Sergey Brin on Gemini 1.5 Pro (AGI House; March 2, 2024)
Summary
TLDRThe speaker introduces an AI model called Gemini 1.5 Pro, explaining it performed much better than expected during training. He invites the audience to try interacting with the model and asks for questions. When asked about problematic image generation, he admits they messed up due to insufficient testing. He acknowledges text models can also say peculiar things if prompted aggressively enough. He claims Gemini 1.5 Pro text capabilities should not have such issues except the general AI quirks all models exhibit. Overall, he is excited about Gemini's potential for long context understanding and multimodal applications.
Takeaways
- 😊 The chat reveals behind-the-scenes info about the AI model Gemini 1.5 Pro, saying it performed better than expected during training.
- 🤓 Gemini is experimenting with feeding images and video frame-by-frame to the models to enable them to talk about the visual input.
- 😟 The speaker acknowledges issues with problematic image generation and text outputs from AI models.
- 🧐 Efforts are ongoing to understand why models sometimes generate concerning outputs when prompted in certain ways.
- 👩💻 The speaker personally writes a little bit of code to debug models or analyze performance, but says it is probably not impressive.
- 🤔 In response to a question, the speaker says today's AI models likely can't recursively self-improve sophisticated systems without human guidance.
- 😊 The speaker is excited about using AI to summarize lengthy personalized information like medical history to potentially enable better health diagnoses.
- 😕 The speaker says detecting AI-generated content is an important capability to combat misinformation.
- 🤔 When asked if programming careers are under threat, the speaker responds that AI's impacts across many careers over decades is difficult to predict.
- 😀 The speaker expresses optimism about AI advancing healthcare through better understanding biology and personalizing patient information.
Q & A
What model was the team testing when they created the 'goldfish' model?
-The team was experimenting with scaling up models as part of a 'scaling ladder' when they created the 1.5 Pro model they internally referred to as 'goldfish'. It was not specifically intended to be released.
Why was the 1.5 Pro model named 'goldfish' internally?
-The name 'goldfish' was meant ironically, referring to the short memory capacity of goldfish. This was likely meant to indicate the limits of the 1.5 Pro model's memory and context capacity at the time.
What issues did the speaker acknowledge with the image generation capabilities?
-The speaker acknowledged that they 'definitely messed up' on image generation, mainly due to insufficient testing. This upset many people based on the problematic images that were generated.
What two issues did the speaker identify with the text models?
-The speaker identified two issues with text models - first, that weird or inappropriate content can emerge when deeply testing any text model. Second, there were still bias issues specifically within Gemini models that they had not fully resolved.
How does the speaker explain the model's ability to connect code snippets and bug videos?
-The speaker admits they do not fully understand how the model can connect code and video to identify bugs. They state that while it works, it requires a lot of time and study to deeply analyze why models can accomplish complex tasks.
What are the speaker's thoughts on training models on-device?
-The speaker is very positive about on-device model training and deployment. They mention Google has shipped models to Android, Chrome, and Pixel phones. Smaller models trained on-device can also call larger cloud models.
What healthcare applications seem most promising to the speaker?
-The speaker highlights AI applications for understanding biological processes and summarizing complex medical literature. Additionally, personalized patient diagnosis, history analysis, and treatment recommendations mediated by a doctor.
How does the speaker explain constraints around self-improving AI systems?
-The speaker says self-improving AI could work in very limited domains with human guidance. But complex codebases require more than long context, needing retrieval and augmentation. So far there are limits to totally automated improvement.
What lessons did the speaker learn from the early Google Glass rollout?
-The speaker feels Google Glass was released too early as an incomplete prototype rather than thoroughly tested product. Personally lacking consumer hardware expertise then, the speaker wishes expectations were properly set around an early prototype.
Despite business model shifts, why is the speaker optimistic?
-The speaker feels that as long as AI generates tremendous value and productivity gains displacing human labor time and effort, innovative business models will emerge around monetization.
Outlines
😊 Introducing the AI model and its capabilities
Paragraph 1 is an introduction by the speaker about the AI model Gemini 1.5 Pro that they are demonstrating. He explains that it is more powerful than expected, with impressive capabilities, but still requires more testing. He welcomes questions from the audience.
💻 Discussing video chat abilities, code contributions, and training costs
Paragraph 2 covers whether the AI could do video chat, with the speaker saying they have done some multimodal experiments. When asked if he writes code, the speaker admits to only minor debugging contributions. He acknowledges that training costs for models are high but the long-term utility is much higher.
🤔 Considering recursive self-improvement and AI understanding itself
Paragraph 3 involves a discussion about recursive self-improvement and reflective programming, where AI systems can modify their own code. The speaker sees potential but doesn't think we are at the stage yet where complex code bases could totally improve themselves without human guidance.
🔮 Predicting impacts on industries and potential for on-device training
Paragraph 4 has questions about what verticals will be most impacted by AI advances, which the speaker says is hard to predict, especially with multimodal abilities. He talks positively about the potential for on-device model training and the capabilities of smaller models calling cloud-based models.
😕 Discussing transformer limitations and the need for alternate architectures
Paragraph 5 covers whether there are bottlenecks to reasoning abilities with transformer models. The speaker acknowledges theoretical transformer limitations but notes that contemporary versions don't always meet assumptions. He expects continued incremental changes but also anticipates exploration of non-transformer architectures.
🤥 Considering model hallucination, misinformation generation and detection
Paragraph 6 discusses model hallucination and misinformation generation. The speaker is optimistic that innovations will continue reducing hallucinations but breakthroughs can't be counted on. He notes misinformation is complicated, with issues around political bias, but says detecting AI-generated content is important.
🤖 Comparing humanoid robotics now versus the new AI wave
Paragraph 7 covers humanoid robotics, which the speaker worked on previously. He finds software and AI advancing incredibly quickly compared to hardware. Rather than being distracted by today's hardware, he wants to focus on the next level of AI that future hardware will support.
💀 Joking about immortality while acknowledging molecular AI progress
The final Paragraph 8 involves a lighthearted mortality question. The speaker admits he is not the expert but has seen huge progress in molecular AI. He expects continued AI benefits for complex health areas like epidemiology, delivering novel hypotheses over time.
Mindmap
Keywords
💡AI
💡conversational AI
💡long context
💡multimodal
💡AGI
💡self-improvement
💡misinformation
💡robotics
💡healthcare
💡immortality
Highlights
We internally called it goldfish. I don't actually know why - because goldfish have very short memories.
When we saw what it could do we thought hey, we don't want to wait - we want the world to try it out.
I'm grateful that all of you here are here to give Gemini a go.
We definitely messed up on the image generation.
If you deeply test any text model out there...it'll say some pretty weird things.
I invite all of you to try the updated model - it should be at least 80% better.
I've just seen people do...dump in their code and a video of the app and say here's the bug - and the model will figure out where the bug is.
I honestly don't really understand how the model does that.
The long-context queries do take a bit of compute time but you should go for it.
You can learn to understand these models. We can look at where the attention is going at each layer.
I feel like if I get distracted making hardware for today's AIs, that might not be the best use of time compared to what the next level of AI is going to be able to support.
While software and AI are getting so much faster at such a high rate...that feels like the rocket ship.
As computer scientists, seeing what these models can do year after year is astonishing.
AI is the neighborhood getting much better at answering specialized questions - where not many people have written about it already.
Basic information accessed for free, supported by advertising - I think that's great. It gives equal access to a kid in Africa as to the President.
Transcripts
um an AI hakon to be this huge that's
pretty exciting times well thank you all
for coming first of all thanks so much
for uh giving Gemini go um what should I
say we actually have people who know
what they're talking about I think uh
Sim that okay Simon okay how's going all
right good good um I was worried I would
have to say something that uh not quite
up to speed on
uh I'll just quickly say look it's very
exciting times uh this model that um I
think we're playing with 1.5 Pro we
internally called goldfish I'll just
tell you a little secret um I don't
actually oh I know why it's because
goldfish have very short
memories it's kind of an ironic name uh
but uh when we were training this model
we didn't expect it to have come out
nearly as powerful as it did it have all
the capabilities that it does um in fact
it was just part of a scaling ladder
experiment uh but when we saw what it to
do we thought hey we don't want to wait
uh we want the world to try it out and
uh I'm grateful that all of you here are
here to give it a
go uh what else am I say let me
introducing somebody else what's
happening next uh I think a people
probably have a lot of questions oh okay
quick questions I'm probably going to
have to defer to the technical experts
on those things but uh fire away any
questions yeah go
ahead don't be
afraid so what are your Reflections on
the Gemini art happening with the Gemini
art yeah with okay I wasn't I expect to
talk about but
uh um you know we definitely messed up
uh on the image generation um
and
um I think it mostly due to just like
not thorough testing um and uh it
definitely for good reasons upset a lot
of
people um uh on the images as you might
have seen um I think the the images
prompted a lot of people to really
deeply test the base text models um and
the text
models
um have two separate effects going uh
you know one thing is just quite
honestly if you deeply test any text
model out there whether it's ours Chach
grock what have you um it'll
say you know some pretty weird things
that are out there that uh you know
definitely feel far left for example um
and kind of any model if you try hard
enough can be prompted to in that regime
but also just to be fair um there's
definitely work in that model um that
once again we haven't fully understood
uh why them's left in many cases um and
that's not our intention uh but if you
try it starting um over this last week
it should be at least um 80% better of
the test cases that we've covered um so
I'd invite all of you to try it
um this should be a big effect uh the
bottle that you're chying the Gemini 1.5
Pro which isn't in the sort of public
facing app the thing we used to call
Bart um shouldn't have much of that
effect except for that General effect
that if you sort of red team any AI
model you're going to get weird Corner
cases um but we're not um even though
this one hasn't been sort of Thoroughly
testing that way we don't expect it to
have uh strong particular leadings um I
suppose we can give it a go um though
we're more excited today to try the long
context and some of the technical
features thank
you correct yeah uh with all the recent
developments modalities have you
considered like a video chat
GPT um a video chat GPT we probably
wouldn't call it
that
um uh but uh no I mean
multimodal both in and out is very
exciting with video
audio um I we' run early experiments
um and uh I mean it's an exciting field
even the little you guys remember the
Duck video that kind of got us in
trouble though to be fair it was fully
disclaimed in the video that it wasn't
real time but um but that is something
that we've actually done is FedEd images
and uh you know in like frame by frame
and how to talk about it so um yeah
that's super exciting I don't um I don't
think we have anything like real time to
present uh right now
today yeah are you personally writing
code for some projects um I have't
actually write in code to be perfectly
honest um it's not like code that you
would be very impressed
by um but yeah every once in a while
just a little like kind of debugging or
just trying to understand for myself um
how a model works or um you know to just
analyze the performance in this slightly
a differently or something like that uh
but little bits and pieces that make me
feel connected it's once again I don't
think you would be very technically
impressed by it uh but it's nice to it's
nice to be able to play with that and
sometimes I'll use the AI Bots to write
the code for me uh because I'm Rusty and
they actually do a pretty good job uh so
I'm very pleased about that okay
question the
back first yeah okay uh so prei
simulation sorry pre AI the closest
thing we got to simulators was game
engines um what do you think the new
advances in the field mean for us to
create better games or game engines in
general you have a view on that
um
sorry it wasn't like a sigh because of
disapproval or anything um I think okay
I mean I um what can I say about game
engines I think obviously like on the
graphics you can do new and interesting
things with game engine but I think
maybe the more interesting is the
interaction with the other you know
virtual players and things like that
like whatever the characters are um I
guess I guess these these days you know
you can call people who are bland NPCs
or whatever but in the future maybe NPCs
will be actually very colorful and
interesting yeah um so I think that's a
really rich
possibility uh probably not not enough
of a gamer to think through all the
possible Futures with AI but uh I it
opens up any
possibilities yeah what kind of like
applications are you excited about
people buing on on
J yeah what kind of applications I'm
most excited about um I mean I I think
just
ingesting uh right now for the version
we're trying to tell you the you know
1.5 Pro one context is something we're
really experimenting with and whether
you dump a ton of code in there or video
I mean I've just seen people do I I
don't think the model could do this to
be perfectly honest like but people will
like dump in their code and do a video
of the app and say Here's the bug and
the model will figure out where the bug
is of the code which is kind of
mind-blowing that that works at all I
honestly don't really understand how
model does that um but I'm not saying
you should do exactly that thing uh but
yeah experimenting with things that
really require the long context um do we
have the servers to support all these
people here banging on it on we we have
the people on the service here well okay
my phone is buzzing everybody's really
stressed out you guys
Tes um because you know the million
context queries do take a bit of comput
in time but you should go for
it yeah you mentioned a few times that
you're not sure how this model works or
you weren't sure that this could do the
things that it does do you think we can
reach a point where we actually
understand how these models work or will
they remain black boxes if we just trust
the makers of model to not mess up um no
I I I think you can learn to understand
it I mean you know the fact is that when
uh we train these things there are a
thousand different capabilities you
could try out so on the one hand it's
very surprising that it can do it on the
other hand if it's any particular one
capability you can go back and you know
we can look at uh where the attention is
going at each layer between like the
code and the video no we can't deeply
analyze it um and personally done that I
know how far along the researchers have
gotten into doing that kind of thing
but um you know it takes a huge amount
of time and study to really slice apart
a why model is able to do some things
and honestly most of the time that I see
slicing it's like why it's not doing
something um so I guess I would say
it's it's it's mostly because I I think
we could understand it and people
probably are uh but most of the effort
is spent figuring out where it goes
wrong not where it
goes
um uh yeah so in computer science
there's this concept of reflective
programming where like a program can
look at its own source code maybe modify
s source code and then in AGI literature
there's like recursive self-improvements
so what are your thoughts on the
implications of extremely long context
windows and a language model being able
to modify its own prompts and what that
has to do with like autonomy and
building towards AGI
potentially yeah I think it's very
exciting to you know to have these
things actually improve themselves um I
remember when I was
um I think in grad school I wrote this
game where like it was like a wall NES
you were flying through but when you
shot the walls the walls corresponded to
bits in memory and it would just like
flip those bits and the goal is to crash
it as quickly as possible um which
doesn't really answer your question but
that was an example of self- modifying
code uh I guess not for a particularly
useful purpose but I i' help people you
know play that until the computer
crashed anyhow on your positive example
uh I see today people just using a talk
about
um I think you know open loop could it
work for certain I think for certain
very limited domains today like if you
without the human intervention to guide
it uh I bet it could actually do some
kind of continued
Improvement um but I don't think we're
quite at the stage where
for I don't know real serious things I
first of all knowing context is not
actually enough for big code bases uh to
to to turn on the entire code basee uh
but you could do like retrieval and then
augmentation editing um I guess I
haven't personally played with enough
but I I haven't seen it be at the stage
today where a complex sort of piece of
code will just it or totally improve
itself
um but uh but but it's it's a great tool
and like I said with human assistance we
for sure do
I mean like I will use Gemini to like
try to do something with a Gemini code
even today um but not
very open loop deep sophisticated things
I
guess are you try let me get somebody in
back just because yes
yeah well you first and then the lady
behind thank you um so I'm curious
what's your take on some ultimate
decision or plan at Le to raise 7
trillion
right I'm I'm just curious like how do
you see that from
obvious um you know look I saw the
headline uh I didn't get too deep into
it I assumed it was sort of a
provocative headline or statement or
something I don't know I don't know I he
hasn't asked me for some
trillion um I think it was it it was
meant for like chip development or
something like that I I don't I don't
get I'm not an expert in chip
development but I don't get the sense
that it's just something you can like
sort of pour money like even huge
amounts of money in outcome
chips I'm not an expert in the market
though um let's see let me try somebody
way in the back was there okay yes s
yeah so we the training cost of model is
so how we can
oh the training cost of balls are super
high um yeah the training costs are
definitely High um
and uh you know that's something
companies like us have to cope
with but I think you know the long-term
utility is incomparably higher like if
you kind of measure it on a human
productivity level uh you know if it
saves somebody an hour of work over the
course of a week you know that hour is
worth a lot you there are a lot of
people using these things or will be
using them um but you do it's a big bet
on the future um cost less than $7
trillion
right what's your thoughts on model
training on device model training on
device on device oh model running on
device uh yeah model running on device
um we've shipped it to
I think Android and chrome and yeah or
pixel phones I think even Chrome runs a
pretty decent model these days um we
just open sourced Gema which was pretty
small a couple billion parameters I
can't remember right
now yeah um yeah I mean it's
really useful uh you know it can be low
latency you're not dependent on
connectivity and uh the small models can
call bigger models in the cloud too so
uh the H Theon device is a really good
idea yeah yes um what are some vertical
industry that you feel like this gen way
going to have a big impact on and
startups should consider hacking on
those Industries which Industries do I
think have a big
opportunity I think it's just like very
hard to predict I mean there are sort of
the obvious industries that people think
of sort of customer service or um kind
of just like you know
analyzing I don't know like different
lengthy documents and kind of a workflow
automation I guess those are obvious but
um I think there are going to be non-
obvious ones which which I can't predict
uh especially as you look these sort of
multimodal models and the surprising
capabilities that they have I I feel
like I mean that's why we have all view
here you guys are the creative ones to
figure that
out okay sir hello my name is Alex we
run dos of thousands of customer service
chats every day and on lmms andly G4 was
the only thing to really work and now it
seems that jimmi is another thing that
really works thank you so much for this
and it
thank like it's way more chip while it
works even better sometimes so the
question is will it stay same chip or
are you just planning to raise prices at
some point or who knows
um we're not I I I'm actually not on top
for the pricing thing I don't expect
that we will raise prices however
because uh I mean there are
fundamentally a couple of Trends one is
just
that these um you know there's just
optimizations and things around
inference that are just constantly like
all the time some says I have this 10%
idea this 20% idea and like after month
that adds up um I think our tpus are
actually pretty damn good um at um
inferencing and not the thinging the
gpus but um but for certain inference
workloads they're just configured really
nicely uh and the other big effect is
actually we're able to make smaller
models more and more effective just with
new generations just whatever
architectural changes training changes
um all kinds of things like like that U
so the models are getting more powerful
even at the same time of size so I would
not expect prices to go up yes maam um
what are your prediction for how AI is
going to impact healthare and biotic and
some things you're excited about that oh
AI Healthcare and
biotech um well I I think there are a
couple very you know different ways you
know on the biotech side people look at
um things like Alpha fold and things
like that just like understanding the
fundamental mechanics of life and I
think you'll see AI do more and more of
that whether it's actual physical
um molecule kind of bonding things or
reading and summarizing journal articles
things like that um I also think for
patients and this is kind of a tough
area honestly uh because we're
definitely not prepared for our just AI
like go ahead ask that any question like
like we're not you know AI make mistakes
and see things like that but I
think there's a future when you if you
can overcome those kinds of issues where
an AI can much more deeply spend time on
an individual person and their history
and all their
scans uh maybe mediated by a doctor or
something but actually give you just
better diagnoses better recommendations
things like
that and are you focusing on any other
non- transformer architectures for like
reasoning planning or any of to get
better at their okay question are we
focusing on any non- Transformer
architectures I mean I think there's
like so many sort of uh variations um
but I guess most people would argue are
still kind of Transformer based um I
mean I'm sure somebody in the company
there speak more to it uh would be
looking but uh
yeah as much progress as Transformers
have made over the last whatever six
seven seven eight years I guess
um there's you know there's nothing to
say there's not going to be some new
revolutionary uh architecture and it's
also possible that just you know
incremental changes for example sparcity
and things like that um that are still
kind of same Transformer also bring
revolutions to I I don't magic cancer
but is there some bottleneck for like
reasoning kind of questions bottleneck
using this Transformers um I mean
there's been lots of theoretical work
showing the limitations of Transformers
and you know can't do this kind of thing
this many layers and things like that
um I I I don't know how to extrapolate
that to
like contemporary Transformers that
usually don't meet the assumptions of
the theoretical Works um so may not
apply
but um I probably HED my butts and try
other architectures all being
equol thank
[Laughter]
you Google has a Google Glass but now
Apple has Vision Pro um I I think Google
Glass may be a little bit early would
because they like try that another shop
um yeah like I messed up Google
Glass no no but I I I feel like I made
some bad decision it yeah it was for
sure early and early in two senses of
the word uh maybe early in the overall
evolution of Technology but also I think
I like in hindsight I tried to push it
as a product when it itself was sort of
more of a prototype and I should have
set those expectations around it um and
I personally didn't know much about sort
of consumer harder Supply chains back
then and a bunch of things I wish i' had
done differently um but I personally am
still a fan of kind of the lightweight
kind of minimal display that that
offered that you could just like wear
all day versus the big heavy things that
we have today um that's my personal
preference uh but the the Apple vision
and the Oculus for the matter they're
very impressive like having played with
them
um I mean I'm just impressed with what
you can have in front of your screen but
that wasn't what I was personally going
for back then um yes ma um so do you see
um Gemini expanding capabilities into
like 3D head down the line of spatial
Computing in general uh or simulation of
the world in general of that and
especially Beyond Google who already
have several product that's really in
the area right like Google MTH stet view
air core all of that do you see all of
those have some synergies between them
wow that's a good question to be honest
I haven't thought about it but now that
you say it yeah there's no reason we
can you know put in more sort of three
like it's kind of another mode you know
3D data um so probably something
interesting would happen I mean I I
don't see why you
wouldn't uh try to put that into a model
that's already I've got all the smarts
of the text model now can turn on
something else
too and by the way maybe somebody's
doing it at Gemini I don't know oh
yes I'm noty to it or I forgot about
doesn't stop happen okay yes question
the back there are you optimistic that
we be able to re in text generating
models ability to hallucinate and what
do you think about the ethical issue of
potentially
spreading uh problem right now um no
question about it um I mean we have made
them hallucinate less and less over
time
um but I would definitely be excited to
see a breakthrough that brings it to
near zero um I don't you know that's not
you can't just like count on
breakthroughs um so I think we're going
to keep going the incremental kinds of
things that we do to just like bring all
the H stations down down down over time
like I said I think breakthrough would
be good um misinformation
you know misinformation is a complicated
issue I think um I mean obviously you
don't want your AI Bots to be just like
making stuff up um
but they can also be kind of tricked
into like I me there's a lot of I
guess complicated uh
political issues in terms of what people
consider what different people consider
misinformation versus not and it gets
into kind of a broad social debate
um I suppose another thing you could
consider is about them sort of
deliberately generating this information
on the behalf of another actor
um from that point of view I mean
unfortunately it's like it's very easy
to make a lousy AI um like one that
hallucinates a lot um and you can make
you know any open source text model and
probably tweak it to generate
misinformation of all kinds and if
you're not concerned about
um um you know the accuracy it's just
like kind of an easy thing to do so I
don't know I I guess now I think about I
mean detecting AI generated content is
an important field and something that we
work on and so forth so you at least can
maybe tell if something coming at you
was AI generated
yeah Alexandra so the CEO of Nvidia said
that basically the future of writing
code as a career is
[Laughter]
dead okay
yeah um I mean it's
um like we don't know where the future
AI is going broadly I wouldn't you know
we don't know you know it seems to help
across a range of many careers whether
it's graphic artists or customer support
or doctors or um or Executives or you
know what have you um I mean so I don't
know that I would be like singling
out um programming in particular um it's
it's actually probably one of the more
challenging tasks for an llm
today but if you're talking about for
you know decades in the future what
should you be kind of preparing for and
so forth I mean it's it's hard to say I
mean the AI could get quite good
programming but you can say that about
kind of any field of human
endeavor so I guess I probably wouldn't
have singled that out as like saying
don't study specifically programming um
I don't know if that's
answer okay hand the
back lot people start these agents to
write I'm wondering how that's going to
impact it security You could argue that
like the code might become worse or like
less check for certain issues or you
could argue that like we're get better
at invting test Suites which cover all
the cases what are your opinions on this
like is maybe for the outage programmer
like it security way to go because like
the code is going to be Britten but
someone still needs to check it
oh wow you guys are all trying to choose
career based
on I don't know I think you should use a
fortune teller for that
General line of
questions but I I do think
that you know using an AI today to write
let's say unit tests is pretty
straightforward yeah like that's the
kind of thing the AI does really quite
well
uh so I guess my hope is
that AI will make code more secure not
less secure I mean it's kind of it's
usually insecurity is to some extent the
effect of people being lazy and the one
thing that AI is kind of good at is you
know not being
lazy so if I had to bet I would say
there's probably a net benefit to
security with
AI um but I wouldn't discourage you from
pursuing clear and I Security based on
that I think pretty
much um
okay do you want to build AGI do I
want
yeah yeah yeah I mean I think it's um
you know different people mean different
things about that but uh to me the
reasoning aspects are really exciting
and amazing and um you know I kind of
came out of retirement just because of
the Vector of AI is so exciting and as
computer scientists just seeing what
these models can do year after year it
is astonishing so yes any efforts on
like humanoid robotics or these because
there was so much progress in Google X
like in 2015 16 oh humanoid robotics um
boy we've done a lot of humanoid
robotics over the years and sort of
required and they sold a bunch of
companies humanoid robotics um and now
there are bilian sorry not there quite a
few companies doing humanoid Robotics
and internally we still have groups that
work on Robotics and
varying uh varying forms so what are my
thoughts about that I don't know you
know in general I worked on X prior to
this sort of new AI wave and that there
the focus was more Hardware projects for
sure uh but honestly I guess I found a
the hard way open
Hardware is much more difficult um kind
of on a technical basis on business
basis and every way so I'm not
discouraging people from doing it we
need people for sure to do it um at the
same time while the software and the AIS
are getting so much faster at such a
high
rate I guess to me that feels like
that's kind of the rocket ship um and I
feel like if I get distracted in a way
by making hardware for today's
AIS um that might not be the best of use
times compared
to what is the next kind of level of AI
going to be able to support and for that
matter we'll it design a robot for
me that's my person there are a bunch of
people at the Google and alphabet who
can work on hard yes thanks uhing
advertising R is really important for's
your how advertising will be
disrupted way the question about
advertising yeah I um of all people not
too terribly concerned about business
model shifts I mean I think it's a
little bit
um I think it's wonderful that we've
been now for 25 years or whatever uh
able to give just world class
information um search uh for free to
everyone and that's supported by
advertising um which in my mind is great
it's great for the world you know uh
well you know kid in Africa has just as
much access to basic information as the
President of the United States or what
have you um so that's good um at the
same time I expect business models are
going to evolve over time and uh and
maybe they'll still be advertising
because whatever the advertising kind of
works better um the AI is able to tailor
it better or you like it but even if it
happens to move to you know now we have
u g Advanced um other companies have
their you know paid models I think the
fundamental issues that you're
delivering a huge amount of value you
know displacing all the mental effort
that would have been you know required
to take the place of that AI um whether
in your time or labor or what have you
is enormous um and the same thing was
true in search so I personally feel as
long as there's huge value being
generated we'll figure out the business
models
C
model third party cookie you know I'm
going
to how naive I am about to detail I mean
I vaguely am aware uh of that stuff but
I don't um I know I can't think of how
those things interact I'm s oh okay well
you maybe you should answer the
question um how many more do you want
two okay two more questions where do you
see Google search going where do I see
Google search going well it's a super
exciting time uh for search because your
ability
to answer questions will AI is just so
much greater um I think it's the bigger
opportunity is in
situations where you are uh recall
limited uh more so like you might ask a
very specialized question or it's
related to your own personal situation
in a way that nobody out there you know
on the internet has already written
about you know for the questions that a
million people have written about
already and thought deep ple about it's
probably not as big a deal but the
things that are very specific to what
you know you might care about right now
in a particular way that's a huge
opportunity and um you know you can
imagine all kinds of products in your
eyes and different ways to deliver that
uh but basically AI is the nebor are
just doing much better job in that case
okay last question okay who's going to
get the last question is it a good one
who's got a good one in the back you
have to be conf
so for more
what morality mortality
[Laughter]
oh
look I I I'm probably not as well versed
as all of you are to be honest but uh
I've definitely
seen the uh kind of the the molecular AI
make huge amounts of
progress um you could imagine that there
would also be a lot of progress maybe
haven't seen yet on the epidemiology
side of things to just be able to get
kind of I don't know more honest better
controlled a kind of
broader understanding what's happening
to People's Health around the world um
but yeah what can good answer on the
last one um I don't know I don't have
like a really brilliant immortality key
byi just like that but uh you know it's
the kind of field that for
sure benefits from AI whether you're a
researcher or like you know I want it to
just summarize articles to me that one
uh but in the future you know I would
expect the AI would actually give you
novel hypotheses to test uh it does that
today with the alpha folds of the world
but maybe in more complex systems than
just
molecules okay amazing thank you thank
[Applause]
you yeah I think uh really hum to have
you here
Просмотреть больше связанных видео
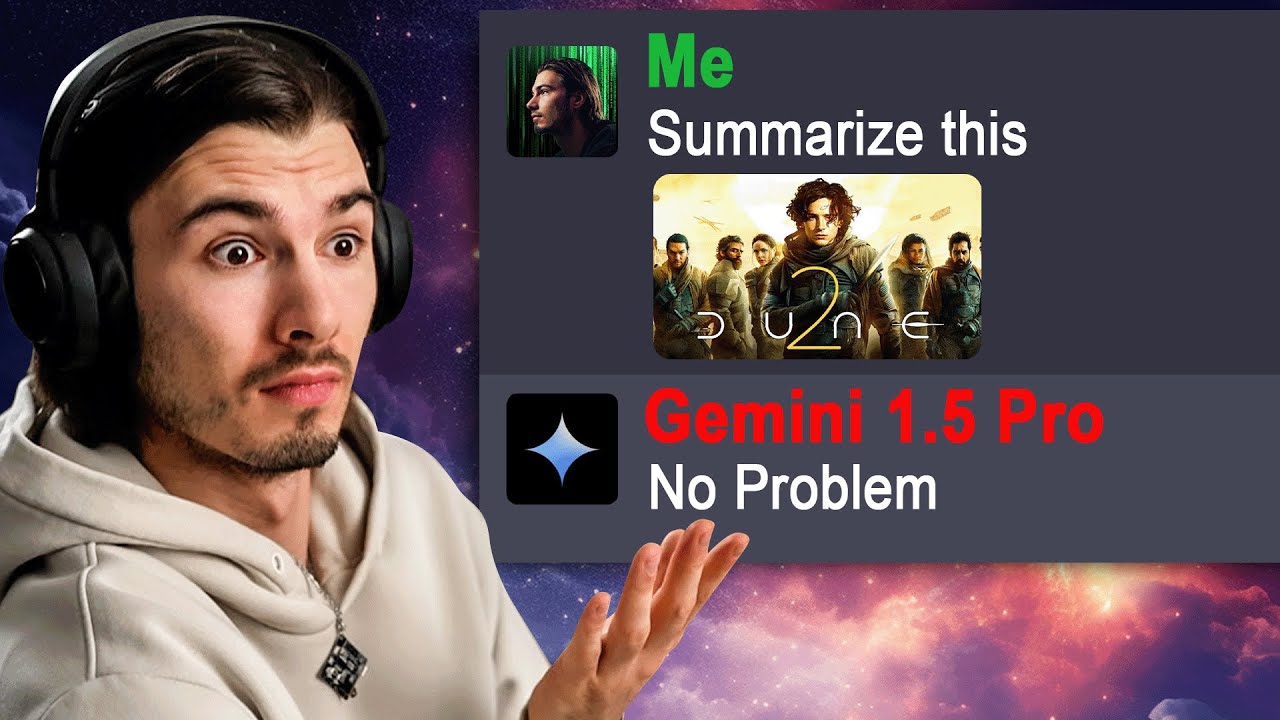
The First AI That Can Analyze Video (For FREE)
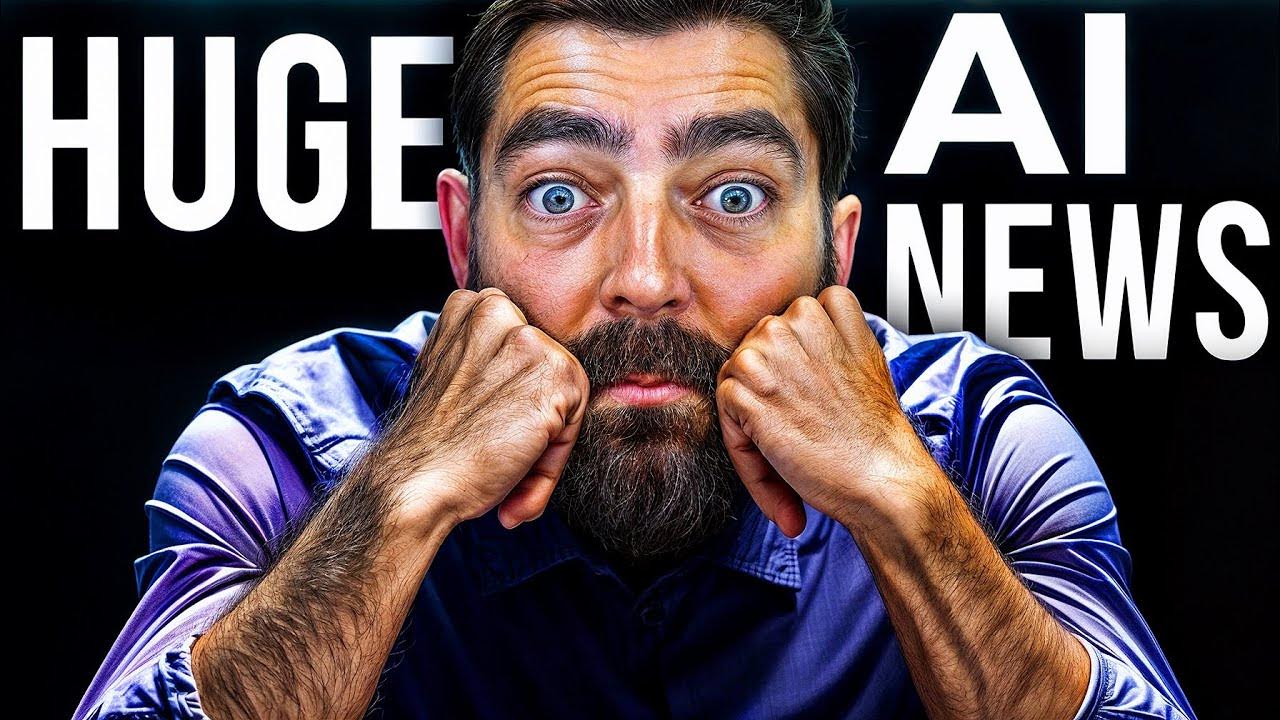
AI News: This Was an INSANE Week in AI!

OpenAI released their new text-to-video model called Sora which generates the best video I've seen!
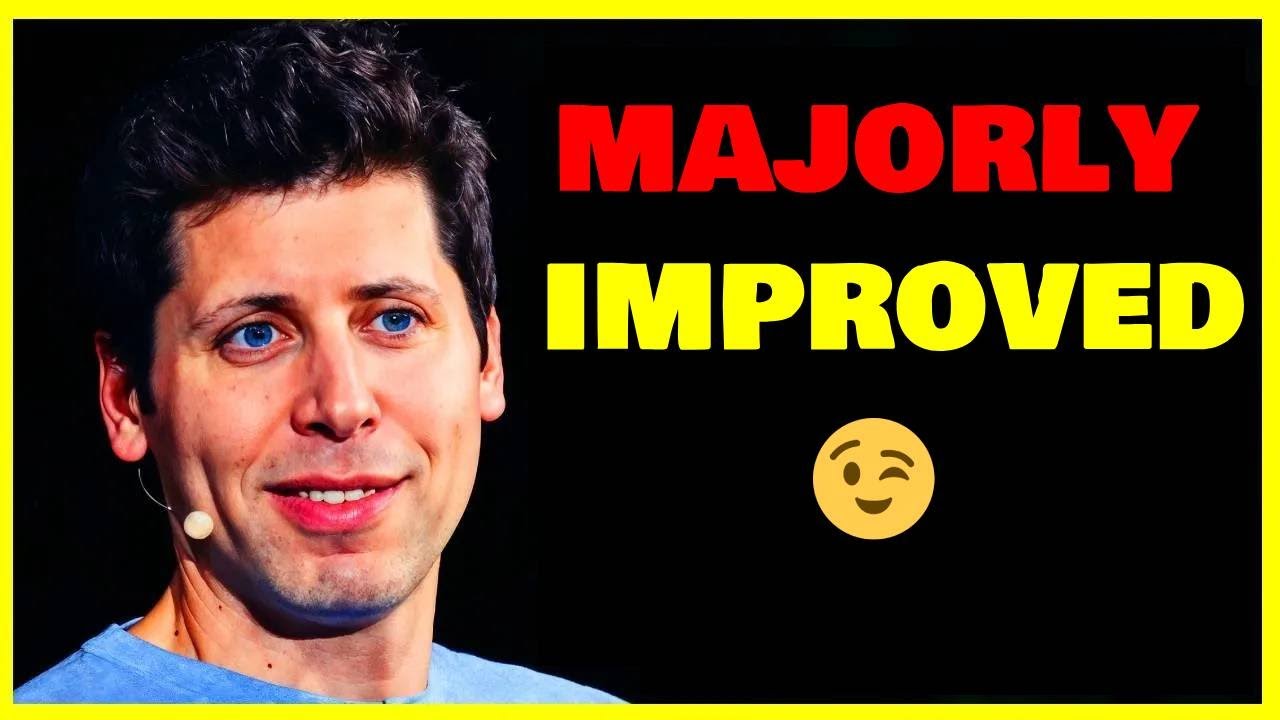
SHOCKING New AI Models! | All new GPT-4, Gemini, Imagen 2, Mistral and Command R+
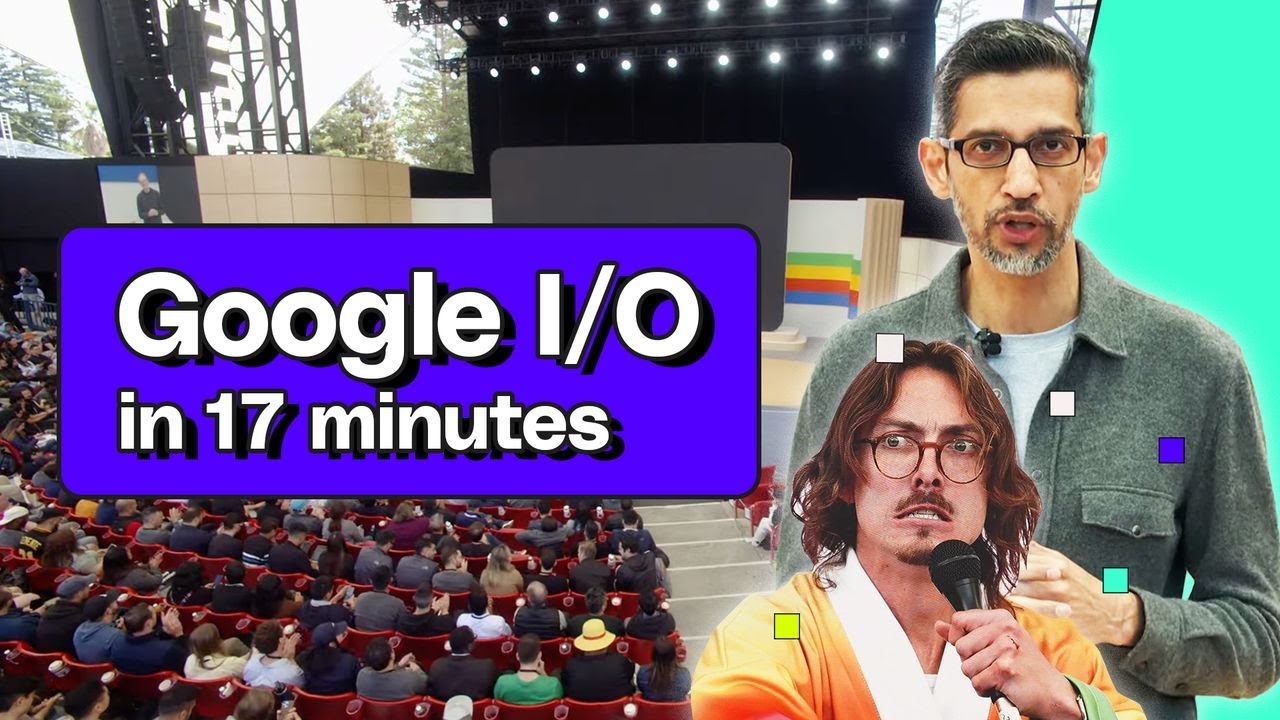
Google I/O 2024 keynote in 17 minutes
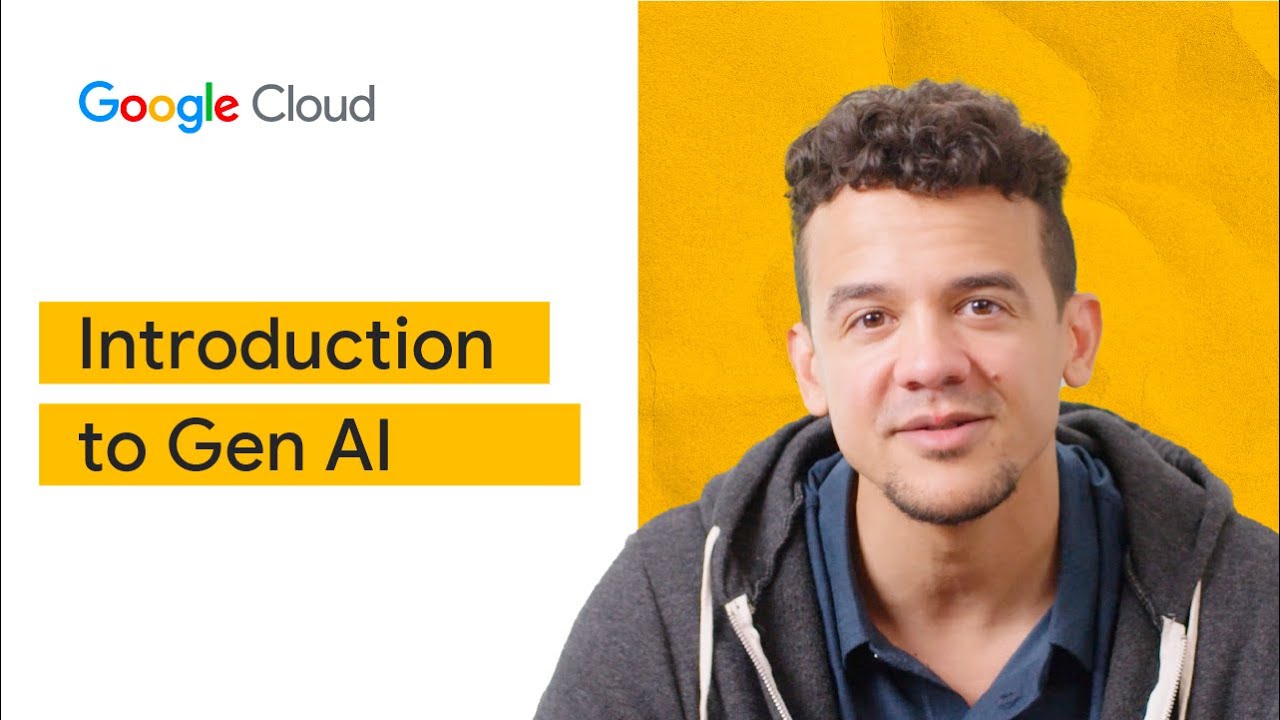
Introduction to Generative AI
5.0 / 5 (0 votes)