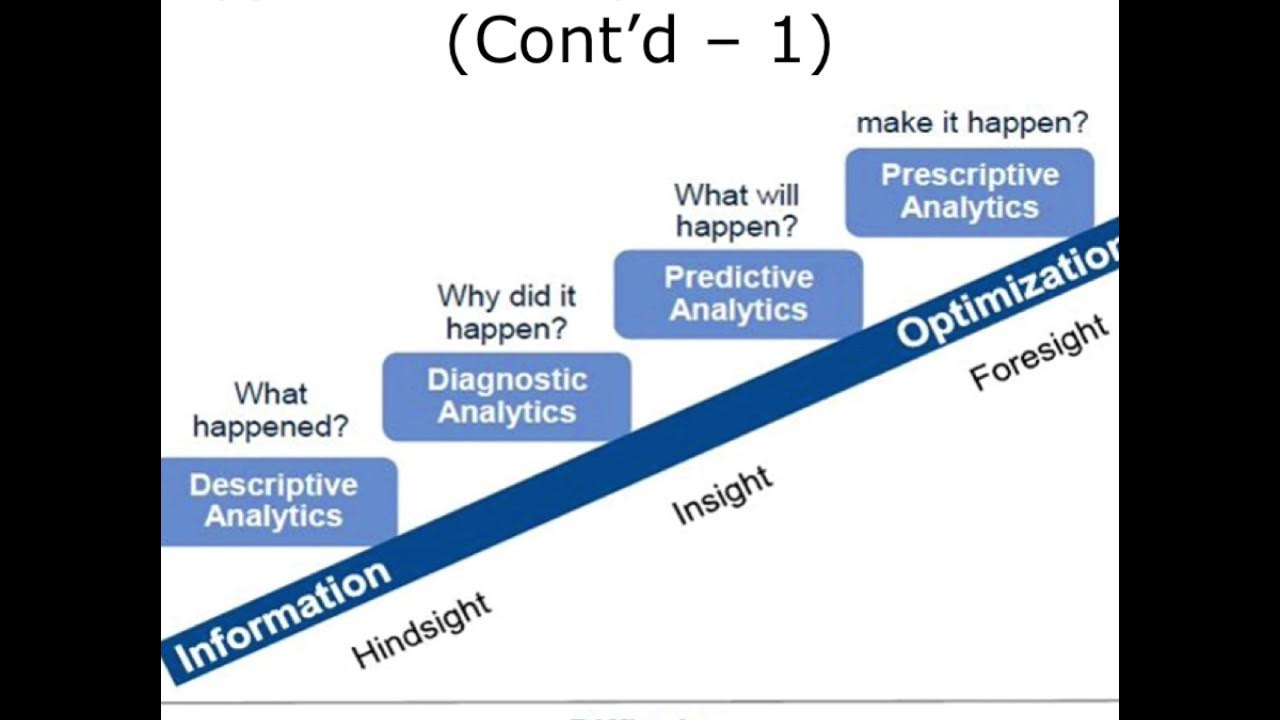
Overview of Health Care Data Analytics

Big Data Analytics Explained | What Is Big Data Analytics? | Big Data Tutorial | Simplilearn
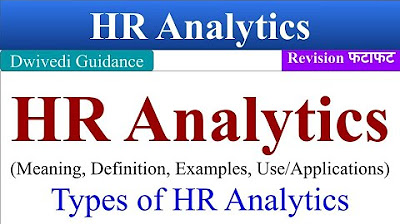
HR Analytics, hr analytics meaning, hr analytics notes, hr analytics example, types of hr analytic
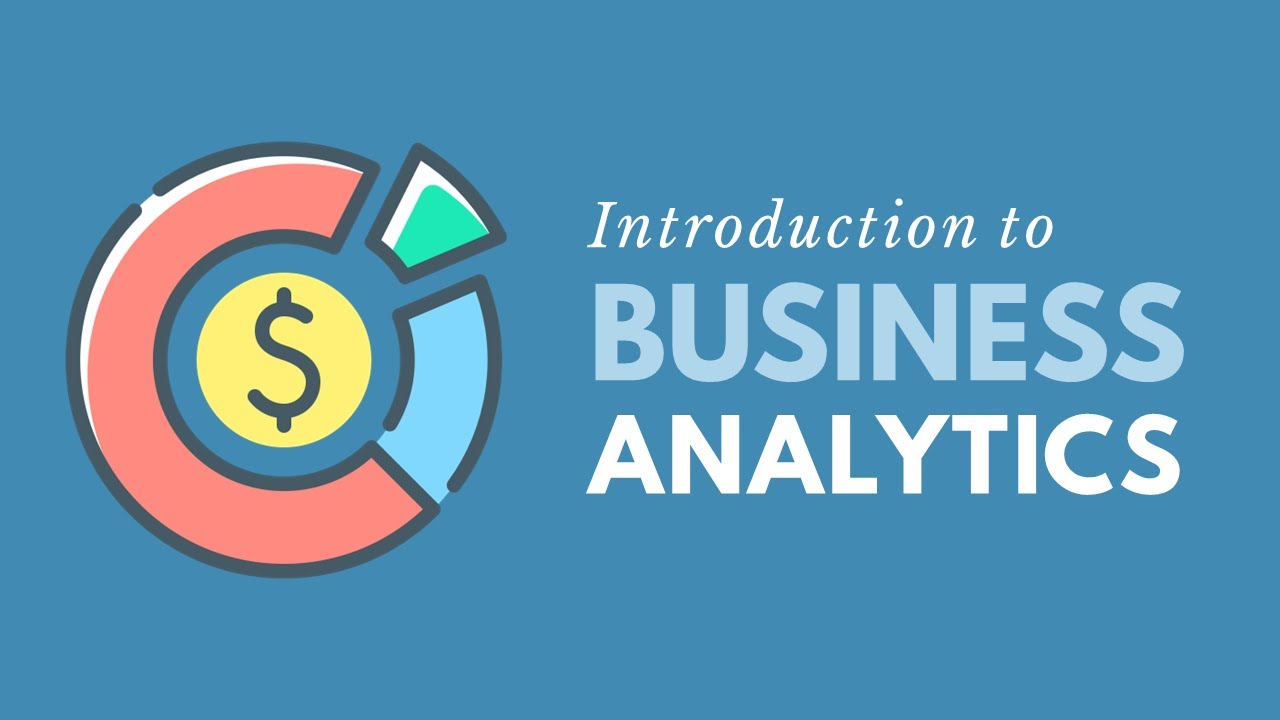
Introduction to Business Analytics (Updated Edition)
5.0 / 5 (0 votes)