AI Pioneer Shows The Power of AI AGENTS - "The Future Is Agentic"
Summary
TLDRDr. Andrew Ng, a prominent figure in AI and co-founder of Google Brain, delivered a talk at Sequoia Capital, emphasizing the transformative potential of AI agents. Ng highlighted the importance of an 'agentic workflow' in AI, where multiple agents with distinct roles collaborate and iterate on tasks, leading to superior outcomes over traditional single-model approaches. He discussed the effectiveness of this method using benchmarks like the HumanEval coding test, where an agentic workflow with GPT 3.5 outperformed GPT-4 with zero-shot prompting. Ng also touched on key agentic design patterns, including reflection, tool use, planning, and multi-agent collaboration, which are set to expand the scope of AI capabilities. He concluded by advocating for patience with AI agents, as their iterative processes may require longer wait times for more refined results, and expressed optimism about the future of AI with the advent of faster inference speeds.
Takeaways
- 🧠 Dr. Andrew Ng, a leading AI expert, is very optimistic about the future of agents in AI, emphasizing their iterative and collaborative nature.
- 📈 Agents, when compared to non-agentic workflows, can produce better results through a process of planning, iteration, and collaboration.
- 🤖 The concept of 'reflection' in agents allows language models to review and improve their own outputs, leading to higher quality results.
- 🛠️ 'Tool use' is a significant feature where agents can utilize predefined tools to perform specific tasks, enhancing their capabilities.
- 📈 Sequoia, a renowned venture capital firm, has a portfolio representing over 25% of the NASDAQ's total value, highlighting their successful tech investments.
- 🔍 'Planning' and 'multi-agent collaboration' are emerging as robust technologies that can lead to surprising and effective outcomes.
- 📝 An agentic workflow can outperform even the latest language models like GPT-4 when used in conjunction with GPT-3.5.
- 🚀 The potential of agentic workflows is expected to expand the scope of tasks AI can perform, possibly leading to significant productivity boosts.
- ⏱️ Fast token generation is crucial for agentic workflows, as it allows for more iterations and quicker responses in complex tasks.
- 🔗 The use of multi-agent systems, where different agents play different roles, can lead to better performance and more reliable outcomes.
- 🌟 The path to Artificial General Intelligence (AGI) is viewed as a journey, and agentic workflows are seen as a step forward in this long-term goal.
Q & A
Who is Dr. Andrew Ng and why is he considered a leading mind in artificial intelligence?
-Dr. Andrew Ng is a computer scientist known for co-founding and heading Google Brain, being the former Chief Scientist at Baidu, and his significant contributions to the field of AI. He has studied at prestigious institutions like UC Berkeley, MIT, and Carnegie Mellon, and he co-founded Coursera, an online learning platform offering a wide range of courses in computer science and other subjects.
What is the significance of Sequoia in the context of Silicon Valley venture capital firms?
-Sequoia is one of the most legendary venture capital firms in Silicon Valley, known for its ability to pick technological winners. Their portfolio of companies represents more than 25% of the total value of the NASDAQ, which is an incredible statistic considering the vast number of companies listed on the exchange.
What is the difference between a non-agentic workflow and an agentic workflow in the context of using language models?
-A non-agentic workflow involves using a language model to generate an answer to a prompt in one go, without any back-and-forth interaction. An agentic workflow, on the other hand, is iterative and involves multiple agents with different roles working together, revising, and iterating on a task to achieve the best possible outcome.
How does the agentic workflow improve the results of tasks compared to a non-agentic approach?
-The agentic workflow improves results by allowing multiple agents, each with different roles and tools, to work together and iterate on a task. This collaborative and iterative process is more aligned with how humans work, leading to higher quality outcomes.
What is the 'reflection' tool in the context of agentic workflows?
-Reflection is a tool used in agentic workflows where a large language model is prompted to review and find ways to improve its own output. This self-evaluation and iterative improvement process can significantly enhance the performance of the language model.
How does tool use enhance the capabilities of language models?
-Tool use allows language models to leverage pre-existing, hardcoded code for specific functions, such as web scraping or stock information retrieval. By providing these tools to the language model, it can use them as needed, enhancing its capabilities without the need to generate new code from scratch.
What is the concept of planning in the context of AI agents?
-Planning in AI agents involves giving the language model the ability to think through steps more slowly and methodically, often by explaining its reasoning step by step. This forced planning can lead to more thoughtful and accurate results.
What are the benefits of multi-agent collaboration in AI workflows?
-Multi-agent collaboration allows different agents, potentially powered by different models, to work together, each contributing their specialized skills or perspectives. This collaboration can lead to more robust and higher quality outcomes compared to a single-agent approach.
How does the concept of 'fast token generation' relate to agentic workflows?
-Fast token generation is important for agentic workflows because these workflows often involve multiple iterations. The ability to generate more tokens quickly, even from a slightly lower quality language model, can lead to better results due to the increased number of iterations possible.
What are some of the challenges in implementing agentic workflows?
-One of the challenges is that agents can be finicky and may not always work as expected. However, the iterative nature of agentic workflows allows for recovery from earlier failures. Another challenge is adjusting to the slower pace of response, as agentic workflows may require waiting for minutes or even hours for the best results.
How does Dr. Andrew Ng's talk relate to the future of AI and the concept of AGI (Artificial General Intelligence)?
-Dr. Ng's talk emphasizes the potential of agentic workflows in pushing the boundaries of what AI can do, which aligns with the pursuit of AGI. While AGI is a long-term goal, the advancements in agentic workflows, tool use, and multi-agent collaboration could contribute to incremental progress towards achieving more general intelligence in AI systems.
What are some of the key takeaways from Dr. Ng's talk regarding the future applications of AI?
-Dr. Ng suggests that the set of tasks AI can perform will expand dramatically due to agentic workflows. He also highlights the importance of fast token generation and the potential for productivity boosts in various workflows. Additionally, he emphasizes the need for patience and a shift in expectations regarding the speed of AI responses, especially when leveraging agentic reasoning.
Outlines
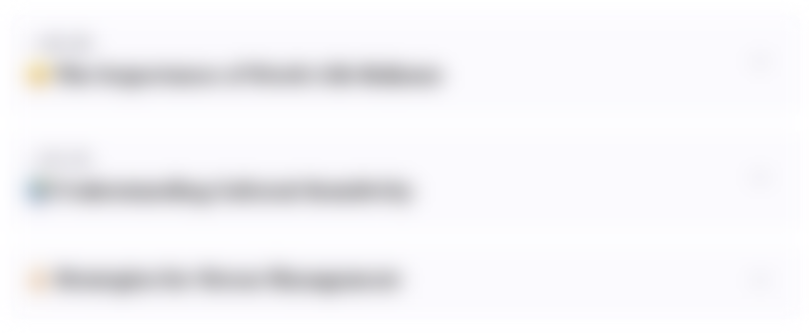
Этот раздел доступен только подписчикам платных тарифов. Пожалуйста, перейдите на платный тариф для доступа.
Перейти на платный тарифMindmap
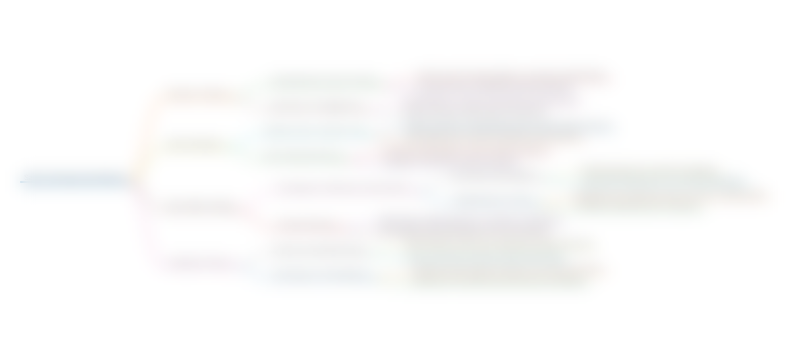
Этот раздел доступен только подписчикам платных тарифов. Пожалуйста, перейдите на платный тариф для доступа.
Перейти на платный тарифKeywords
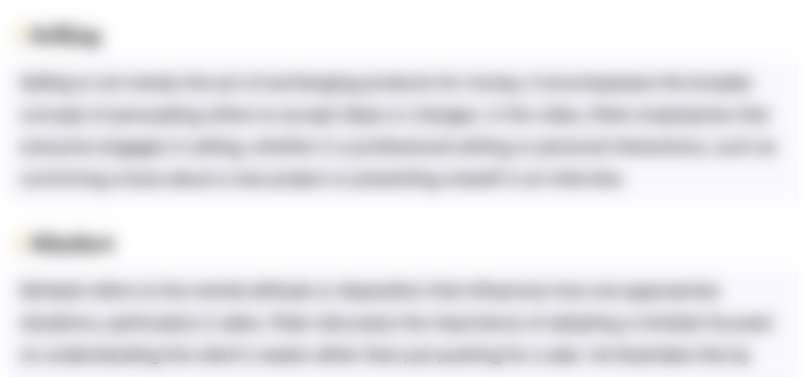
Этот раздел доступен только подписчикам платных тарифов. Пожалуйста, перейдите на платный тариф для доступа.
Перейти на платный тарифHighlights
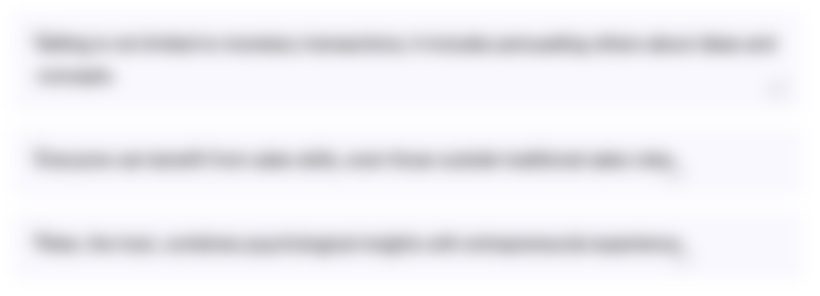
Этот раздел доступен только подписчикам платных тарифов. Пожалуйста, перейдите на платный тариф для доступа.
Перейти на платный тарифTranscripts
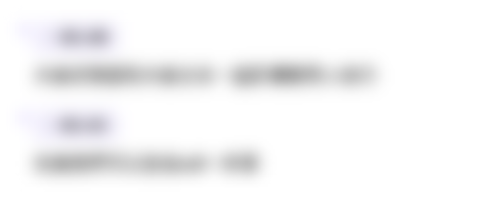
Этот раздел доступен только подписчикам платных тарифов. Пожалуйста, перейдите на платный тариф для доступа.
Перейти на платный тарифПосмотреть больше похожих видео

Think Cultural Health Case Study: Cultural and religious beliefs
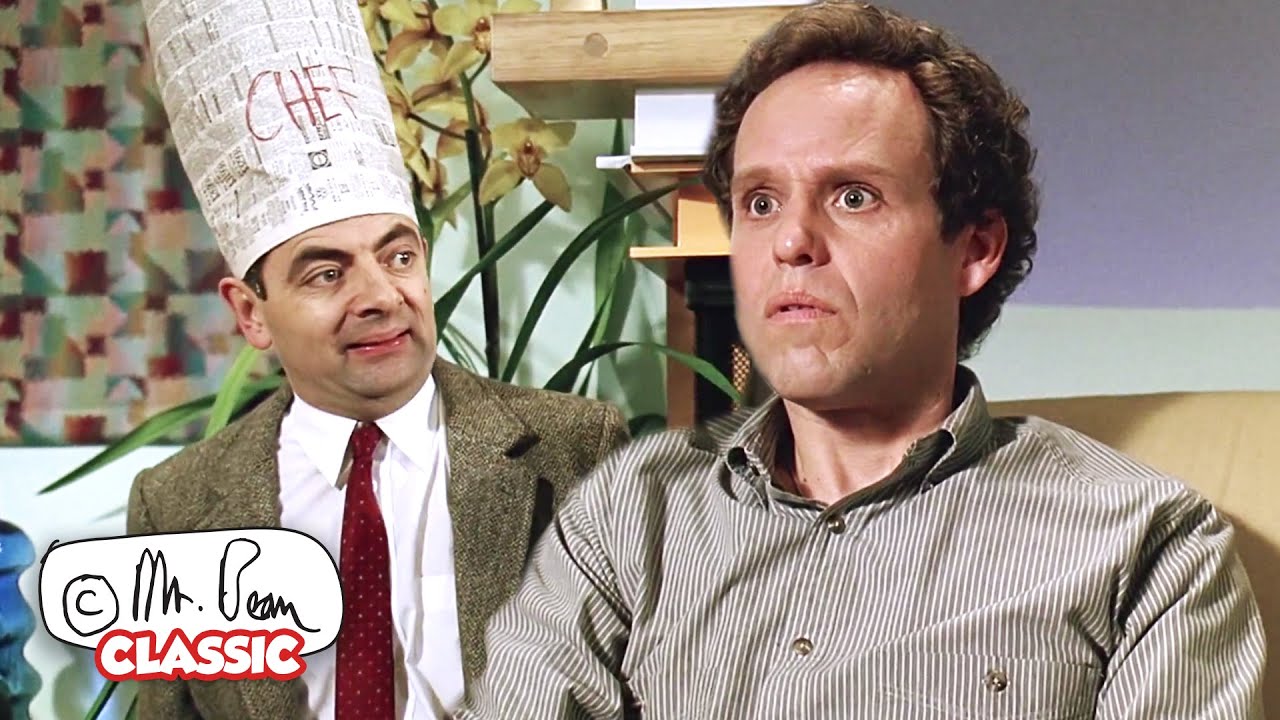
Mr Bean Cooking the CHRISTMAS Dinner | Mr Bean: The Movie | Classic Mr Bean
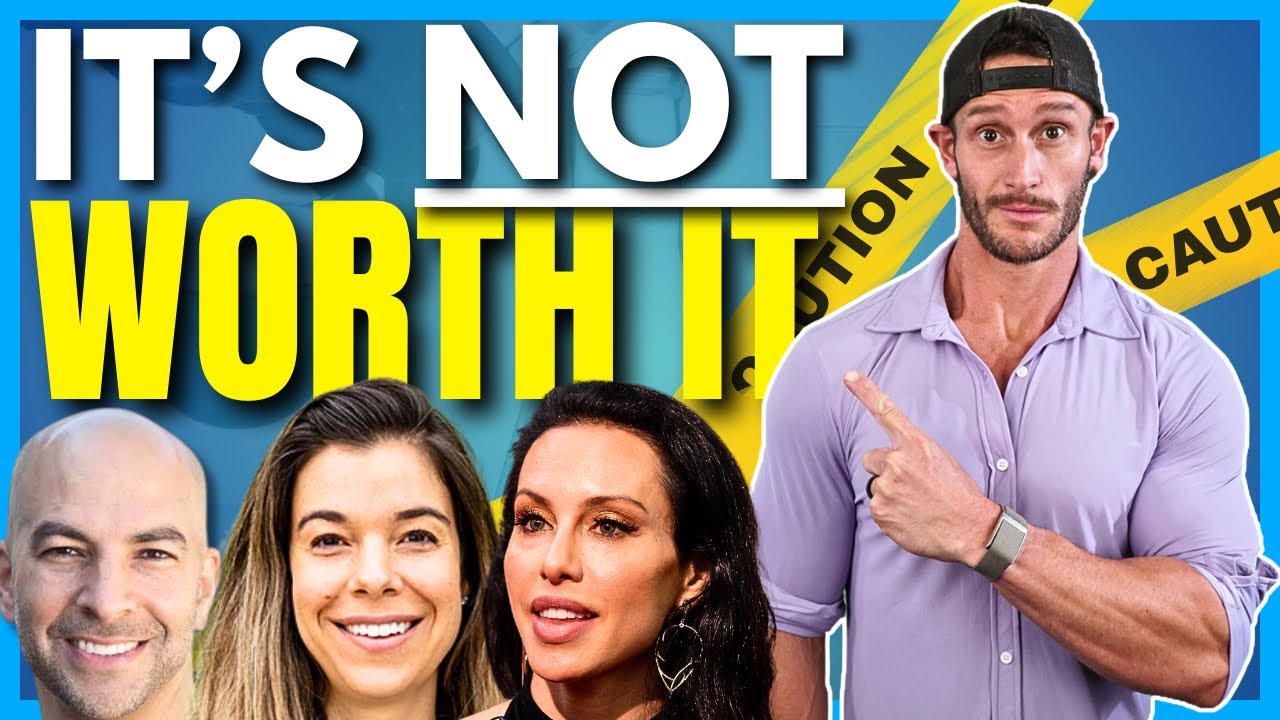
Why Experts are Warning Against Fasting - Dr. Peter Attia, Dr. Rhonda Patrick, Dr. Gabrielle Lyon

What if AI debated ABORTION?
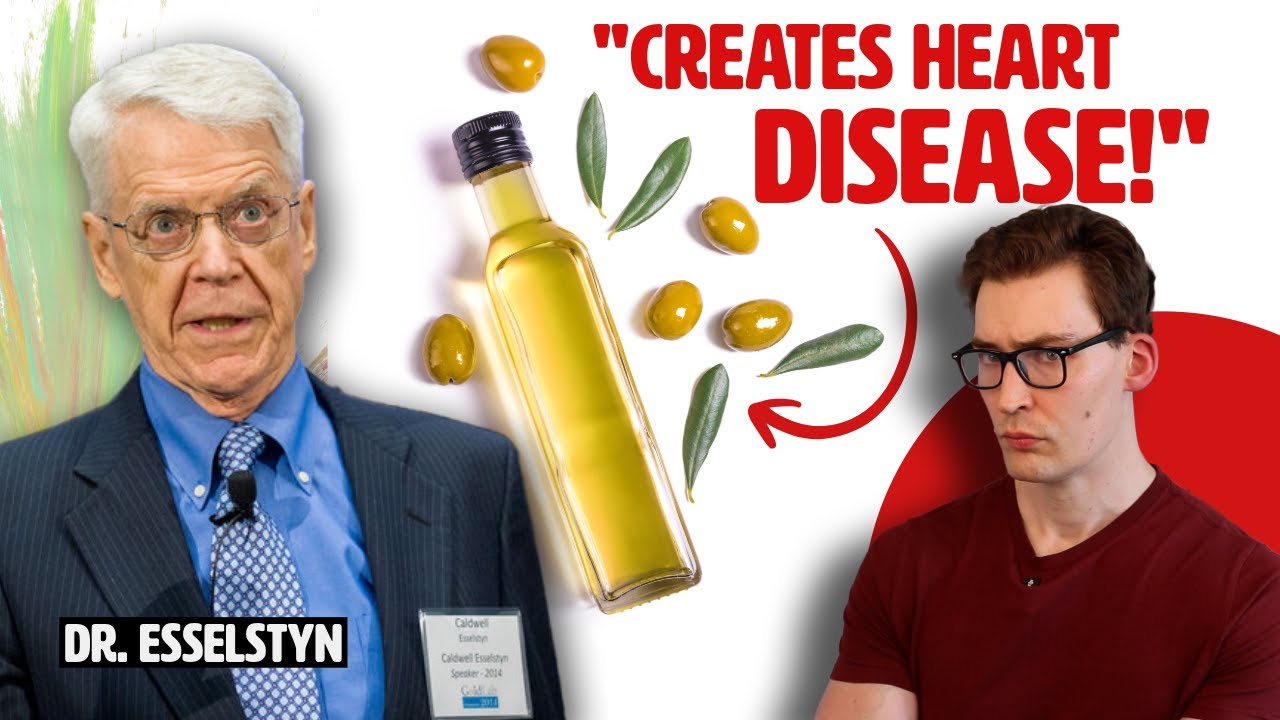
Dr. Esselstyn: “Mediterranean Diet (and Olive Oil) creates Heart Disease!”

Field Study 1-Learning Episode 1: The School Environment
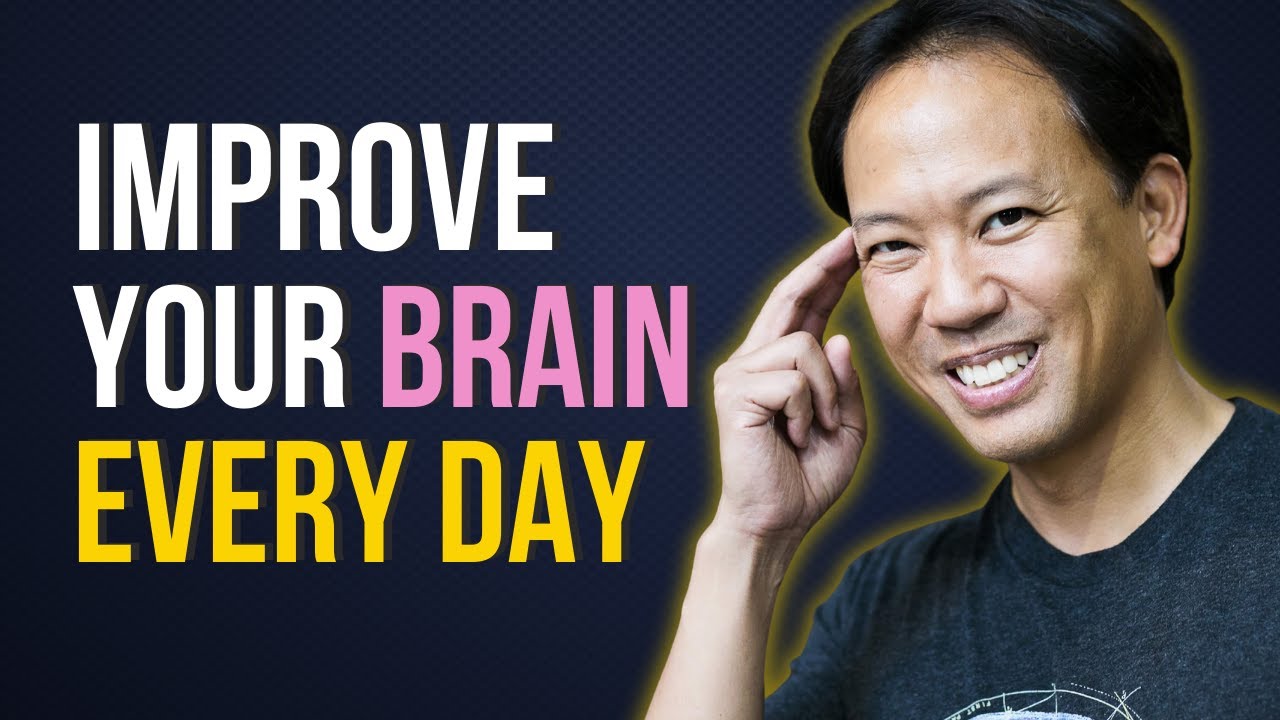
Daily Habits for Better Brain Health | Jim Kwik & Dr. Daniel Amen
5.0 / 5 (0 votes)