MINI-LESSON 8: Power Laws (maximally simplified)
Summary
TLDRThis lecture delves into the concept of power laws and the Pareto distribution, illustrating their prevalence through the 80/20 rule. It contrasts power laws with Gaussian and sub-exponential distributions, highlighting their unique properties like constant ratio in tail behavior and scale-free nature. The speaker explains the implications of power laws in real-world scenarios, such as wealth distribution and company sizes, emphasizing their intuitive understanding despite the absence of traditional statistical measures like mean and variance in certain cases.
Takeaways
- 📚 The lecture discusses the concept of power laws and the Pareto distribution, introducing them in the context of different distribution classes.
- 🔍 The Pareto principle, often cited as the 80/20 rule, is highlighted as a real-world example of power law distribution, illustrating how a small percentage of people own a large percentage of resources.
- 📈 Power laws are characterized by a constant ratio in the tail of the distribution, unlike Gaussian distributions where the ratio changes as you move further from the mean.
- 📊 The script differentiates between three main classes of distributions: Gaussian, subexponential, and power laws, each with their own properties and implications for statistical inference.
- 🤔 The Gaussian distribution is safe for statistical inference due to its convergence properties, but power laws present challenges due to their fat tails and lack of traditional statistical measures like mean and variance.
- 📉 Power law distributions are intuitive and can be understood through the concept of recursion, where the same 80/20 rule can be applied repeatedly to smaller subsets.
- 📝 The script explains that power laws can be mathematically expressed as a constant times x to the power of negative alpha for large x, which is key to understanding their behavior.
- 📊 The log-log plot is a useful tool for identifying power laws, as it should show a straight line with a slope of alpha for distributions that follow a power law.
- 🔢 The 'alpha' parameter in power laws determines the thickness of the tail; lower alpha values indicate fatter tails and higher values result in thinner tails approaching Gaussian behavior.
- 🚫 Power laws with alpha less than or equal to one have no mean, and those with alpha less than or equal to two have no variance, challenging traditional statistical analysis.
- 🌐 The lecture concludes by emphasizing the importance of understanding power laws for statistical inference and for developing a more empirical and mathematically solid view of the world.
Q & A
What is the Pareto Principle often associated with?
-The Pareto Principle is often associated with the idea that 80% of the effects come from 20% of the causes, specifically in the context of wealth distribution where 20% of the people own 80% of the land.
What does the term 'fractal' imply in the context of the Pareto Principle?
-In the context of the Pareto Principle, 'fractal' implies that the 80/20 distribution can be recursively applied at different levels, such as within the 20% itself, leading to further refinements like 50% of the land being owned by 1% of the people.
What are the three main classes of distributions mentioned in the script?
-The three main classes of distributions mentioned are Gaussian (including lower Gaussian like binomial and upper Gaussian like the actual Gaussian distribution), subexponential (including rank and log normal distributions), and power laws (including Pareto, Student's t, and stable distributions).
What is special about the probability ratios in a power law distribution compared to a Gaussian distribution?
-In a power law distribution, the probability ratios are constant regardless of the value of x, whereas in a Gaussian distribution, these ratios change as x increases, showing an acceleration of decline but not maintaining a constant ratio.
Why is the Gaussian distribution considered safe for statistical inference?
-The Gaussian distribution is considered safe for statistical inference because, under the central limit theorem, the sum of a large sample tends to converge to a Gaussian distribution, allowing for more predictable and reliable statistical analysis.
What does the term 'thick tail' or 'semi-fat tail' refer to in the context of distributions?
-In the context of distributions, 'thick tail' or 'semi-fat tail' refers to distributions that have heavier tails than Gaussian distributions but do not follow a power law, meaning that extreme events are less likely but not as rare as in a Gaussian distribution.
How is the concept of 'scale and variance' related to power law distributions?
-The concept of 'scale and variance' in power law distributions refers to the property that the ratio of exceedance probabilities remains constant regardless of the scale of x, indicating a consistent pattern of distribution without a specific mean or variance.
What does the 'Lindy effect' imply about the life expectancy of non-perishable items or concepts?
-The Lindy effect implies that the life expectancy of non-perishable items or concepts is proportional to their current age, suggesting that the longer something has already existed, the longer it is expected to continue to exist.
How does the script differentiate between Gaussian and power law distributions in terms of life expectancy?
-The script differentiates by stating that in Gaussian distributions, life expectancy shrinks as the age increases beyond certain thresholds, while in power law distributions, life expectancy remains constant or even increases, reflecting the 'Lindy effect'.
What mathematical property characterizes power law distributions?
-Power law distributions are characterized by the property that the probability of exceeding a value x, for large x, can be approximated as a constant times x to the power of negative alpha, which is indicative of the distribution's tail behavior.
What are the implications of different alpha values in power law distributions?
-Different alpha values in power law distributions indicate the fatness of the tail: lower alpha values result in fatter tails (more extreme events), while higher alpha values result in thinner tails. Alpha equal to one indicates a Cauchy distribution with no mean, and alpha values of two or lower imply no variance.
Outlines
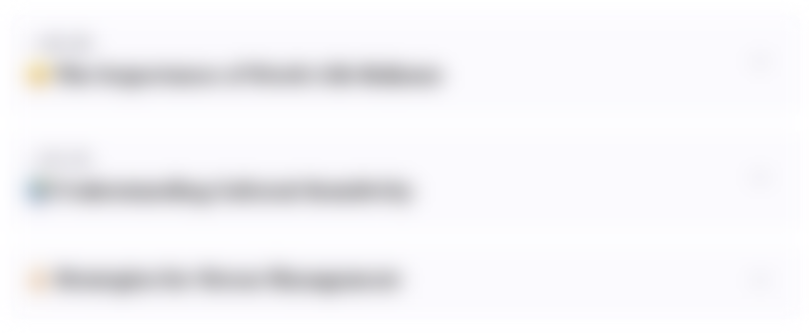
このセクションは有料ユーザー限定です。 アクセスするには、アップグレードをお願いします。
今すぐアップグレードMindmap
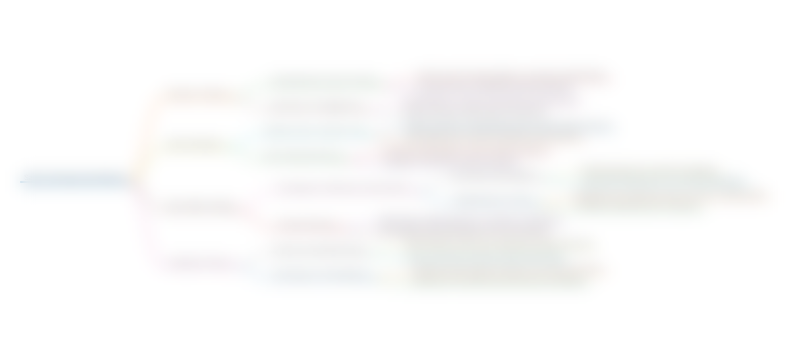
このセクションは有料ユーザー限定です。 アクセスするには、アップグレードをお願いします。
今すぐアップグレードKeywords
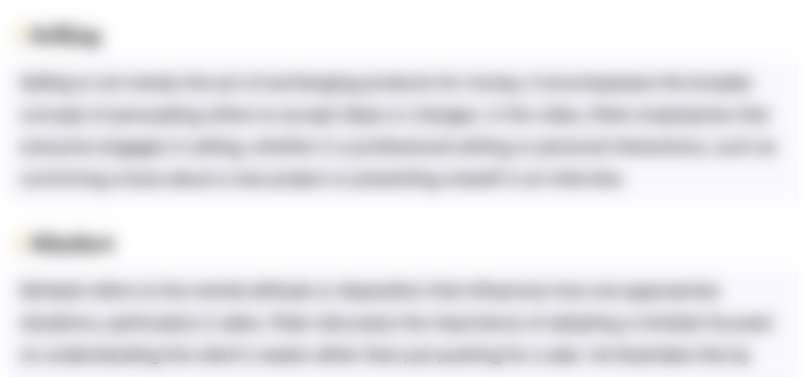
このセクションは有料ユーザー限定です。 アクセスするには、アップグレードをお願いします。
今すぐアップグレードHighlights
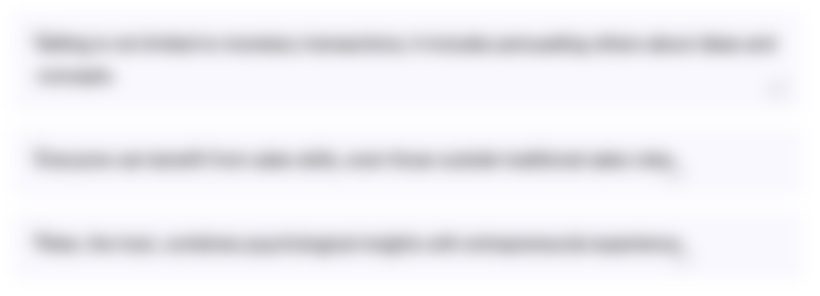
このセクションは有料ユーザー限定です。 アクセスするには、アップグレードをお願いします。
今すぐアップグレードTranscripts
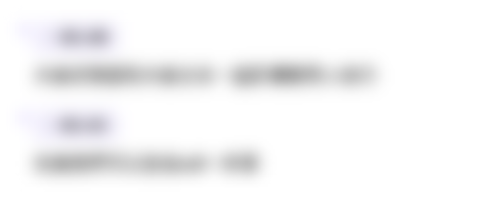
このセクションは有料ユーザー限定です。 アクセスするには、アップグレードをお願いします。
今すぐアップグレード5.0 / 5 (0 votes)