Using Linear Models for t-tests and ANOVA, Clearly Explained!!!
Summary
TLDRIn this StatQuest episode, the host delves into General Linear Models, focusing on the application of linear regression techniques to perform t-tests and ANOVA. The concept of a design matrix is introduced, allowing for the comparison of means and calculation of p-values to determine significant differences between groups, such as control and mutant mice in gene expression studies. The episode simplifies complex statistical methods, making them accessible for further exploration in future videos.
Takeaways
- 📊 **General Linear Models**: The video discusses the application of general linear models, specifically focusing on linear regression and its extension to T-tests and ANOVA.
- 🧬 **Gene Expression Study**: It uses a study comparing gene expression between control and mutant mice to illustrate statistical concepts.
- 📈 **Design Matrix Introduction**: Introduces the concept of a design matrix, a tool used to expand linear regression techniques to more complex tests.
- 🔍 **T-Test Application**: Explains how to apply linear regression techniques to perform a T-test to compare means between two groups.
- 📉 **Sum of Squared Residuals**: Describes the calculation of the sum of squared residuals around the mean and around the fitted line.
- 📝 **Fitting Lines to Data**: Demonstrates how to fit lines to control and mutant data separately and then combine them into a single equation.
- 🔢 **Calculating F and P Values**: Shows how to calculate F and P values for both linear regression and T-tests using the sums of squares.
- 📚 **ANOVA Test**: Discusses how to perform an ANOVA test to compare more than two categories, using the same principles as for T-tests.
- 🔄 **Design Matrix Variations**: Notes that there are different design matrices that can be used for T-tests and ANOVA, with one being more common than the other.
- 🔄 **Flexibility of Design Matrix**: Emphasizes the flexibility of the design matrix to allow for computer-based solutions to statistical problems without manual calculations.
Q & A
What is the main focus of the StatQuest video?
-The main focus of the StatQuest video is to explain how to apply linear regression techniques to perform T-tests and ANOVA using a design matrix.
What is a design matrix?
-A design matrix is a concept used in statistics, particularly in the context of general linear models, to represent the structure of the data and how different variables relate to each other.
What was the goal of the T-test discussed in the video?
-The goal of the T-test discussed in the video was to compare gene expression between control mice and mutant mice to see if their means are significantly different.
What does 'mutant mice' refer to in the context of the video?
-In the context of the video, 'mutant mice' refers to normal mice that have a specific gene that has been knocked out and is no longer functioning correctly.
How is the mean used in the context of a T-test?
-In the context of a T-test, the mean is used as the least squares fit to the data, which is a horizontal line that intercepts the Y-axis at the mean value for each group.
What is the purpose of calculating the sum of squared residuals around the mean?
-Calculating the sum of squared residuals around the mean helps to determine the variability of the data points relative to the overall mean, which is a step in both linear regression and T-tests.
How does the design matrix help in calculating F and the P-value?
-The design matrix helps in calculating F and the P-value by providing a way to combine the data and parameters into a single equation, allowing for a unified approach to statistical analysis.
What is the difference between the design matrix used in the video and the standard design matrix for T-tests?
-The design matrix used in the video is a simplified version created for the purpose of the tutorial, while the standard design matrix for T-tests is more commonly used in practice and may include more complex structures.
Why is it important to be able to calculate P-values for T-tests and ANOVA?
-Being able to calculate P-values for T-tests and ANOVA is important because it allows researchers to determine if observed differences between groups are statistically significant and not due to chance.
What is the significance of the P-value in statistical tests?
-The P-value in statistical tests indicates the probability of obtaining results at least as extreme as the observed results, assuming the null hypothesis is true. A low P-value suggests that the results are significant and not due to chance.
How does the video demonstrate the application of linear regression to T-tests?
-The video demonstrates the application of linear regression to T-tests by showing how the same techniques used to calculate P-values in linear regression can be adapted to perform T-tests by using a design matrix to fit lines to the data and calculate residuals.
Outlines
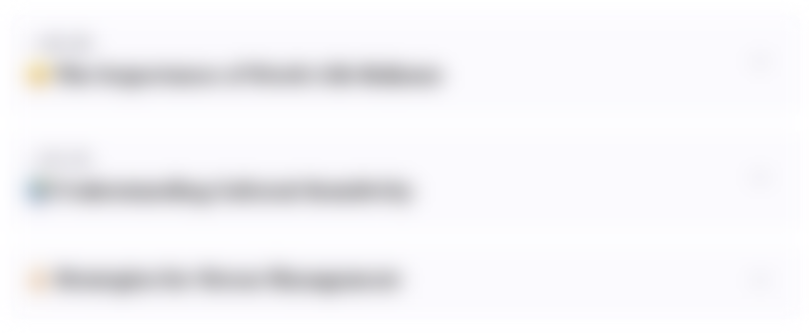
Cette section est réservée aux utilisateurs payants. Améliorez votre compte pour accéder à cette section.
Améliorer maintenantMindmap
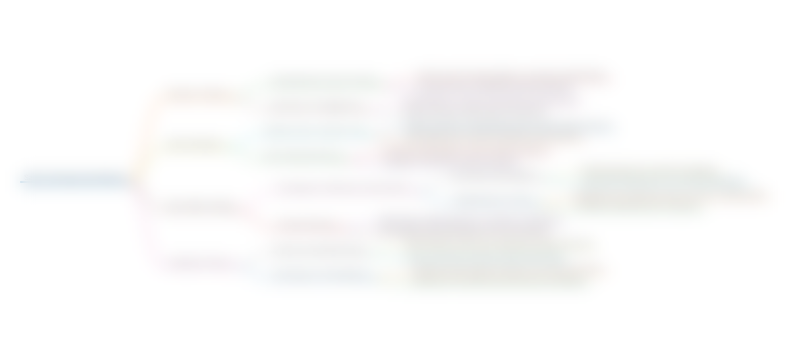
Cette section est réservée aux utilisateurs payants. Améliorez votre compte pour accéder à cette section.
Améliorer maintenantKeywords
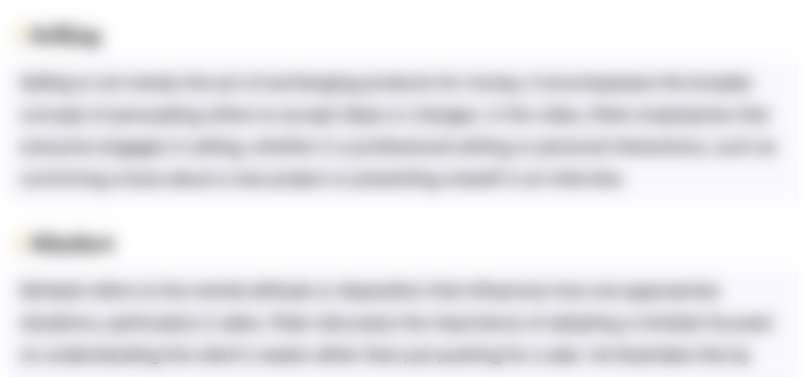
Cette section est réservée aux utilisateurs payants. Améliorez votre compte pour accéder à cette section.
Améliorer maintenantHighlights
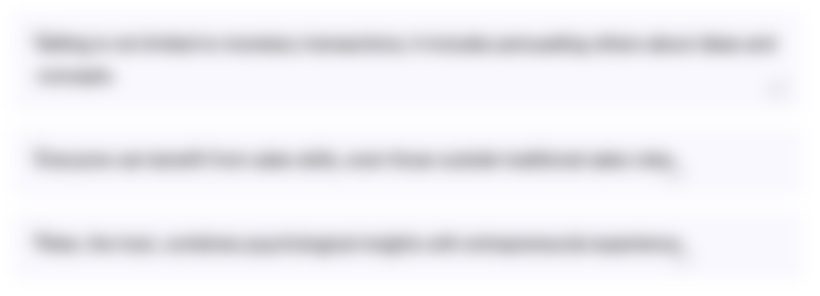
Cette section est réservée aux utilisateurs payants. Améliorez votre compte pour accéder à cette section.
Améliorer maintenantTranscripts
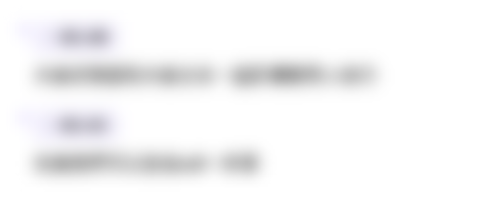
Cette section est réservée aux utilisateurs payants. Améliorez votre compte pour accéder à cette section.
Améliorer maintenantVoir Plus de Vidéos Connexes
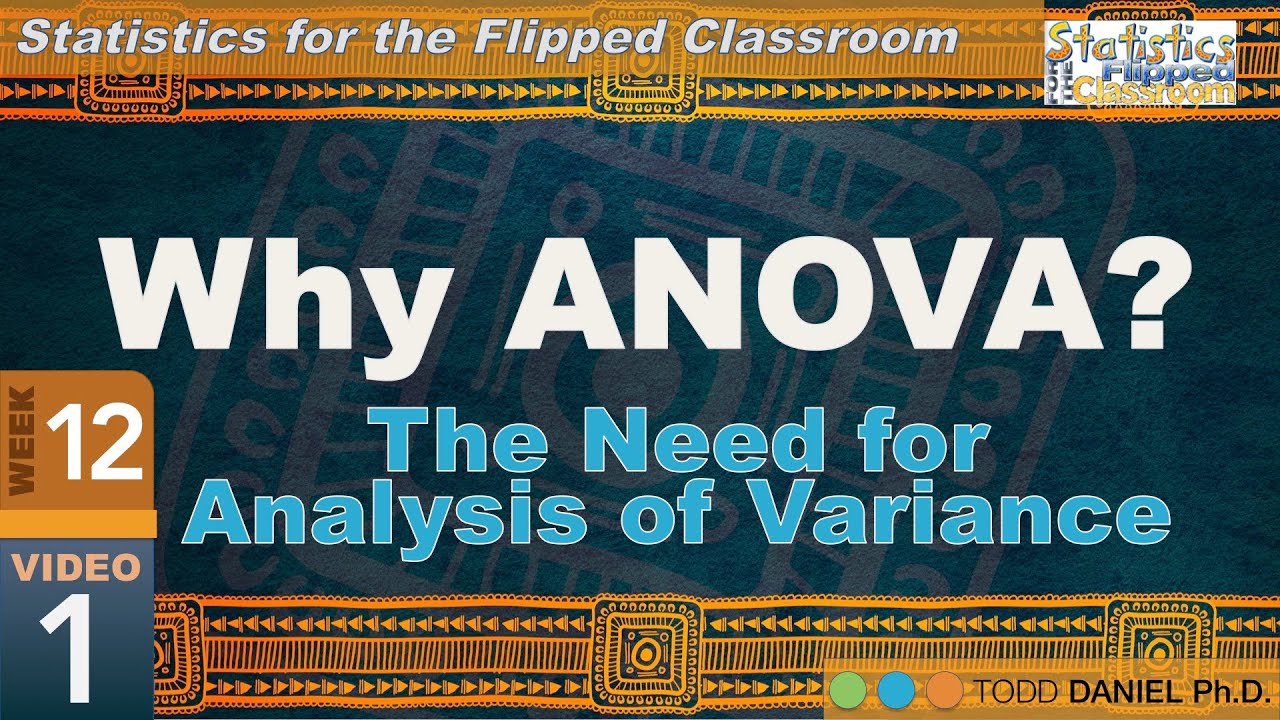
A Gentle Introduction to ANOVA – The Problem of Probability Pyramiding (12-1)
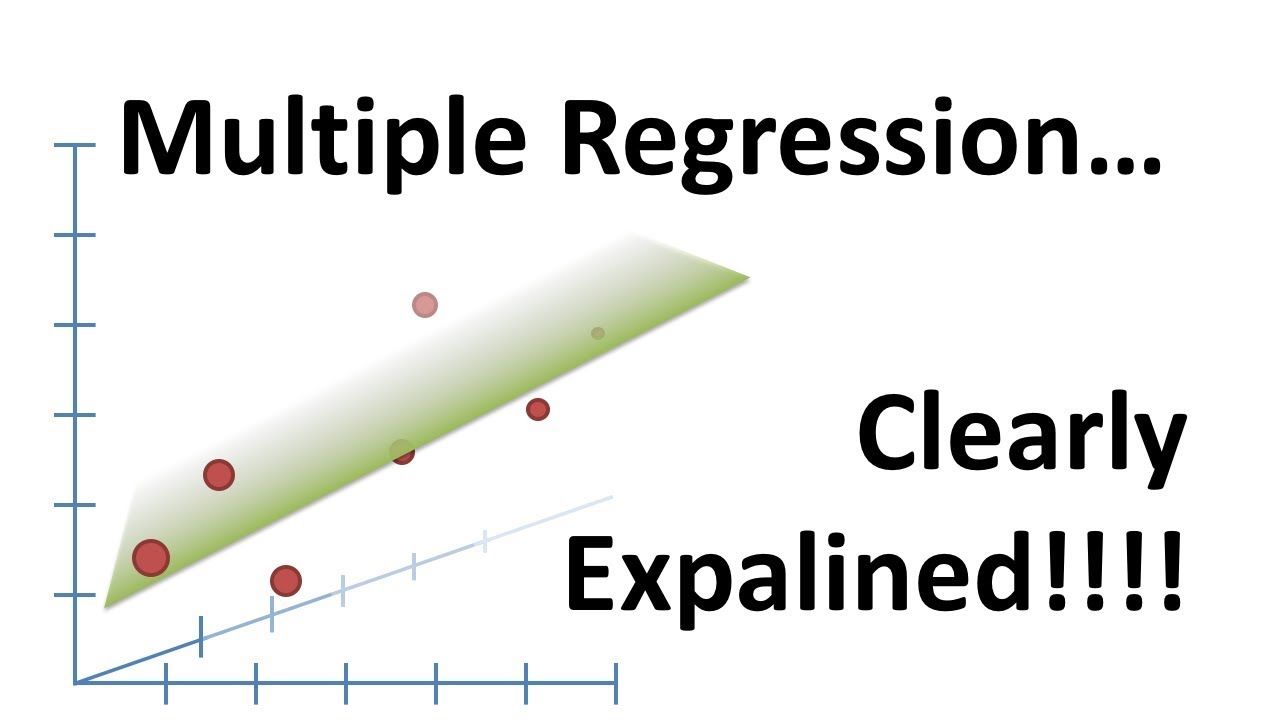
Multiple Regression, Clearly Explained!!!
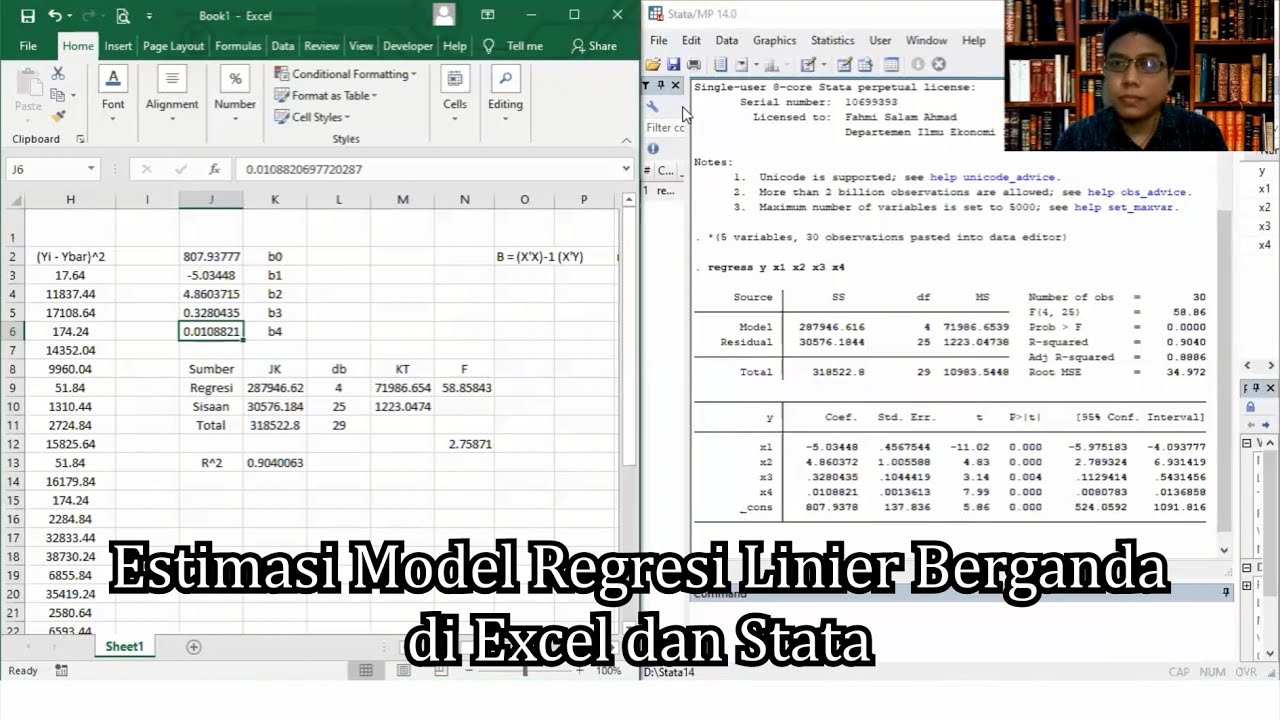
Praktikum Ekonometrika I P4 - Regresi Linier Berganda

Machine Learning Fundamentals: Bias and Variance
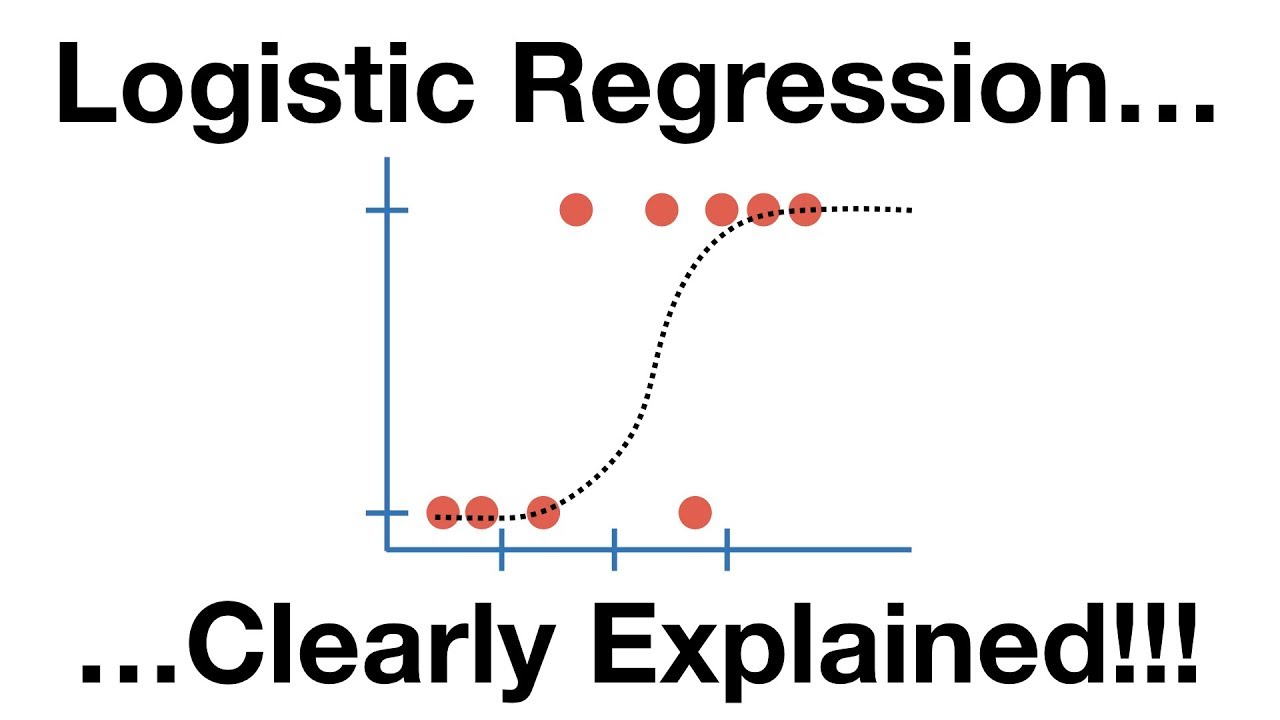
StatQuest: Logistic Regression

PERBEDAAN UJI REGRESI LOGISTIK DAN UJI REGRESI LINEAR | PENJELASAN MUDAH DIPAHAMI MAHASISWA
5.0 / 5 (0 votes)