Sample and Population in Statistics | Statistics Tutorial | MarinStatsLectures
Summary
TLDRThis video script delves into the concepts of samples and populations in statistics. It uses examples of disease prevalence and height to illustrate how sample proportions and means can be used to estimate population parameters. The script explains the use of bar plots and histograms to visualize data distribution and introduces the binomial and normal distributions to model theoretical probability distributions of populations. The goal is to understand how to make statistical inferences about populations based on sample data.
Takeaways
- 🔍 The video discusses the concepts of samples and populations in statistics.
- 📊 A sample is a subset of a population used to estimate characteristics of the whole population.
- 📈 The categorical variable 'X' in the sample is used to record the presence of a disease, summarized by a sample proportion (P-hat).
- 📉 A bar plot is used to visualize the distribution of the sample data, showing the probability of having the disease.
- 🧬 The true probability of having the disease in the entire population can be modeled using a theoretical probability distribution.
- 📚 The binomial distribution is introduced as a model for the population, with parameters n (number of trials) and p (probability of success).
- 📊 For numeric variables like height, the sample mean and standard deviation are calculated to summarize the data.
- 📊 A histogram or box plot is used to graphically summarize the distribution of the numeric variable in the sample.
- 📊 The population's distribution of height is assumed to be normally distributed with a mean of 175 cm and a standard deviation of 10 cm.
- 🔮 The normal distribution is used to describe the theoretical distribution of the entire population's height.
- 🔍 Understanding the population's true distribution helps in making statistical inferences from sample data.
Q & A
What is the main focus of the video?
-The video focuses on explaining the concepts of samples and populations in statistics, and how they are connected.
What is a sample in the context of the video?
-A sample refers to a subset of individuals taken from a larger population for the purpose of analysis, such as recording whether they have a particular disease.
How is the presence of disease in the sample quantified?
-The presence of disease in the sample is quantified using a sample proportion, which is calculated as the number of individuals with the disease divided by the total number in the sample.
What is the sample proportion if 12 out of 100 individuals have a disease?
-The sample proportion is 0.12 or 12%, indicating that 12% of the sample has the disease.
How is the distribution of a sample represented in the video?
-The distribution of a sample is represented using a bar plot, showing the probability of individuals having or not having the disease.
What is a population in the context of statistics?
-A population in statistics refers to the entire group of individuals or items that are the subject of the study.
How is the true probability of having a disease in the entire population modeled?
-The true probability of having a disease in the entire population is modeled using a theoretical probability distribution, such as a binomial distribution with parameters n (number of trials) and p (probability of success).
What is the difference between a sample mean and a population mean?
-The sample mean is the average of a subset of data from the population, while the population mean is the average of the entire population.
What is the role of the normal distribution in describing population data?
-The normal distribution is used to describe the population data when the data is approximately bell-shaped and symmetrically distributed around the mean.
Why is it important to understand the distribution of a sample?
-Understanding the distribution of a sample is important for making inferences about the population from which the sample was drawn.
What is the purpose of statistical inference?
-The purpose of statistical inference is to make statements about a population based on the data collected from a sample.
Outlines
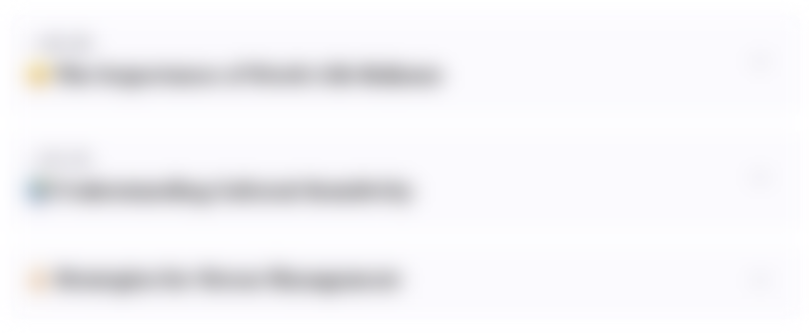
Cette section est réservée aux utilisateurs payants. Améliorez votre compte pour accéder à cette section.
Améliorer maintenantMindmap
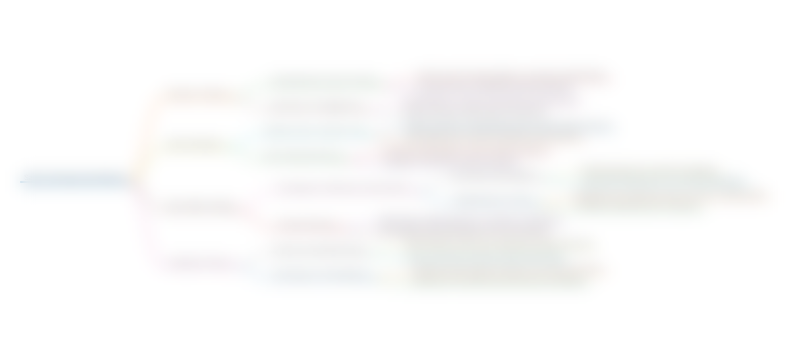
Cette section est réservée aux utilisateurs payants. Améliorez votre compte pour accéder à cette section.
Améliorer maintenantKeywords
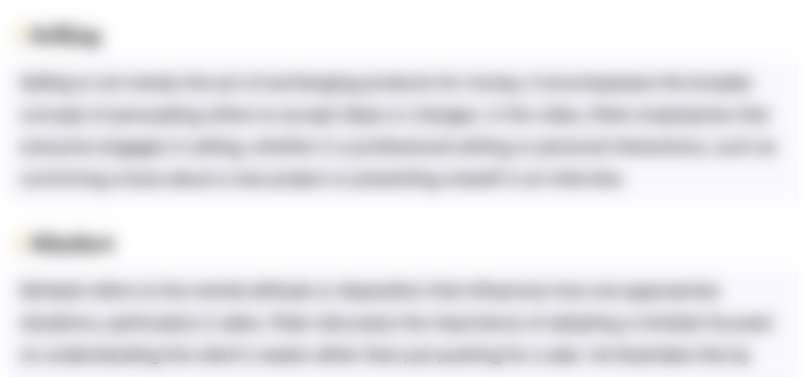
Cette section est réservée aux utilisateurs payants. Améliorez votre compte pour accéder à cette section.
Améliorer maintenantHighlights
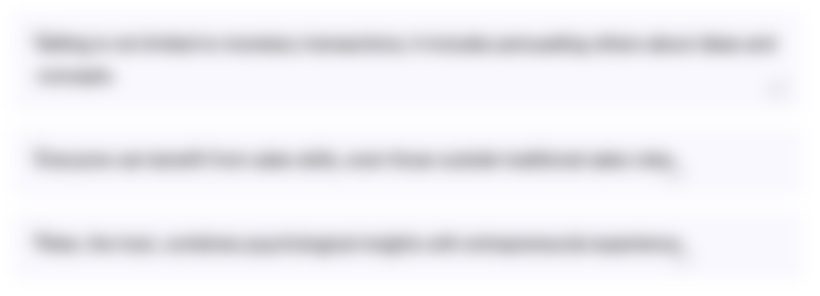
Cette section est réservée aux utilisateurs payants. Améliorez votre compte pour accéder à cette section.
Améliorer maintenantTranscripts
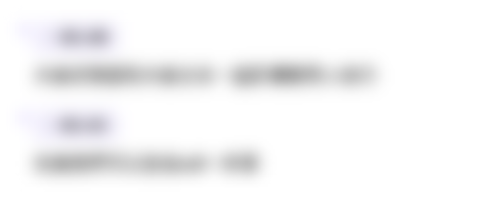
Cette section est réservée aux utilisateurs payants. Améliorez votre compte pour accéder à cette section.
Améliorer maintenant5.0 / 5 (0 votes)