Maximum Likelihood, clearly explained!!!
Summary
TLDRIn this engaging video from Stack Quest, viewers are introduced to the concept of maximum likelihood estimation (MLE) through the analysis of mouse weights. The presenter explains the significance of fitting distributions, particularly the normal distribution, to observed data. By shifting the mean and standard deviation, the optimal values that maximize the likelihood of the observed weights are determined. The video highlights the differences between 'probability' and 'likelihood' in statistical terminology. Overall, it serves as an informative guide to understanding how MLE is used in statistical modeling, making complex concepts accessible and interesting.
Takeaways
- 😀 Maximum likelihood estimation (MLE) is used to optimally fit statistical distributions to observed data.
- 📊 Various types of distributions, such as normal, exponential, and gamma, can be applied depending on the data characteristics.
- 🐭 The video uses mouse weights as a practical example to demonstrate how to fit a normal distribution to data.
- 📈 A normal distribution is defined by its mean and standard deviation, with data expected to cluster around the mean.
- 🔍 The likelihood of observing data decreases when the distribution's mean is misaligned with the actual average.
- ➡️ Shifting the normal distribution to align with the observed average increases the likelihood of the measurements.
- 🧮 The process of MLE involves calculating likelihoods as the distribution is systematically shifted across possible means.
- 🌟 The maximum likelihood estimate for the mean is where the likelihood of observing the data is maximized.
- 📏 A similar approach is used to estimate the standard deviation that maximizes the likelihood of the observed weights.
- 📖 In statistics, 'likelihood' refers specifically to the optimal parameter values derived from observed data, differing from general probability.
Q & A
What is the main focus of the video segment?
-The main focus of the video is on maximum likelihood estimation (MLE) and how to fit a normal distribution to a dataset, specifically mouse weights.
Why is it important to fit a distribution to data?
-Fitting a distribution to data simplifies analysis and allows for generalization across experiments of the same type.
What are some types of distributions mentioned in the transcript?
-The transcript mentions several types of distributions, including normal, exponential, and gamma distributions.
What characteristics define a normal distribution?
-A normal distribution is characterized by its symmetry around the mean, with most measurements clustered near the average.
How does shifting the mean of a normal distribution affect likelihood?
-Shifting the mean affects the likelihood of observing the data; the goal is to find the mean that maximizes this likelihood.
What is the maximum likelihood estimate for the mean?
-The maximum likelihood estimate for the mean is the value that maximizes the likelihood of observing the given data.
How do you estimate the standard deviation using MLE?
-To estimate the standard deviation, you plot the likelihood of observing the data against different values for the standard deviation and select the value that maximizes the likelihood.
What distinction does the host make between probability and likelihood?
-The host notes that while probability and likelihood can mean the same in everyday conversation, in statistics, likelihood specifically refers to the context of finding optimal values for distribution parameters given observed measurements.
What is the significance of obtaining maximum likelihood estimates?
-Obtaining maximum likelihood estimates for the mean and standard deviation provides a statistical basis for understanding how well the chosen distribution fits the observed data.
What does the host encourage viewers to do at the end of the video?
-The host encourages viewers to subscribe for more content and to leave suggestions for future topics in the comments.
Outlines
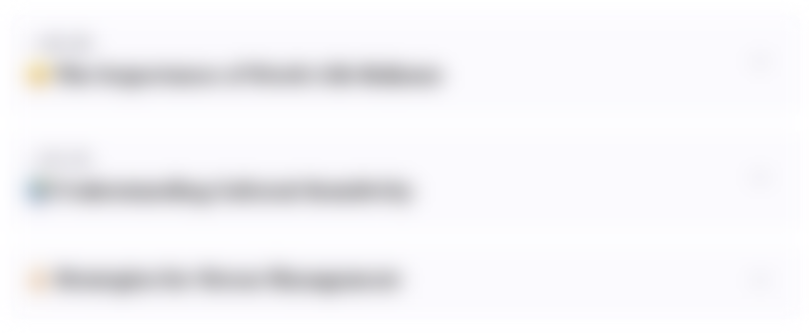
Cette section est réservée aux utilisateurs payants. Améliorez votre compte pour accéder à cette section.
Améliorer maintenantMindmap
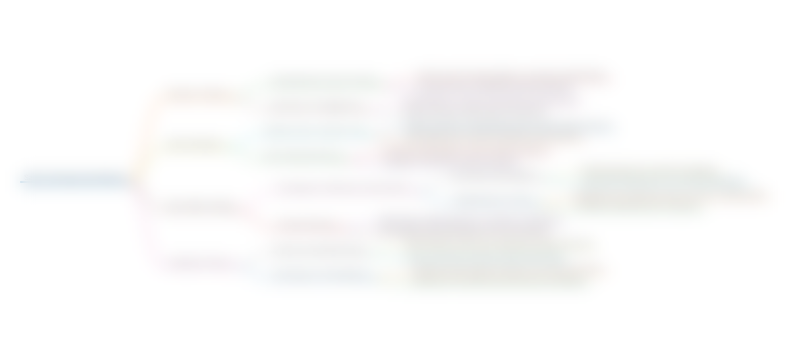
Cette section est réservée aux utilisateurs payants. Améliorez votre compte pour accéder à cette section.
Améliorer maintenantKeywords
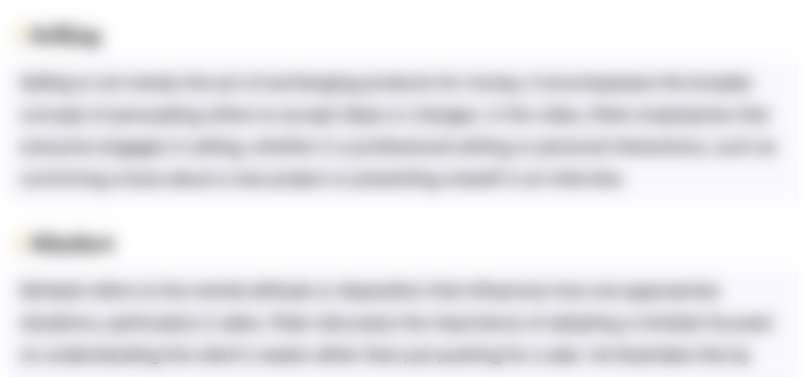
Cette section est réservée aux utilisateurs payants. Améliorez votre compte pour accéder à cette section.
Améliorer maintenantHighlights
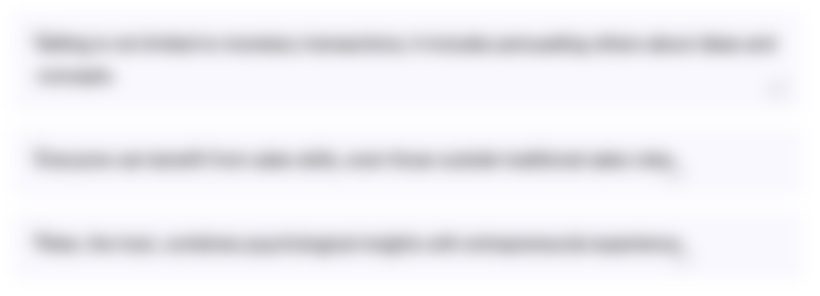
Cette section est réservée aux utilisateurs payants. Améliorez votre compte pour accéder à cette section.
Améliorer maintenantTranscripts
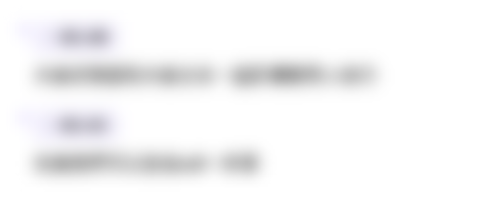
Cette section est réservée aux utilisateurs payants. Améliorez votre compte pour accéder à cette section.
Améliorer maintenantVoir Plus de Vidéos Connexes
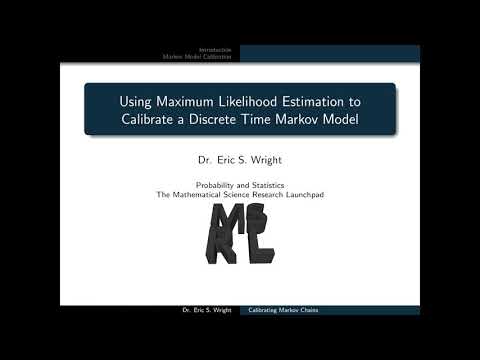
Presentation16: Using Maximum Likelihood Estimation to Calibrate a Discrete Time Markov Model

Probabilistic view of linear regression
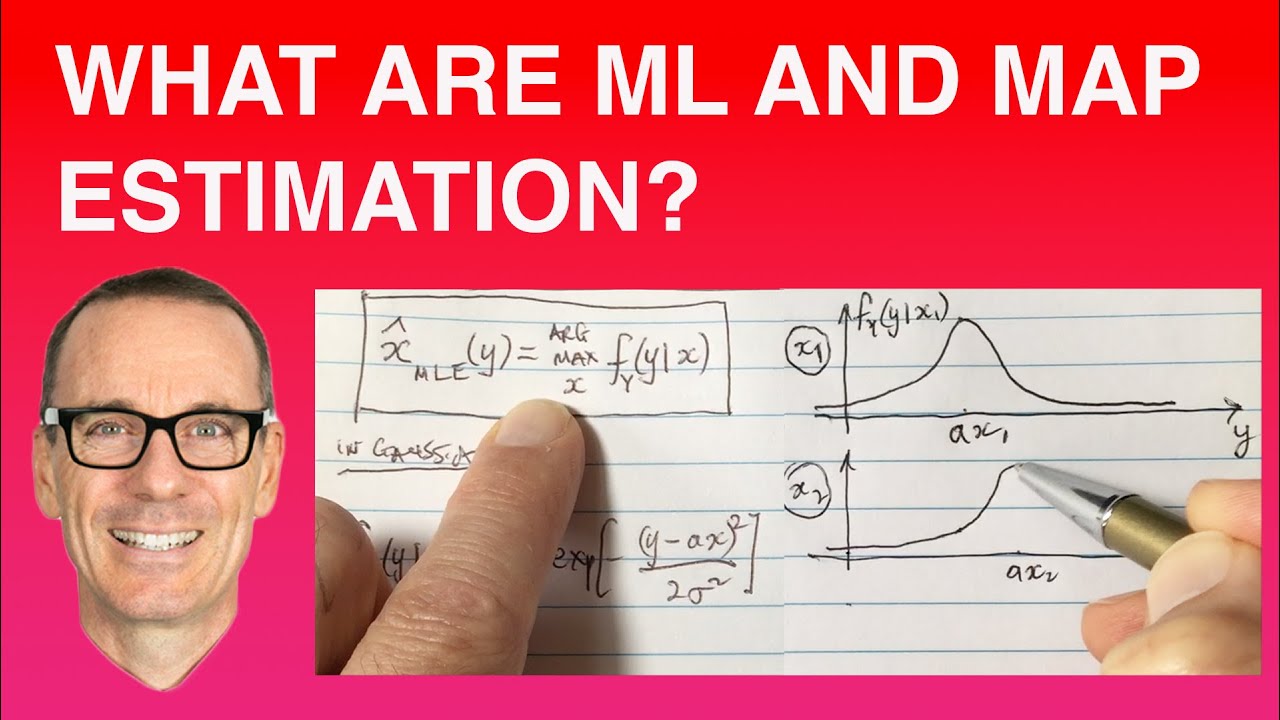
What are Maximum Likelihood (ML) and Maximum a posteriori (MAP)? ("Best explanation on YouTube")
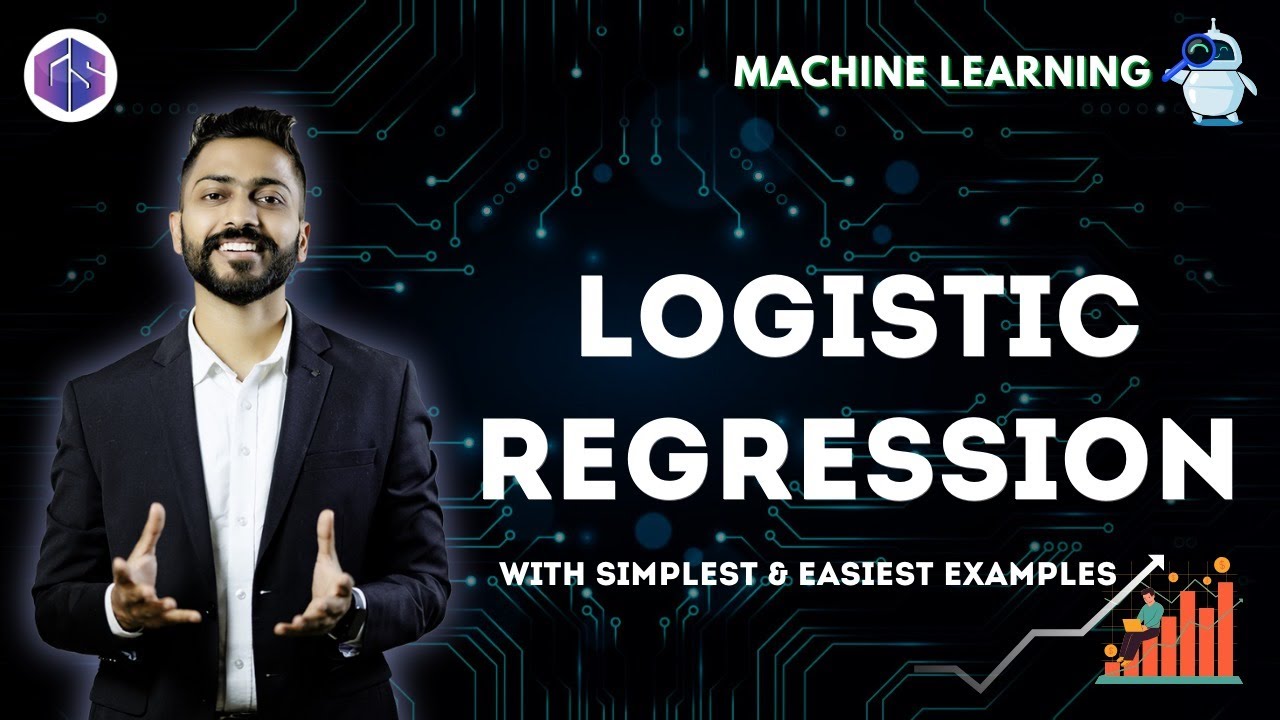
Lec-5: Logistic Regression with Simplest & Easiest Example | Machine Learning
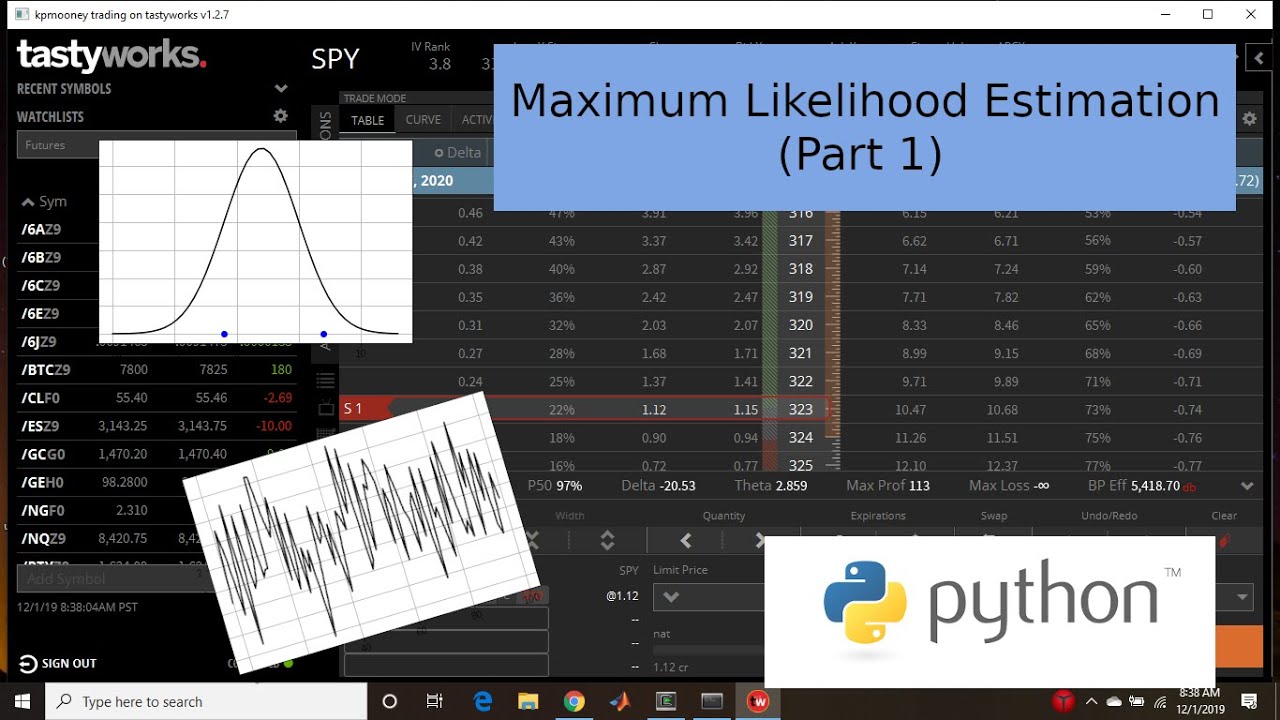
Maximum Likelihood Estimation (Part 1)
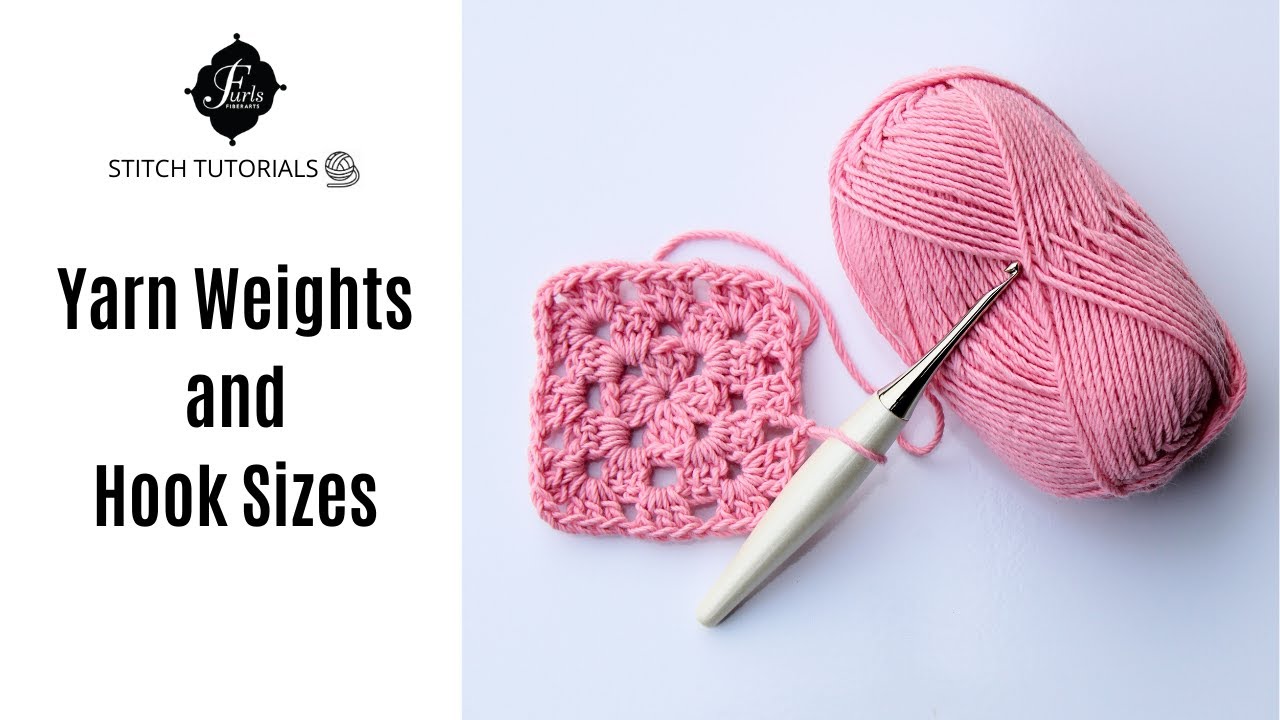
Yarn Weights and Hook Sizes [Crochet Basics]
5.0 / 5 (0 votes)